Keywords
Computer Science and Digital Science
- A6.1. Methods in mathematical modeling
- A6.1.1. Continuous Modeling (PDE, ODE)
- A6.1.2. Stochastic Modeling
- A6.1.3. Discrete Modeling (multi-agent, people centered)
- A6.1.4. Multiscale modeling
- A6.2.1. Numerical analysis of PDE and ODE
- A6.2.3. Probabilistic methods
- A6.2.4. Statistical methods
- A6.3.1. Inverse problems
Other Research Topics and Application Domains
- B1.1.2. Molecular and cellular biology
- B1.1.3. Developmental biology
- B1.1.4. Genetics and genomics
- B1.1.5. Immunology
- B1.1.6. Evolutionnary biology
- B1.1.7. Bioinformatics
- B1.1.8. Mathematical biology
- B1.1.10. Systems and synthetic biology
- B2.2.1. Cardiovascular and respiratory diseases
- B2.2.3. Cancer
- B2.2.5. Immune system diseases
- B2.2.6. Neurodegenerative diseases
1 Team members, visitors, external collaborators
Research Scientists
- Mostafa Adimy [Team leader, Inria, Senior Researcher, HDR]
- Samuel Bernard [CNRS, Researcher, HDR]
- Vincent Calvez [CNRS, Researcher, HDR]
- Olivier Gandrillon [CNRS, Senior Researcher, HDR]
- Thomas Lepoutre [Inria, Researcher, HDR]
- Vitaly Volpert [CNRS, Senior Researcher, HDR]
Faculty Members
- Thibault Espinasse [Univ Claude Bernard, Associate Professor]
- Laurent Pujo Menjouet [Univ Claude Bernard, Associate Professor, HDR]
- Leon Matar Tine [Univ Claude Bernard, Associate Professor]
PhD Students
- Ghada Abi Younes [Univ Claude Bernard]
- Kyriaki Dariva [Univ Claude Bernard]
- Leonard Dekens [Univ Claude Bernard]
- Mete Demircigil [École Normale Supérieure de Paris]
- Cheikh Gueye [Senegal]
- Alexey Koshkin [Inria]
- Paul Lemarre [Univ Claude Bernard]
- Elias Ventre [École Normale Supérieure de Lyon]
Interns and Apprentices
- Louis Babin [École Normale Supérieure de Lyon, until Jul 2020]
- Benjamin Faucher [Inria, from Sep 2020]
- Arsene Marzorati [Inria, from Jun 2020 until Aug 2020]
- Clemence Metayer [Inria, from May 2020 until Jun 2020]
- Julien Molina [Inria, from Apr 2020 until Jul 2020]
Administrative Assistant
- Claire Sauer [Inria]
External Collaborator
- Fabien Crauste [CNRS, HDR]
2 Overall objectives
2.1 Presentation
Dracula is a joint research team between INRIA, Université Claude Bernard Lyon 1 (UCBL) and CNRS (Institut Camille-Jordan (ICJ, UMR 5208) and Laboratoire de Biologie et Modélisation de la Cellule (LBMC, UMR 5239)).
The Dracula project is devoted to multi-scale modeling in biology and medicine, and more specifically to the development of tools and methods to describe multi-scale processes in biology and medicine. Applications include normal and pathological hematopoiesis (for example leukemia), immune response, and other biological processes, like: tissue renewal, morphogenesis, atherosclerosis, prion disease, hormonal regulation of food intake, and so on. Multi-scale modeling implies simultaneous modeling of several levels of descriptions of biological processes: intra-cellular networks (molecular level), cell behavior (cellular level), dynamics of cell populations (organ or tissue) with the control by other organs (organism) (see Figure 1). Such modeling represents one of the major challenges in modern science due to its importance and because of the complexity of biological phenomena and of the presence of very different interconnected scales.
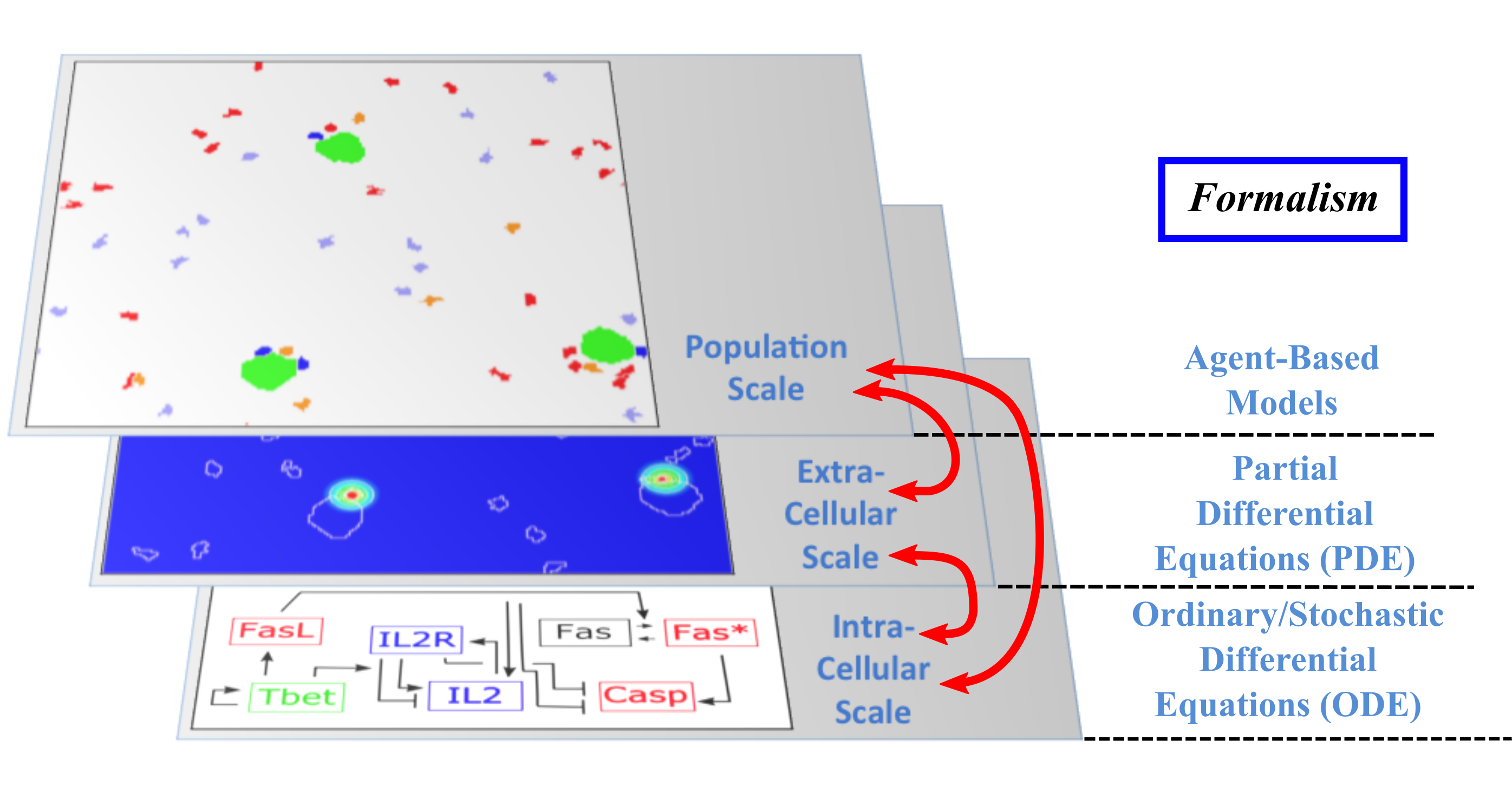
Although multi-scale modeling holds a great potential for biology and medicine, and despite the fact that a variety of techniques exists to deal with such problems, the complexity of the systems poses new challenges and needs the development of new tools. Moreover, different biological questions usually require different types of multi-scale modeling. The expected results of these studies are numerous. On one hand, they will shed new light on the understanding of specific biological and medical questions (for instance, what is the behavior of hematopoietic stem cells under pathological conditions? Or how to efficiently stimulate an immune response in order to design new vaccines?). On the other hand, the modeling methods developed here for specific processes are relevant to study other complex biological systems. We pay a special attention on developing methods that are not restricted to one or two applications.
An important part of our researches is performed in close collaboration with biologists and physicians in order to stay in contact with the biological and medical goals. The presence, within the project, of a biologist (Olivier Gandrillon) who has acquired over the years the know-how required for interacting with mathematicians is probably one of the main assets of the project. He participates actively in many tasks of our program, stimulates interactions between members of the project and biologists, and everyone benefits from his expertise in molecular and cell biology.
2.2 Keywords
Multi-scale modeling; Hybrid modeling; Mathematical Biology; Computational Biology; Immune response modeling; Normal and pathological hematopoiesis; Multi-scale cancer modeling; Regulatory networks; Reaction-diffusion equation; Structured partial differential equations; Delay differential equations; Agent-based modeling; Dynamical systems.
2.3 Research axis 1: Mathematical modeling for cell population dynamics
Executive summary
Stem cells are essential for development and keep the maintenance of many tissues homeostasis. They are characterized by their ability to self-renew as well as to produce differentiated cells. They vary enormously, for each organ, in their proliferation capacity, their potency to produce different cell lineage and their response to various environmental cues. How a cell will react to a given external signal does not depend only on its current state but also on its environment. Understanding the effect of cell-to-cell heterogeneity and the spatial organization of cell populations is therefore necessary to help keeping the normal function of an organ.
We develop mathematical tools and methods to study cell population dynamics and other biological processes: stability of steady sates, existence of bifurcations, kinetic properties, spatial organization, in finely detailed cell populations. The main tools we use are hybrid discrete-continuous models, reaction-diffusion equations, structured models (in which the population is endowed with relevant structures or traits), delay differential systems, agent-based models. Our team has acquired an international expertise in the fields of analysis of reaction-diffusion and structured equations, particularly integro-differential and delay differential equations.
The mathematical methods we develop are not restricted to hematopoietic system (Research axis 2), and immune response (Research axis 3), rather we apply them in many other biological phenomena, for example: tissue renewal, morphogenesis, prion disease, atherosclerosis, hormonal regulation of food intake, cancer, and others.
Project-team positioning
The focus of this objective is the development, analysis and application of hybrid discrete-continuous, reaction-diffusion and structured partial differential models. The structured equations allow a fine description of a population as some structures (age, maturity, intracellular content) change with time. In many cases, structured equations can be partially integrated to yield integro-differential equations (ordinary or partial differential equations involving non-local integral terms), time-delay differential or time-delay partial differential, or coupled differential-difference models. Analysis of integro-differential and time-delay systems deals with existence of solutions and their stability. Applications are found in the study of normal and pathological hematopoietic system (Research axis 2), immune response (Research axis 3), morphogenesis, prion disease, cancer development and treatment, and generally in tissue renewal problems. Models based on structured equations are especially useful to take into account the effect of finite time cells take to divide, die or become mature. Reaction-diffusion equations are used in order to describe spatial distribution of cell populations. It is a well developed area of research in our team which includes qualitative properties of travelling waves for reaction-diffusion systems with or without delay, and complex nonlinear dynamics.
Our team has developed a solid expertise in mathematical analysis of reaction-diffusion with or without delay and structured equations (in particular, delay differential equations) and one of the most prolific. Other major groups are the teams of Benoit Perthame (Pierre et Marie CURIE University and Mamba, Paris, https://
Collaborations
- University of Toronto, Canada; Mathematical analysis and applications of reaction-diffusion equations (more than 30 joint papers).
- Institute of Problems of Mechanical Engineering, St.Petersburg, Russia; Dynamics of cell renewal (more than 10 joint papers).
- Department of Cell and Molecular Biology and Department of Forensic Medicine, Stockholm, Sweden; Dynamics of cell generation and turnover (3 joint papers).
- Universities of Tlemcen (Algeria) and Marrakech (Morocco); Delay differential equations (7 joint papers)
2.4 Research axis 2: Multi-scale modeling of hematopoiesis and leukemia
Executive summary
Hematopoiesis is a complex process that begins with hematopoietic stem cells (HSCs) and results in formation of mature cells: red blood cells, white cells and platelets. Blood cells are produced in the bone marrow, from where mature cells are released into the blood stream. Hematopoiesis is based on a balance between cell proliferation (including self-renewal), differentiation and apoptosis. The choice between these three possibilities is determined by intra-cellular regulatory networks and by numerous control mechanisms in the bone marrow or carried out by other organs. Intra-cellular regulatory networks are complex biochemical reactions involving proteins, enzymes and signalling molecules. The deregulation of hematopoiesis can result in numerous blood diseases including leukemia (a cancer of blood cells). One important type of leukemia is Chronic Myeloid Leukemia (CML). The strong tyrosine kinase activity of the BCR-ABL protein is the basis for the main cell effects that are observed in CML: significant proliferation, anti-apoptotic effect, disruption of stroma adhesion properties. This explains the presence in CML blood of a very important number of cells belonging to the myeloid lineage, at all stages of maturation.
Multi-scale modeling in hematopoiesis holds a great potential. A variety of techniques exists to deal with this problem. However, the complexity of the system poses new difficulties and leads to the development of new tools. The expected results of this study are numerous. On one hand, it will shed new light on the different physiological mechanisms that converge toward the continuous regeneration of blood cells, for example: the understanding of deregulation of erythropoiesis (the process of red blood cell production) under drug treatments (this can lead to lack of red blood cells (anemia), or a surplus of red blood cells), the dynamic of leukemic cells under the action of drugs and the control of their resistance to these treatments.
Project team positioning
Multi-scale modeling of hematopoiesis is one of the key points of the project that has started in the early stage of the Dracula team. Investigated by all the team members, it took many years of close discussion with biologists to get the best understanding of the key role played by the most important molecules, hormones, kinase cascade, cell communication up to the latest knowledge. One of the important questions here is to identify particular biological mechanisms (intracellular regulation, control mechanisms) and to integrate them in the different models. Our main work consisted in the development of a hybrid (continuous/discrete) model for red blood cell progenitor proliferation, survival/death, differentiation, and migration. Cells are modeled as discrete objects, and the extracellular medium is described by continuous equations for extracellular concentrations. This is to our knowledge the most complete model for erythropoiesis to date, and the only one using a multi-scale formalism. Other models published by our group and others for hematopoiesis are population-based models, mostly population structured equations (transport partial differential equations or delay differential equations). The interest in modeling hematopoiesis dates back to the 70's and two groups have been responsible for most of development in the past 40 years: Markus Loeffer's team in Leipzig, Germany (Wichmann et al. 1976, in Mathematical Models in Medicine) and Michael Mackey's team at McGill University, Montreal, Canada (Mackey 1978, Blood). Our model differs from population based models in that the regulation is directly modeled at the molecular level (See Figure 1) rather than acting on rates at the population level. Thus we can take into account non-predictable effects of interactions between different molecular pathways and between cells that would otherwise be lost in the global population rates.
Regarding modeling leukemia, we concentrated on Chronic Myeloid Leukemia (CML) and its treatment. We considered models based on ordinary differential equations for the action of the main proteins involved in CML (as BCR-ABL protein), and of transport equations (with or without delay, physiologically structured or not) to represent healthy and leukemic cell populations, take into account many interactions between proteins (especially BCR-ABL), cells (anti-apoptotic effect, etc.). The development of models for CML allowed us to interact with Franck Nicolini in Lyon (Centre Hospitalier de Lyon) and Doron Levy (Maryland University, http://
Collaborations
Members of the team have worked for several years in collaboration with biologists (François Morlé, University Lyon 1) and hematologists (Charles Dumontet, Lyon and Mark Koury, Nashville, http://
The work on modeling Leukemia is based on two major collaborations: firstly, an ongoing (since 2011) mathematical collaboration with the University of Maryland through the program Associate Teams Inria project, “Modelling Leukemia” (http://
2.5 Research axis 3: Multi-scale modeling of the immune response
Executive summary
Vaccination represents a worldwide health, social and economical challenge as it has allowed the eradication or the strong containment of several devastating diseases over the past century. However to date, most of the effective vaccines rely on the generation of neutralizing antibody responses and such vaccines have proven largely unsuccessful in the prevention against some pathogens, such as HIV or malaria. In such cases, vaccines geared towards the generation of CD8 T cell immunity may provide a better protection. The generation of memory CD8 T cells following antigenic immunization is a long process (lasting up to month in murine preclinical models), therefore strongly slowing the process of vaccine monitoring in preclinical studies. Thus, the dynamical modeling of the CD8 T cell immune response both at the cellular and molecular levels should provide an important tool to better understand the dynamics of the response and to speed-up the process and reduce costs of vaccine development.
However, currently published cellular models of the immune response are either over-simplified, not predicting important parameters of this response, or too complicated for most of their parameters to be accessible for experimental measurements, thus impeding their biological validation. Dynamical models of the CD8 T cell response at the molecular level are very scarce and there is no multi-scale model of the immune response giving insights into both the regulation at the molecular scale and the consequences on cell population dynamics.
The objective of this research axis is therefore to develop a predictive multi-scale model of the CD8 T cell response, by confronting the model at different stages to in vivo-acquired experimental data, in order to be able to investigate the influence of early molecular events on cell population dynamics few days or weeks later.
Project-team positioning
We are aiming at building and analyzing a multi-scale model of the CD8 T cell immune response, from the molecular to the cellular and potentially organismal scale. This consists in describing the dynamics at each scale with relevant formalisms as well as the careful description of the couplings between scales.
Only few research groups are actually working on the CD8 T cell immune response around the world, and none of them deals with multi-scale modeling of this response. A network developed around Alan Perelson's work in theoretical immunology in the last decades, at Los Alamos National Laboratory, and involves mainly people in various US universities or institutes. In Europe, Rob De Boer's group (http://
Ron Germain's group at the NIH, and Grégoire Altan-Bonnet in subsequent works, focused on the molecular regulation of the CD4 and CD8 T cell immune responses. In particular, they built the Simmune software, which allows the modeling and simulation of molecular interactions (https://
Within Inria project-teams, NUMED develops multi-scale approaches for biological problems, and MAMBA and MONC (https://
Collaborations
On this topic our main collaborators are members of Jacqueline Marvel's team in Lyon in the CIRI (Centre International de Recherche en Infectiologie INSERM U1111): Dr. Jacqueline Marvel, head of the team, Dr. Christophe Arpin (CR CNRS), and other technicians and engineers of the team. They are all immunologists, specialists of the CD8 T cell response and of the generation of memory CD8 T cells.
We also interact with private companies: AltraBio (http://
2.6 Evolution of research direction during the last evaluation
Reminder of the objectives given for the last evaluation
The aim of this project is the development of modern tools for multi-scale modeling in biological phenomena. During the period 2014-2017, the objectives we had fixed were to develop modern tools for multi-scale modeling of biological phenomena, as detailed hereafter:
- Multi-scale modeling of erythropoiesis, the process of red blood cell production, in order to describe normal, stress, and pathological erythropoiesis, using mathematical and computational models. This led to:
- The modeling of hemoglobin instability in dialysis patients: Thomas Lepoutre has been progressively taking part in this theme through a collaboration with P. Kim (University of Sydney, Australia);
- Multi-scale modeling of the CD8 T cell immune response, in order to develop a predictive model of the CD8 T cell response, by confronting the model at different stages to in vivo-acquired experimental data;
- Population dynamics modeling, with the aim to develop general mathematical tools to study them. The main tools we were using were structured equations, in which the cell population is endowed with relevant structures, or traits. We identified limitations in using these formalisms, this is why we started developing multi-scale approaches;
- Modeling of Chronic Myeloid Leukemia (CML) treatment, using ordinary differential equations models. Our team had already developed a first model of mutant leukemic cells being resistant to chemotherapy. A next step would be to identify the parameters using experimental data;
- Multi-scale modeling carried out on the basis of hybrid discrete-continuous models, where dissipative particle dynamics (DPD) are used in order to describe individual cells and relatively small cell populations, partial differential equations (PDE) are used to describe concentrations of bio-chemical substances in the extracellular matrix, and ordinary differential equations for intracellular regulatory networks (Figure 1). An emphasis would be made on developing codes that are both flexible and powerful enough to implement variants of the model, perform simulations, produce desired outputs, and provide tools for analysis; to do so:
- We planned to contribute to a recent project named chronos, whose code (written in C++) represents heterogeneous populations of individual cells evolving in time and interacting physically and biochemically, and the objective is to make the code flexible enough to implement different formalisms within the same model, so that different components of the model can be represented in the most appropriate way;
- Partial differential equations (PDE) analysis, with a focus on reaction-diffusion equations, transport equations (hyperbolic PDEs) in which the structure can be age, maturity, protein concentration, etc., with particular cases where transport equations are reduced to delay differential equations (DDE).
Comments on these objectives over the evaluation period
We have had strong contributions to objectives 1, 3, 4, 5, and consequently to objective 6, as well as to objective 8, as mentioned in previous sections. These contributions represented the core of the team's research activity over the evaluation period, as stressed by our publications. It is however noticeable that multi-scale modeling of the immune response and of pathological hematopoiesis (leukemia) has come to represent a proportionally more important part of our activity.
Objective 2 has been cancelled few months after the previous evaluation, following meetings with clinicians who did not show any particular interest in our approaches. The modeling of chronic myeloid leukemia instead took a bigger part of the team's research activity, both project being at the time coordinated by Thomas Lepoutre.
Objective 7 has been pursued, the project chronos evolved to a better defined project SiMuScale that is currently being developed and aims at structuring the team's activity and providing a simulation platform that could be adapted to various biological questions necessitating multi-scale modeling.
Objectives for the next four years
The main objectives for the next four years are to continue to improve the 3 previous points: 1) Mathematical and computational modeling for cell population dynamics; 2) Multi-scale modeling of hematopoiesis and leukemia; 3) Multi-scale modeling of the immune response. In addition, we will pursue our effort to develop a simulation platform for multi-scale models (SiMuScale) and we intend to develop the use of mixed effect models and other statistical approaches to deal with the challenges offered by modern biology, in particular the generation of single cell data.
3 Research program
3.1 Mixed-effect models and statistical approaches
Most of biological and medical data our team has to deal with consist in time series of experimental measurements (cell counts, gene expression level, etc.). The intrinsic variability of any biological system complicates its confrontation to models. The trivial use of means, eliminating the data variance, is but a second-best solution. Furthermore, the amount of data that can be experimentally generated often limits the use of classical mathematical approaches because model’s identifiability or parameter identifiability cannot be obtained. In order to overcome this issue and to efficiently take advantage of existing and available data, we plan to use mixed effect models for various applications (for instance: leukemia treatment modeling, immune response modeling). Such models were initially developed to account for individual behaviors within a population by characterizing distributions of parameter values instead of a unique parameter value. We plan to use those approaches both within that frame (for example, taking into account longitudinal studies on different patients, or different mice) but also to extend its validity in a different context: we will consider different ex vivo experiments as being “different individuals”: this will allow us to make the most of the experience-to-experience variations.
Such approaches need expertise in statistics to be correctly implemented, and we will rely on the presence of Céline Vial in the team to do so. Céline Vial is an expert in applied statistics and her experience already motivated the use of better statistical methods in various research themes. The increasing use of single cell technologies in biology make such approaches necessary and it is going to be critical for the project to acquire such skills.
3.2 Development of a simulation platform
We have put some effort in developing the SiMuScale platform, a software coded in dedicated to exploring multiscale population models, since 2014. In order to answer the challenges of multi-scale modeling it is necessary to possess an all-purpose, fast and flexible modeling tool, and SiMuScale is the choice we made. Since it is based on a core containing the simulator, and on plug-ins that contain the biological specifications of each cell, this software will make it easier for members of the team – and potentially other modelers – to focus on the model and to capitalize on existing models, which all share the same framework and are compatible with each other. Within the next four years, SiMuScale should be widely accessible and daily used in the team for multi-scale modeling. It will be developed into a real-case context, the modeling of the hematopoietic stem cell niche, in collaboration with clinicians (Eric Solary, INSERM) and physicists (Bertrand Laforge, UPMC).
3.3 Mathematical and computational modeling
Multi-scale modeling of hematopoiesis is one of the key points of the project that has started in the early stage of the Dracula team. Investigated by the team members, it took many years of close discussion with biologists to get the best understanding of the key role played by the most important molecules, hormones, kinase cascade, cell communication up to the latest knowledge. An approach that we used is based on hybrid discrete-continuous models, where cells are considered as individual objects, intracellular regulatory networks are described with ordinary differential equations, extracellular concentrations with diffusion or diffusion-convection equations (see Figure 1). These modeling tools require the expertise of all team members to get the most qualitative satisfactory model. The obtained models will be applied particularly to describe normal and pathological hematopoiesis as well as immune response.
3.4 From hybrid dynamics to continuum mechanics
Hybrid discrete-continuous methods are well adapted to describe biological cells. However, they are not appropriate for the qualitative investigation of the corresponding phenomena. Therefore, hybrid model approach should be combined with continuous models. If we consider cell populations as a continuous medium, then cell concentrations can be described by reaction-diffusion systems of equations with convective terms. The diffusion terms correspond to a random cell motion and the reaction terms to cell proliferation, differentiation and death. We will continue our studies of stability, nonlinear dynamics and pattern formation. Theoretical investigations of reaction-diffusion models will be accompanied by numerical simulations and will be applied to study cell population dynamic.
3.5 Structured partial differential equations
Hyperbolic problems are also of importance when describing cell population dynamics. They are structured transport partial differential equations, in which the structure is a characteristic of the considered population, for instance age, size, maturity, etc. In the scope of multi-scale modeling, protein concentrations as structure variables can precisely indicate the nature of cellular events cells undergo (differentiation, apoptosis), by allowing a representation of cell populations in a multi-dimensional space. Several questions are still open in the study of this problem, yet we will continue our analysis of these equations by focusing in particular on the asymptotic behavior of the system (stability, oscillations) and numerical simulations.
3.6 Delay differential equations
The use of age structure in PDE often leads to a reduction (by integration over the age variable) to delay differential equations. Delay differential equations are particularly useful for situations where the processes are controlled through feedback loops acting after a certain time. For example, in the evolution of cell populations the transmission of control signals can be related to some processes as division, differentiation, maturation, apoptosis, etc. Delay differential equations offer good tools to study the behavior of the systems. Our main investigation will be the effect of perturbations of the parameters, as cell cycle duration, apoptosis, differentiation, self-renewal, etc., on the behavior of the system, in relation for instance with some pathological situations. The mathematical analysis of delay differential equations is often complicated and needs the development of new criteria to be performed.
3.7 Multi-scale modeling of the immune response
The main objective of this part is to develop models that make it possible to investigate the dynamics of the adaptive CD8 T cell immune response, and in particular to focus on the consequences of early molecular events on the cellular dynamics few days or weeks later: this would help developing predictive tools of the immune response in order to facilitate vaccine development and reduce costs. This work requires a close and intensive collaboration with immunologist partners.
We recently published a model of the CD8 T cell immune response characterizing differentiation stages, identified by biomarkers, able to predict the quantity of memory cells from early measurements ( 38). In parallel, we improved our multiscale model of the CD8 T cell immune response, by implementing a full differentiation scheme, from naïve to memory cells, based on a limited set of genes and transcription factors.
Our first task will be to infer an appropriate gene regulatory network (GRN) using single cell data analysis (generate transcriptomics data of the CD8 T cell response to diverse pathogens), the previous biomarkers we identified and associated to differentiation stages, as well as piecewise-deterministic Markov processes (Ulysse Herbach's PhD thesis, ongoing).
Our second task will be to update our multiscale model by first implementing the new differentiation scheme we identified ( 38), and second by embedding CD8 T cells with the GRN obtained in our first task (see above). This will lead to a multi-scale model incorporating description of the CD8 T cell immune response both at the molecular and the cellular levels (Simon Girel's PhD thesis, ongoing).
In order to further develop our multiscale model, we will consider an agent-based approach for the description of the cellular dynamics. Yet, such models, coupled to continuous models describing GRN dynamics, are computationally expensive, so we will focus on alternative strategies, in particular on descriptions of the cellular dynamics through both continuous and discrete models, efficiently coupled. Using discrete models for low cell numbers and continuous (partial differential equations) models for large cell numbers, with appropriate coupling strategies, can lead to faster numerical simulations, and consequently can allow performing intense parameter estimation procedures that are necessary to validate models by confronting them to experimental data, both at the molecular and cellular scales.
The final objective will be to capture CD8 T cell responses in different immunization contexts (different pathogens, tumor) and to predict cellular outcomes from molecular events.
3.8 Dynamical network inference from single-cell data
Up to now, all of our multiscale models have incorporated a dynamical molecular network that was build “by hand” after a thorough review of the literature. It would be highly valuable to infer it directly from gene expression data. However, this remains very challenging from a methodological point of view. We started exploring an original solution for such inference by using the information contained within gene expression distributions. Such distributions can be acquired through novel techniques where gene expression levels are quantified at the single cell level. We propose to view the inference problem as a fitting procedure for a mechanistic gene network model that is inherently stochastic and takes not only protein, but also mRNA levels into account. This approach led to very encouraging results 39 and we will actively pursue in that direction, especially in the light of the foreseeable explosion of single cell data.
3.9 Leukemia modeling
Imatinib and other tyrosine kinase inhibitors (TKIs) have marked a revolution in the treatment of Chronic Myelogenous Leukemia (CML). Yet, most patients are not cured, and must take their treatment for life. Deeper mechanistic understanding could improve TKI combination therapies to better control the residual leukemic cell population.
In a collaboration with the Hospital Lyon Sud and the University of Maryland, we have developed mathematical models that integrate CML and an autologous immune response ( 35, 36 and 37). These studies have lent theoretical support to the idea that the immune system plays a rôle in maintaining remission over long periods. Our mathematical model predicts that upon treatment discontinuation, the immune system can control the disease and prevent a relapse. There is however a possibility for relapse via a sneak-though mechanism 35. Research in the next four years will focus in the Phase III PETALS trial. In the PETALS trial (https://
4 Application domains
See the previous sections.
5 New software and platforms
5.1 New software
5.1.1 CelDyn
- Keywords: Modeling, Bioinformatics, Biology
- Functional Description: Software "Celdyn" is developed in order to model cell population dynamics for biological applications. Cells are represented either as soft spheres or they can have more complex structure. Cells can divide, move, interact with each other or with the surrounding medium. Different cell types can be introduced. When cells divide, the types of daughter cells are specified. A user interface is developed.
- Authors: Nikolai Bessonov, Vitaly Volpert
- Contacts: Nicolas Jourdan, Vitaly Volpert
- Participants: Alen Tosenberger, Laurent Pujo-Menjouet, Nikolai Bessonov, Vitaly Volpert
6 New results
6.1 Models of immune response
6.1.1 Modeling and characterization of inter-individual variability in CD8 T cell responses
To develop vaccines, it is mandatory yet challenging to account for inter-individual variability during immune responses. Even in laboratory mice, T cell responses of single individuals exhibit a high heterogeneity that may come from genetic backgrounds, intra-specific processes (e.g. antigen-processing and presentation) and immunization protocols. To account for inter-individual variability in CD8 T cell responses in mice, we propose in 4, a dynamical model coupled to a statistical, nonlinear mixed effects model. Average and individual dynamics during a CD8 T cell response are characterized in different immunization contexts (vaccinia virus and tumor). On one hand, we identify biological processes that generate inter-individual variability (activation rate of naive cells, the mortality rate of effector cells, and dynamics of the immunogen). On the other hand, introducing categorical covariates to analyze two different immunization regimens, we highlight the steps of the response impacted by immunogens (priming, differentiation of naive cells, expansion of effector cells and generation of memory cells). The robustness of the model is assessed by confrontation to new experimental data. Our approach allows to investigate immune responses in various immunization contexts, when measurements are scarce or missing, and contributes to a better understanding of inter-individual variability in CD8 T cell immune responses.
6.1.2 Multiscale modeling of germinal center recapitulates the temporal transition from memory B cells to plasma cells
Germinal centers play a key role in the adaptive immune system since they are able to produce memory B cells and plasma cells that produce high affinity antibodies for an effective immune protection. The mechanisms underlying cell-fate decisions are not well understood but asymmetric division of antigen, B-cell receptor affinity, interactions between B-cells and T follicular helper cells (triggering CD40 signaling), and regulatory interactions of transcription factors have all been proposed to play a role. In addition, a temporal switch from memory B-cell to plasma cell differentiation during the germinal center reaction has been shown. To investigate if antigen affinity-based Tfh cell help recapitulates the temporal switch we implemented (see 20) a multiscale model that integrates cellular interactions with a core gene regulatory network comprising BCL6, IRF4, and BLIMP1. Using this model we show that affinity-based CD40 signaling in combination with asymmetric division of B-cells result in switch from memory B-cell to plasma cell generation during the course of the germinal center reaction. We also show that cell fate division is unlikely to be (solely) based on asymmetric division of Ag but that BLIMP1 is a more important factor. Altogether, our model enables to test the influence of molecular modulations of the CD40 signaling pathway on the production of germinal center output cells.
6.1.3 Maternal passive immunity and dengue hemorrhagic fever in infants
Dengue hemorrhagic fever (DHF) can occur in primary dengue virus infection of infants less than one year of age. To understand the presumed role of maternal dengue-specific antibodies received until birth in the development of this primary DHF in infants, we investigated in 2 a mathematical model based on a system of nonlinear ordinary differential equations that mimics cells, virus and antibodies interactions. The neutralization and enhancement activities of maternal antibodies against the virus are represented by a function derived from experimental data and knowledge from the medical literature. The analytic study of the model shows the existence of two equilibriums, a disease-free equilibrium and an endemic one. We performed the asymptotic stability analysis for these two equilibriums. The local asymptotic stability of the endemic equilibrium (DHF equilibrium) corresponds to the occurrence of DHF. Numerical results are also presented in order to illustrate the mathematical analysis performed, highlighting the most important parameters that drive model dynamics. We defined the age at which DHF occurs as the time when the infection takes off that means at the inflection point of the curve of infected cell population. We showed that this age corresponds to the one at which maximum enhancing activity for dengue infection appears. This critical time for the occurrence of DHF is calculated from the model to be approximately two months after the time for maternal dengue neutralizing antibodies to degrade below a protective level, which corresponds to what is observed in the experimental data from the literature.
6.1.4 Viral infection model with CTL immunity
A mathematical model describing viral dynamics in the presence of the latently infected cells and the cytotoxic T-lymphocytes cells (CTL), taking into consideration the spatial mobility of free viruses, is presented and studied in 16. The model includes five nonlinear differential equations describing the interaction among the uninfected cells, the latently infected cells, the actively infected cells, the free viruses, and the cellular immune response. First, we establish the existence, positivity, and boundedness for the suggested diffusion model. Moreover, we prove the global stability of each steady state by constructing some suitable Lyapunov functionals. Finally, we validated our theoretical results by numerical simulations for each case. A review on mathematical modeling of the immune system in homeostasis, infection and disease is presented in 17.
6.1.5 Genotype-dependent virus distribution and competition of virus strains
Virus density distribution as a function of genotype considered as a continuous variable and of time is studied with a nonlocal reaction-diffusion equation taking into account virus competition for the host cells and its elimination by the immune response and by the genotype-dependent mortality, 9. The existence of virus strains, that is, of positive stable stationary solutions decaying at infinity, is determined by the admissible intervals in the genotype space where the genotype-dependent mortality is less than the virus reproduction rate, and by the immune response under some appropriate assumptions on the immune response function characterizing virus elimination by immune cells. The competition of virus strains is studied, first, without immune response and then with the immune response. In the absence of immune response, the strain dynamics is different in a short time scale where they converge to some intermediate slowly evolving solutions depending on the initial conditions, and in a long time scale where their distribution converges to a stationary solution. Immune response can essentially influence the strain dynamics either stabilizing them or eliminating one of the strains. An antiviral treatment can also influence the competition of virus strains, and it can lead to the emergence of resistant strains, which were absent before the treatment because of the competition with susceptible strains.
6.1.6 Reaction–diffusion waves in a model of immune response
In 27, existence of travelling waves is studied for a reaction-diffusion system of equations describing the distribution of viruses and immune cells in the tissue. The proof uses the Leray-Schauder method based on the topological degree for elliptic operators in unbounded domains and on a priori estimates of solutions in weighted spaces.
6.1.7 Nonlocal reaction–diffusion model of viral evolution: emergence of virus strains
The paper 10 is devoted to the investigation of virus quasi-species evolution and diversification due to mutations, competition for host cells, and cross-reactive immune responses. The model consists of a nonlocal reaction-diffusion equation for the virus density depending on the genotype considered to be a continuous variable and on time. This equation contains two integral terms corresponding to the nonlocal effects of virus interaction with host cells and with immune cells. In the model, a virus strain is represented by a localized solution concentrated around some given genotype. Emergence of new strains corresponds to a periodic wave propagating in the space of genotypes. The conditions of appearance of such waves and their dynamics are described.
6.2 Cell population dynamics
Platelets upregulate the generation of thrombin and reinforce the fibrin clot which increases the incidence risk of venous thromboembolism (VTE). However, the role of platelets in the pathogenesis of venous cardiovascular diseases remains hard to quantify. An experimentally validated model of thrombin generation dynamics is formulated in 12. The model predicts that a high platelet count increases the peak value of generated thrombin as well as the endogenous thrombin potential (ETP) as reported in experimental data. To investigate the effects of platelets density, shear rate, and wound size on the initiation of blood coagulation, we calibrate a previously developed model of venous thrombus formation and implement it in 3D using a novel cell-centered finite-volume solver. We conduct numerical simulations to reproduce in vitro experiments of blood coagulation in microfluidic capillaries. Then, we derive a reduced one-equation model of thrombin distribution from the previous model under simplifying hypotheses and we use it to determine the conditions of clotting initiation on the platelet count, the shear rate, and the plasma composition. The initiation of clotting also exhibits a threshold response to the size of the wounded region in good agreement with the reported experimental findings.
6.3 Model for the propagation of prion proteins in yeast system
The use of yeast systems to study the propagation of prions and amyloids has emerged as a crucial aspect of the global endeavor to understand those mechanisms. Yeast prion systems are intrinsically multi-scale: the molecular chemical processes are indeed coupled to the cellular processes of cell growth and division to influence phenotypical traits, observable at the scale of colonies. We introduce in 18 a novel modeling framework to tackle this difficulty using impulsive differential equations. We apply this approach to the [PSI+] yeast prion, which is associated with the misconformation and aggregation of Sup35. We build a model that reproduces and unifies previously conflicting experimental observations on [PSI+] and thus sheds light onto characteristics of the intracellular molecular processes driving aggregate replication. In particular our model uncovers a kinetic barrier for aggregate replication at low densities, meaning the change between prion or prion-free phenotype is a bi-stable transition. This result is based on the study of prion curing experiments, as well as the phenomenon of colony sectoring, a phenotype which is often ignored in experimental assays and has never been modeled. Furthermore, our results provide further insight into the effect of guanidine hydrochloride (GdnHCl) on Sup35 aggregates. To qualitatively reproduce the GdnHCl curing experiment, aggregate replication must not be completely inhibited, which suggests the existence of a mechanism different than Hsp104-mediated fragmentation. Those results are promising for further development of the [PSI+] model, but also for extending the use of this novel framework to other yeast prion or amyloid systems.
6.4 Mathematical models for treatment of cancer
6.4.1 A mathematical model for treatment of papillary thyroid cancer using the allee effect
The incidence of thyroid cancer is rising all over the world, and the papillary subtype (PTC) is the primary factor for this increase. The presence of thyroid tumors is commonly associated with increased levels of cytokines, such as interleukin 6 (IL-6). Considering PTC patients treated with thyroidectomy and radioactive iodine (RAI), we propose in 15 an ordinary differential system using four variables: the RAI activity, the number of cancer cells and the serum concentrations of IL-6 and thyroglobulin (Tg). Our objective is to study the efficacy of different therapeutic doses of RAI in the treatment of thyroid cancer. The Allee effect is taken into account when modeling cancer cells growth under the influence of IL-6. From the results obtained, the main factors and conditions correlated with successful treatment, such as the RAI activity used and the tumor response are addressed. The detection of minimal doses of RAI that can cause tumor extinction is performed, though this has also meant longer periods for tumor cell elimination. The critical number of tumor cells due to the Allee effect is analyzed and linked to the immune system or biological factors that can slow the progression of the tumor but are insufficient after thyroid resection surgery.
6.4.2 A multiscale model to design therapeutic strategies that overcome drug resistance to tyrosine kinase inhibitors in multiple myeloma
Drug resistance (DR) is a phenomenon characterized by the tolerance of a disease to pharmaceutical treatment. In cancer patients, DR is one of the main challenges that limit the therapeutic potential of the existing treatments. Therefore, overcoming DR by restoring the sensitivity of cancer cells would be greatly beneficial. In this context, mathematical modeling can be used to provide novel therapeutic strategies that maximize the efficiency of anti-cancer agents and potentially overcome DR. In 13, we present a new multiscale model devoted to the interaction of potential treatments with multiple myeloma (MM) development. In this model, MM cells are represented as individual objects that move, divide, and die by apoptosis. The fate of each cell depends on intracellular and extracellular regulation, as well as the administered treatment. The model is used to explore the combined effects of a tyrosine-kinase inhibitor (TKI) with a pentose phosphate pathway (PPP) inhibitor. We use numerical simulations to tailor effective and safe treatment regimens that may eradicate the MM tumors. The model suggests that an interval for the daily dose of the PPP inhibitor can maximize the responsiveness of MM cells to the treatment with TKIs. Then, it demonstrates that the combination of high-dose pulsatile TKI treatment with high-dose daily PPP inhibitor therapy can potentially eradicate the tumor. Predictions of numerical simulations using such a model can be considered as testable hypotheses in future pre-clinical experiments and clinical studies.
6.5 COVID-19 (SARS-CoV-2) and epidemiological models
6.5.1 Coronavirus - Scientific insights and societal aspects
In December 2019, the first case of infection with a new virus COVID-19 (SARS-CoV-2), named coronavirus, was reported in the city of Wuhan, China. At that time, almost nobody paid any attention to it. The new pathogen, however, fast proved to be extremely infectious and dangerous. Over the few months that followed, coronavirus has spread over entire world. At the end of March, the total number of infections is fast approaching the psychological threshold of one million, resulting so far in tens of thousands of deaths. Due to the high number of lives already lost and the virus high potential for further spread, and due to its huge overall impact on the economies and societies, it is widely admitted that coronavirus poses the biggest challenge to the humanity after the second World war. The COVID-19 epidemic is provoking numerous questions at all levels. It also shows that modern society is extremely vulnerable and unprepared to such events. A wide scientific and public discussion becomes urgent. Some possible directions of this discussion are suggested in 28 and 26.
6.5.2 On a quarantine model of coronavirus infection and data analysis
Attempts to curb the spread of coronavirus by introducing strict quarantine measures apparently have different effect in different countries: while the number of new cases has reportedly decreased in China and South Korea, it still exhibit significant growth in Italy and other countries across Europe. In the brief note 25, we endeavour to assess the efficiency of quarantine measures by means of mathematical modelling. Instead of the classical SIR model, we introduce a new model of infection progression under the assumption that all infected individual are isolated after the incubation period in such a way that they cannot infect other people. Disease progression in this model is determined by the basic reproduction number R0 (the number of newly infected individuals during the incubation period), which is different compared to that for the standard SIR model. If R0>1, then the number of latently infected individuals exponentially grows. However, if R0<1 (e.g. due to quarantine measures and contact restrictions imposed by public authorities), then the number of infected decays exponentially. We then consider the available data on the disease development in different countries to show that there are three possible patterns: growth dynamics, growth-decays dynamics, and patchy dynamics (growth-decay-growth). Analysis of the data in China and Korea shows that the peak of infection (maximum of daily cases) is reached about 10 days after the restricting measures are introduced. During this period of time, the growth rate of the total number of infected was gradually decreasing. However, the growth rate remains exponential in Italy. Arguably, it suggests that the introduced quarantine is not sufficient and stricter measures are needed.
6.5.3 Global dynamics of a differential-difference system: a case of Kermack-McKendrick SIR model with age-structured protection phase
In 1, we are concerned with an epidemic model of susceptible, infected and recovered (SIR) population dynamic by considering an age-structured phase of protection with limited duration, for instance due to vaccination or drugs with temporary immunity. The model is reduced to a delay differential-difference system, where the delay is the duration of the protection phase. We investigate the local asymptotic stability of the two steady states: disease-free and endemic. We also establish when the endemic steady state exists, the uniform persistence of the disease. We construct quadratic and logarithmic Lyapunov functions to establish the global asymptotic stability of the two steady states. We prove that the global stability is completely determined by the basic reproduction number.
6.6 Stochastic intracellular regulation can remove oscillations in a model of tissue growth
The paper 6 is devoted to the analysis of cell population dynamics where cells make a choice between differentiation and apoptosis. This choice is based on the values of intracellular proteins whose concentrations are described by a system of ordinary differential equations with bistable dynamics. Intracellular regulation and cell fate are controlled by the extracellular regulation through the number of differentiated cells. It is shown that the total cell number necessarily oscillates if the initial condition in the intracellular regulation is fixed. These oscillations can be suppressed if the initial condition is a random variable with a sufficiently large variation. Thus, the result of the work suggests a possible answer to the question about the role of stochasticity in the intracellular regulation.
6.7 Phenotypic noise and the cost of complexity
Experimental studies demonstrate the existence of phenotypic diversity despite constant genotype and environment. Theoretical models based on a single phenotypic character predict that during an adaptation event, phenotypic noise should be positively selected far from the fitness optimum because it increases the fitness of the genotype, and then be selected against when the population reaches the optimum. It is suggested that because of this fitness gain, phenotypic noise should promote adaptive evolution. However, it is unclear how the selective advantage of phenotypic noise is linked to the rate of evolution, and whether any advantage would hold for more realistic, multidimensional phenotypes. Indeed, complex organisms suffer a cost of complexity, where beneficial mutations become rarer as the number of phenotypic characters increases. Using a quantitative genetics approach, we first show, 23, that for a one‐dimensional phenotype, phenotypic noise promotes adaptive evolution on plateaus of positive fitness, independently from the direct selective advantage on fitness. Second, we show that for multidimensional phenotypes, phenotypic noise evolves to a low‐dimensional configuration, with elevated noise in the direction of the fitness optimum. Such a dimensionality reduction of the phenotypic noise promotes adaptive evolution and numerical simulations show that it reduces the cost of complexity.
6.8 Population dynamics and ecology
6.8.1 Prey-predator model with nonlocal and global consumption in the prey dynamics
A prey-predator model with a nonlocal or global consumption of resources by prey is studied in 5. Linear stability analysis about the homogeneous in space stationary solution is carried out to determine the conditions of the bifurcation of stationary and moving pulses in the case of global consumption. Their existence is confirmed in numerical simulations. Periodic travelling waves and multiple pulses are observed for the nonlocal consumption.
6.8.2 Modeling the dynamics of Wolbachia-infected and uninfected A edes aegypti populations by delay differential equations
In the paper 7, starting from an age structured partial differential model, constructed taking into account the mosquito life cycle and the main features of the Wolbachia-infection, we derived a delay differential model using the method of characteristics, to study the colonization and persistence of the Wolbachia-transinfected Aedes aegypti mosquito in an environment where the uninfected wild mosquito population is already established. Under some conditions, the model can be reduced to a Nicholson-type delay differential system; here, the delay represents the duration of mosquito immature phase that comprises egg, larva and pupa. In addition to mortality and oviposition rates characteristic of the life cycle of the mosquito, other biological features such as cytoplasmic incompatibility, bacterial inheritance, and deviation on sex ratio are considered in the model. The model presents three equilibriums: the extinction of both populations, the extinction of Wolbachia-infected population and persistence of uninfected one, and the coexistence. The conditions of existence for each equilibrium are obtained analytically and have been interpreted biologically. It is shown that the increase of the delay can promote, through Hopf bifurcation, stability switch towards instability for the nonzero equilibriums. Overall, when the delay increases and crosses predetermined thresholds, the populations go to extinction.
6.8.3 Spatio-temporal Bazykin’s model for prey-predator interaction
The paper 22 deals with a reaction-diffusion model for prey-predator interaction with Bazykin's reaction kinetics and a nonlocal interaction term in prey growth. The kernel of the integral characterizes nonlocal consumption of resources and depends on space and time. Linear stability analysis determines the conditions of the emergence of Turing patterns without and with nonlocal term, while weakly nonlinear analysis allows the derivation of amplitude equations. The bifurcation analysis and numerical simulation carried out in this work reveal the existence of stationary and dynamic patterns appearing due to the loss of stability of the coexistence homogeneous steady-state.
6.8.4 Dynamics of a diffusive two-prey-one-predator model with nonlocal intra-specific competition for both the prey species
Investigation of interacting populations is an active area of research, and various modeling approaches have been adopted to describe their dynamics. Mathematical models of such interactions using differential equations are capable to mimic the stationary and oscillating (regular or irregular) population distributions. Recently, some researchers have paid their attention to explain the consequences of transient dynamics of population density (especially the long transients) and able to capture such behaviors with simple models. Existence of multiple stationary patches and settlement to a stable distribution after a long quasi-stable transient dynamics can be explained by spatiotemporal models with nonlocal interaction terms. However, the studies of such interesting phenomena for three interacting species are not abundant in literature. Motivated by these facts here we have considered in 19 a three species prey-predator model where the predator is generalist in nature as it survives on two prey species. Nonlocalities are introduced in the intra-specific competition terms for the two prey species in order to model the accessibility of nearby resources. Using linear analysis, we have derived the Turing instability conditions for both the spatiotemporal models with and without nonlocal interactions. Validation of such conditions indicates the possibility of existence of stationary spatially heterogeneous distributions for all the three species. Existence of long transient dynamics has been presented under certain parametric domain. Exhaustive numerical simulations reveal various scenarios of stabilization of population distribution due to the presence of nonlocal intra-specific competition for the two prey species. Chaotic oscillation exhibited by the temporal model is significantly suppressed when the populations are allowed to move over their habitat and prey species can access the nearby resources.
6.9 Periodic waves in neural field models
6.9.1 Dynamics of periodic waves in a neural field model
Periodic traveling waves are observed in various brain activities, including visual, motor, language, sleep, and so on. There are several neural field models describing periodic waves assuming nonlocal interaction, and possibly, inhibition, time delay or some other properties. In 8, we study the influences of asymmetric connectivity functions and of time delay for symmetric connectivity functions on the emergence of periodic waves and their properties. Nonlinear wave dynamics are studied, including modulated and aperiodic waves. Multiplicity of waves for the same values of parameters is observed. External stimulation in order to restore wave propagation in a damaged tissue is discussed.
6.9.2 Nonlinear analysis of periodic waves in a neural field model
In the paper 14, we consider the possible role of epicenters and explore a neural field model with two nonlinear integrodifferential equations for the distributions of activating and inhibiting signals. It is studied with symmetric connectivity functions characterizing signal exchange between two populations of neurons, excitatory and inhibitory. Bifurcation analysis is used to investigate the emergence of periodic traveling waves and of standing oscillations from the stationary, spatially homogeneous solutions, and the stability of these solutions. Both types of solutions can be started by local oscillations indicating a possible role of epicenters in the initiation of wave propagation.
6.9.3 Cortical stimulation in aphasia following ischemic stroke
The aim of the paper 11 is to integrate different bodies of research including brain traveling waves, brain neuromodulation, neural field modeling and post-stroke language disorders in order to explore the opportunity of implementing model-guided, cortical neuromodulation for the treatment of post-stroke aphasia. Worldwide according to WHO, strokes are the second leading cause of death and the third leading cause of disability. In ischemic stroke, there is not enough blood supply to provide enough oxygen and nutrients to parts of the brain, while in hemorrhagic stroke, there is bleeding within the enclosed cranial cavity. The present paper focuses on ischemic stroke. We first review accumulating observations of traveling waves occurring spontaneously or triggered by external stimuli in healthy subjects as well as in patients with brain disorders. We examine the putative functions of these waves and focus on post-stroke aphasia observed when brain language networks become fragmented or partly silent, thus perturbing the progression of traveling waves across perilesional areas. Secondly, we focus on a simplified model based on the current literature in the field and describe cortical traveling wave dynamics and their modulation. This model uses a biophysically realistic integrodifferential equation describing spatially distributed and synaptically coupled neural networks producing traveling wave solutions. The model is used to calculate wave parameters (speed, amplitude and frequency) and to guide the reconstruction of the perturbed wave. A stimulation term is included in the model to restore wave propagation to a reasonably good level. Thirdly, we examine various issues related to the implementation model-guided neuromodulation in the treatment of post-stroke aphasia given that closed-loop invasive brain stimulation studies have recently produced encouraging results. Finally, we suggest that modulating traveling waves by acting selectively and dynamically across space and time to facilitate wave propagation is a promising therapeutic strategy especially at a time when a new generation of closed-loop cortical stimulation systems is about to arrive on the market.
6.10 Radioprotective effects during human torpor on deep space missions
Human metabolic suppression is not a new concept, with 1950s scientific literature and movies demonstrating its potential use for deep space travel (Hock, 1960). An artificially induced state of metabolic suppression in the form of torpor would improve the amount of supplies required and therefore lessen weight and fuel required for missions to Mars and beyond (Choukèr et al., 2019). Transfer habitats for human stasis to Mars have been conceived (Bradford et al., 2018). Evidence suggests that animals, when hibernating, demonstrate relative radioprotection compared to their awake state. Experiments have also demonstrated relative radioprotection in conditions of hypothermia as well as during sleep (Bellesi et al., 2016 and Andersen et al., 2009). Circadian rhythm disrupted cells also appear to be more susceptible to radiation damage compared to those that are under a rhythmic control (Dakup et al., 2018).
An induced torpor state for astronauts on deep space missions may provide a biological radioprotective state due to a decreased metabolism and hypothermic conditions. A regular enforced circadian rhythm might further limit DNA damage from radiation.
The As Low As Reasonably Achievable (A.L.A.R.A.) radiation protection concept defines time, distance and shielding as ways to decrease radiation exposure. Whilst distance cannot be altered in space and shielding either passively or actively may be beneficial, time of exposure may be drastically decreased with improved propulsion systems. Whilst chemical propulsion systems have superior thrust to other systems, they lack high changes in velocity and fuel efficiency which can be achieved with nuclear or electric based propulsion systems.
Radiation toxicity could be limited by reduced transit times, combined with the radioprotective effects of enforced circadian rhythms during a state of torpor or hibernation.
The objectives of the paper 24 are, first to investigate how the circadian clock and body temperature may contribute to radioprotection during human torpor on deep space missions, and second to estimate radiation dose received by astronauts during a transit to Mars with varying propulsion systems.
We simulated three types of conditions to investigate the potential radioprotective effect of the circadian clock and decreased temperature on cells being exposed to radiation such that may be the case during astronaut torpor. These conditions were: 1) Circadian clock strength: strong vs weak; 2) Light exposure: dark-dark vs light-dark cycle; 3) Body temperature: 37C vs hypothermia vs torpor.
We estimated transit times for a mission to Mars from Earth utilizing chemical, nuclear and electrical propulsion systems. Transit times were generated using the General Mission Analysis Tool (GMAT) and Matlab. These times were then input into the National Aeronautics and Space Administration (NASA) Online Tool for the Assessment of Radiation In Space (OLTARIS) computer simulator to estimate doses received by an astronaut for the three propulsion methods.
Our simulation demonstrated an increase in radioprotection with decreasing temperature. The greatest degree of radioprotection was shown in cells that maintained a strong circadian clock during torpor. This was in contrast to relatively lower radioprotection in cells with a weak clock during normothermia. We were also able to demonstrate that if torpor weakened the circadian clock, a protective effect could be partially restored by an external drive such as lighting schedules to aid entrainment i.e.: Blue light exposure for periods of awake and no light for rest times. For the propulsion simulation, estimated transit times from Earth to Mars were 258 days for chemical propulsion with 165.9mSv received, 209 days for nuclear propulsion with 134.4mSv received and 80 days for electrical propulsion with 51.4mSv received.
A state of torpor for astronauts on deep space missions may not only improve weight, fuel and storage requirements but also provide a potential biological radiation protection strategy. Moreover, maintaining a controlled circadian rhythm during torpor conditions may aid radioprotection. In the not too distant future, propulsion techniques will be improved to limit transit time and hence decrease radiation dose to astronauts. Limiting exposure time and enhancing physiological radioprotection during transit could provide superior radioprotection benefits compared with active and passive radiation shielding strategies alone.
6.11 Dynamics of solutions of reaction-diffusion equations and non‐Fredholm integro‐differential equations
6.11.1 Dynamics of solutions of a reaction-diffusion equation with delayed inhibition
Reaction-diffusion equation with a logistic production term and a delayed inhibition term is studied in 21. Global stability of the homogeneous in space equilibrium is proved under some conditions on the delay term. In the case where these conditions are not satisfied, this solution can become unstable resulting in the emergence of spatiotemporal pattern formation studied in numerical simulations.
6.11.2 Existence of solutions for some non-Fredholm integro-differential equations with the bi-Laplacian
In the paper 29, we prove the existence of solutions for some semilinear elliptic equations in the appropriate spaces using the fixed‐point technique where the elliptic equation contains fourth-order differential operators with and without Fredholm property, generalizing the previous results.
6.11.3 Dynamics of convective thermal explosion in porous media
In the paper 3, we study complex dynamics of the interaction between natural convection and thermal explosion in porous media. This process is modeled with the nonlinear heat equation coupled with the nonstationary Darcy equation under the Boussinesq approximation for a fluid-saturated porous medium in a rectangular domain. Numerical simulations with the Radial Basis Functions Method (RBFM) reveal complex dynamics of solutions and transitions to chaos after a sequence of period doubling bifurcations. Several periodic windows alternate with chaotic regimes due to intermittence or crisis. After the last chaotic regime, a final periodic solution precedes transition to thermal explosion.
7 Partnerships and cooperations
7.1 International initiatives
7.1.1 Inria associate team not involved in an IIL
MathModelingHematopoiesis
- Title: Mathematical modeling of hematopoietic stem cell dynamics in normal and pathological hematopoiesis with optimal control for drug therapy
- Duration: 2019 - 2020
- Coordinator: Mostafa Adimy
- Partners: Department of Mathematics, Presidency University, Kolkata (India)
- Inria contact: Mostafa Adimy
-
Summary:
The project proposes to develop and analyse new mathematical models of Hematopoietic Stem Cell population dynamics in normal and pathological hematopoiesis. Two important questions will be explored in this project: i) the biological data concerning the hematopoiesis process evolves constantly, and new understanding modifies the established mathematical models, ii) modeling constraints us to simplify the complicated biological scenarios, which moving away from the reality, but enabling us to reach a certain comprehension of the hematopoiesis process.
The project will shed new light on the different physiological mechanisms that converge toward the continuous regeneration of blood cells, for example: the behavior of hematopoietic stem cells under stress conditions, the understanding of deregulation of erythropoiesis under drug treatments (this can lead to lack of red blood cells (anemia), or a surplus of red blood cells (erythrocytoses)), the appearance of oscillations in patients with Chronic Myeloid Leukemia (CML); Or, the overproduction of blasts in patients with Acute Myeloid Leukemia (AML)). The effect of the immune system and drug therapy in the presence of CML or AML will be included in the model and optimal control method will also be used.
7.2 European initiatives
7.2.1 FP7 & H2020 Projects
COSMIC
- Title: COmbatting disorders of adaptive immunity with Systems MedICine
- Duration: 2018-2021
- Coordinator: Antpoine van Kampen
-
Partners:
- Bioinformatics Laboratory, Dept. of Pathology and Rheumatology and clinical immunology, ACADEMISCH MEDISCH CENTRUM BIJ DE UNIVERSITEIT VAN AMSTERDAM (Netherlands)
- ANAXOMICS BIOTECH S.L. (ANX) (Spain)
- Biotecture (Netherlands)
- ELEVATE BV (Netherlands)
- ETHNIKO KENTRO EREVNAS KAI TECHNOLOGIKIS ANAPTYXIS (Greece)
- HELMHOLTZ-ZENTRUM FUR INFEKTIONSFORSCHUNG GMBH (Germany)
- IBM RESEARCH GMBH (Switzerland)
- KAROLINSKA INSTITUTET (Sweden)
- REDOXIS AB (Sweden)
- Inria contact: Olivier Gandrillon
- Summary: COSMIC delivers the next generation of systems medicine professionals who successfully combat complex human disorders. We will focus on B-cell lymphoma and rheumatoid arthritis, diseases that originate from abnormal functioning of the adaptive immune system, in particular the germinal center. COSMIC develops and integrates experimental and computational approaches, and establishes a unique cross-fertilization between oncology and auto-immunity.
7.3 National initiatives
7.3.1 ANR
- ANR SinCity “Single cell transcriptomics on genealogically identified differentiating cells” (https://
anr. fr/ Projet-ANR-17-CE12-0031), 2017-2020. Participants: Olivier Gandrillon.
- Olivier Gandrillon participates in the ANR MEMOIRE (head Jacqueline Marvel) dedicated to “MultiscalE MOdeling of CD8 T cell Immune REsponses”, 2018-2021.
7.3.2 Other projects
- Thomas Lepoutre is a member of the ERC MESOPROBIO (head Vincent Calvez) dedicated to "Mesoscopic models for propagation in biology", 2015-2020: (http://
vcalvez. perso. math. cnrs. fr/ mesoprobio. html).
7.4 Regional initiatives
The Région ARA project INGERENCE dedicated to “INferring GEne REgulatory NEtworks from single CEll Data to improve vaccine design", 2018-2021.
Participants : Olivier Gandrillon, Fabien Crauste [Coordinator].
8 Dissemination
8.1 Teaching
- Licence: Laurent Pujo-Menjouet, Fondamentaux des mathématiques, 138h EQTD, L1, UCBL 1
- Licence: Laurent Pujo-Menjouet, 3ème année biosciences BIM, Systèmes Dynamiques et EDP, 45h EQTD, INSA Lyon
- Licence : Léon Tine, Techniques mathématiques de base, 53h (EqTD), niveau L0, UCBL 1
- Licence : Léon Tine, Techniques mathématiques de base, 62h (EqTD), niveau L1, UCBL 1
- Licence : Léon Tine, Initiation LaTex+ stage, 12h (EqTD), niveau L3, UCBL 1
- Licence : Vincent Calvez, Cours de math pour étudiants médecins, cursus Médecine-Sciences (2emr année fac de médecine), 40h, Lyon Est/Sud
- Master : Samuel Bernard, Population Dynamics, 36h ETD, M2, UCBL 1
- Master : Mostafa Adimy, Population Dynamics, 9h ETD, M2, UCBL, UCBL 1
- Master : Mostafa Adimy, Epidemiology, 21h ETD, M2, UCBL, UCBL 1
- Master: Thomas Lepoutre, préparation à l'option pour l'agrégation, 45 h eq TD, M2 UCBL 1
- Master: Laurent Pujo-Menjouet, Systèmes Dynamiques, 72 h EQTD, M1, UCBL1
- Master: Laurent Pujo-Menjouet, Systèmes complexes: modelling biology and medicine, M2, 9h EQTD, ENS-Lyon
- Master: Laurent Pujo-Menjouet, 4ème année biosciences BIM: ED-EDP, 24h EQTD, INSA Lyon
- Master: Léon Tine, Maths en action, Remise à niveau analyse, 12h (EqTD), niveau M2, UCBL 1
- Master: Léon Tine, Maths en action, épidémiologie, 18h (EqTD), niveau M2, UCBL 1
- Master: Vincent Calvez, Modèles mathématiques et analyse pour Ecologie et Evolution, 24h, niveau M2 avancé, ENS-Lyon
8.2 Supervision
- PhD in progress: Kyriaki Dariva , “Modélisation mathématique des interactions avec le système immunitaire en leucémie myéloide chronique”. Université Lyon 1, since September 2018, supervisor: Thomas Lepoutre
- PhD in progress: Alexey Koshkin, “Inferring gene regulatory networks from single cell data”, ENS de Lyon, since September 2018, supervisors: Olivier Gandrillon and Fabien Crauste
- PhD in progress: Paul Lemarre, “Modélisation des souches de prions”. Université Lyon 1, since May 2017, supervisors: Laurent Pujo-Menjouet et Suzanne Sindi (University of California, Merced)
- PhD in progress: Léonard Dekens, “Adaptation d'une population avec une reproduction sexuée à un environnement hétérogène : modélisation mathématique et analyse asymptotique dans un régime de petite variance”, Université Lyon 1, since September 2019, supervisor: Vincent Calvez.
- PhD in progress: Mete Demircigil, “Etude du mouvement collectif chez Dictyostelium Discoideum et autres espèces. Modélisation, Analyse et Simulation”, ENS-Lyon, since September 2019, supervisor: Vincent Calvez.
- PhD in progress: Ghada Abi Younes, “Modélisation mathématique des maladies inflammatoires”, Université Lyon, since November 2019, supervisor: Vitaly Volpert.
- PhD in progress: Elias Ventre, “Paysage et trajectoire - des réseaux de régulation génique aux trajectoires cellulaires", ENS, since October 2019, supervisors: Olivier Gandrillons and Thibault Espinasse.
- PhD in progress: Cheikh Gueye, “Problèmes inverses pour l'estimation de paramètre de modèles mathématiques”, Université Lyon, since October 2019, supervisors: Laurent Pujo-Menjouet and Léon Tine.
9 Scientific production
9.1 Publications of the year
International journals
Reports & preprints
9.2 Cited publications
- 35 articleStability Analysis of a Model of Interaction Between the Immune System and Cancer Cells in Chronic Myelogenous LeukemiaBulletin of Mathematical Biology2017, 1--27
- 36 articleLong-term treatment effects in chronic myeloid leukemiaJournal of Mathematical Biology2017, 1-26
- 37 articleImplication of the Autologous Immune System in BCR-ABL Transcript Variations in Chronic Myelogenous Leukemia Patients Treated with Imatinib.Cancer Research7519October 2015, 4053-62
- 38 articleIdentification of Nascent Memory CD8 T Cells and Modeling of Their OntogenyCell Systems43February 2017, 306-317
- 39 articleInferring gene regulatory networks from single-cell data: a mechanistic approachBMC Systems Biology111November 2017, 105