Keywords
Computer Science and Digital Science
- A3.1.1. Modeling, representation
- A3.4.5. Bayesian methods
- A6.1.1. Continuous Modeling (PDE, ODE)
- A6.1.2. Stochastic Modeling
- A6.2.1. Numerical analysis of PDE and ODE
- A6.2.4. Statistical methods
- A6.3.1. Inverse problems
- A6.3.2. Data assimilation
- A6.3.3. Data processing
- A6.4.1. Deterministic control
Other Research Topics and Application Domains
- B1. Life sciences
- B1.1.2. Molecular and cellular biology
- B1.1.4. Genetics and genomics
- B1.1.7. Bioinformatics
- B1.1.8. Mathematical biology
- B1.1.10. Systems and synthetic biology
- B4.3.1. Biofuels
1 Team members, visitors, external collaborators
Research Scientists
- Hidde de Jong [Team leader, Inria, Senior Researcher, HDR]
- Eugenio Cinquemani [Inria, Researcher, HDR]
- Aline Marguet [Inria, Researcher]
- Delphine Ropers [Inria, Researcher]
Faculty Members
- Johannes Geiselmann [Univ Grenoble Alpes, Professor, HDR]
- Stephan Lacour [Univ Grenoble Alpes, Associate Professor]
- Yves Markowicz [Univ Grenoble Alpes, Associate Professor]
- Michel Page [Univ Grenoble Alpes, Associate Professor]
Post-Doctoral Fellow
- Thibault Etienne [Inria, from Dec 2020]
PhD Students
- Thibault Clavier [Univ Grenoble Alpes, From Oct 2020]
- Thibault Etienne [INRAE/Inria, until Mar 2020]
- Antrea Pavlou [Inria/Univ Grenoble Alpes]
- Emrys Reginato [Inria/Univ Grenoble Alpes, from Oct 2020]
- Maaike Sangster [Inria/Univ Grenoble Alpes]
Technical Staff
- Tamas Muszbek [Inria, Engineer]
- Corinne Pinel [CNRS, Engineer]
Interns and Apprentices
- Tommy Burnoud [Inria, Intern, from Feb 2020 until Jun 2020]
- Ignacia Cancino Aguirre [Inria, Intern, until Jun 2020]
- Thibault Clavier [Université Grenoble Alpes, Intern, From Feb 2020 until Jul 2020]
- Alan Flatres [Inria, Intern, from Apr 2020 until Jul 2020]
- Achille Fraisse [École Normale Supérieure de Lyon, Intern, from Apr 2020 until Jul 2020]
Administrative Assistant
- Diane Courtiol [Inria]
External Collaborator
- Thibault Etienne [Univ Claude Bernard, from Jun 2020 until Nov 2020]
2 Overall objectives
2.1 Overview
When confronted with changing environmental conditions, bacteria and other microorganisms have a remarkable capacity to adapt their functioning. The responses of bacteria to changes in their environment are controlled on the molecular level by large and complex networks of biochemical interactions involving genes, mRNAs, proteins, and metabolites. The study of bacterial regulatory networks requires experimental tools for mapping the interaction structure of the networks and measuring the dynamics of cellular processes. In addition, when dealing with such large and complex systems, we need mathematical modeling and computer simulation to integrate available biological data, and understand and predict the dynamics of the system under various physiological and genetic perturbations. The analysis of living systems through the combined application of experimental and computational methods has gathered momentum in recent years under the name of systems biology.
The first aim of the IBIS project-team is to apply such a systems-biology approach to gain a deeper understanding, on the mechanistic level, of the strategies that bacteria have developed to respond to changes in their environment. 1 In particular, we focus on the enterobacterium Escherichia coli, for which enormous amounts of genomic, genetic, biochemical and physiological data have accumulated over the past decades. A better understanding of the adaptive capabilities of E. coli to nutritional limitations or other environmental changes is an aim in itself, but also a necessary prerequisite for the second and most ambitious aim of the project: interfering with the cellular responses by specific perturbations or by rewiring the underlying regulatory networks. This does not only spawn fundamental research on the control of living matter, but may ultimately also lead to practical applications. Because E. coli is easy to manipulate in the laboratory, it serves as a model for many pathogenic bacteria and is widely used in biotechnology, for such diverse applications as the development of vaccines, the mass production of enzymes and other (heterologous) proteins, and the production of biofuels.
The aims of IBIS raise new questions on the interface of biology, applied mathematics, and computer science. In particular, the following objectives have structured the work of the project-team: (1) the analysis of the qualitative dynamics of gene regulatory networks, (2) the inference of gene regulatory networks from time-series data, (3) the analysis of integrated metabolic and regulatory networks, and (4) natural and engineered control of regulatory networks. Although these axes cover most of the work carried out in IBIS, some members have also made contributions to research projects on different topics. Since this usually represents a minor proportion of the overall research effort of the project-team, we will not describe this work in detail in the activity report. The publications resulting from these side-tracks have been included in the bibliography.
The challenges of the research programme of the IBIS team require a wide range of competences on the interface of (experimental) biology, applied mathematics, and computer science (Figure 1). Since no single person can be expected to possess all of these competences, the international trend in systems biology is to join researchers from different disciplines into a single group. In line with this development, the IBIS team is a merger of a microbiology and molecular genetics group on the one hand, and a bioinformatics and mathematical biology group on the other hand. In particular, the IBIS team is composed of members of the group of Johannes Geiselmann, formerly at the Laboratoire Adaptation et Pathogénicité des Microorganismes of the Univ Joseph Fourier (UJF, CNRS UMR 5163), and since September 2014 at the Laboratoire Interdisciplinaire de Physique (CNRS UMR 5588), and the members of the network modeling and simulation group formerly part of the HELIX project-team at Inria Grenoble - Rhône-Alpes, a group coordinated by Hidde de Jong. The two groups have established a fruitful collaboration, which has resulted in more than 80 peer-reviewed publications in journals, conferences, and books since 2000. 2
Hidde de Jong is the head of the IBIS project-team and Johannes Geiselmann its co-director. The experimental component of IBIS is also part of the Laboratoire Interdisciplinaire de Physique, and Johannes Geiselmann continues to represent this group in the interactions with the laboratory and university administration.
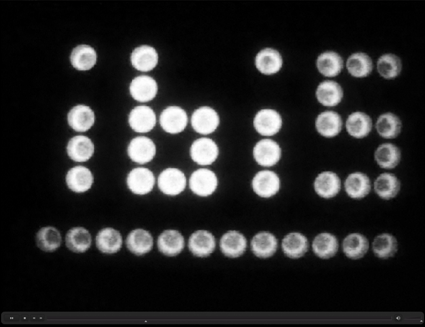
Display of the project-team name on a "bacterial billboard" (see http://
3 Research program
3.1 Analysis of qualitative dynamics of gene regulatory networks
Participants: Hidde de Jong, Michel Page, Delphine Ropers.
The dynamics of gene regulatory networks can be modeled by means of ordinary differential equations (ODEs), describing the rate of synthesis and degradation of the gene products as well as regulatory interactions between gene products and metabolites. In practice, such models are not easy to construct though, as the parameters are often only constrained to within a range spanning several orders of magnitude for most systems of biological interest. Moreover, the models usually consist of a large number of variables, are strongly nonlinear, and include different time-scales, which makes them difficult to handle both mathematically and computationally. This has motivated the interest in qualitative models which, from incomplete knowledge of the system, are able to provide a coarse-grained picture of its dynamics.
A variety of qualitative modeling formalisms have been introduced over the past decades. Boolean or logical models, which describe gene regulatory and signalling networks as discrete-time finite-state transition systems, are probably most widely used. The dynamics of these systems are governed by logical functions representing the regulatory interactions between the genes and other components of the system. IBIS has focused on a related, hybrid formalism that embeds the logical functions describing regulatory interactions into an ODE formalism, giving rise to so-called piecewise-linear differential equations (PLDEs, Figure 2). The use of logical functions allows the qualitative dynamics of the PLDE models to be analyzed, even in high-dimensional systems. In particular, the qualitative dynamics can be represented by means of a so-called state transition graph, where the states correspond to (hyperrectangular) regions in the state space and transitions between states arise from solutions entering one region from another.
First proposed by Leon Glass and Stuart Kauffman in the early seventies, the mathematical analysis of PLDE models has been the subject of active research for more than four decades. IBIS has made contributions on the mathematical level, in collaboration with the BIOCORE and BIPOP project-teams, notably for solving problems induced by discontinuities in the dynamics of the system at the boundaries between regions, where the logical functions may abruptly switch from one discrete value to another, corresponding to the (in)activation of a gene. In addition, many efforts have gone into the development of the computer tool Genetic Network Analyzer (GNA) and its applications to the analysis of the qualitative dynamics of a variety of regulatory networks in microorganisms. Some of the methodological work underlying GNA, notably the development of analysis tools based on temporal logics and model checking, which was carried out with the Inria project-teams CONVEX (ex-VASY) and POP-ART, has implications beyond PLDE models as they apply to logical and other qualitative models as well.
![]() |
|
(Left) Example of a gene regulatory network of two genes (a and b), each of which codes for a regulatory protein (A and B). Protein B inhibits the expression of gene a, while protein A inhibits the expression of gene b and its own gene. (Right) PLDE model corresponding to the network in (a). Protein A is synthesized at a rate , if and only if the concentration of protein A is below its threshold () and the concentration of protein B below its threshold (). The degradation of protein A occurs at a rate proportional to the concentration of the protein itself ().
3.2 Inference of gene regulatory networks from time-series data
Participants: Eugenio Cinquemani, Johannes Geiselmann, Hidde de Jong, Stéphan Lacour, Aline Marguet, Michel Page, Corinne Pinel, Delphine Ropers.
Measurements of the transcriptome of a bacterial cell by means of DNA microarrays, RNA sequencing, and other technologies have yielded huge amounts of data on the state of the transcriptional program in different growth conditions and genetic backgrounds, across different time-points in an experiment. The information on the time-varying state of the cell thus obtained has fueled the development of methods for inferring regulatory interactions between genes. In essence, these methods try to explain the observed variation in the activity of one gene in terms of the variation in activity of other genes. A large number of inference methods have been proposed in the literature and have been successful in a variety of applications, although a number of difficult problems remain.
Current reporter gene technologies, based on Green Fluorescent Proteins (GFPs) and other fluorescent and luminescent reporter proteins, provide an excellent means to measure the activity of a gene in vivo and in real time (Figure 3). The underlying principle of the technology is to fuse the promoter region and possibly (part of) the coding region of a gene of interest to a reporter gene. The expression of the reporter gene generates a visible signal (fluorescence or luminescence) that is easy to capture and reflects the expression of a gene of interest. The interest of the reporter systems is further enhanced when they are applied in mutant strains or combined with expression vectors that allow the controlled induction of any particular gene, or the degradation of its product, at a precise moment during the time-course of the experiment. This makes it possible to perturb the network dynamics in a variety of ways, thus obtaining precious information for network inference.
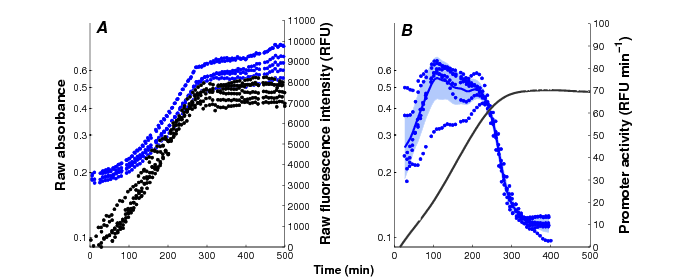
Monitoring of bacterial gene expression in vivo using fluorescent reporter genes (Stefan et al., PLoS Computational Biology, 11(1):e1004028, 2015). The plots show the primary data obtained in a kinetic experiment with E. coli cells, focusing on the expression of the motility gene tar in a mutant background. A: Absorbance (, black) and fluorescence (, blue) data, corrected for background intensities, obtained with the cpxR strain transformed with the ptar-gfp reporter plasmid and grown in M9 with glucose. B: Activity of the tar promoter, computed from the primary data. The solid black line corresponds to the mean of 6 replicate absorbance measurements and the shaded blue region to the mean of the promoter activities twice the standard error of the mean.
The specific niche of IBIS in the field of network inference has been the development and application of genome engineering techniques for constructing the reporter and perturbation systems described above, as well as the use of reporter gene data for the reconstruction of gene regulation functions. We have developed an experimental pipeline that resolves most technical difficulties in the generation of reproducible time-series measurements on the population level. The pipeline comes with data analysis software that converts the primary data into measurements of time-varying promoter activities. In addition, for measuring gene expression on the single-cell level by means of microfluidics and time-lapse fluorescence microscopy, we have established collaborations with groups in Grenoble and Paris. The data thus obtained can be exploited for the structural and parametric identification of gene regulatory networks, for which methods with a solid mathematical foundation are developed, in collaboration with colleagues at ETH Zürich and EPF Lausanne (Switzerland). The vertical integration of the network inference process, from the construction of the biological material to the data analysis and inference methods, has the advantage that it allows the experimental design to be precisely tuned to the identification requirements.
3.3 Analysis of integrated metabolic and gene regulatory networks
Participants: Eugenio Cinquemani, Hidde de Jong, Thibault Etienne, Johannes Geiselmann, Stéphan Lacour, Yves Markowicz, Michel Page, Corinne Pinel, Delphine Ropers.
The response of bacteria to changes in their environment involves responses on several different levels, from the redistribution of metabolic fluxes and the adjustment of metabolic pools to changes in gene expression. In order to fully understand the mechanisms driving the adaptive response of bacteria, as mentioned above, we need to analyze the interactions between metabolism and gene expression. While often studied in isolation, gene regulatory networks and metabolic networks are closely intertwined. Genes code for enzymes which control metabolic fluxes, while the accumulation or depletion of metabolites may affect the activity of transcription factors and thus the expression of enzyme-encoding genes.
The fundamental principles underlying the interactions between gene expressions and metabolism are far from being understood today. From a biological point of view, the problem is quite challenging, as metabolism and gene expression are dynamic processes evolving on different time-scales and governed by different types of kinetics. Moreover, gene expression and metabolism are measured by different experimental methods generating heterogeneous, and often noisy and incomplete data sets. From a modeling point of view, difficult methodological problems concerned with the reduction and calibration of complex nonlinear models need to be addressed.
Most of the work carried out within the IBIS project-team specifically addressed the analysis of integrated metabolic and gene regulatory networks in the context of E. coli carbon metabolism (Figure 4). While an enormous amount of data has accumulated on this model system, the complexity of the regulatory mechanisms and the difficulty to precisely control experimental conditions during growth transitions leave many essential questions open, such as the physiological role and the relative importance of mechanisms on different levels of regulation (transcription factors, metabolic effectors, global physiological parameters, ). We are interested in the elaboration of novel biological concepts and accompanying mathematical methods to grasp the nature of the interactions between metabolism and gene expression, and thus better understand the overall functioning of the system. Moreover, we have worked on the development of methods for solving what is probably the hardest problem when quantifying the interactions between metabolism and gene expression: the estimation of parameters from hetereogeneous and noisy high-throughput data. These problems are tackled in collaboration with experimental groups at Inra/INSA Toulouse and CEA Grenoble, which have complementary experimental competences (proteomics, metabolomics) and biological expertise.
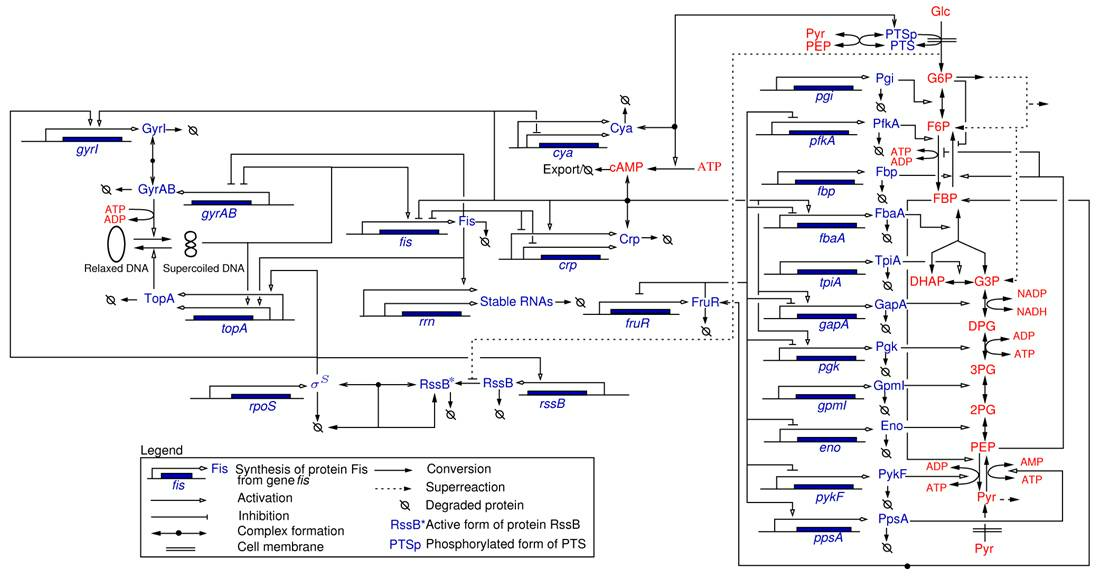
Network of key genes, proteins, and regulatory interactions involved in the carbon assimilation network in E. coli (Baldazzi et al., PLoS Computational Biology, 6(6):e1000812, 2010). The metabolic part includes the glycolysis/gluconeogenesis pathways as well as a simplified description of the PTS system, via the phosphorylated and non-phosphorylated form of its enzymes (represented by PTSp and PTS, respectively). The pentose-phosphate pathway (PPP) is not explicitly described but we take into account that a small pool of G6P escapes the upper part of glycolysis. At the level of the global regulators the network includes the control of the DNA supercoiling level, the accumulation of the sigma factor RpoS and the CrpcAMP complex, and the regulatory role exerted by the fructose repressor FruR.
3.4 Natural and engineered control of growth and gene expression
Participants: Eugenio Cinquemani, Thibault Clavier, Johannes Geiselmann, Hidde de Jong, Stéphan Lacour, Tamas Muszbek, Michel Page, Antrea Pavlou, Delphine Ropers, Maaike Sangster.
The adaptation of bacterial physiology to changes in the environment, involving changes in the growth rate and a reorganization of gene expression, is fundamentally a resource allocation problem. It notably poses the question how microorganisms redistribute their protein synthesis capacity over different cellular functions when confronted with an environmental challenge. Assuming that resource allocation in microorganisms has been optimized through evolution, for example to allow maximal growth in a variety of environments, this question can be fruitfully formulated as an optimal control problem. We have developed such an optimal control perspective, focusing on the dynamical adaptation of growth and gene expression in response to envrionmental changes, in close collaboration with the BIOCORE project-team.
A complementary perspective consists in the use of control-theoretical approaches to modify the functioning of a bacterial cell towards a user-defined objective, by rewiring and selectively perturbing its regulatory networks. The question how regulatory networks in microorganisms can be externally controlled using engineering approaches has a long history in biotechnology and is receiving much attention in the emerging field of synthetic biology. Within a number of on-going projects, IBIS is focusing on two different questions. The first concerns the development of open-loop and closed-loop growth-rate controllers of bacterial cells for both fundamental research and biotechnological applications (Figure 5). Second, we are working on the development of methods for the real-time control of the expression of heterologous proteins in communities of interacting bacterial populations. The above projects involve collaborations with, among others, the Inria project-teams LIFEWARE (INBIO), BIOCORE, and McTAO as well as with a biophysics group at Univ Paris Descartes and a mathematical modeling group at INRA Jouy-en-Josas.
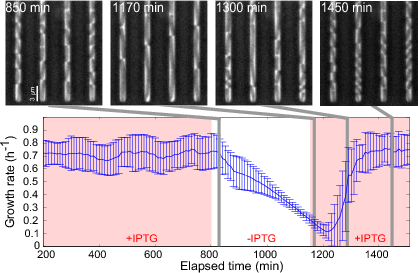
Growth arrest by external control of the gene expression machinery (Izard, Gomez Balderas et al., Molecular Systems Biology, 11:840, 2015). An E. coli strain in which an essential component of the gene expression machinery, the subunits of RNA polymerase, was put under the control of an externally-supplied inducer (IPTG), was grown in a microfluidics device and phase-contrast images were acquired every 10 min. The cells were grown in minimal medium with glucose, initially in the presence of 1 mM IPTG. 6 h after removing IPTG from the medium, the growth rate slows down and cells are elongated. About 100 min after adding back 1 mM IPTG into the medium, the elongated cells divide and resume normal growth. The growth rates in the plot are the (weighted) mean of the growth rates of 100 individual cells. The error bars correspond to one standard deviation. The results of the experiment show that the growth rate of a bacterial can be switched off in a reversible manner by an external inducer, based on the reengineering of the natural control of the expression of RNA polymerase.
4 Application domains
The research questions in IBIS concern problems at the interface of microbiology, computer science, and mathematics, with applications in biotechnology.
5 Highlights of the year
The results of the PhD theses of Thibault Etienne and Manon Morin on the degradation of mRNA in bacteria, co-supervised by Muriel Cocaign-Bousquet and Delphine Ropers, have been selected as a highlight of the year by INRAE (https://
6 New software and platforms
6.1 New software
6.1.1 WellFARE
- Name: WellFARE
- Keywords: Bioinformatics, Statistics, Data visualization, Data modeling
- Scientific Description: WellFARE is a Python library implementing linear inversion methods for the reconstruction of gene expression profiles from fluorescent or luminescent reporter gene data. WellFARE form the computational core of the WellInverter web application.
- Functional Description: As input, WellFARE reads the primary data file produced by a 96-well microplate reader, containing time-series measurements of the absorbance (optical density) as well as the fluorescence and luminescence intensities in each well (if available). Various functions exist to analyze the data, in particular for detecting outliers, subtracting background, estimating growth rates, promoter activities and protein concentrations, visualizing expression profiles, synchronizing replicate profiles, etc. WellFARE is the computational core of the web application WellInverter.
- News of the Year: Publication in BMC Bioinformatics describing the new version of WellFARE
-
URL:
https://
github. com/ ibis-inria/ wellfare - Publication: hal-01217800
- Authors: Valentin Zulkower, Hidde de Jong, Michel Page, Valentin Zulkower, Yannick Martin, Delphine Ropers, Hans Geiselmann
- Contacts: Hidde de Jong, Valentin Zulkower
- Participants: Delphine Ropers, Hans Geiselmann, Hidde de Jong, Michel Page, Valentin Zulkower, Yannick Martin
- Partner: UGA
6.1.2 WellInverter
- Name: WellInverter
- Keywords: Bioinformatics, Statistics, Data visualization, Data modeling
- Scientific Description: WellInverter is a web application that implements linear inversion methods for the reconstruction of gene expression profiles from fluorescent or luminescent reporter gene data. WellInverter makes the methods available to a broad audience of biologists and bioinformaticians. In particular, we have put in place a parallel computing architecture with a load balancer to distribute the analysis queries over several back-end servers, redesigned the graphical user interface, and developed a plug-in system for defining high-level routines for parsing data files produced by microplate readers from different manufacturers.
- Functional Description: As input, WellInverter reads the primary data file produced by a 96-well microplate reader, containing time-series measurements of the absorbance (optical density) as well as the fluorescence and luminescence intensities in each well (if available). Various modules exist to analyze the data, in particular for detecting outliers, subtracting background, estimating growth rates, promoter activities and protein concentrations, visualizing expression profiles, synchronizing replicate profiles, etc. The computational core of the web application consists of the Python library WellFARE.
- News of the Year: Deployment of WellInverter on an Inria server and on the new cloud of the French Institute for Bioinformatics (see the web page for details). Publication in BMC Bioinformatics describing the new version of the application.
-
URL:
https://
team. inria. fr/ ibis/ wellinverter/ - Publication: hal-01217800
- Authors: Yannick Martin, Michel Page, Hidde de Jong, Delphine Ropers, Johannes Geiselmann, Valentin Zulkower, Valentin Zulkower
- Contact: Hidde de Jong
- Participants: Delphine Ropers, Hans Geiselmann, Hidde de Jong, Johannes Geiselmann, Michel Page, Valentin Zulkower, Yannick Martin
- Partner: UGA
6.1.3 GNA
- Name: Genetic Network Analyzer
- Keywords: Model Checking, Bioinformatics, Gene regulatory networks, Qualitative simulation
- Scientific Description: Genetic Network Analyzer (GNA) is the implementation of methods for the qualitative modeling and simulation of gene regulatory networks developed in the IBIS project-team.
- Functional Description: The input of GNA consists of a model of the regulatory network in the form of a system of piecewise-linear differential equations (PLDEs), supplemented by inequality constraints on the parameters and initial conditions. From this information, GNA generates a state transition graph summarizing the qualitative dynamics of the system. In order to analyze large graphs, GNA allows the user to specify properties of the qualitative dynamics of a network in temporal logic, using high-level query templates, and to verify these properties on the state transition graph by means of standard model-checking tools, either locally installed or accessible through a remote web server.
- Release Contributions: (1) it supports the editing and visualization of regulatory networks, in an SBGN-compatible format, (2) it semi-automatically generates a prototype model from the network structure, thus accelerating the modeling process, and (3) it allows models to be exported in the SBML Qual standard.
- News of the Year: New mode of distribution from the IBIS web site. Tutorial on the use of the model formalism for analyzing synthetic genetic circuits, published in an edited book.
-
URL:
http://
www-helix. inrialpes. fr/ gna - Publications: hal-00762122, hal-01417975
- Authors: Céline Hernandez, Hidde de Jong, Michel Page
- Contact: Hidde de Jong
- Participants: Hidde de Jong, Michel Page, Delphine Ropers
- Partner: UGA
7 New results
7.1 Inference of parameters on lineage trees
Recent technological developments have made it possible to obtain time-course single-cell measurements of gene expression as well as the associated lineage information. However, most of the existing methods for the identification of mathematical models of gene expression are not well-suited to single-cell data and make the simplifying assumptions that cells in a population are independent, thus ignoring cell lineages. The development of statistical tools taking into account the correlations between individual cells will allow in particular for the investigation of inheritance of traits in bacterial populations.
Modelling and identification of gene expression models with mother-daughter inheritance have been investigated in the context of the soon-ending ANR project MEMIP (Section 8.2). In the previous year, collaboration among Eugenio Cinquemani, Marc Lavielle (XPOP, Inria Saclay–Île-de-France) and Aline Marguet brought to a publication in Bioinformatics (proceedings of ISMB/ECCB 2019) 9. Here, a novel method generalizing the Mixed-Effects (ME) paradigm to tree-structured populations, called ARME, was developed for the inference of an Auto-Regressive (AR) inheritance model of kinetic gene expression parameters. Leveraging single-cell gene expression time profiles and lineage information, the ability of the method to outperform alternative approaches was demonstrated on simulated data, and application to yeast osmotic shock data revealed a strong inheritance of gene expression kinetics along generations. For putative kinetic parameters, the method assumes deterministic gene expression dynamics, which constitutes a limitation for systems with strong gene expression noise. Work this year addressed the further generalization of the method to stochastic gene expression dynamics. Focusing on one extrinsic source of variability, that is, noisy promoter activation dynamics in response to an environmental stimulus common to all cells, we considered an augmented gene expression model where promoter activation is described by an Ornstein-Uhlenbeck process. We then extended the ARME approach so as to cope with this additional noise source via Kalman filtering techniques, and demonstrated in simulation that despite a simplifying assumption, the new method ourperforms its predecessor in case of significant promoter noise. The work has been submitted for presentation at the international IFAC conference SysId 2021. Further exploration of the subject, including inference of inheritance dynamics from different types of data, constitutes the subject of the recently started Ph.D. project of Emrys Reginato.
7.2 Mathematical analysis of structured branching populations
The investigation of cellular populations at a single-cell level has already led to the discovery of important phenomena, such as the occurrence of different phenotypes in an isogenic population. Nowadays, several experimental techniques, such as microscopy combined with the use of microfluidic devices, enable one to take investigation further by providing time-profiles of the dynamics of individual cells over entire lineage trees. The development of models that take into account the genealogy is an important step in the study of inheritance in bacterial population. And the efficient analysis of single-cell data relies on the mathematical analysis of those models.
Structured branching processes allows for the study of populations, where the lifecycle of each cell is governed by a given characteristic or trait, such as the internal concentration of proteins. The dependence on this characteristic of cellular mechanisms, such as division or ageing, has been investigated by Aline Marguet via the mathematical analysis of those processes. In collaboration with Charline Smadi (INRAE Grenoble), Aline Marguet investigated the long-time behavior of continuous-state nonlinear branching processes with catastrophes. This work, which has been submitted for publication 25, is motivated by the study of parasite infection in a cell line. The continuous-state branching processes models the dynamic of the parasites inside a cell and the catastrophes model cellular divisions that dilute the number of parasites in the cell. We exhibit conditions on the dynamics of the parasites to explode or get extinct in a cell line. Combining some results of 25 and the Many-to-one formula proved in 10 that characterizes the dynamic of a typical individual, we investigated the long time behaviour of an infected cell population. In this companion work, submitted for publication 26, we model the dynamic of the cell population using a structured branching process where the cell cycles depends on the dynamics of the parasites contained in the cell. We investigate the role of different sharing strategies at division on the fate of the cell population and give conditions for recovery or proliferation of the infection.
The study of the asymptotic behavior of general non-conservative semigroups is important for several aspects of branching processes, especially to prove the efficiency of statistical procedures. Vincent Bansaye from École Polytechnique, Bertrand Cloez from INRAE Montpellier, Pierre Gabriel from Université Versailles Saint-Quentin and Aline Marguet obtained necessary and sufficient conditions for uniform exponential contraction in weighted total variation norm of non-conservative semigroups. It ensures the existence of Perron eigenelements and provides quantitative estimates of spectral gaps, complementing Krein-Rutman theorems and generalizing recent results relying on probabilistic approaches. This work has been submitted for publication 24.
7.3 Modeling and inference of RNA degradation
The ability to rapidly respond to changing nutrient availability is crucial for E. coli to survive in many environments including the gut. Reorganization of gene expression is the first step for bacteria to adjust their metabolism accordingly. It involves fine-tuning of both transcription and mRNA stability by dedicated regulatory interactions. While transcriptional regulation has been largely studied, the role of mRNA stability during a metabolic switch is poorly understood.
This question was addressed in the framework of the PhD thesis of Manon Morin funded by an INRA-Inria grant. Using combined genome-wide transcriptome and mRNA decay analyses, Manon Morin, Delphine Ropers and colleagues from the Toulouse Biotechnology Institute (INRA/INSA Toulouse) investigated the role of mRNA stability in the response of E. coli to nutrient changes. They demonstrated that while transcription of most genes is downregulated when glucose is exhausted, concomitant mRNA stabilization of many mRNAs occurs and leads to the upregulation of genes involved in responses to nutrient changes and stresses. This work was published in mSphere this year 21.
The observation of a global stabilization of cellular mRNAs during adaptation to carbon source depletion raises questions about the regulatory mechanisms at work. Known regulators of mRNA stability such as the protein Hfq, the carbon storage regulator Csr, and several small regulatory RNAs, specifically target mRNAs. Are these regulatory mechanisms sufficient to explain the systematic adjustment of mRNA half-lives? The collaboration with Muriel Cocaign-Bousquet and colleagues from the Toulouse Biotechnology Institute has been pursued to answer these questions, in the context of the PhD thesis of Thibault Etienne, funded by an INRA-Inria PhD grant. The objective was to develop models able to explain how cells coordinate their physiology and the functioning of the degradation machinery following environmental changes. In a paper published in Journal of Theoretical Biology17, Thibault Etienne, Muriel Cocaign-Bousquet, and Delphine Ropers investigated the possibility that competition between mRNAs for their binding to the degradation machinery is an important mechanism for the regulation of mRNA half-lives. They develop a mathematical model of mRNA degradation and assess the role of competitive effects on mRNA degradation kinetics by numerical simulation and sensitivity analysis. Competition appears to globally increase the stability of cellular mRNAs and to amplify the effect of post-transcriptional regulation. In a follow-up study, the model is currently being used to interpret large data sets corresponding to the degradation kinetics of 4254 mRNAs in E. coli cells growing in four different environmental conditions.
The above two studies 17, 21 have been highlighted by INRAE (https://
7.4 Modeling synthetic microbial communities for improving productivity
Modelling, analysis and control of microbial community dynamics is a fast-developing subject with great potential implications in the understanding of natural processes and the enhancement of biotechnological processes. Within the now-ending project IPL COSY (Section 8.2), we picked up the challenge to design and investigate the dynamics of synthetically engineered microbial communities with a consortium of Inria partners.
In IBIS, in particular, we have addressed the design of a bacterial community of two E. coli strains, mimicking mutualistic relationships found in nature, and with the potential to outperform a single producer strain in the production of a heterologous protein. We developed an ODE model of the key growth phenotypes of the community and their interactions, calibrated the model on literature data, and analysed the model for an in-depth understanding of the conditions supporting coexistence and of the tradeoffs encountered in this production process. The results were published in PLoS Computational Biology20.
Experimental validation of these findings are part of the PhD project of Maaike Sangster, currently in the second year. The construction of the microbial consortium is underway, and first experimental results on growth dynamics of the different species at population and single-cell (population snapshot) level are being produced. Biological extensions toward real-time control of the microbial community for optimal protein biosynthesis are also being addressed by the synthesis of optogenetic circuits on bacterial DNA. In parallel, investigation of real-time estimation and control problems for this microbial consortium is underway with IPL partners. Mathematical analysis of alternative microbial community designs is yet another direction being pursued with BIOCORE, with one publication in preparation for a mathematical biology journal.
As a follow-up of IPL Cosy, a new ANR project on algal-bacterial communities, named Ctrl-AB (Section 8.1), has been accepted and is now starting. The project aims at leveraging natural synergies in the simultaneous growth of the two species, and at further enhancing the interactions with a synthetic consortium via optogenetic control, to optimize lypid productivity in view of biotechnology applications. The project is also the occasion to explore advanced control methodologies in this challenging context, with possible contributions to the control field itself.
7.5 Resource allocation models of bacterial growth
Various mathematical approaches have been used in the literature to describe the networks of biochemical reactions involved in microbial growth. With different levels of detail, the resulting models provide an integrated view of these reaction networks, including the transport of nutrients from the environment and the metabolism and gene expression allowing the conversion of these nutrients into biomass. Recent work has shown that coarse-grained models of resource allocation can account for a number of empirical regularities relating the the macromolecular composition of the cell to the growth rate. Some of these models hypothesize control strategies enabling microorganisms to optimize growth. While these studies focus on steady-state growth, such conditions are rarely found in natural habitats, where microorganisms are continually challenged by environmental fluctuations.
In recent years, in the framework of the PhD thesis of Nils Giordano, we extended the study of microbial growth strategies to dynamical environments, using a self-replicator model. In collaboration with the BIOCORE project-team, we formulated dynamical growth maximization as an optimal control problem that can be solved using Pontryagin’s Maximum Principle and we compared the theoretical results thus obtained with different possible implementations of growth control in bacterial cells 6. The experimental validation of some of these results are currently being carried out by Antrea Pavlou in the framework of her PhD project, funded by the ANR project Maximic (Section 8.2).
Also within the context of Maximic, we have worked on several extensions of the original self-replicator model. In collaboration with the BIOCORE and McTAO project-teams, we have developed a model that accounts for additional classes of proteins, in particular a class of growth-rate-independent housekeeping proteins in addition to ribosomes and enzymes. Moreover, the model takes into account that the protein synthesis rate does not linearly depend on the total ribosome concentration, but only on its growth-rate-dependent fraction. In a journal paper prepared for publication, we have performed an optimal control analysis of the extended model, using a combination of analytical and numerical tools. Our major conclusion is that, while the predicted dynamics of the system does not significantly change in comparison with the original model, the extended model more closely adheres to the experimental data. Moreover, it is capable of predicting the effects of metabolic load due to heterologous protein expression on the growth-rate dynamics.
In another follow-up work, carried out in collaboration with Francis Mairet (Ifremer Nantes) and Jean-Luc Gouzé (BIOCORE), we study the effect of temperature on microbial growth, in particular the role of proteome allocation in bringing about temperature-induced changes. To tackle this problem, we propose an extended coarse-grained model of microbial growth that includes the processes of temperature-sensitive protein unfolding and chaperone-assisted (re)folding. We determine the proteome sector allocation that maximizes balanced growth rate as a function of nutrient limitation and temperature. Calibrated with quantitative proteomic data for Escherichia coli, the model allows us to bring out general principles of temperature-dependent proteome allocation and growth laws. The same activation energy for enzymes and ribosomes leads to an Arrhenius increase in growth rate at constant proteome composition over a large range of temperatures, whereas at extreme temperatures resources are diverted away from growth to chaperone-mediated stress responses. Our approach points at risks and possible remedies for the use of ribosome content to characterize complex ecosystems with temperature gradients. A paper based on this work will be published in the journal npj Systems Biology and Applications19.
7.6 Qualitative modeling of synthetic gene circuits
Qualitative modeling approaches are promising and still underexploited tools for the analysis and design of synthetic gene circuits. They can make predictions of circuit behavior in the absence of precise, quantitative information. Moreover, they provide direct insight into the relation between the feedback structure and the dynamical properties of a network. In a book chapter for an edited volume 23, co-written with Madalena Chaves (BIOCORE), we review qualitative modeling approaches by focusing on two specific formalisms, Boolean networks and piecewise-linear differential equations, and illustrate their application by means of three well-known synthetic circuits. We describe various methods for the analysis of state transition graphs, the discrete representations of the network dynamics that are generated in both modeling frameworks. We also briefly present the problem of controlling synthetic circuits, an emerging topic that could profit from the capacity of qualitative modeling approaches to rapidly scan a space of design alternatives.
8 Partnerships and cooperations
8.1 Regional Initiatives
Project name | MOSTIC: Stochastic modelling and inference for cell communities in interaction |
Coordinator | A. Marguet |
IBIS participants | E. Cinquemani, J. Geiselmann, A. Marguet, E. Reginato |
Type | IXXI/BioSyl project (2020-2022) |
Web page |
http:// |
Project name | MuSE: MUlti-Omics and Metabolic models integration to study growth transition in Escherichia coli |
Coordinator | D. Ropers |
IBIS participants | D. Ropers, T. Etienne |
Type | IXXI/BioSyl project (2018-2020) |
Web page |
http:// |
8.2 National Initiatives
Project name | Ctrl-AB : Optimization and control of the productivity of an algal-bacterial consortium |
Coordinator | J.-L. Gouzé |
IBIS participants | E. Cinquemani, H. de Jong, J. Geiselmann, A. Marguet, T. Muszbek, C. Pinel, M. Sangster |
Type | ANR project (2020-2024) |
Project name | OPTICO : OPTImal COntrol software for microbial communities in a system of minibioreactors |
Coordinator | E. Cinquemani |
IBIS participants | E. Cinquemani, H. de Jong, J. Geiselmann, T. Muszbek |
Type | Inria ADT (2019-2021) |
Project name | RIBECO (RIBonucleotide ECOnomy): Engineering RNA life cycle to optimize economy of microbial energy |
Coordinator | M. Cocaign-Bousquet |
IBIS participants | E. Cinquemani, T. Etienne, D. Ropers |
Type | ANR project (2018-2022) |
Web page |
https:// |
Project name | MAXIMIC: Optimal control of microbial cells by natural and synthetic strategies |
Coordinator | H. de Jong |
IBIS participants | E. Cinquemani, T. Clavier, J. Geiselmann, H. de Jong, A. Pavlou, C. Pinel, D. Ropers |
Type | ANR project (2017-2021) |
Web page |
https:// |
Project name | COSY: real-time COntrol of SYnthetic microbial communities |
Coordinator | E. Cinquemani |
IBIS participants | E. Cinquemani, H. de Jong, J. Geiselmann, A. Marguet, T. Muszbek, C. Pinel, E. Reginato, D. Ropers, M. Sangster |
Type | Inria Project Lab (2017-2021) |
Web page |
https:// |
Project name | MEMIP – Modèles à effets mixtes de processus intracellulaires : méthodes, outils et applications |
Coordinator | G. Batt |
IBIS participants | E. Cinquemani, A. Marguet, E. Reginato, D. Ropers |
Type | ANR project (2016-2020) |
Project name | ENZINVIVO – Détermination in vivo des paramètres enzymatiques dans une voie métabolique synthétique |
Coordinator | G. Truan |
IBIS participants | J. Geiselmann, H. de Jong |
Type | ANR project (2016-2020) |
8.3 Other national and international collaborations
In this section, we list a number of active national and international research collaborations that are not covered by the above-mentioned projects in which IBIS is an official partner.
- A. Marguet contributes to the ANR JCJC NOLO of Bertrand Cloet (INRAE Montpellier) on non-local branching processes.
- H. de Jong and D. Ropers collaborate with T. Gedeon (Montana State University), former invited researcher in IBIS, on research allocation strategies in microorganisms
- J. Geiselmann and H. de Jong work with P. Hersen (Unité Physico-chimique, Institut Curie) on growth control in microorganisms
- H. de Jong, J. Geiselmann, and D. Ropers collaborate with Y. Couté and M. Ferro (CEA Grenoble) on the proteomic quantification of microbial physiology
9 Dissemination
9.1 Promoting scientific activities
9.1.1 Scientific events: organisation
Member of organizing committees
IBIS members | Conference, workshop, school | Date |
Eugenio Cinquemani | Modelling Heterogeneous Populations with applications in Biology, Grenoble | May 2021 |
Eugenio Cinquemani | 19th International Conference on Computational Methods in Systems Biology (CMSB 2021), Bordeaux | Sep 2021 |
Hidde de Jong | CompSysBio: Advanced Lecture Course on Computational Systems Biology, Aussois | Nov 2021 |
Aline Marguet | Biohasard: Stochastic models for biology, Grenoble | May 2020 |
Delphine Ropers | Séminaire de Modélisation du Vivant (SeMoVi), Lyon and Grenoble | 2020 |
9.1.2 Scientific events: selection
Chair of conference program committees
IBIS member | Conference, workshop, school | Role |
Eugenio Cinquemani | European Control Conference (ECC 2020) | Associate editor |
Eugenio Cinquemani | 19th International Conference on Computational Methods in Systems Biology (CMSB 20201) | Program co-chair |
Member of Conference Program Committees
IBIS member | Conference, workshop, program |
Eugenio Cinquemani | ECC 2020 and 2021, CMSB 2020, HSB 2020, IEEE CIBCB 2019 |
Hidde de Jong | CMSB 2020, HSB 2020, IFAC DYCOPS-CAB 2022 |
Delphine Ropers | CSBio 2020, ECCB 2020 |
9.1.3 Journal
Member of Editorial Boards
IBIS member | Journal |
Johannes Geiselmann | Frontiers in Microbiology (reviews editor) |
Hidde de Jong | Journal of Mathematical Biology |
Hidde de Jong | Biosystems (reviews editor) |
9.1.4 Invited Talks and other Presentations
Eugenio Cinquemani
Title | Event and location | Date |
Enhanced production of heterologous proteins by a synthetic microbial consortium: Conditions and trade-offs | Invited talk at workshop Reaction, Diffusion and Molecular Communication (online) | May 2020 |
Inheritance and variability of kinetic gene expression parameters in microbial cells | Invited talk at Séminaire de Statistique, MAP5 Laboratory, Université de Paris | Feb 2020 |
Inference of the statistics of a modulated promoter process from population snapshot gene expression data | Presentation IFAC World Congress (online) | Jul 2020 |
Hidde de Jong
Title | Event and location | Date |
Modélisation de la croissance de microorganismes | Conférences ISN 2020, Grenoble | Mar 2020 |
Aline Marguet
Title | Event and location | Date |
Long time behaviour for a general class of Markov branching processes | Séminaire de probabilités et statistique, Lille | Jan 2020 |
Mathématiques appliquées à la biologie | Conférences ISN 2020, Grenoble | Feb 2020 |
Inheritance and variability of kinetic gene expression parameters in microbial cell | Séminaire du LMAC, Compiègne | Feb 2020 |
Inheritance and variability of kinetic gene expression parameters in microbial cell | Séminaire de Statistique d'AgroParisTech, Paris (videoconference) | May 2020 |
On the fate of an infected cell line | Bernoulli-IMS One World Symposium 2020 (videoconference) | Aug 2020 |
Delphine Ropers
Title | Event and location | Date |
Advanced application in precision medicine: Integrative analysis of big data with genome-scale metabolic models | Summer school Learning from health data (online) | Nov 2020 |
9.1.5 Scientific Evaluation and Expertise
IBIS member | Organism | Role |
Johannes Geiselmann | INRA | Member scientific advisory committee Microbiologie, Adaptation, Pathogénie |
Johannes Geiselmann | UMR5240 CNRS-UCBL-INSA-BayerCropScience | Member scientific council |
Johannes Geiselmann | ANR | Member of evaluation committee CES30 |
Hidde de Jong | Microbiology and Food Chain Department, Inra | Member scientific council |
Hidde de Jong | Univ Grenoble Alpes | Member scientific council of Pôle MSTIC |
Delphine Ropers | Elixir | Member of Microbial Biotechnology Community |
9.1.6 Research administration
IBIS member | Committee | Role |
Eugenio Cinquemani | Inria Grenoble - Rhône-Alpes | Member Comité des Emplois Scientifiques (CES) |
Eugenio Cinquemani | Inria Grenoble - Rhône-Alpes | Member Comité des Utilisateurs des Moyens Informatiques (CUMI) |
Eugenio Cinquemani | Inria | Member Comité Administrative Paritaire (CAP) |
Eugenio Cinquemani | Inria Grenoble - Rhône-Alpes | Member Comité Développement Technologique (CDT) |
Hidde de Jong | Inria Grenoble - Rhône-Alpes | Member scientific council (COS) |
Aline Marguet | Inria Grenoble - Rhône-Alpes | Correspondent Sciences Environnements Sociétés |
Delphine Ropers | Inria Grenoble - Rhône-Alpes | Référente chercheurs |
Delphine Ropers | Inria Grenoble - Rhône-Alpes | Co-coordinator of mentoring program |
Maaike Sangster | Laboratoire Interdisciplinaire de Physique (LIPhy) | Member scientific council |
9.2 Teaching - Supervision - Juries
9.2.1 Teaching
Four members of the IBIS team are either full professor or associate professor at Univ Grenoble Alpes. They therefore have a full teaching service (at least 192 hours per year) and administrative duties related to the organization and evaluation of the university course programs on all levels (from BSc to PhD). Besides the full-time academic staff in IBIS, the following people have contributed to courses last year.
Eugenio Cinquemani
- Course: Statistics for systems biology, M1, Master Approches Interdisciplinaires du Vivant, CRI/Univ Paris Descartes (20 h, also in charge of 20 h of practicals)
- Course: Modelling and identification of metabolic networks, M1, Phelma, INP Grenoble (4 h)
Hidde de Jong
- Course and practicals: Modeling and simulation of gene regulatory networks, M2, BIM, INSA de Lyon (34 h)
Aline Marguet
- Practicals: Biostatistics, M2, Univ Grenoble Alpes (27 h)
Antrea Pavlou
- Course: Statistics, L2, Mathematics, Univ Grenoble Alpes (24 h)
- Course: Statistics for biology, L2, Mathematics, Univ Grenoble Alpes (56 h)
Delphine Ropers
- Course and practicals: Modelling in systems biology, M1, Phelma, INP Grenoble (16 h)
- Course and practicals: Cell systems biology and modelling cell functions, M1, Master ingéniérie de la santé, Univ Grenoble Alpes (14 h)
- Course: Modeling and simulation of genetic regulatory networks, M2, INSA de Toulouse (4 h)
- Course: Metabolic modelling with Omics data, M2, IA4 Health International master course, Univ Grenoble Alpes (6 h)
9.2.2 Supervision
- PhD completed: Thibault Etienne, Analyse intégrative de la coordination entre stabilité des ARNm et physiologie cellulaire chez Escherichia coli. Supervisors: Delphine Ropers and Muriel Cocaign-Bousquet (INRA Toulouse). Defense: Dec 2020
- PhD completed: Joël Espel, RNA engineering: Design of the dynamical folding of RNA and of RNA switches. Supervisors: Alexandre Dawid (Univ Grenoble Alpes) and Johannes Geiselmann. Defense: Oct 2020
- PhD in progress: Antrea Pavlou, Experimental and computational analysis of bacterial self-replicators. Supervisors: Hidde de Jong and Johannes Geiselmann
- PhD in progress: Maaike Sangster, Development, characterization and control of E. coli communities on an automated experimental platform. Supervisors: Eugenio Cinquemani and Johannes Geiselmann
- PhD in progress: Emrys Reginato, Development, analysis, and inference of stochastic models of gene expression in growing cell populations. Supervisors: Eugenio Cinquemani and Aline Marguet
- PhD in progress: Thibault Clavier, Genetic growth control to maximize the bioproduction in E. coli. Supervisors: Johannes Geiselmann and Hidde de Jong
9.2.3 Juries
PhD thesis committees
IBIS member | Role | PhD student | University | Date |
Hidde de Jong | Examinateur | Daniel Figueiredo | Univ of Aveiro (Portugal) | Feb 2020 |
Hidde de Jong | Rapporteur | Lin Liu | Technical University Berlin | Apr 2020 |
Hidde de Jong | Rapporteur/ Président | Shiny Martis | INSA de Lyon | Nov 2020 |
Delphine Ropers | Examinatrice | Joël-Gabriel Espel | Univ Grenoble Alpes | Oct 2020 |
Delphine Ropers | Directrice | Thibault Etienne | Univ de Lyon | Dec 2020 |
Habilitation (HDR) committees
IBIS member | Role | HDR candidate | University | Date |
Hidde de Jong | Rapporteur | Laurent Guyon | Univ Grenoble Alpes | Dec 2020 |
PhD advisory committees
IBIS member | PhD student | University |
Eugenio Cinquemani | Arthur Carcano | Institut Pasteur/Inria |
Eugenio Cinquemani | Alexey Koshkin | ENS de Lyon |
Johannes Geiselmann | Alain Lombard | Univ Grenoble Alpes |
Johannes Geiselmann | Ihab Boulas | Collège de France |
Johannes Geiselmann | Clément Caffaratti | Univ Grenoble Alpes |
Hidde de Jong | Charlotte Coton | INRA Moulon |
Hidde de Jong | Kapil Newar | Univ Grenoble Alpes |
Hidde de Jong | Agustín Yabo | Univ Nice–Sophia-Antipolis |
Stéphan Lacour | Julien Trouillon | CEA Grenoble |
Stéphan Lacour | Camille Brunet | CHU Grenoble |
Stéphan Lacour | Raphael Fourquet | INSA de Lyon/Univ de Lyon |
Stéphan Lacour | Kevin Pick | Univ de Lyon |
Delphine Ropers | Manon Barthe | INSA de Toulouse |
Delphine Ropers | Charlotte Roux | Univ Paul Sabatier, Toulouse |
9.2.4 Teaching administration
Yves Markowicz is director of the BSc department at Univ Grenoble Alpes.
Michel Page is coordinator of the master Systèmes d'information et d'organisation at the Institut d'Adminstration des Entreprises (IAE), Univ Grenoble Alpes.
Eugenio Cinquemani organizes a module on statistics in systems biology at CRI/Univ Paris Descartes.
Delphine Ropers organizes a module on the mathematical modeling of biological systems at PHELMA, INP Grenoble.
Hidde de Jong organizes a module on the modeling of genetic and metabolic networks at INSA de Lyon.
9.3 Popularization
9.3.1 Interventions
Aline Marguet et Hidde de Jong participated in the program Informatique et Sciences du Numériques (ISN) 2020, a seminar series addressing highschool students and teachers. For more information, see https://
10 Scientific production
10.1 Major publications
- 1 articleThe carbon assimilation network in Escherichia coli is densely connected and largely sign-determined by directions of metabolic fluxes.PLoS Computational Biology66June 2010, e1000812
- 2 articleIdentification of metabolic network models from incomplete high-throughput datasetsBioinformatics27132011, i186-i195
- 3 articleShared control of gene expression in bacteria by transcription factors and global physiology of the cellMolecular Systems Biology91January 2013, 11URL: http://hal.inria.fr/hal-00793352
- 4 articleEstimation of time-varying growth, uptake and excretion rates from dynamic metabolomics dataBioinformatics33142017, i301--i310
- 5 articleStochastic reaction networks with input processes: Analysis and application to gene expression inferenceAutomatica1012019, 150-156
- 6 articleDynamical allocation of cellular resources as an optimal control problem: Novel insights into microbial growth strategiesPLoS Computational Biology123March 2016, e1004802
- 7 articleA synthetic growth switch based on controlled expression of RNA polymeraseMolecular Systems Biology1111November 2015, 16
- 8 articleWhat population reveals about individual cell identity: Single-cell parameter estimation of models of gene expression in yeastPLoS Computational Biology122February 2016, e1004706
- 9 articleInheritance and variability of kinetic gene expression parameters in microbial cells: modeling and inference from lineage tree dataBioinformatics35142019, i586-i595
- 10 articleUniform sampling in a structured branching populationBernoulli254A2019, 2649-2695
- 11 articleEnhanced production of heterologous proteins by a synthetic microbial community: Conditions and trade-offsPLoS Computational Biology1642020, e1007795
- 12 articleThe Csr system regulates Escherichia coli fitness by controlling glycogen accumulation and energy levelsmBio852017, 1-14
- 13 articleThe post-transcriptional regulatory system CSR controls the balance of metabolic pools in upper glycolysis of Escherichia coliMolecular Microbiology4January 2016, 15
- 14 articleCharacterization of the Escherichia coli σ(S) core regulon by Chromatin Immunoprecipitation-sequencing (ChIP-seq) analysisScientific Reports52015, 15
- 15 article Inference of quantitative models of bacterial promoters from time-series reporter gene data PLoS Computational Biology 11 1 e1004028 2015
- 16 articleRobust reconstruction of gene expression profiles from reporter gene data using linear inversionBioinformatics31122015, i71--i79
10.2 Publications of the year
International journals
International peer-reviewed conferences
Scientific book chapters
Reports & preprints