Keywords
Computer Science and Digital Science
- A3.3. Data and knowledge analysis
- A3.4. Machine learning and statistics
- A4.4. Security of equipment and software
- A4.8. Privacy-enhancing technologies
- A5.2. Data visualization
- A5.3. Image processing and analysis
- A5.4. Computer vision
- A5.6. Virtual reality, augmented reality
- A5.9. Signal processing
- A6.1. Methods in mathematical modeling
- A6.2. Scientific computing, Numerical Analysis & Optimization
- A6.3. Computation-data interaction
- A8.3. Geometry, Topology
- A9. Artificial intelligence
- A9.2. Machine learning
- A9.3. Signal analysis
- A9.6. Decision support
- A9.7. AI algorithmics
- A9.9. Distributed AI, Multi-agent
Other Research Topics and Application Domains
- B2.2. Physiology and diseases
- B2.3. Epidemiology
- B2.4. Therapies
- B2.6. Biological and medical imaging
- B2.6.1. Brain imaging
- B2.6.2. Cardiac imaging
- B2.6.3. Biological Imaging
1 Team members, visitors, external collaborators
Research Scientists
- Nicholas Ayache [Team leader, Inria, Senior Researcher, HDR]
- Irene Balelli [Inria, ISFP, from Oct 2021]
- James Benn [Inria, Starting Research Position]
- Hervé Delingette [Inria, Senior Researcher, HDR]
- Marco Lorenzi [Inria, Researcher, HDR]
- Marta Nunez Garcia [Inria, Starting Research Position, from Nov 2021]
- Xavier Pennec [Inria, Senior Researcher, HDR]
- Maxime Sermesant [Inria, Senior Researcher, HDR]
Post-Doctoral Fellows
- Irene Balelli [Inria, until Sep 2021]
- Francisco Burgos [Université polytechnique de Catalogne - Espagne, from Feb 2021]
- Anna Calissano [Inria, from May 2021]
- Marie Deprez [Inria]
- Marta Nunez Garcia [Univ de Bordeaux, until Oct 2021]
- Dimbihery Rabenoro [Inria]
- Jesus Jairo Rodriguez Padilla [Inria, from Jul 2021]
PhD Students
- Clement Abi Nader [Université Côte d'Azur, until Jun 2021]
- Luigi Antelmi [Univ Côte d'Azur, until Jul 2021]
- Benoit Audelan [Univ Côte d'Azur, until Jul 2021]
- Tania Marina Bacoyannis [Inria]
- Jaume Banus Cobo [Inria, until Sep 2021]
- Paul Blanc-Durand [Université Paris Descartes]
- Nicolas Cedilnik [Inria, until Feb 2021]
- Mikael Chelli [Centre hospitalier universitaire de Nice]
- Hind Dadoun [Inria]
- Gaetan Desrues [Inria]
- Zhijie Fang [Inria, from Oct 2021]
- Yann Fraboni [Inria, Accenture Labs Sophia Antipolis, CIFRE]
- Nicolas Guigui [Inria]
- Dimitri Hamzaoui [Univ Côte d'Azur]
- Josquin Harrison [Inria]
- Etrit Haxholli [Inria]
- Florent Jousse [QuantificCare, CIFRE]
- Victoriya Kashtanova [Inria]
- Huiyu Li [Inria, from Sep 2021]
- Buntheng Ly [Univ Côte d'Azur]
- Elodie Maignant [Inria]
- Morten Pedersen [Inria]
- Santiago Smith Silva Rincon [Univ Côte d'Azur]
- Hari Sreedhar [Univ Côte d'Azur]
- Riccardo Taiello [Inria, from Oct 2021]
- Yann Thanwerdas [Univ Côte d'Azur]
- Paul Tourniaire [Inria]
- Clair Vandersteen [Centre hospitalier universitaire de Nice]
- Zihao Wang [Inria]
- Yingyu Yang [Inria]
Interns and Apprentices
- Hava Chaptoukaev [Inria, from Apr 2021 until Aug 2021]
- Pierre Lindet [Inria, from May 2021 until Jul 2021]
- Hippolyte Mayard [Inria, from May 2021 until Sep 2021]
- Andrea Senacheribbe [Inria, until Feb 2021]
Administrative Assistant
- Isabelle Strobant [Inria]
Visiting Scientists
- Marc Olivier Gauci [Centre hospitalier universitaire de Nice, from Jun 2021 until Aug 2021]
- Pamela Moceri [Université Côte d'Azur]
- Mihaela Pop [Inria, from Sep 2021]
External Collaborators
- Guillaume Lajoinie [University of Twente, until Jun 2021]
- Marco Milanesio [Université Côte d'Azur]
2 Overall objectives
2.1 Description
Our long-term goal is to contribute to the development of what we call the e-patient (digital patient) for e-medicine (digital medicine).
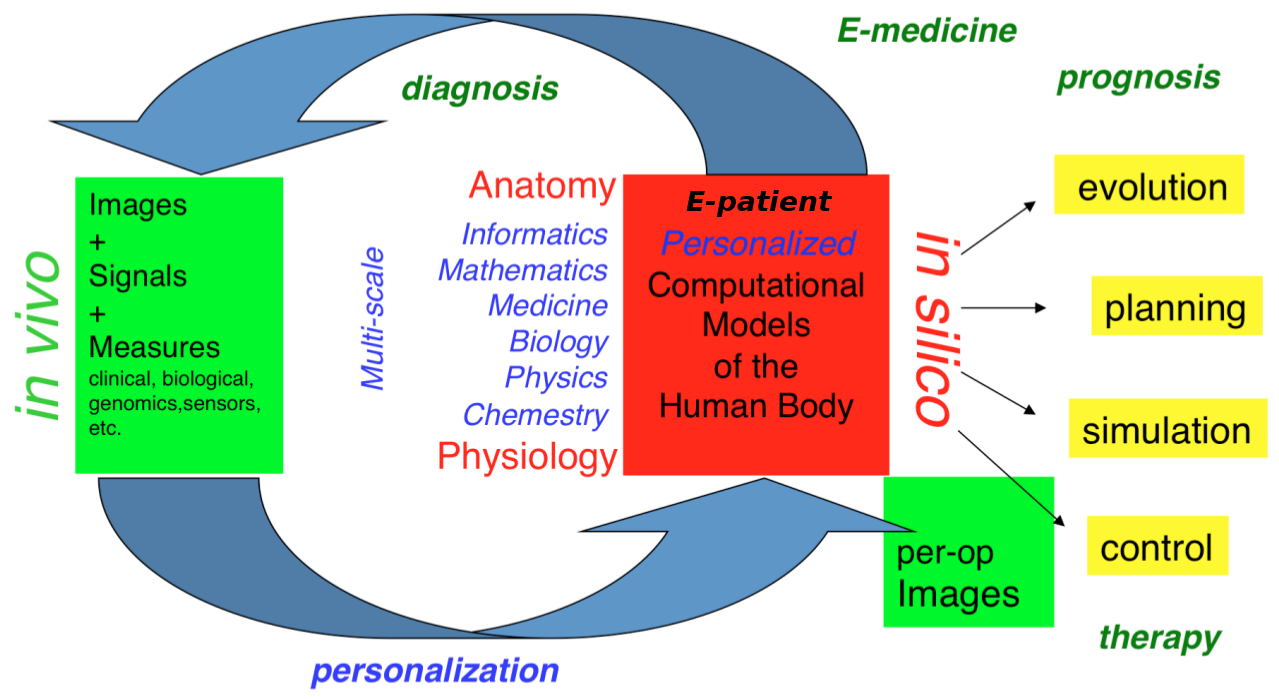
- the e-patient (or digital patient) is a set of computational models of the human body able to describe and simulate the anatomy and the physiology of the patient's organs and tissues, at various scales, for an individual or a population. The e-patient can be seen as a framework to integrate and analyze in a coherent manner the heterogeneous information measured on the patient from disparate sources: imaging, biological, clinical, sensors, ...
- e-medicine (or digital medicine) is defined as the computational tools applied to the e-patient to assist the physician and the surgeon in their medical practice, to assess the diagnosis/prognosis, and to plan, control and evaluate the therapy.
The models that govern the algorithms designed for e-patients and e-medicine come from various disciplines: computer science, mathematics, medicine, statistics, physics, biology, chemistry, etc. The parameters of those models must be adjusted to an individual or a population based on the available images, signals and data. This adjustment is called personalization and usually requires solving difficult inverse problems. The overall picture of the construction of the personalized e-patient for e-medicine was presented at the College de France through an inaugural lecture and a series of courses and seminars (fr), concluded by an international workshop.
2.2 Organisation
The research organization in our field is often built on a virtuous triangle. On one vertex, academic research requires multidisciplinary collaborations associating informatics and mathematics to other disciplines: medicine, biology, physics, chemistry ... On a second vertex, a clinical partnership is required to help defining pertinent questions, to get access to clinical data, and to clinically evaluate any proposed solution. On the third vertex, an industrial partnership can be introduced for the research activity itself, and also to transform any proposed solution into a validated product that can ultimately be transferred to the clinical sites for an effective use on the patients.
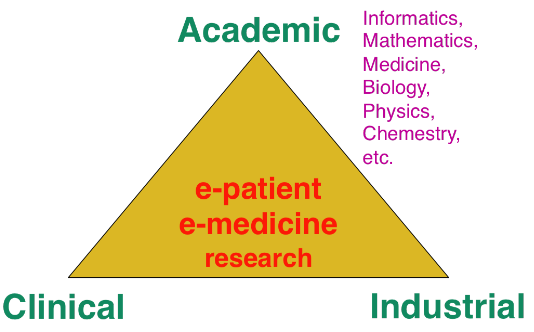
Keeping this triangle in mind, we choose our research directions within a virtuous circle: we look at difficult problems raised by our clinical or industrial partners, and then try to identify some classes of generic fundamental/theoretical problems associated to their resolution. We also study some fundamental/theoretical problems per se in order to produce fundamental scientific advances that can help in turn to promote new applications.
3 Research program
3.1 Introduction
Our research objectives are organized along 5 scientific axes:
- Biomedical Image Analysis & Machine Learning
- Imaging & Phenomics, Biostatistics
- Computational Anatomy, Geometric Statistics
- Computational Physiology & Image-Guided Therapy
- Computational Cardiology & Image-Based Cardiac Interventions
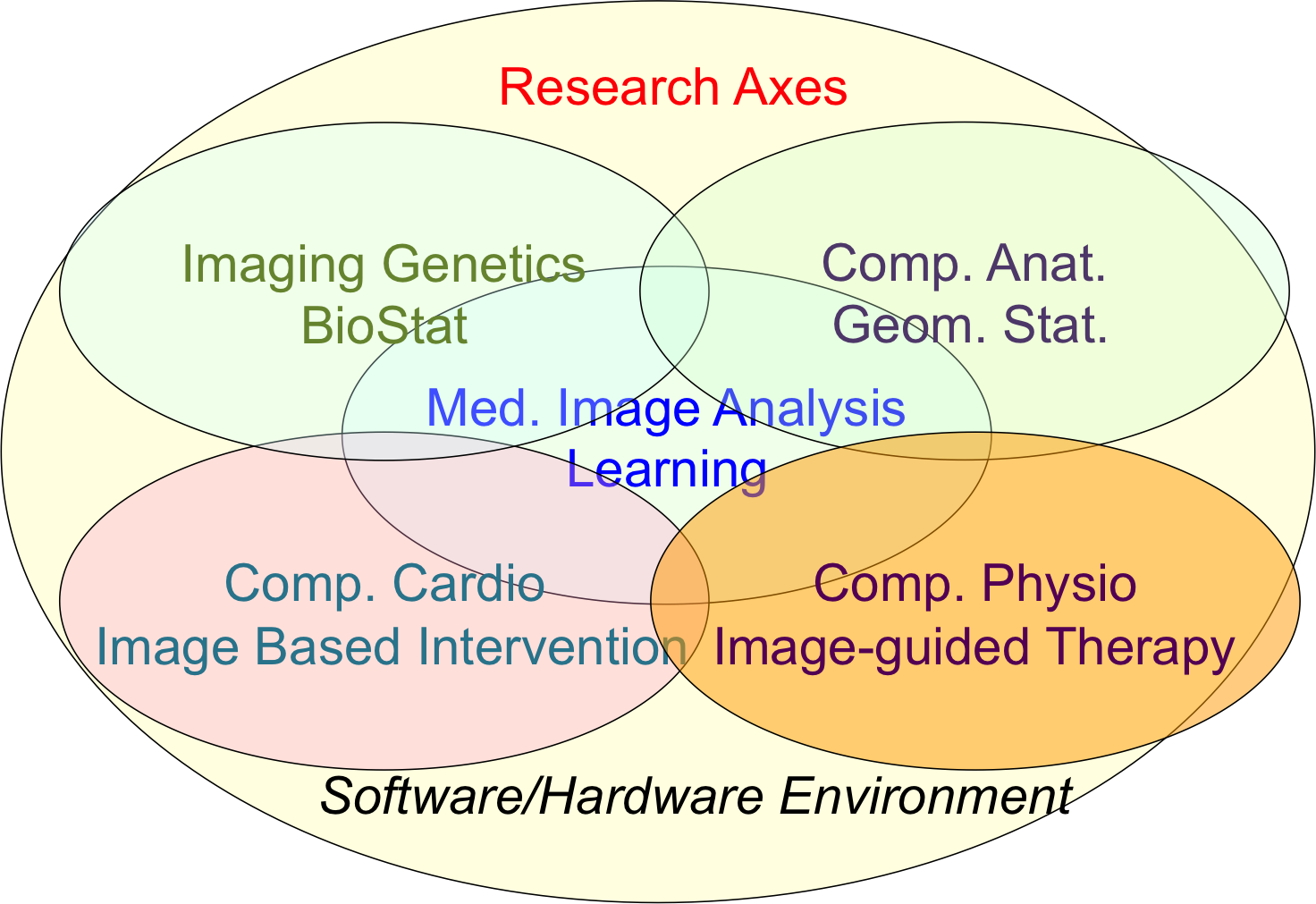
For each scientific axis, we introduce the context and the long term vision of our research.
3.2 Biomedical Image Analysis & Machine Learning
The long-term objective of biomedical image analysis is to extract, from biomedical images, pertinent information for the construction of the e-patient and for the development of e-medicine. This relates to the development of advanced segmentation and registration of images, the extraction of image biomarkers of pathologies, the detection and classification of image abnormalities, the construction of temporal models of motion or evolution from time-series of images, etc.
In addition, the growing availability of very large databases of biomedical images, the growing power of computers and the progress of machine learning (ML) approaches have opened up new opportunities for biomedical image analysis.
This is the reason why we decided to revisit a number of biomedical image analysis problems with ML approaches, including segmentation and registration problems, automatic detection of abnormalities, prediction of a missing imaging modality, etc. Not only those ML approaches often outperform the previous state-of-the-art solutions in terms of performances (accuracy of the results, computing times), but they also tend to offer a higher flexibility like the possibility to be transferred from one problem to another one with a similar framework. However, even when successful, ML approaches tend to suffer from a lack of explanatory power, which is particularly annoying for medical applications. We also plan to work on methods that can interpret the results of the ML algorithms that we develop.
3.3 Imaging & Phenomics, Biostatistics
The human phenotype is associated with a multitude of heterogeneous biomarkers quantified by imaging, clinical and biological measurements, reflecting the biological and patho-physiological processes governing the human body, and essentially linked to the underlying individual genotype. In order to deepen our understanding of these complex relationships and better identify pathological traits in individuals and clinical groups, a long-term objective of e-medicine is therefore to develop the tools for the joint analysis of this heterogeneous information, termed Phenomics, within the unified modeling setting of the e-patient.
To date the most common approach to the analysis of the joint variation between the structure and function of organs represented in medical images, and the classical -omics modalities from biology, such as genomics or lipidomics, is essentially based on the massive univariate statistical testing of single candidate features out of the many available. This is for example the case of genome-wide association studies (GWAS) aimed at identifying statistically significant effects in pools consisting of up to millions of genetics variants. Such approaches have known limitations such as multiple comparison problems, leading to underpowered discoveries of significant associations, and usually explain a rather limited amount of data variance . Although more sophisticated machine learning approaches have been proposed, the reliability and generalization of multivariate methods is currently hampered by the low sample size relatively to the usually large dimension of the parameters space.
To address these issues this research axis investigates novel methods for the integration of this heterogeneous information within a parsimonious and unified multivariate modeling framework. The cornerstone of the project consists in achieving an optimal trade-off between modeling flexibility and ability to generalize on unseen data by developing statistical learning methods informed by prior information, either inspired by "mechanistic" biological processes, or accounting for specific signal properties (such as the structured information from spatio-temporal image time series). Finally, particular attention will be paid to the effective exploitation of the methods in the growing Big Data scenario, either in the meta-analysis context, or for the application in large datasets and biobanks.
Federated learning in multi-centric studies. The current research scenario is characterised by medium/small scale (typically from 50 to 1000 patients) heterogeneous datasets distributed across centres and countries. The straightforward extension of learning algorithms successfully applied to big data problems is therefore difficult, and specific strategies need to be envisioned in order to optimally exploit the available information. To address this problem, we focus on learning approaches to jointly model clinical data localized in different centres. This is an important issue emerging from recent large-scale multi-centric imaging-genetics studies in which partners can only share model parameters (e.g. regression coefficients between specific genes and imaging features), as represented for example by the ENIGMA imaging-genetics study, led by the collaborators at University of Southern California. This problem requires the development of statistical methods for federated model estimation, in order to access data hosted in different clinical institutions by simply transmitting the model parameters, that will be in turn updated by using the local available data. This approach is extended to the definition of stochastic optimization strategies in which model parameters are optimized on local datasets, and then summarized in a meta-analysis context. Finally, this project studies strategies for aggregating the information from heterogeneous datasets, accounting for missing modalities due to different study design and protocols. The developed methodology finds important applications within the context of Big Data, for the development of effective learning strategies for massive datasets in the context of medical imaging (such as with the UK biobank), and beyond.
3.4 Computational Anatomy, Geometric Statistics
Computational anatomy is an emerging discipline at the interface of geometry, statistics and image analysis which aims at developing algorithms to model and analyze the biological shape of tissues and organs. The goal is not only to establish generative models of organ anatomies across diseases, populations, species or ages but also to model the organ development across time (growth or aging) and to estimate their variability and link to other functional, genetic or structural information. Computational anatomy is a key component to support computational physiology and is evidently crucial for building the e-patient and to support e-medicine.
Pivotal applications include the spatial normalization of subjects in neuroscience (mapping all the anatomies into a common reference system) and atlas to patient registration to map generic knowledge to patient-specific data. Our objectives will be to develop new efficient algorithmic methods to address the emerging challenges described below and to generate precise specific anatomical model in particular for the brain and the heart.
The objects of computational anatomy are often shapes extracted from images or images of labels (segmentation). The observed organ images can also be modeled using registration as the random diffeomorphic deformation of an unknown template (i.e. an orbit). In these cases as in many other applications, invariance properties lead us to consider that these objects belong to non-linear spaces that have a geometric structure. Thus, the mathematical foundations of computational anatomy rely on statistics on non-linear spaces.
Geometric Statistics aim at studying this abstracted problem at the theoretical level. Our goal is to advance the fundamental knowledge in this area, with potential applications to new areas outside of medical imaging. Beyond the now classical Riemannian spaces, we aim at developping the foundations of statistical estimation on affine connection spaces (e.g. Lie groups), quotient and stratified metric spaces (e.g. orbifolds and tree spaces). In addition to the curvature, one of the key problem is the introduction of singularities at the boundary of the regular strata (non-smooth and non-convex analysis).
A second objective is to develop parametric and non-parametric dimension reduction methods in non-linear space. An important issue is to estimate efficiently not only the model parameters (mean point, subspace, flag) but also their uncertainty. We also want to quantify the influence of curvature and singularities on non-asymptotic estimation theory since we always have a finite (and often too limited) number of samples. A key challenge in developing such a geometrization of statistics will not only be to unify the theory for the different geometric structures, but also to provide efficient practical algorithms to implement them.
A third objective is to learn the geometry from the data. In the high dimensional but low sample size (small data) setting which is the common situation in medical data, we believe that invariance properties are essential to reasonably interpolate and approximate. New apparently antagonistic notions like approximate invariance could be the key to this interaction between geometry and learning.
Beyond the traditional statistical survey of the anatomical shapes that is developed in computational anatomy above, we intend to explore other application fields exhibiting geometric but non-medical data. For instance, applications can be found in Brain-Computer Interfaces (BCI), tree-spaces in phylogenetics, Quantum Physics, etc.
3.5 Computational Physiology & Image-Guided Therapy
Computational Physiology aims at developing computational models of human organ functions, an important component of the e-patient , with applications in e-medicine and more specifically in computer-aided prevention, diagnosis, therapy planning and therapy guidance. The focus of our research is on descriptive (allowing to reproduce available observations), discriminative (allowing to separate two populations), and above all predictive models which can be personalized from patient data including medical images, biosignals, biological information and other available metadata. A key aspect of this scientific axis is therefore the coupling of biophysical models with patient data which implies that we are mostly considering models with relatively few and identifiable parameters. To this end, data assimilation methods aiming at estimating biophysical model parameters in order to reproduce available patient data are preferably developed as they potentially lead to predictive models suitable for therapy planning.
Previous research projects in computational physiology have led us to develop biomechanical models representing quasi-static small or large soft tissue deformations (e.g. liver or breast deformation after surgery), mechanical growth or atrophy models (e.g. simulating brain atrophy related to neurodegenerative diseases), heat transfer models (e.g. simulating radiofrequency ablation of tumors), and tumor growth models (e.g. brain or lung tumor growth).
To improve the data assimilation of biophysical models from patient data, a long term objective of our research will be to develop joint imaging and biophysical generative models in a probabilistic framework which simultaneously describe the appearance and function of an organ (or its pathologies) in medical images. Indeed, current approaches for the personalization of biophysical models often proceed in two separate steps. In a first stage, geometric, kinematic or/ functional features are first extracted from medical images. In a second stage, they are used by personalization methods to optimize model parameters in order to match the extracted features. In this process, subtle information present in the image which could be informative for biophysical models is often lost which may lead to limited personalization results. Instead, we propose to develop more integrative approaches where the extraction of image features would be performed jointly with the model parameter fitting. Those imaging and biophysical generative models should lead to a better understanding of the content of images, to a better personalization of model parameters and also better estimates of their uncertainty.
3.6 Computational Cardiology & Image-Based Cardiac Interventions
Computational Cardiology has been an active research topic within the Computational Anatomy and Computational Physiology axes of the previous Asclepios project, leading to the development of personalized computational models of the heart designed to help characterizing the cardiac function and predict the effect of some device therapies like cardiac resynchronisation or tissue ablation . This axis of research has now gained a lot of maturity and a critical mass of involved scientists to justify an individualized research axis of the new project Epione, while maintaining many constructive interactions with the 4 other research axes of the project. This will develop all the cardiovascular aspects of the e-patient for cardiac e-medicine.
The new challenges we want to address in computational cardiology are related to the introduction of new levels of modeling and to new clinical and biological applications. They also integrate the presence of new sources of measurements and the potential access to very large multimodal databases of images and measurements at various spatial and temporal scales.
4 Application domains
The main applications of our research are in the field of healthcare and more precisely the domain of digital medicine and biomedical data analysis. The axes of research presented above are related to many branches of medicine including cardiology, oncology, urology, neurology, otology, pneumology, radiology, surgery, dermatology, nuclear medicine. Within those branches, the applications cover the following different stages of medicine : prevention, diagnosis, prognosis, treatment.
5 Highlights of the year
5.1 Awards
- Nicholas Ayache is the laureate of the 2020 International Steven Hoogendijk Award that he received in Rotterdam in 2021. More info on this prize is available on the Wikipedia page.
- Irene Balelli was awarded with an ISFP position with the research project on Causal machine learning: from imaging to in silico trials.
- Marta Nunez Garcia was awarded with a Starting Research Position.
- Maxime Sermesant coordinated two successful EU proposals, SimCardioTest and inEurHeart.
- Benoît Audelan received the second prize of the STIC doctoral school for his PhD thesis.
- Hind Dadoun earned 2nd prize at the Pierre Laffitte PhD competition.
- Buntheng Ly received the Young Investigator Award at the 4th International Scientific Workshop of the IHU Liryc.
- Zihao Wang’s paper One-shot Learning Landmarks Detection46 was selected for the People’s Choice Award at MICCAI DALI 2021.
6 New software and platforms
Let us describe new/updated software.
6.1 New software
6.1.1 CardiacSegmentationPropagation
-
Keywords:
3D, Segmentation, Cardiac, MRI, Deep learning
-
Functional Description:
Training of a deep learning model which is used for cardiac segmentation in short-axis MRI image stacks.
- Publication:
-
Authors:
Qiao Zheng, Hervé Delingette, Nicolas Duchateau, Nicholas Ayache
-
Contact:
Qiao Zheng
6.1.2 CardiacMotionFlow
-
Keywords:
3D, Deep learning, Cardiac, Classification
-
Functional Description:
Creation of a deep learning model for the motion tracking of the heart, extraction of characteristic quantities of the movement and shape of the heart to classify a sequence of cine-MRI cardiac images in terms of the types of pathologies (infarcted heart, dilated , hypertrophied, abnormality of the right ventricle).
-
Authors:
Qiao Zheng, Hervé Delingette, Nicholas Ayache
-
Contact:
Qiao Zheng
6.1.3 MedINRIA
-
Keywords:
Visualization, DWI, Health, Segmentation, Medical imaging
-
Scientific Description:
MedInria aims at creating an easily extensible platform for the distribution of research algorithms developed at Inria for medical image processing. This project has been funded by the D2T (ADT MedInria-NT) in 2010, renewed in 2012. A fast-track ADT was awarded in 2017 to transition the software core to more recent dependencies and study the possibility of a consortium creation.The Empenn team leads this Inria national project and participates in the development of the common core architecture and features of the software as well as in the development of specific plugins for the team's algorithm.
-
Functional Description:
MedInria is a free software platform dedicated to medical data visualization and processing.
- URL:
-
Contact:
Olivier Commowick
-
Participants:
Maxime Sermesant, Olivier Commowick, Théodore Papadopoulo
-
Partners:
HARVARD Medical School, IHU - LIRYC, NIH
6.1.4 GP-ProgressionModel
-
Name:
GP progression model
-
Keywords:
Data modeling, Data visualization, Data integration, Machine learning, Biostatistics, Statistical modeling, Medical applications, Evolution, Brain, Uncertainly, Uncertainty quantification, Alzheimer's disease, Probability, Stochastic models, Stochastic process, Trajectory Modeling, Marker selection, Health, Statistic analysis, Statistics, Bayesian estimation
-
Functional Description:
Disease progression modeling (DPM) of Alzheimer's disease (AD) aims at revealing long term pathological trajectories from short term clinical data. Along with the ability of providing a data-driven description of the natural evolution of the pathology, DPM has the potential of representing a valuable clinical instrument for automatic diagnosis, by explicitly describing the biomarker transition from normal to pathological stages along the disease time axis.
In this software we reformulate DPM within a probabilistic setting to quantify the diagnostic uncertainty of individual disease severity in an hypothetical clinical scenario, with respect to missing measurements, biomarkers, and follow-up information. The proposed formulation of DPM provides a statistical reference for the accurate probabilistic assessment of the pathological stage of de-novo individuals, and represents a valuable instrument for quantifying the variability and the diagnostic value of biomarkers across disease stages.
This software is based on the publication:
Probabilistic disease progression modeling to characterize diagnostic uncertainty: Application to staging and prediction in Alzheimer's disease. Marco Lorenzi, Maurizio Filippone, Daniel C. Alexander, Sebastien Ourselin Neuroimage. 2019 Apr 15,190:56-68. doi: 10.1016/j.neuroimage.2017.08.059. Epub 2017 Oct 24. HAL Id : hal-01617750 https://hal.archives-ouvertes.fr/hal-01617750/
-
Release Contributions:
- New interface and output - Completely based on Pytorch
- URL:
- Publication:
-
Contact:
Marco Lorenzi
-
Participant:
Marco Lorenzi
6.1.5 Music
-
Name:
Multi-modality Platform for Specific Imaging in Cardiology
-
Keywords:
Medical imaging, Cardiac Electrophysiology, Computer-assisted surgery, Cardiac, Health
-
Functional Description:
MUSIC is a software developed by the Asclepios research project in close collaboration with the IHU LIRYC in order to propose functionalities dedicated to cardiac interventional planning and guidance. This includes specific tools (algorithms of segmentation, registration, etc.) as well as pipelines. The software is based on the MedInria platform.
- URL:
-
Contact:
Maxime Sermesant
-
Participants:
Florent Collot, Mathilde Merle, Maxime Sermesant
-
Partner:
IHU- Bordeau
6.1.6 SOFA
-
Name:
Simulation Open Framework Architecture
-
Keywords:
Real time, Multi-physics simulation, Medical applications
-
Functional Description:
SOFA is an Open Source framework primarily targeted at real-time simulation, with an emphasis on medical simulation. It is mostly intended for the research community to help develop new algorithms, but can also be used as an efficient prototyping tool. Based on an advanced software architecture, it allows the creation of complex and evolving simulations by combining new algorithms with algorithms already included in SOFA, the modification of most parameters of the simulation (deformable behavior, surface representation, solver, constraints, collision algorithm etc.) by simply editing an XML file, the building of complex models from simpler ones using a scene-graph description, the efficient simulation of the dynamics of interacting objects using abstract equation solvers, the reuse and easy comparison of a variety of available methods.
-
News of the Year:
The new version v20.06 has been released including new elements on SoftRobots + ModelOrderReduction integration, in addition to an improved architecture and lots of cleans and bugfixes.
- URL:
-
Contact:
Hugo Talbot
-
Participants:
Christian Duriez, François Faure, Hervé Delingette, Stephane Cotin, Hugo Talbot, Maud Marchal
-
Partner:
IGG
6.1.7 geomstats
-
Name:
Computations and statistics on manifolds with geometric structures
-
Keywords:
Geometry, Statistic analysis
-
Scientific Description:
Geomstats is an open-source Python package for computations and statistics on manifolds. The package is organized into two main modules: “geometry“ and “learning“.
The module `geometry` implements concepts in differential geometry, and the module `learning` implements statistics and learning algorithms for data on manifolds.
The goal is to provide an easily accessible library for learning algorithms on Riemannian manifolds.
-
Functional Description:
Geomstats is a Python package that performs computations on manifolds such as hyperspheres, hyperbolic spaces, spaces of symmetric positive definite matrices and Lie groups of transformations. It provides efficient and extensively unit-tested implementations of these manifolds, together with useful Riemannian metrics and associated Exponential and Logarithm maps. The corresponding geodesic distances provide a range of intuitive choices of Machine Learning loss functions. We also give the corresponding Riemannian gradients. The operations implemented in geomstats are available with different computing backends such as numpy, tensorflow and keras. Geomstats manifold computations have are integrated into keras deep learning framework thanks to GPU-enabled implementations.
-
Release Contributions:
Major update of the library with full documentation and code restructuring before publication in JMLR.
-
News of the Year:
In 2021, several coding sprints were organized in order to restructure and enhance the library. Par of the PhD of Nicolas Guigui was dedicated to the mathematical structuring of quotient spaces through submersion mechanisms and to parallel transport with applications to Kendal shape spaces. A challenge was organized in conjunction with ICLR 2021.
- URL:
- Publications:
-
Contact:
Nicolas Guigui
-
Participants:
Nicolas Guigui, Xavier Pennec, Yann Thanwerdas, Nina Miolane
-
Partner:
Stanford Department of Statistics
6.1.8 MC-VAE
-
Name:
Multi Channel Variational Autoencoder
-
Keywords:
Machine learning, Artificial intelligence, Medical applications, Dimensionality reduction, High Dimensional Data, Unsupervised learning, Heterogeneity
-
Scientific Description:
Interpretable modeling of heterogeneous data channels is essential in medical applications, for example when jointly analyzing clinical scores and medical images. Variational Autoencoders (VAE) are powerful generative models that learn representations of complex data. The flexibility of VAE may come at the expense of lack of interpretability in describing the joint relationship between heterogeneous data. To tackle this problem, this software extends the variational framework of VAE to introduce sparsity of the latent representation, as well as interpretability when jointly account for latent relationships across multiple channels. In the latent space, this is achieved by constraining the variational distribution of each channel to a common target prior. Parsimonious latent representations are enforced by variational dropout. Experiments on synthetic data show that our model correctly identifies the prescribed latent dimensions and data relationships across multiple testing scenarios. When applied to imaging and clinical data, our method allows to identify the joint effect of age and pathology in describing clinical condition in a large scale clinical cohort.
-
Functional Description:
This software implements the work published in the paper "Sparse Multi-Channel Variational Autoencoder for the Joint Analysis of Heterogeneous Data" presented at the conference ICML 2019 (Long Beach, California, USA).
The software extends classical variational autoencoders by identifying a joint latent code associated to heterogeneous data represented in different channels. The software is implemented in Python and is based on Pytorch. It can be applied to any kind of data arrays, and provides functions for optimisation, visualisation and writing of the modelling results.
-
Release Contributions:
First release
-
News of the Year:
Method presented in the International Conference on Machine Learning (ICML 2019).
- URL:
-
Contact:
Luigi Antelmi
-
Participants:
Luigi Antelmi, Marco Lorenzi, Nicholas Ayache
-
Partner:
CoBteK
6.1.9 SOFA-CardiacReduction
-
Keywords:
Simulation, 3D modeling, Model Order Reduction, Cardiac
-
Scientific Description:
Modification of a finite element deformation model : meshless approach and frame-based description, reduction in the number of affine degrees of freedom and integration points.
-
Functional Description:
This SOFA plugin is intented to build a reduced model for deformable solids (especially cardiac simulations).
-
Contact:
Gaetan Desrues
-
Participants:
Gaetan Desrues, Hervé Delingette, Maxime Sermesant
6.1.10 Fed-BioMed
-
Name:
A general software framework for federated learning in healthcare
-
Keywords:
Federated learning, Medical applications, Machine learning, Distributed Applications, Deep learning
-
Scientific Description:
While data in healthcare is produced in quantities never imagined before, the feasibility of clinical studies is often hindered by the problem of data access and transfer, especially regarding privacy concerns. Federated learning allows privacy-preserving data analyses using decentralized optimization approaches keeping data securely decentralized. There are currently initiatives providing federated learning frameworks, which are however tailored to specific hardware and modeling approaches, and do not provide natively a deployable production-ready environment. To tackle this issue, Fed-BioMed proposes an open-source federated learning frontend framework with application in healthcare. Fed-BioMed framework is based on a general architecture accommodating for different models and optimization methods
-
Functional Description:
The project is based on the development of a distributed software architecture, and the establishment of a server instance from which remote experiments are triggered on the clients sites. The software is distributed to the client's sites, allowing to run machine learning models on the local data. Model parameters are then trasmitted to the server for federated aggregation.
Fed-BioMed finds application in all projects based on the development of learning models for multi-centric studies.
-
Release Contributions:
Fed-BioMed is based on Python and Pytorch. The software was recently revised (under an ADT) to adopt the libraries Pygrid and Pysyft.
-
News of the Year:
The develpment is ongoing and Fed-BioMed is currently at the version 3.4. For up-to-date news refer to the official project's page: https://fedbiomed.gitlabpages.inria.fr/
- URL:
- Publication:
-
Authors:
Marco Lorenzi, Santiago Smith Silva Rincon, Marc Vesin, Tristan Cabel, Irene Balelli, Andréa Senacheribbe, Carlos Zubiaga Pena, Jonathan Levy
-
Contact:
Marco Lorenzi
6.1.11 EchoFanArea
-
Name:
delineation of the border of the fan in ultrasound
-
Keywords:
Ultrasound fan area, Deep learning, Statistics, Image processing
-
Functional Description:
This software allows the delimitation of the acquisition cone in ultrasound imaging and the inpainting of annotations (lines, characters) inside the cone. It allows both the perfect de-identification of the images but also to standardize the content of the images. It relies on a parametric probabilistic approach to generate a training dataset with region of interest (ROI) segmentation masks. This data will then be used to train a deep U-Net network to perform the same task in a supervised manner, thus considerably reducing the calculation time of the method, one hundred and sixty times faster. These images are then processed with existing filling methods to remove annotations present within the signal area.
- URL:
- Publication:
-
Authors:
Hind Dadoun, Hervé Delingette, Nicholas Ayache, Anne-Laure Rousseau
-
Contact:
Hind Dadoun
-
Partner:
Nhance
6.1.12 ProMFusion
-
Keywords:
Image segmentation, Data fusion
-
Functional Description:
Code related to the paper "Robust Fusion of Probability Maps" by Benoît Audelan, Dimitri Hamzaoui, Sarah Montagne, Raphaële Renard-Penna and Hervé Delingette. The proposed approach allows to fuse probability maps in a robust manner with a spatial regularization of the consensus.
- URL:
- Publication:
-
Contact:
Benoit Audelan
-
Participants:
Benoit Audelan, Hervé Delingette, Dimitri Hamzaoui
6.1.13 SimulAD
-
Name:
Disease progression modeling for clinical intervention simulation
-
Keywords:
Data modeling, Clinical analysis, Clinical trial simulator, Alzheimer's disease, Pytorch, Variational Autoencoder, Ordinary differential equations
-
Scientific Description:
Recent failures of clinical trials in Alzheimer’s Disease underline the critical importance of identifying optimal intervention time to maximize cognitive benefit. While several models of disease progression have been proposed, we still lack quantitative approaches simulating the effect of treatment strategies on the clinical evolution. In this work, we present a data-driven method to model dynamical relationships between imaging and clinical biomarkers. Our approach allows simulating intervention at any stage of the pathology by modulating the progression speed of the biomarkers, and by subsequently assessing the impact on disease evolution.
-
Functional Description:
A machine-learning framework allowing to simulate the impact of intervention on the long-term progression of imaging and clinical biomarkers from collections of healthcare data
-
Contact:
Marco Lorenzi
-
Participants:
Clément Abi Nader, Marco Lorenzi, Nicholas Ayache, Philippe Robert
-
Partner:
Université Côte d'Azur (UCA)
6.1.14 SOFA-CardiacReduction
-
Keywords:
Simulation, 3D modeling, Model Order Reduction, Cardiac
-
Functional Description:
This SOFA plugin is intented to build a reduced model for deformable solids (especially cardiac simulations).
-
Contact:
Hervé Delingette
7 New results
7.1 Medical Image Analysis & Machine Learning
7.1.1 Lung Nodule Detection for Lung cancer screening
Keywords: Image Detection, Lung cancer, Deep Learning.
Participants: Benoit Audelan [Correspondent], Stéphanie Lopez, Hervé Delingette.
This work was partially funded by the French government, through the UCAJEDI “Investments in the Future” project managed by the National Research Agency (ANR) with the reference number ANR-15-IDEX-01
We have trained a deep learning system for lung nodule detection from low dose CT scans on the LIDC-IDRI dataset containing 888 CT scans. In this dataset, radiologists characterized nodules exclusively based on radiological criteria.We have then tested that algorithm on 1179 patients from the National Lung Screening Trial (NLST) study with biopsy-confirmed nodule malignancy (Figure 4). We have demonstrated the ability of our system to detect malignant lesions one year prior to their diagnosis.
This work was published in the 2021 ESR congress48 and included in the thesis of Benoit Audelan56.
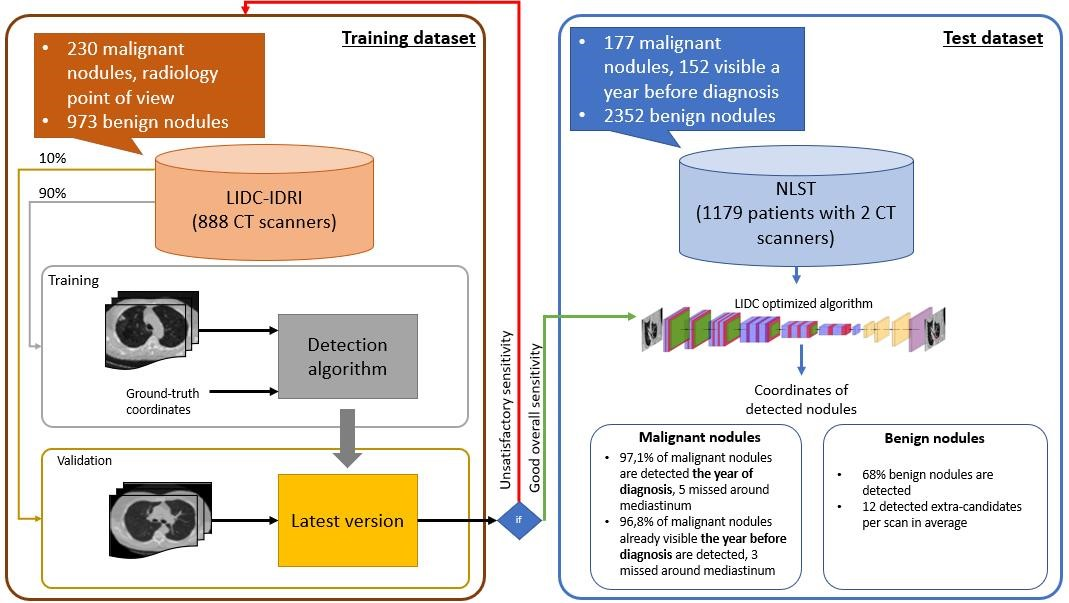
7.1.2 AI-based real-time diagnostic aid for abdominal organs in ultrasound
This work has been supported by the French government, through the 3IA Côte d'Azur Investments in the Future project managed by the National Research Agency (ANR) with the reference number ANR-19-P3IA-0002.
Keywords:
Participants: Hind Dadoun [Correspondent], Hervé Delingette, Anne-Laure Rousseau, Eric De Kerviler, Nicholas Ayache.
The goal of the thesis is to develop AI and machine learning methods for the automatic detection of abdominal organs from time series of ultrasound imaging and the automatic detection and diagnosis of pathologies. Ultrasound (US) images usually contain identifying information outside the ultrasound fan area and manual annotations placed by the sonographers during exams. For those images to be exploitable in a Deep Learning framework we propose an open-source tool 34 to process those images and remove all annotations as shown in Figure 5.
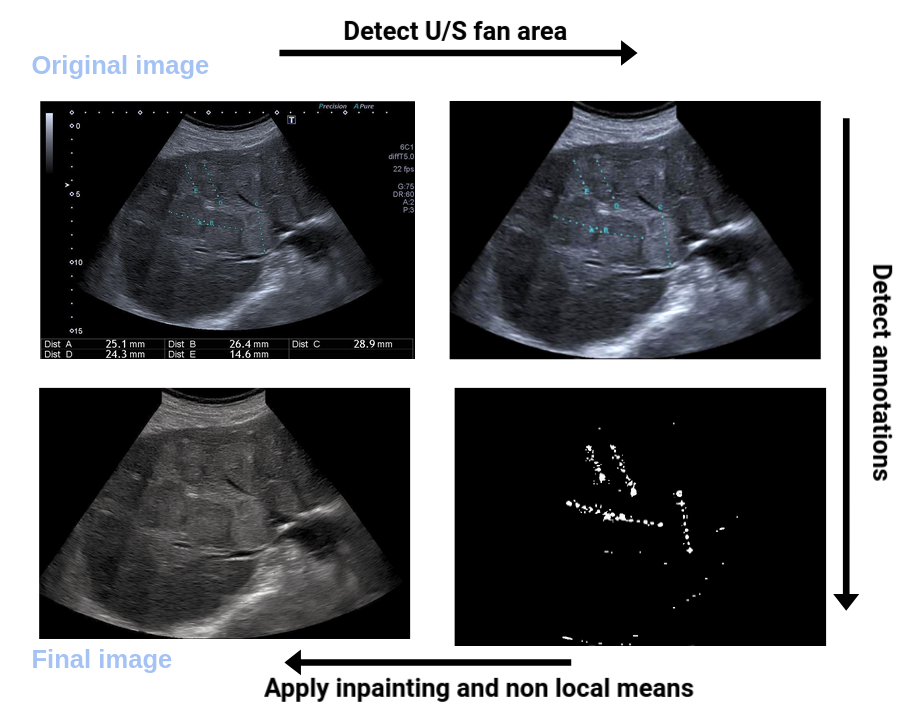
7.1.3 Machine Learning for MR-TRUS Image Fusion
This work has been supported by the French government, through the 3IA Côte d'Azur Investments in the Future project managed by the National Research Agency (ANR) with the reference number ANR-19-P3IA-0002.
Keywords:
Participants: Zhijie Fang [Correspondent], Eric Gaudard, Michael Baumann, Nicholas Ayache, Hervé Delingette.
The diagnosis of prostate cancer widely relies on multiparametric MR images. The localisation of cancer lesions in those images by a specialized radiologist serves to plan targeted prostate biopsies through the fusion of MR and intra-operative transrectal ultrasound (TRUS) images (Figure 6).
The objective of this work is to address the probabilistic registration of MR-TRUS prostate images. The challenge associated with this objective is to jointly learn the spatial transformation and the MR-US similarity metrics within an efficient framework.
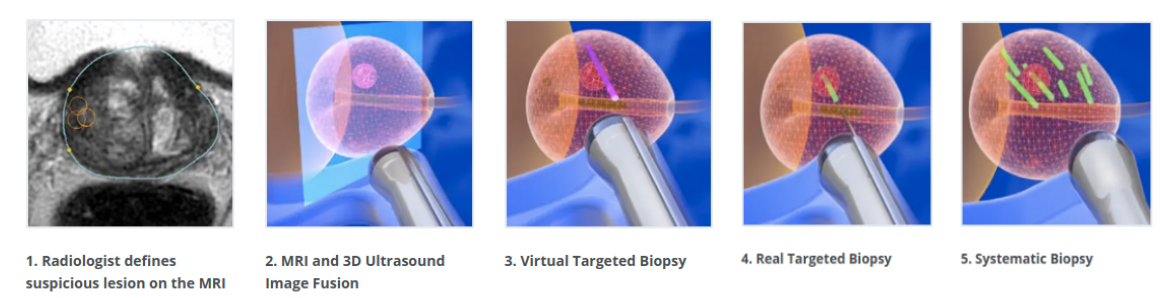
7.1.4 AI-Based Diagnosis of Prostate Cancer from Multiparametric MRI
This work has been supported by the French government, through the 3IA Côte d'Azur Investments and UCA DS4H Investments in the Future project managed by the National Research Agency (ANR) with the reference numbers ANR-19-P3IA-0002and ANR-17-EURE-0004.
Keywords:
Participants: Dimitri Hamzaoui [Correspondent], Sarah Montagne, Raphaele Renard-Penna, Nicholas Ayache, Hervé Delingette.
The aim of our work is to detect and characterize tumorous lesions of the prostate from multiparametric MRI using deep learning-based methods. We investigated several aspects of this problem, including:
- The automatic zonal segmentation of the prostate thanks to a 3D UNet-based network and the detection of lesions using zonal information provided by it (Figure 7, left).
- The study of the variability between the segmentations from different raters 27 and between their volume measurements 20 (Figure 7).
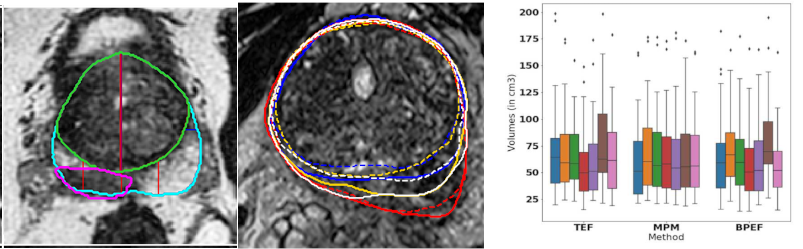
7.1.5 Attention-based Multiple Instance Learning with Mixed Supervision
This work has been supported by the French government, through the 3IA Côte d'Azur Investments in the Future project managed by the National Research Agency (ANR) with the reference number ANR-19-P3IA-0002
Keywords:
Participants: Paul Tourniaire [Correspondent], Marius Ilié, Paul Hofman, Nicholas Ayache, Hervé Delingette.
We have developped an Attention-based Multiple Instance learning algorithm for the classification and detection of tumors in Whole Slide Images.
More precisely, we have introduced the possibility of mixed supervision, combining tile-based and slide-based annotations in the Clustering-constrained Attention Multiple Instance Learning (CLAM) model. This has led to an improvement of both classification and localization performances. This work was published at COMPAY 2021 Workshop 45. An overview of the work done is available in Figure 8.
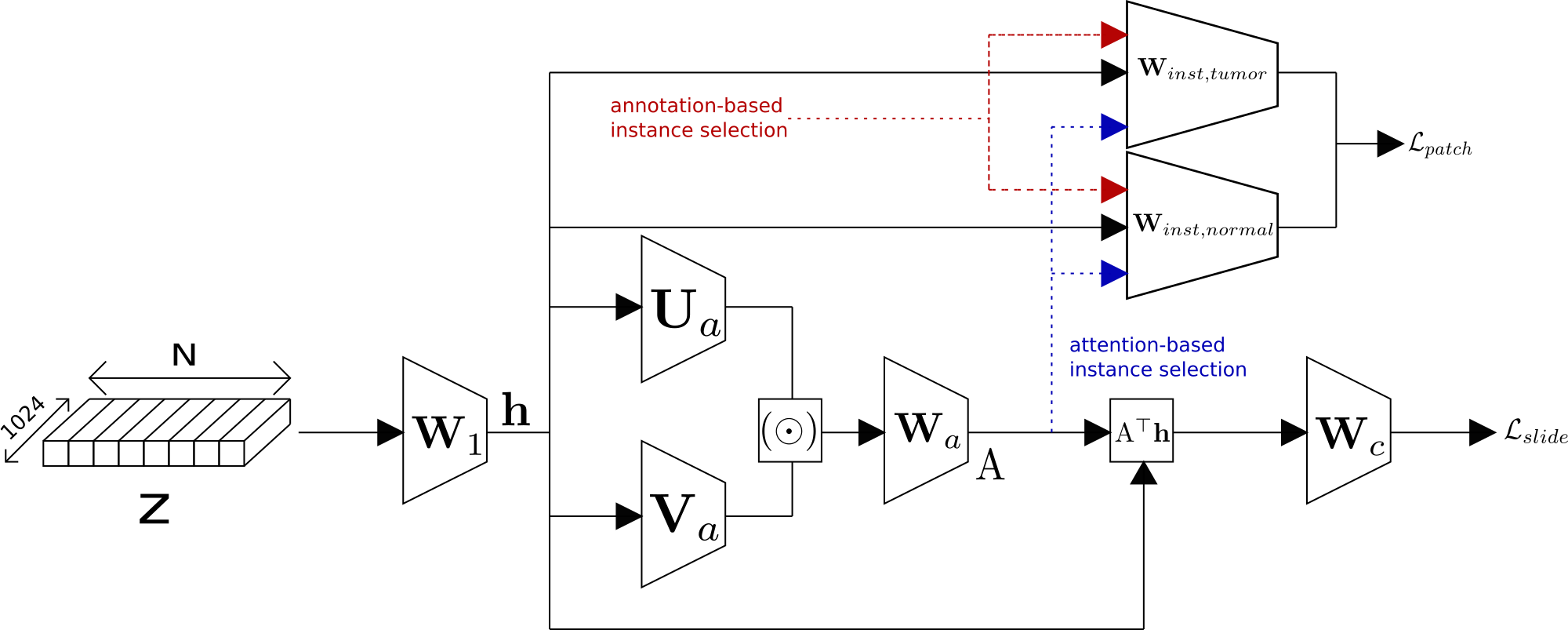
7.1.6 Deep generative learning for medical data processing, analysis and modeling: application to cochlea CT imaging
This work is funded by the Provence-Alpes-Côte-d'Azur region, the Université Côte d'Azur and Oticon Medical through CIMPLE research project.
Keywords:
Participants: Zihao Wang [Correspondent], Clair Vandersteen, Charles Raffaelli, Nicolas Guevara, François Patou, Hervé Delingette.
Our work is focused on generative learning which can help in many aspects of the processing, understanding, and modeling of CT images of the inner ear :
- First, we propose a novel Bayesian framework 30for shape constrained image segmentation based on parametric shape models (instead of parametric spatial transformations). The trade-off between the appearance and shape models is governed by an interpretable parameter : the reference length.
- We also propose a GAN-based metal artifact reduction method that relies on simulated training data and is suitable for preand postoperative images. To the best of our knowledge, our approach is the first MAR algorithm that combines the physical simulation of metal artifacts with 3D GAN networks. 31
- We also tackled the issue of automatic landmark annotation of 3D volumetric images from a single example based on a one-shot learning method 46. It involves the iterative training of a shallow convolutional neural network combined with a 3D registration algorithm in order to perform automatic organ localization and landmark matching.
- Finally, we proposed in 70, a variational auto-encoder based on Langevin dynamic flow. Tight approximate posterior distribution was achieved by incorporating the gradients information in the inference
All these works are also described in the PhD manuscript of Zihao Wang 59.
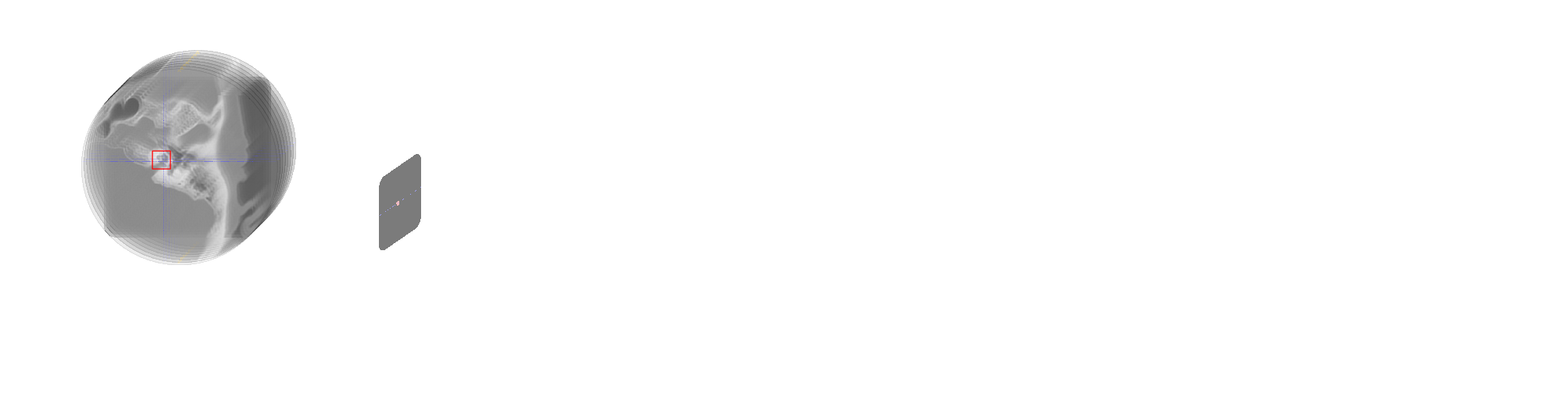
7.2 Imaging & Phenomics, Biostatistics
7.2.1 Statistical Learning of Heterogeneous Data in Large-Scale Clinical Databases
Acknowledgments: IDEX UCAJEDI MNC3 Project (ANR-15-IDEX-01); 3IA (ANR-19-P3IA-0002); the OPAL infrastructure from Université Côte d'Azur.
Keywords: Ordinary Differential Equations, Generative models, Alzheimer's Disease, Disease Progression Modelling.
Participants: Clement Abi Nader [Correspondent], Nicholas Ayache, Philippe Robert, Marco Lorenzi.
Alzheimer's disease is a neurodegenerative disorder whose dynamics still remain partially unknown. The aim of this work is to develop computational models to better understand Alzheimer's disease progression, based on the analysis of imaging and clinical data. Lately, a collaboration with the LANVIE laboratory from the Geneva University Hospitals (HUG) has allowed to test the developed model in a clinical context.
- Development of SimulAD, a statistical model for describing the evolution of Alzheimer's disease 54.
- The method relies on probabilistic generative model, coupled with a system of Ordinary Differential Equations (ODE) to model Alzheimer's disease (Figure 10).
- Recent work focuses on simulating the effect of drug intervention on the progression of Alzheimer's disease 6.
- Assessing the validity and generalization of the method, by applying SimulAD on a new cohort from the Geneva Memory Center (GMC) 7.
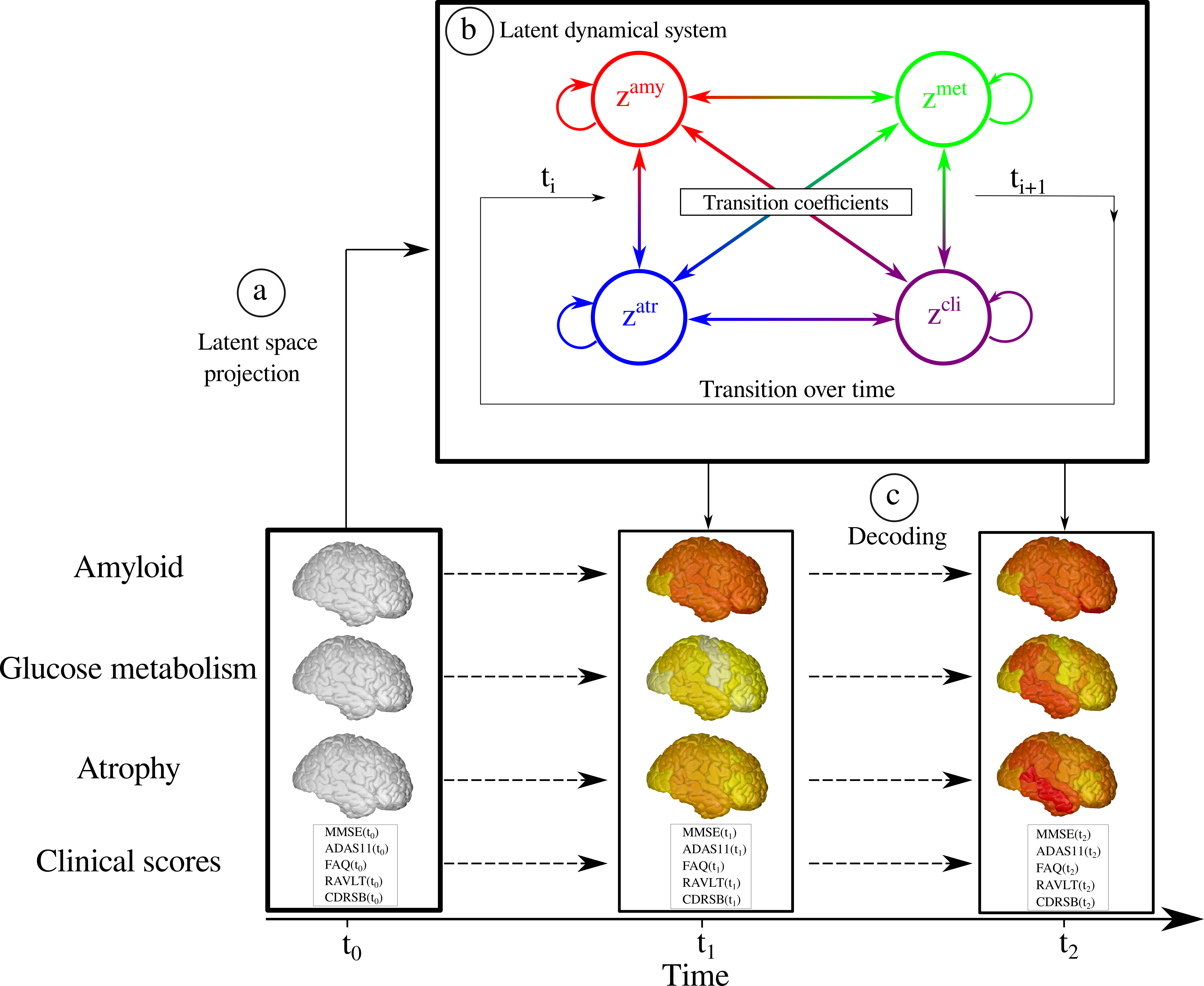
7.2.2 Combining Multi-Task Learning and Multi-Channel Variational Auto-Encoders to Exploit Datasets with Missing Observations - Application to Multi-Modal Neuroimaging Studies in Dementia
Acknowledgments: IDEX UCAJEDI MNC3 Project (ANR-15-IDEX-01); 3IA (ANR-19-P3IA-0002); the OPAL infrastructure from Université Côte d'Azur.
Keywords: Multi Task Learning, Missing Data, Multimodal Data Analysis..
Participants: Luigi Antelmi [Correspondent], Nicholas Ayache [IRCCS Centro San Giovanni di Dio, Fatebenefratelli, Brescia], Philippe Robert [IRCCS Centro San Giovanni di Dio, Fatebenefratelli, Brescia], Federica Ribaldi [IRCCS Centro San Giovanni di Dio, Fatebenefratelli, Brescia], Valentina Garibotto [Department of Medical Imaging, Geneva University Hospitals, Geneva], Giovanni B. Frisoni [Department of Psychiatry, Geneva University Hospitals, Geneva], Marco Lorenzi.
The aim of this work is to build scalable learning models for the joint analysis of heterogeneous biomedical data, to improve diagnosis, treatment, and monitoring of neurological and psychiatric diseases, in collaboration with the Institut Claude Pompidou (CHU of Nice), within the MNC3 initiative (Médecine Numérique: Cerveau, Cognition, Comportement). Data comes from collections of brain imaging, biological and clinical evaluations available in current large-scale databases such as ADNI and local clinical cohorts, where the joint analysis is affected by data missingness, represented by non-overlapping sets of modalities across subjects (e.g. imaging data, clinical scores, biological measurements) and across datasets. The main contributions and results of this work are:
- a multi-task generative latent-variable model (Fig. where the common variability across datasets stems from the estimation of a shared latent representation across heterogeneous data modalities 55;
- to consistently analyze high-dimensional and heterogeneous information with missing data (Figure 11).

7.2.3 Development of Genome-to-Phenome association methods using biologically inspired constraints
This work was funded by the Neuromod Institut of Université Côte d'Azur and the grant ANR PARIS-15087.
Keywords:
Participants: Marie Deprez, Julien Moreira, Maxime Sermesant, Marco Lorenzi.
In the study of complex and rare diseases, genomics analyses have the potential to lead to important discoveries in identifying the genetics underpinning pathological traits. Yet, such analyses are faced with important challenges posed by the large dimensionality of the data, and by the need for interpretable findings. In this paper, we propose Bayesian Genome-to-Phenome Sparse Regression (G2PSR), a novel multivariate regression method to improve the detection and interpretation of genome-to-phenome associations, using biologically inspired constraints and variable selection through sparsity 15. Through benchmark experiment, we demonstrate that G2PSR outperforms state-of-the-art methods with better accuracy at identifying phenotypic-related genes, and by associating them to multiple phenotypic features. Additionally, we observe that our framework is highly scalable with respect to the overall number of SNPs and genes to be jointly analyzed. Overall, our study shows that G2PSR is an effective tool to identify genome-to-phenome associations in a high-dimension/low-sample size regime, and can thus be employed in future challenging applications, such as imaging genetics and rare disease analysis. This project was developed following multiple steps:
- Development of the G2PSR method (Bayesian Genome-to-Phenome Sparse Regression), Figure 12.A.,
- Benchmark of G2PSR and comparison with state-of-the-art group-sparse methods on synthetic genome-to-phenome data,
- Application to real data from ADNI, Figure 12.B.C.
- Preparation of the following steps for the upgrading of the G2PSR method, such as accounting for multi-omics data types and constraints for the upcoming MITOMICS project (Inserm programme MAladies Rares).
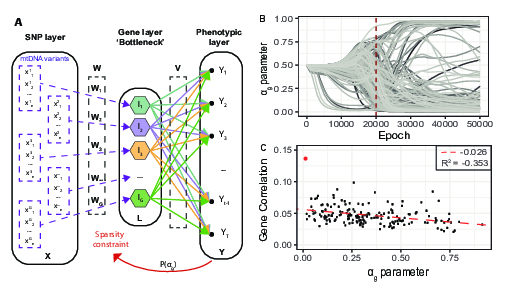
7.2.4 Exploring latent dynamical models for failure prediction in time-series of high-dimensional and heterogeneous data
This work was funded by 3IA Côte d'Azur
Keywords:
Participants: Etrit Haxholli, Marco Lorenzi.
Normalizing flows provide a theoretical framework for normality modelling, while extreme value theory is useful in the choice of an anomaly threshold. Our contributions include:
- Under some assumptions, we give theoretical guarantees that the tail of the marginal distribution coincides with the thickest tail of distributions defined on the range of the marginalized out variables (Figure 13).
- We augment Neural ODE flows in a manner such that the transformation remains a diffeomorphism and give a closed form of its Jacobian using our continuous generalization of the chain rule. Using complimentary dual autoregressive neural networks, we model incompressible vector fields (Figure 13).
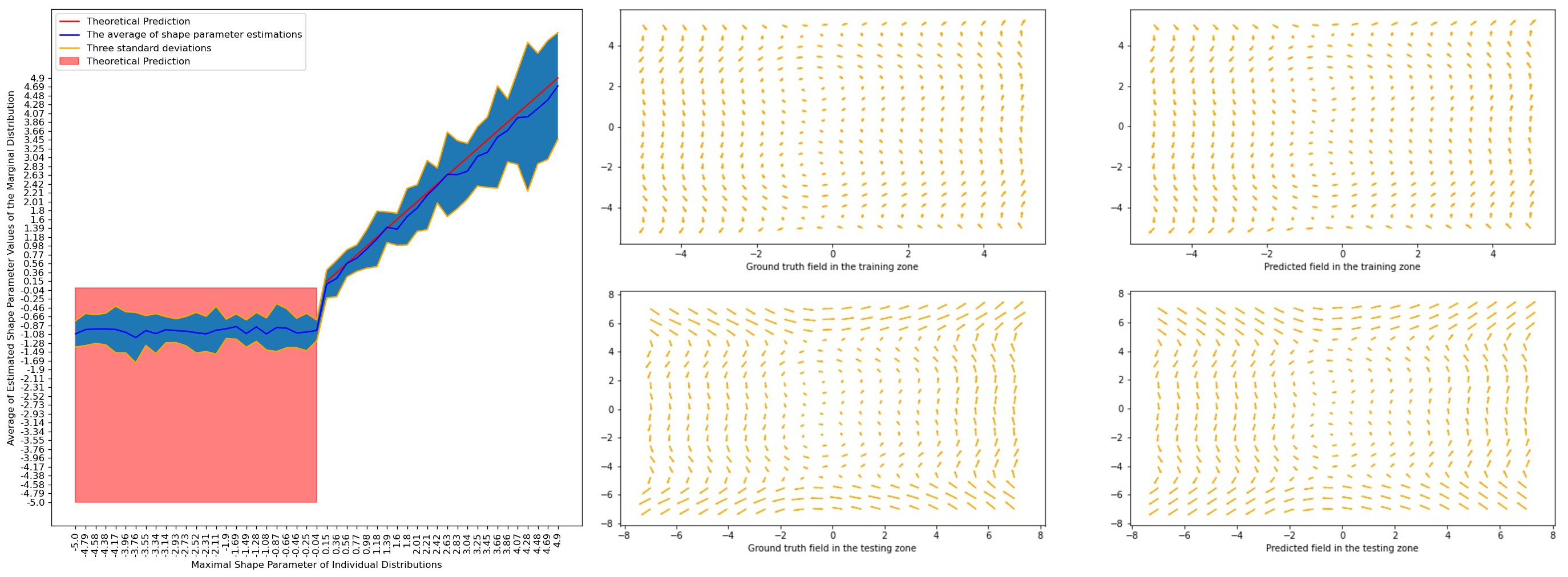
7.2.5 On the role of Fetal Fraction in NIPT (Non-Invasive Prenatal Testing) procedures
ANR-15-IDEX-01
Keywords:
Participants: Marco Milanesio, Véronique Duboc, David Pratella, John Boudjarane, Stéphane Descombes, Véronique Paquis-Flucklinger, Silvia Bottini.
Noninvasive prenatal testing (NIPT) consists of determining fetal aneuploidies by quantifying copy number alteration from the sequencing of cell-free DNA (cfDNA) from maternal blood. Due to the presence of cfDNA of fetal origin in maternal blood, in silico approaches have been developed to accurately predict fetal aneuploidies. In this work 16 we:
- run a benchmark on several tools for NIPT;
- empirically demonstrated results heterogeneity across different tools;
- developed an integrated pipeline to assess the validity of NIPT tests (Figure 14);
- developed a novel method for accurate gender prediction in NIPT.
The code is publicly available on Github github.com/uca-msi/niptune.
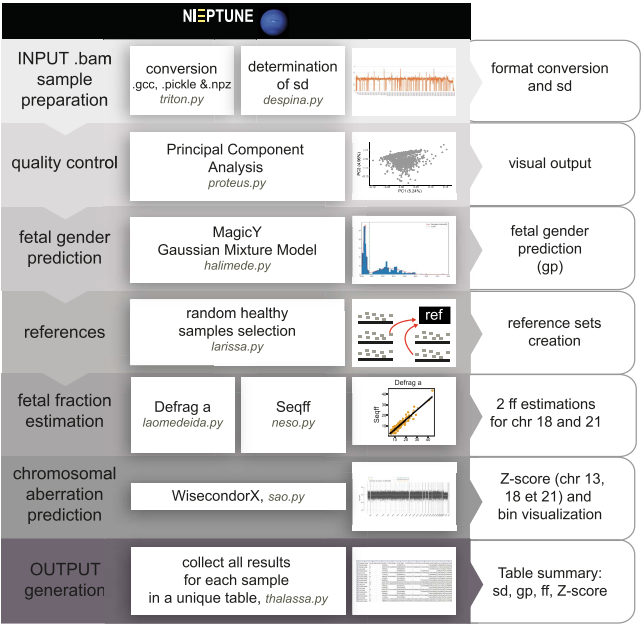
7.3 Computational Anatomy & Geometric Statistics
7.3.1 Population of Networks Analysis
This work was funded by the ERC grant Nr. 786854 G-Statistics from the European Research Council under the European Union's Horizon 2020 research and innovation program.
Keywords:
Participants: Anna Calissano [Correspondent], Xavier Pennec.
- We have been studying the variability and the Fréchet Mean of fMRI brain connectivity graphs in the Graph Space 71;
- We have been exploring possible stratified spaces as interesting embeddings for set of graphs with different number of nodes and Euclidean attributes on edges and nodes;
- We have been exploring how to define a statistical testing procedure for the analysis of a set of graphs with unlabelled nodes.
7.3.2 Geometric computational tools for shape comparison
This work was funded by the ERC grant Nr. 786854 G-Statistics from the European Research Council under the European Union's Horizon 2020 research and innovation program.
Keywords:
Participants: Nicolas Guigui [Correspondent], Elodie Maignant [UC Santa-Barbara, USA], Nina Miolane [UC Santa-Barbara, USA], Xavier Pennec.
The development of the geomstats.ai python library was pursued with the support of Inria SED-SAM team (Service d'Expérimentation et de Développement, Sophia Antipolis Méditerranée) through an ADT and the organisation of a hackathon at the SCAI in Paris. In particular, this led to theoretical developments on the computation of parallel transport on Lie groups 39 with an invariant metric, and on the space of Kendall shapes 37. We obtained improved convergence speed over state of the art methods.
The application of parallel transport as a tool to normalize deformations (Figure 15) was investigated in 38 and allowed to compare the effects of congenital heart diseases on the function of the right ventricle. An unexpected effect of the size of the ventricles on the transported motion parameters was in particular put into evidence. More details are available in the PhD mansucript of N. Guigui 58.
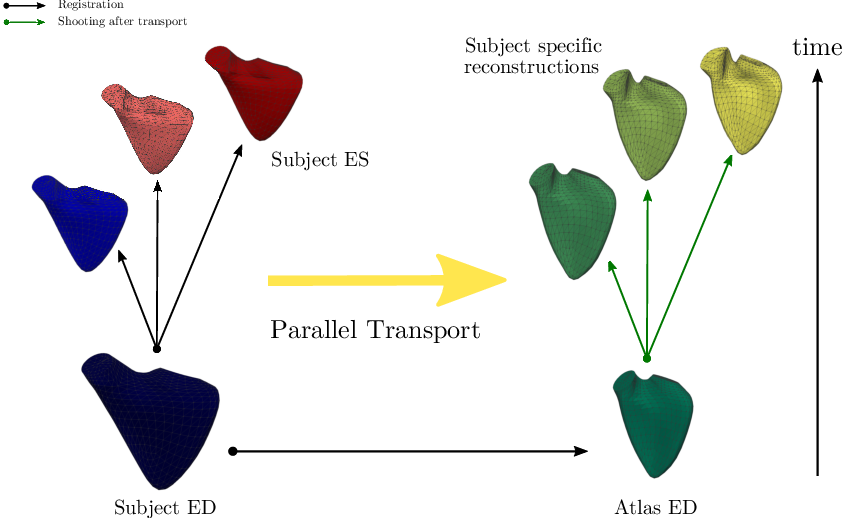
7.3.3 Computational tools for geometry in Medical Imaging
This work was funded by the ERC grant Nr. 786854 G-Statistics from the European Research Council under the European Union's Horizon 2020 research and innovation program.
Keywords:
Participants: Nicolas Guigui [Correspondent], Xavier Pennec.
Our work on Schild's and Pole ladder algorithms (Figure 16 (a)) was consolidated and published in Foundations of Computational Mathematics 19. We proved that this simple constructions based on geodesic parallelograms converged with a quadratic speed, and rate proportional to the Riemannian curvature. This work closed several years of attempts to establish the numerical accuracy of ladders methods. Illustrations on the 2-sphere and the special Euclidean group show that the theoretical errors we have established are measured with a high accuracy (Figure 16 (b)).
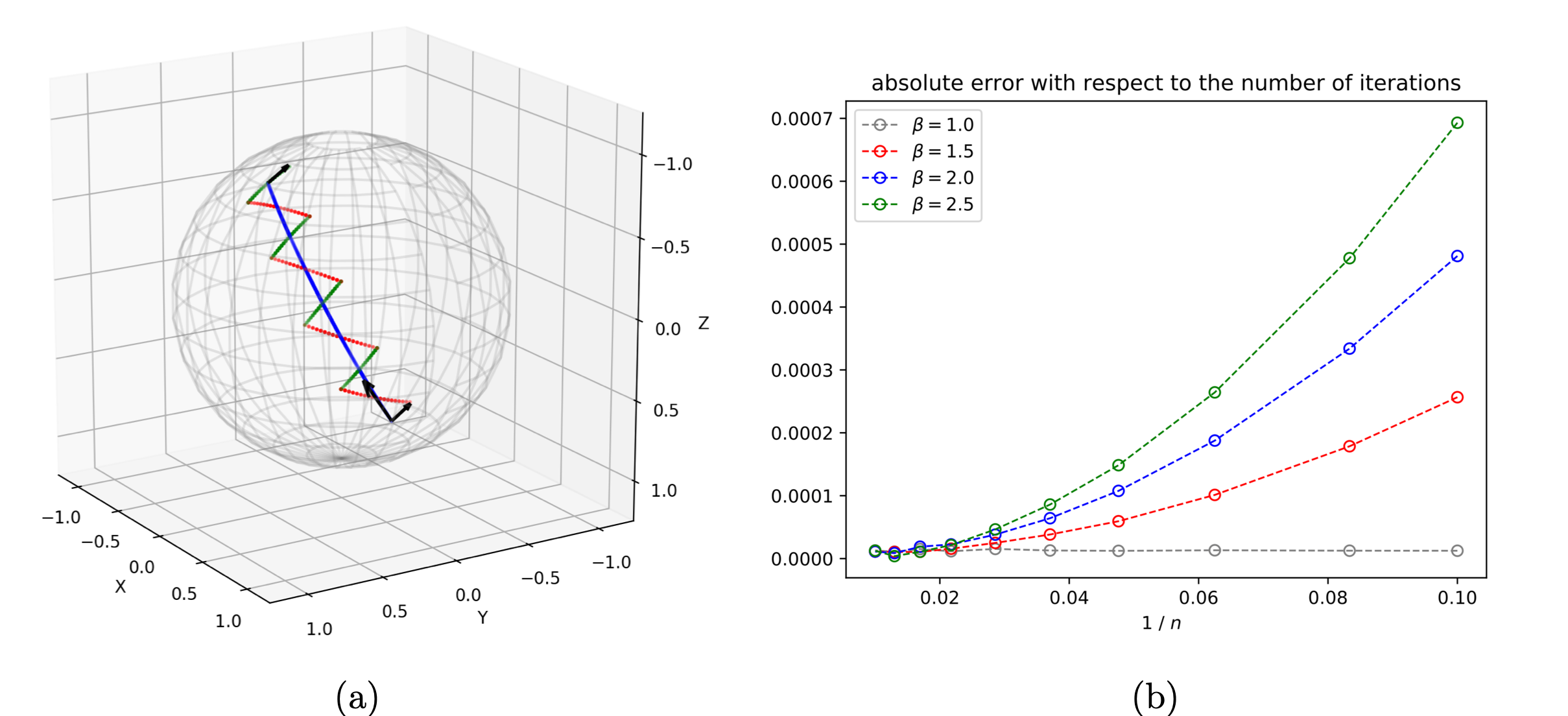
7.3.4 Geodesic squared exponential kernel for non-rigid shape registration
This work was supported by the ANRT Cifre contract 2019/0101 with QuantifiCare & Inria and by the French government through the 3IA Côte d'Azur Investments ANR-19-P3IA-0002 managed by the National Research Agency.
Keywords:
Participants: Florent Jousse [Correspondent], Xavier Pennec, Hervé Delingette, Arnaud Bletterer, Matilde Gonzalez.
We addressed in 41 the problem of non-rigid registration of 3D scans, which is at the core of shape modeling techniques. Firstly, we propose a new kernel based on geodesic distances for the Gaussian Process Morphable Models (GPMMs) framework. The use of geodesic distances into the kernel makes it more adapted to the topological and geometric characteristics of the surface and leads to more realistic deformations around holes and curved areas. Since the kernel possesses hyperparameters we have optimized them for the task of face registration in the FaceWarehouse dataset. We show that the Geodesic squared exponential kernel performs significantly better than state of the art kernels for the task of face registration on all the 20 expressions of the FaceWarehouse dataset (see Figure 17). Second, we propose a modification of the loss function used in the non-rigid ICP registration algorithm, that allows to weight the correspondences according to the confidence given to them. As a use case, we show that we can make the registration more robust to outliers in the 3D scans, such as non-skin parts.
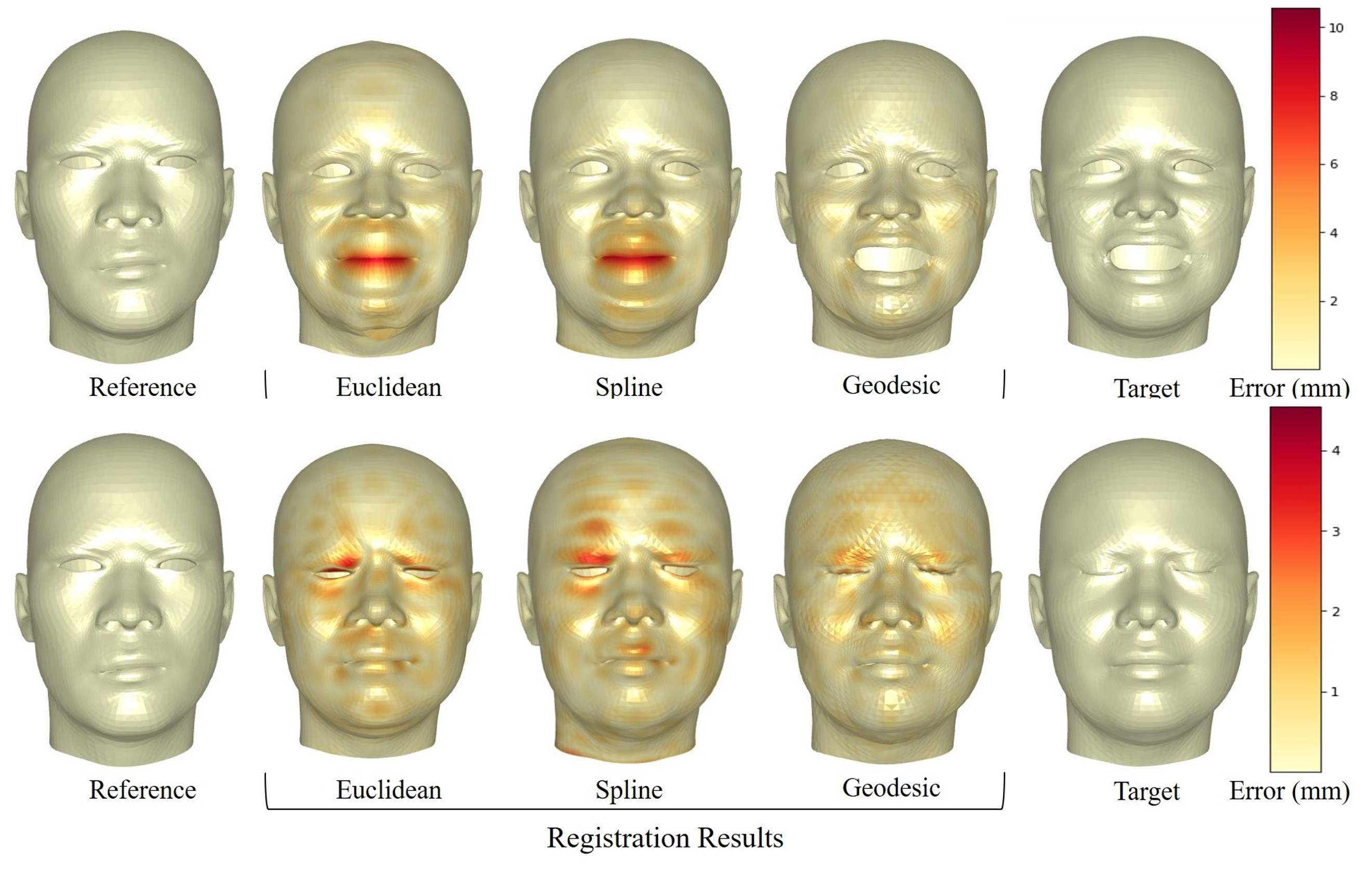
7.3.5 Analysis in Kendall shape spaces
ERC AdG G-Statistics.
Keywords:
Participants: Elodie Maignant [Correspondent], Guigui Nicolas, Trouvé Alain, Pennec Xavier.
This works focuses on analysis in Kendall shape spaces and its applications. In 37 we propose a new implementation of the parallel transport in these spaces (Figure 18). Kendall shape spaces and their related tools have been implemented in the library geomstats, together with some visualisation modules 64.
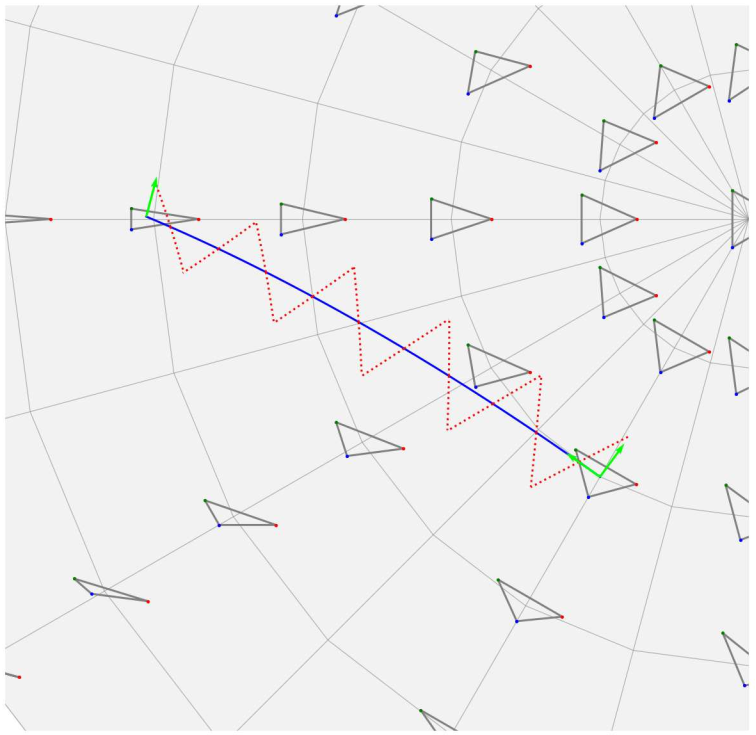
7.3.6 A geometric formulation of the central limit theorem for Principal Component Analysis
This work is funded by ERC AdG G-Statistics.
Keywords:
Participants: Dimbihery Rabenoro, Xavier Pennec.
The goal of this project is to determine the uncertainty of the estimation of subspaces arising from dimension reduction techniques for data living in Euclidian spaces or more generally in manifolds. For that, we study geometric methods using quotient spaces to characterize the concentration of the estimated subspace or family of subspaces resulting from the PCA procedure.
7.3.7 The quotient-affine geometry of full-rank correlation matrices
This project has received funding from the European Research Council (ERC) under the European Union's Horizon 2020 research and innovation program (grant G-Statistics agreement No 786854). This work has been supported by the French government, through the UCAJEDI Investments in the Future project managed by the National Research Agency (ANR) with the reference number ANR-15-IDEX-01 and through the 3IA Côte d'Azur Investments in the Future project managed by the National Research Agency (ANR) with the reference number ANR-19-P3IA-0002.
Keywords:
Participants: Yann Thanwerdas [Correspondent], Xavier Pennec.
The set of full-rank correlation matrices is a manifold called the open elliptope. It is not a vector space so Euclidean tools are not adapted to compute with correlation matrices. In 44, we describe the quotient-affine geometry of the open elliptope (Riemannian metric, Levi-Civita connection, geodesics, curvature), which allows:
- to interpolate, extrapolate, compute Fréchet means, perform PCA on correlation matrices,
- to define new geometries on covariance matrices which decouple the scales of the variables from the correlations between the variables (cf. Figure 19).
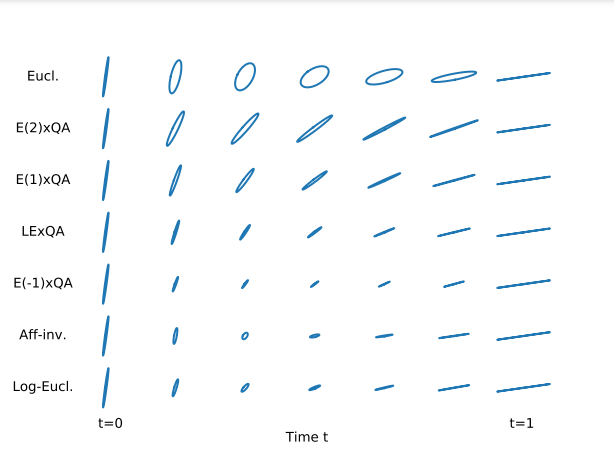
7.4 Computational Cardiology & Image-Based Cardiac Interventions
7.4.1 Deep Probabilistic Generative Model for the Inverse Problem of Electrocardiography.
This work is funded within the ERC Project ECSTATIC with the IHU Liryc, in Bordeaux.
Keywords:
Participants: Tania-Marina Bacoyannis [Inria, Université Côte d'Azur, Epione team, Sophia Antipolis, France], Hubert Cochet [IHU Liryc, Bordeaux, France], Maxime Sermesant [Inria, Université Côte d'Azur, Epione team, Sophia Antipolis, France].
The aim of this project is to propose a new method to solve the inverse problem of Electrocardiography, known to be ill-posed. The core of the model we have developed1 is a variational and conditional autoencoder using deep generative neural networks (Figure 20). Our unique model is able to produce advanced maps of cardiac activation by conditioning the electrical activity of the heart with signals measured on the torso surface and cardiac geometry. This method will soon be evaluated on clinical data.
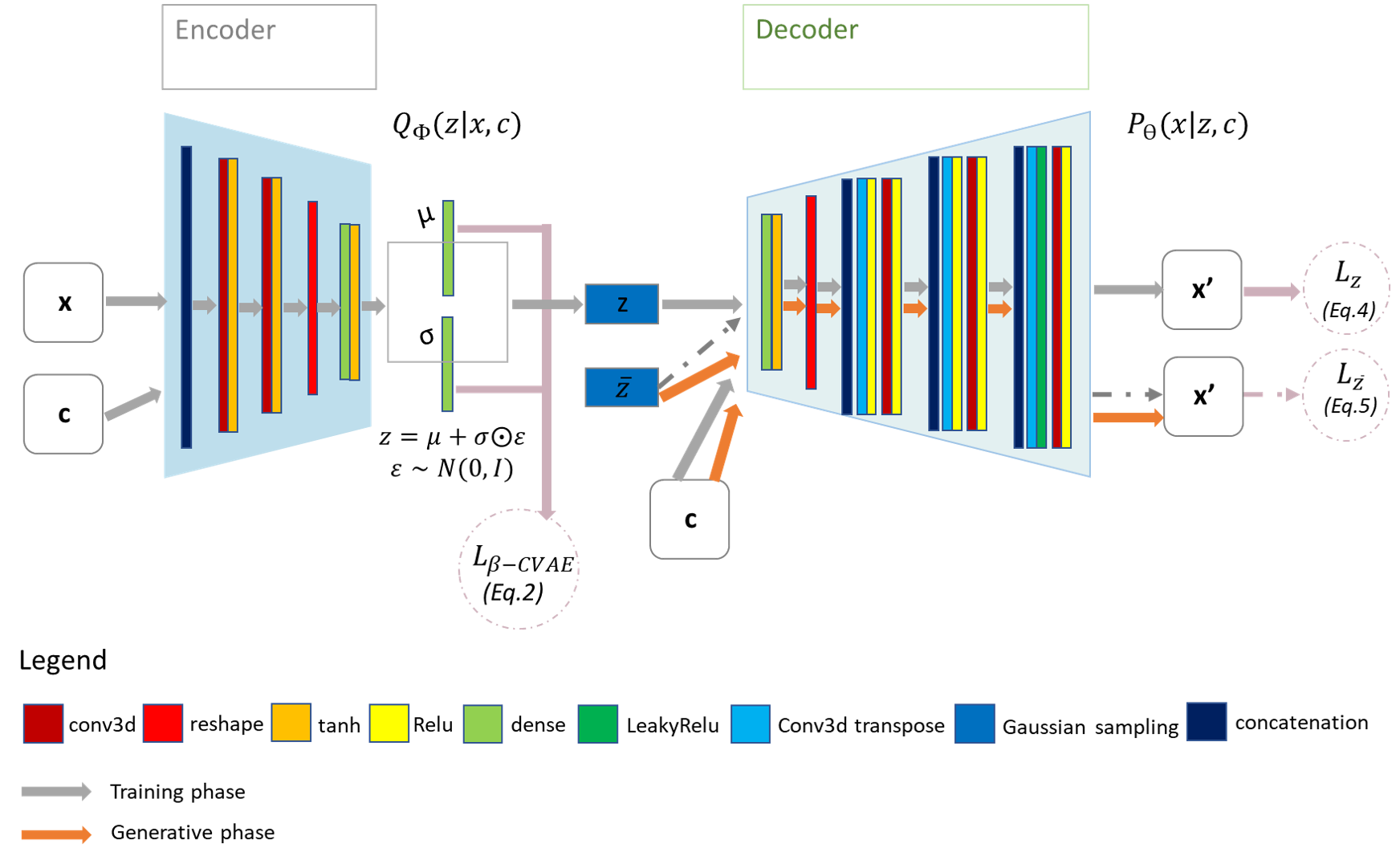
7.4.2 Discovering the link between cardiovascular pathologies and neurodegeneration through biophysical and statistical models of cardiac and brain images
This project is funded by Université Côte d'Azur (UCA)
Keywords: Lumped models - Biophysical simulation - Statistical learning.
Participants: Jaume Banus Cobo [Correspondent], Maxime Sermesant [Pompeu Fabra University, Barcelona, Spain], Marco Lorenzi [Pompeu Fabra University, Barcelona, Spain], Oscar Camara Rey [Pompeu Fabra University, Barcelona, Spain].
The project aims at developing a computational model of the relationship between cardiac function and brain damage from large-scale clinical databases of multimodal and multi-organ medical images (Figure 21) 57. The model is based on advanced statistical learning tools for discovering relevant imaging features related to cardiac dysfunction and brain damage 11. However, the limited cardiac data available in datasets focused on neurodegenerative diseases hinders the study of the role of cardiac function in these diseases. Hence, we developed a joint imputation framework based on variational inference and Gaussian process (GP) regression. The GP emulates the cardiac dynamics of a mechanistic model and constraints the data imputation conditioned on the available brain information.
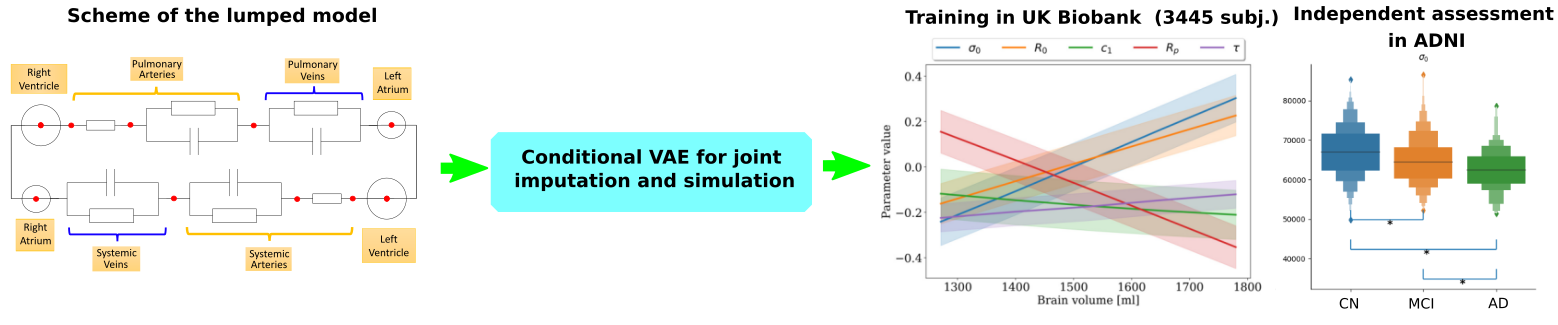
7.4.3 Automated-spectral smartphone for eye fundus diagnosis
This project has received funding from the European Union's Horizon 2020 research and innovation programme under Marie Sklodowska-Curie grant agreement No. 801342 (Tecniospring INDUSTRY) and the Government of Catalonia's Agency for Business Competitiveness (ACCIÓ).
Keywords:
Participants: Francisco Javier Burgos-Fernández [Correspondent], Buntheng Ly, Fernando Díaz-Doutón, Meritxell Vilaseca, Jaume Pujol, Maxime Sermesant.
We modified the conditional variational autoencoder developed by Ly et al. 43 for the automatic classification of eye fundus through multispectral images acquired in the visible and near-infrared range of the electromagnetic spectrum (400 nm - 1300 nm) from healthy and diseased eye fundus (Figure 22). The model 47 led to a classification accuracy of 96.43%, a loss of 0.20, a sensitivity of 92.86% and a specificity of 100.00% when discriminating between healthy and diseased fundus of the test dataset.
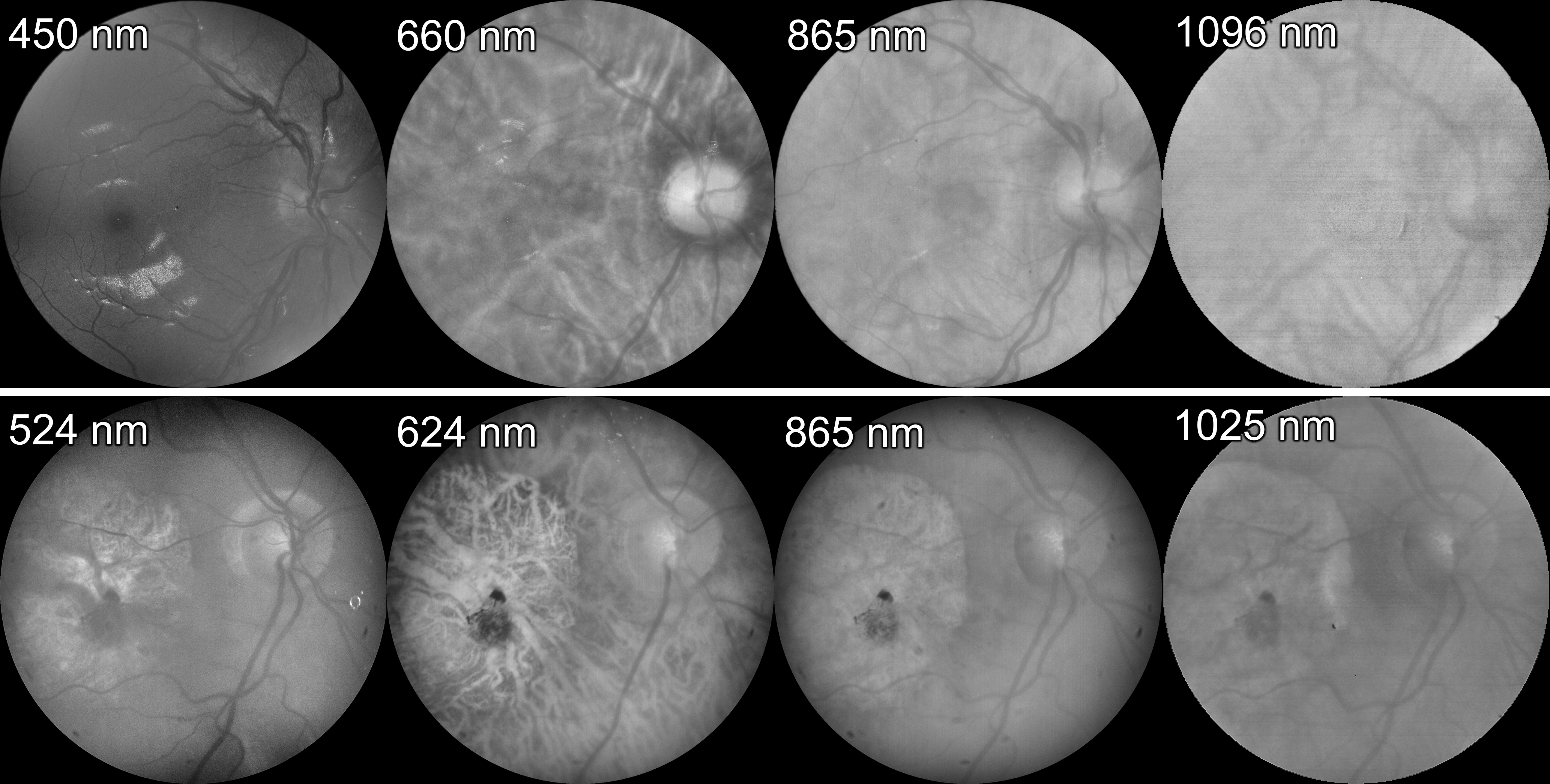
7.4.4 Re-entrant Ventricular Tachycardia Simulation from Non-invasive Data
This work is funded by the IHU Liryc, Bordeaux.
Keywords: computed tomography, cardiac electrophysiology, image-based modelling, ventricular tachycardia.
Participants: Nicolas Cedilnik [Correspondent], Pierre Jaïs [CHU Bordeaux], Frédéric Sacher [CHU Bordeaux], Hubert Cochet [IHU Liryc, Bordeaux, France], Maxime Sermesant.
This project aims at improving ventricular tachycardia ablation procedures planning with in silico electro-anatomical exploration of the arrhythmogenic substrate using cadiac imaging as a support for model personalisation.
- We conducted a large scale study of the relationship between imaging and electrophysiological features using in-vivo human data (Figure 23).
- We used our findings to parametrise a reaction diffusion model of activation wave propagation.
- We showed that this personalisation framework was able to reproduce re-entrant patterns that match intra cardiac.
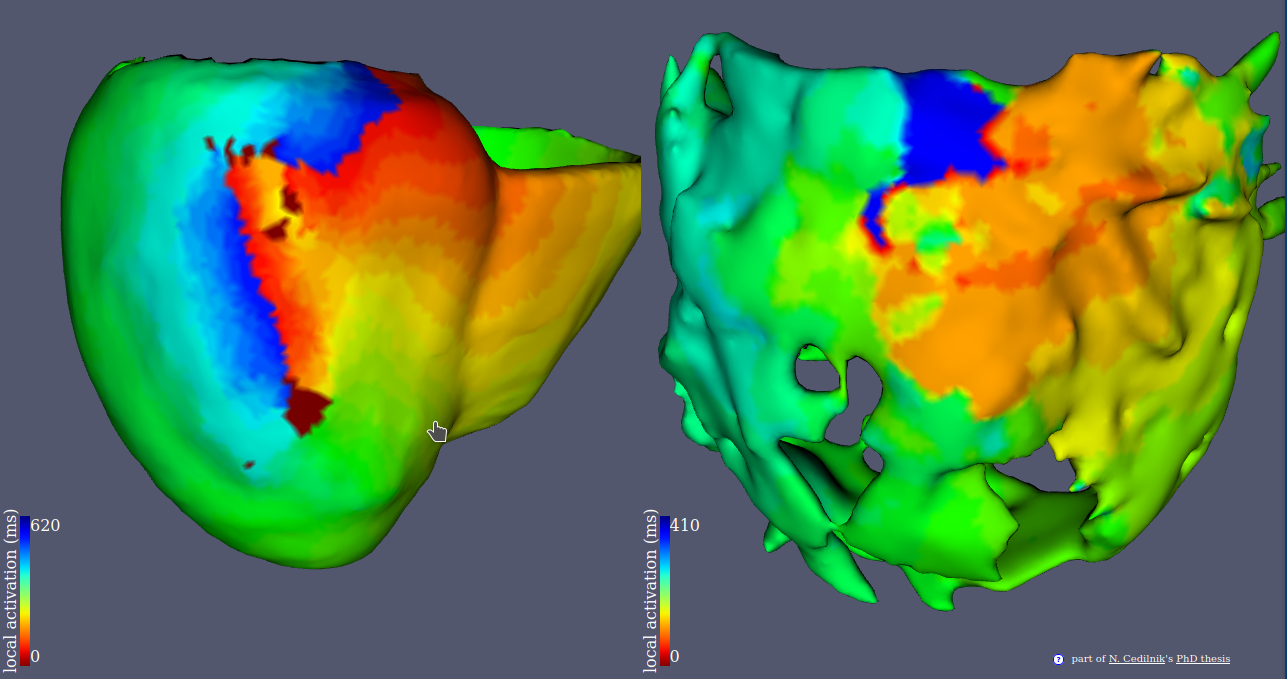
7.4.5 3D electromechanical cardiac modelling for heart failure patients stratification and prediction of cardiac resynchronisation therapy response
This work has been supported by the French government through the National Research Agency (ANR) Investments in the Future 3IA Côte d'Azur (ANR-19-P3IA-000) and by Microport CRM funding.
Keywords:
Participants: Gaëtan Desrues [Correspondent], Serge Cazeau, Thierry Legay, Delphine Feuerstein, Maxime Sermesant.
Patient-specific 3D electromechanical models can help in improving patient selection, therapy optimisation and interventional guidance. The aim of this project is to study cardiac desynchronisation and the resynchronisation therapy, based on personalised models of the heart 35.
- We first build an atlas of deformed heart geometries, including hypertrophic and dilated cardiomyophaties. A regularized linear regression is used to generate a geometry based on patient data (Figure 24 (a)).
- After the heterogeneous meshing and His-Purkinje network generation, parameters of the electrical propagation model (such as tissue conductivities) are optimized so that the simulated 12-lead ECG matches the patient's one (Figure 24 (b)).
- Finally, the parameters of the mechanical model (such as stiffness or contractility) are optimized using the CMA-ES algorithm. The generated contraction for a left bundle branch bloc is presented (Figure 24 (c)).
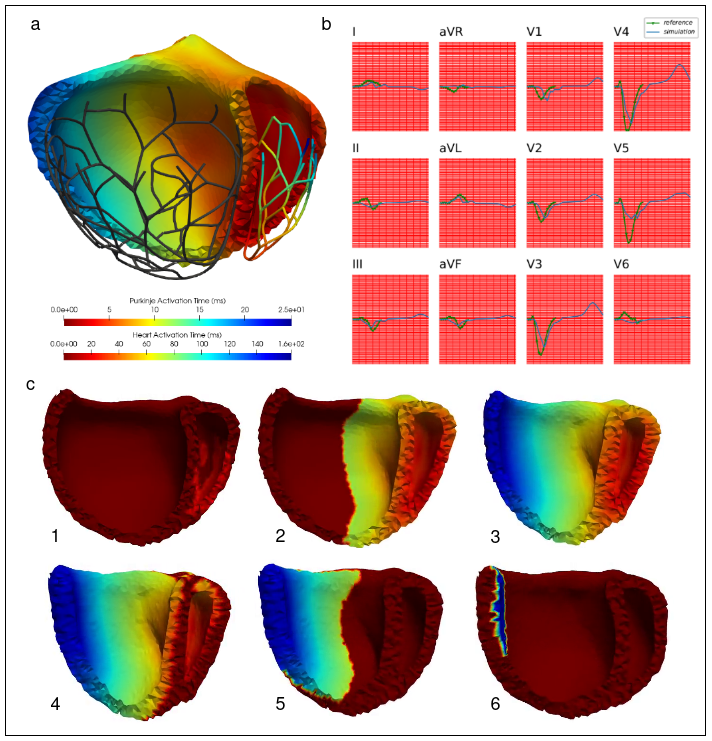
7.4.6 Predicting thrombosis from Left-Atrium CT-Scan
This work is funded by PARIS EU Project, in collaboration with Simula, Oslo, Norway and Hambourg University Hospital, Germany. Also in collaboration with the IHU Liryc of Bordeaux and the Pompeu Fabra University in Barcelona
Keywords:
Participants: Josquin Harrison [Inria, Université Côte d'Azur, Epione team, Sophia Antipolis, France], Hubert Cochet [IHU Liryc, Bordeaux, France], Marco Lorenzi [Inria, Université Côte d'Azur, Epione team, Sophia Antipolis, France], Oscar Camara Rey [Pompeu Fabra University, Barcelona, Spain], Xavier Pennec [Inria, Université Côte d'Azur, Epione team, Sophia Antipolis, France], Maxime Sermesant [Inria, Université Côte d'Azur, Epione team, Sophia Antipolis, France].
The objective of this project is to find image-based biomarkers in order to predict the risk of thrombus-related stroke for atrial fibrillation patients. 3D shapes of the left atrium are analysed, in combination with detailed blood flow simulations. A retrospective database of 300 patients from Bordeaux University Hospital is currently analysed. A focus on the orientations of pulmonary veins lead to a graph representation of the left atrium and a novel latent variable model 40. The complete pipeline is shown in Figure 25
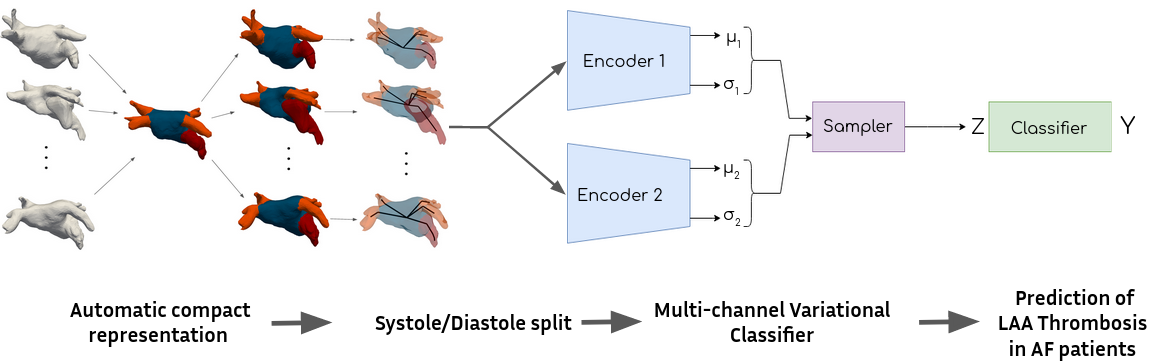
7.4.7 EP-Net 2.0: Learning Cardiac Electrophysiology Dynamics with DL
PhD funding from 3IA Côte d'Azur
Keywords:
Participants: Victoriya Kashtanova [Correspondent], Nicolas Cedilnik [LIP6, Paris], Maxime Sermesant [LIP6, Paris], Ibrahim Ayed [LIP6, Paris], Patrick Gallinari [LIP6, Paris].
We propose a deep learning approach (EP-Net 2.0) to learn the cardiac electrophysiology dynamics from data in the presence of scars in the cardiac tissue slab. We show experimentally in 42 that this model is able to reproduce the transmembrane potential dynamics in situations close to the training context and is able to generalize to new conditions including more complex scar geometries, multiple signal onsets and various conduction velocities. Figure 26 presents the EP-Net 2.0 general experimental setting.
The testing results and trained model are publicly available on Github github.com/KVict-new/EP-Net-2.0.
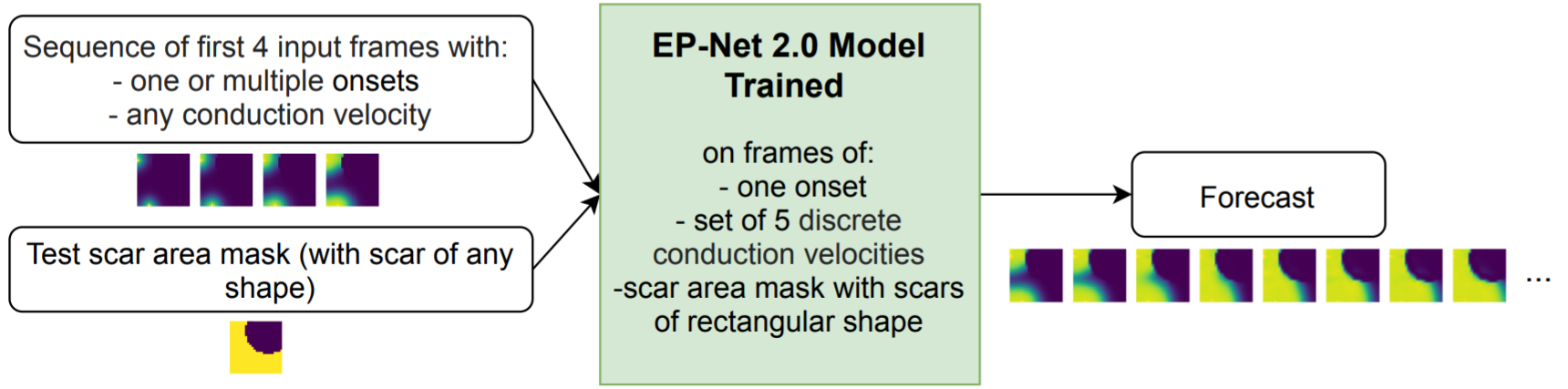
7.4.8 Scar-related Ventricular Arrhythmia Prediction using Explainable Deep Learning
Part of this work has been supported by the French Government, through the National Research Agency (ANR) 3IA Côte d'Azur (ANR-19-P3IA-0002), IHU Liryc (ANR- 10-IAHU-04), Université Côte d'Azur and Ecole Doctorale Sciences et Technologies de l'Information et de la Communication (EDSTIC).
Keywords:
Participants: Buntheng Ly [Correspondent], Sonny Finsterbach, Marta Nuñez-Garcia, Hubert Cochet, Maxime Sermesant.
We proposed an automatic pipeline for ventricular arrhythmia (VA) prediction using the cardiac CT imaging 43. Our theory was based on the relation of the left ventricular (LV) wall thinning in the CT imaging and the myocardium scar, the substrate of the VA mechanism. The complete pipeline is summarised in Figure 27, where the 2D LV thickness map was extracted and used as input to our prediction model. Our model outperformed the LV ejection fraction, the current gold standard predictor of VA.
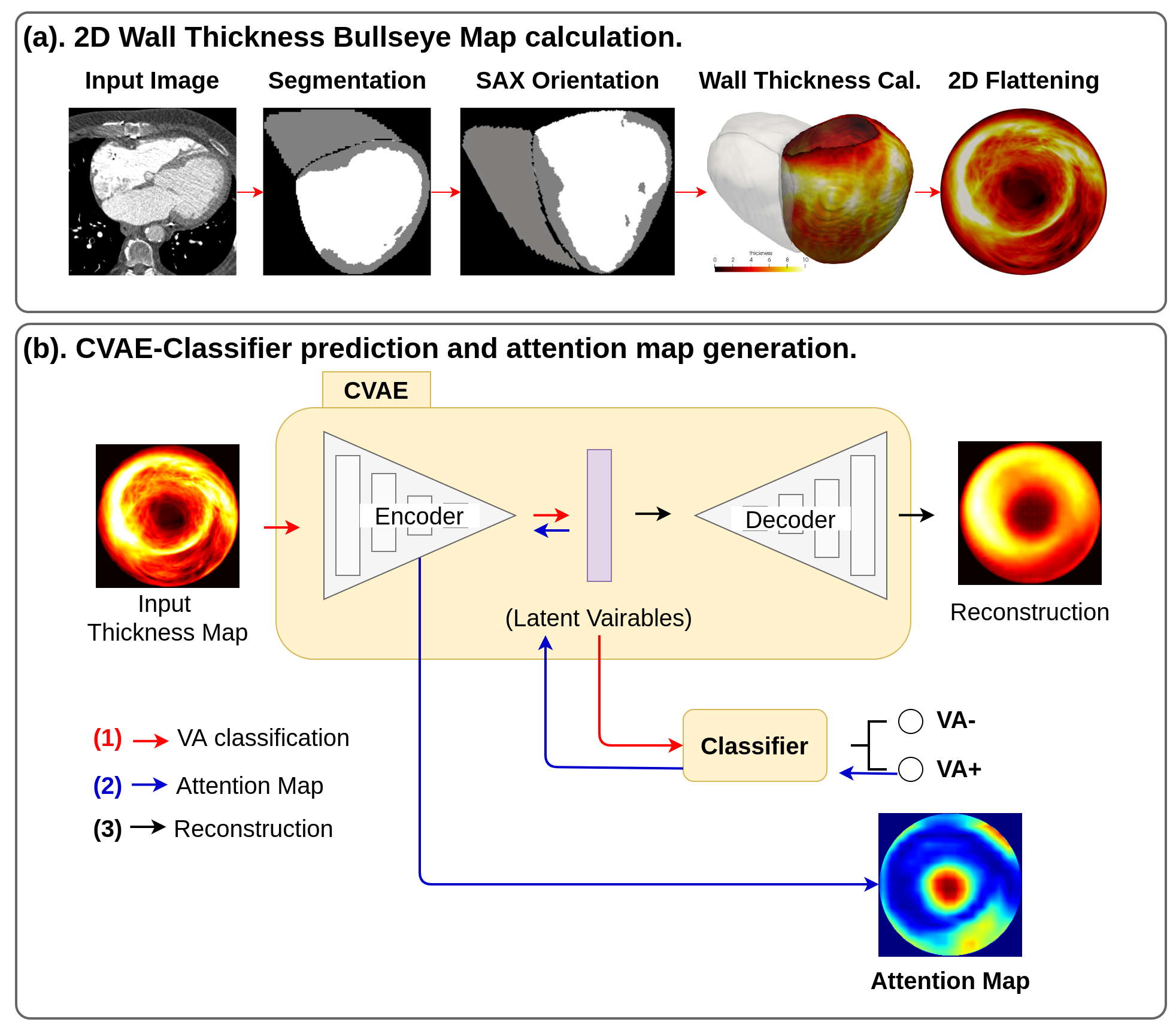
7.4.9 Personalisation of 3D cardiac electromechanical models
European Union's Horizon 2020 research and innovation programme under grant agreement No 101016496 (SimCardioTest)
Keywords:
Participants: Jairo Rodriguez-Padilla [Correspondent], Maxime Sermesant.
Personalised patient-specific electromechanical models of the heart can be a huge asset for clinicians to improve therapy selection in multiple cardiac diseases. However, personalisation of 3D models is still challenging due to the complexity of the system itself, the high number of parameters to be tuned and computational demand. In this work we:
- Use the Bestel-Clément-Sorine model to compute electromechanical activity in the heart (Figure 28, panel (A)),
- personalise the model via Covariance Matrix Adaptation Evolution Strategy (CMA-ES),
- perform a further sensitivity analysis on the impact that APD increase has in mechanical output parameters used in cardiac resyinchronisation therapy (CRT) (Figure 28, panels B, C and D).
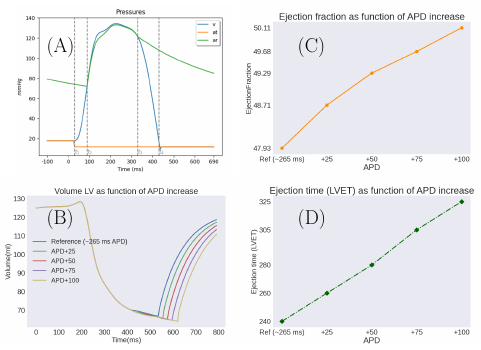
7.4.10 Generalisable segmentation of 2D echocardiography
ANR-19-P3IA-0002
Keywords:
Participants: Yingyu Yang [Correspondent], Maxime Sermesant.
Ultrasound images usually suffer from low ratio of signal-to-noise, boundary ambiguity and out-of-view motion. Automatic algorithms of 2D echocardiography analysis ca nhelp improve diagnostic efficiency as well as improve the accessibility of ultrasound in less developped areas. In this work 51 we:
- proposed methods of introducing shape priors from global, regional and pixel levels for echocardiography segmentation (Figure 29)
- empirically demonstrated results of different shape priors strategies and introducing shape prior through a contour-aware loss in pixel level manifested the best result
- presented the generalisation result of proposed methods in a large unseen dataset
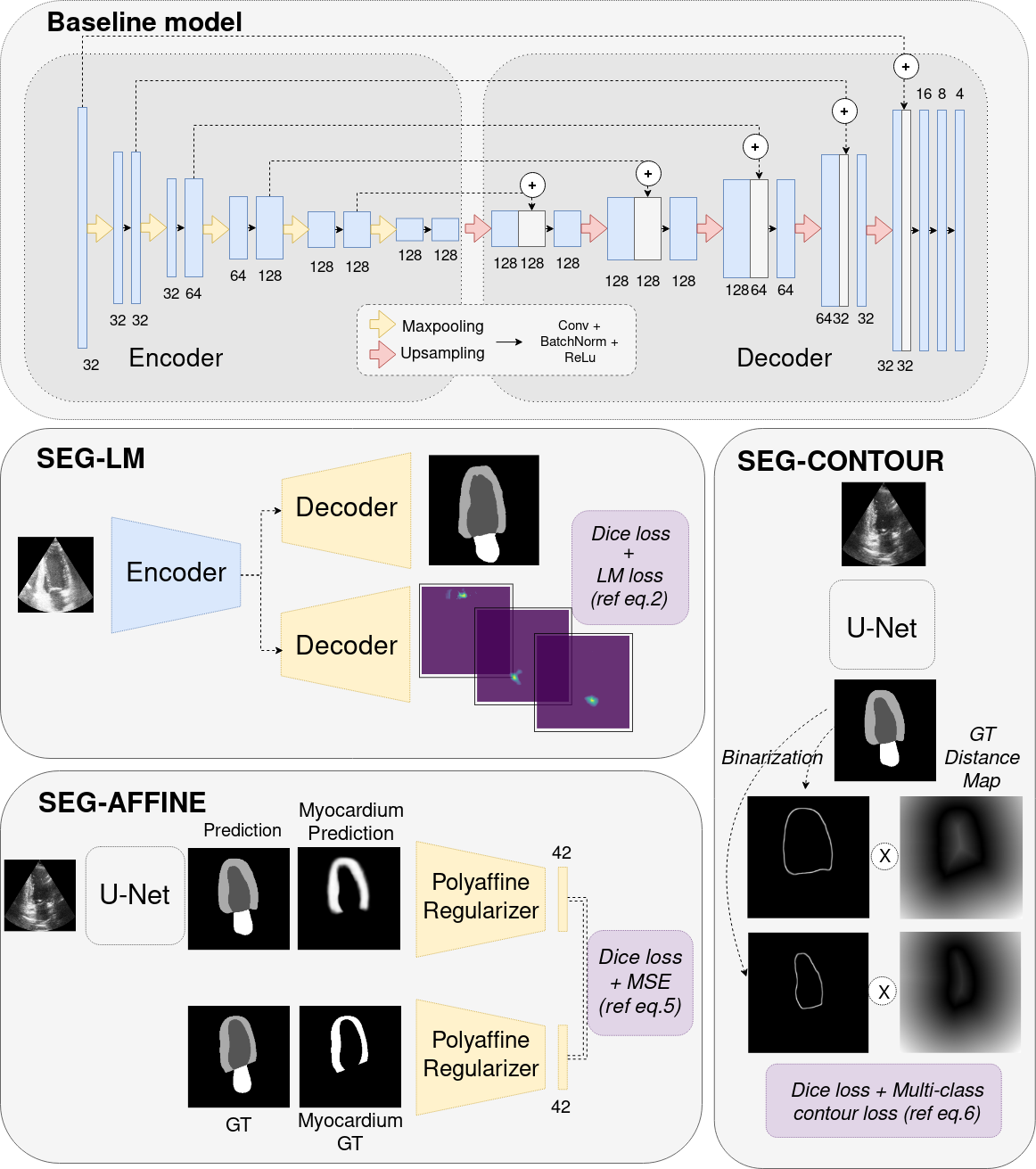
7.5 Multi-centric data and Federated Learning
7.5.1 Differentially private Bayesian federated learning framework for heterogeneous multi-view data assimilation
This project received financial support by the French government through the Agence Nationale de la Recherche (ANR), ref. num. ANR-19-CE45-0006.
Keywords:
Participants: Irene Balelli [Correspondent], Santiago Silva, Marco Lorenzi.
In 10 we developed a novel Bayesian learning framework based on a hierarchical generative model (Figure 30 (a)) for heterogeneous multi-views data assimilation across federated datasets. The Bayesian approach used for parameter estimation allows to handle heterogeneous distribution of datasets across centers, and missing views, while providing an interpretable model of data variability and a tool for missing views imputation (Figure 30 (b)). We further investigate the coupling of our framework with differential privacy: theoretical privacy guarantees against data leakage are provided, and we show that parameter utility can be reasonably preserved (Figure 30 (c)).
- A novel Bayesian federated learning paradigm
- Hierarchical generative model for joint integration of multi-views heterogeneous decentralized data
- High quality data reconstruction even in the presence of missing views
- Formal privacy guarantees
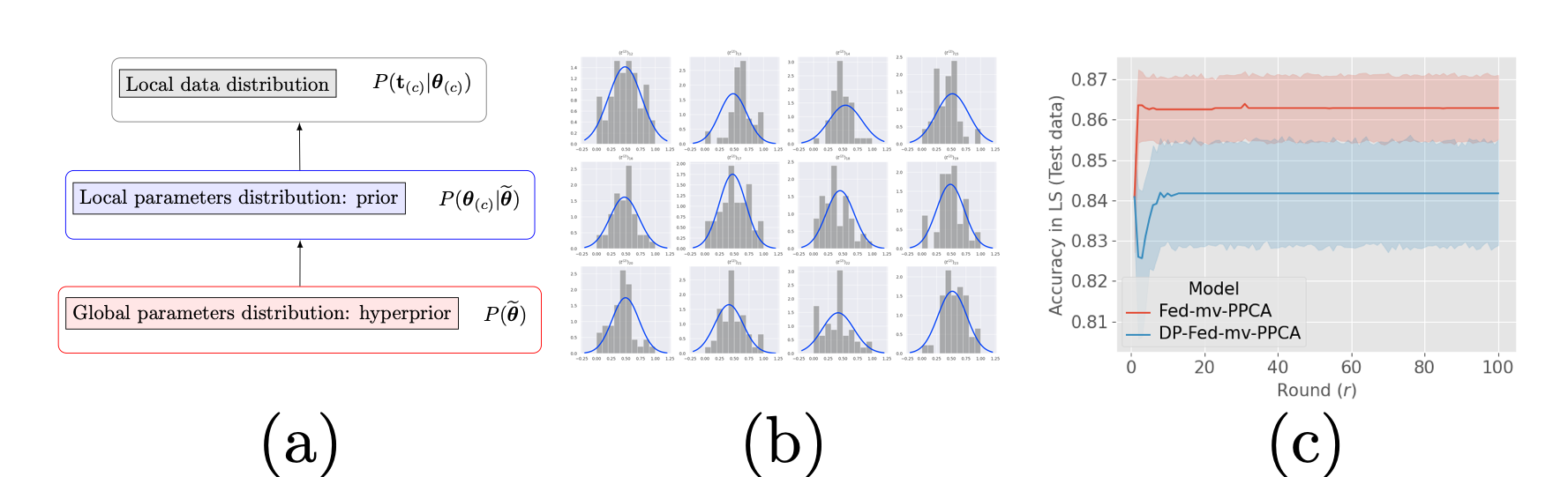
7.5.2 Bias in Federated Learning
Keywords:
Participants: Yann Fraboni [Correspondent], Marco Lorenzi.
Federated learning (FL) enables different clients to jointly learn a global model without sharing their data. Considering a subset of clients instead of all of them for optimization is called client sampling and leads to faster FL convergence. In 63, we theoretically prove the impact of a client sampling scheme on FL convergence speed. In 49, we introduce clustered sampling, a new client sampling strategy outperforming the state-of-the-art (Figure 31).
In 50, we also investigate the feasibility of free-rider attacks on FL. We propose two strategies for free-riders to successfully retrieve the learnt model without contributing to the learning process.
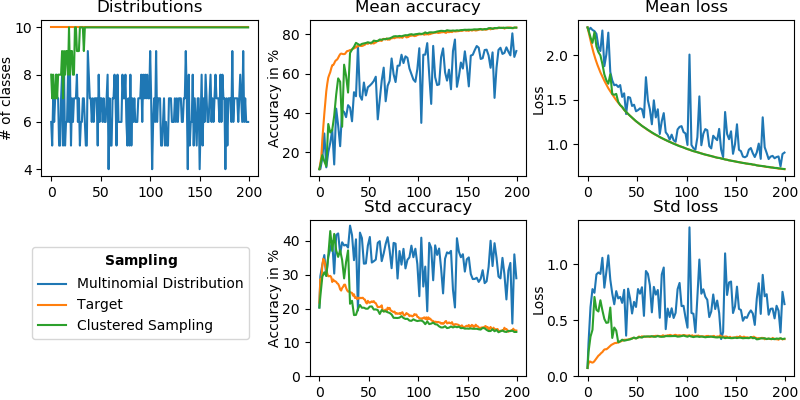
7.5.3 Federated mixed effects modeling
H2020 / Marie Sklodowska-Curie Actions COFUND number 847579
Keywords:
Participants: Santiago Silva [Correspondent], Marco Lorenzi.
Data harmonization is crucial to obtain generalized models in machine learning as well as data privacy and governance, important factors reflected in regulations such as the CCPA/GDPR. We propose to address multi-centric data harmonization by estimating mixed-effects using stochastic variational inference, a method for uncertainty estimation that allows a direct extrapolation to federated learning for secure modeling on decentralized data (Figure 32). An extension of previous works was submitted to JMLR.
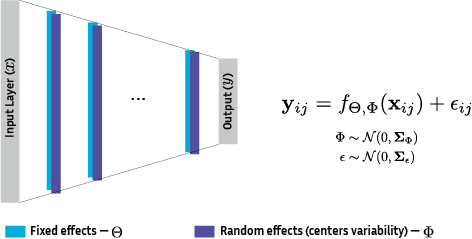
7.5.4 Data Structuration and Security in Large-Scale Collaborative Healthcare Data Analysis
This work has been supported by the French government, through the 3IA Côte d'Azur Investments
Keywords:
Participants: Riccardo Taiello [Correspondent], Marco Lorenzi [EURECOM], Melek Önen [EURECOM], Olivier Humbert.
Our work aims to study the impact of privacy-preserving methods in Federated Learning for biomedical applications, which an architecture example is shown in Figure 33 Due to the highly sensitive nature of the medical data being processed, we also plan to investigate the use or design of privacy-preserving primitives for dedicated operations performed with federated learning. We, therefore, intend to make use of advanced cryptographic tools such as homomorphic encryption (HE) or secure multi-party computation (MPC), which allow the processing of the data without the leakage of additional information.
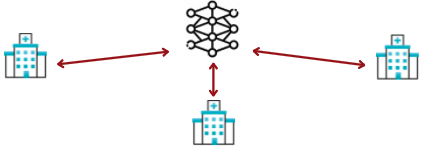
8 Bilateral contracts and grants with industry
8.1 Bilateral contracts with industry
8.1.1 Quantificare
Participants: Xavier Pennec, Hervé Delingette.
The company Quantificare is funding the PhD of Florent Jousse through a CIFRE grant, on the statistical analysis of shapes, deformations and appearance of anatomical surfaces for computer-aided dermatology and plastic surgery. The primary purpose is to model complex face deformations such as natural aging, facial expressions, surgical interventions and posture motions.
8.1.2 Oticon Medical
Participants: Zihao Wang, Hervé Delingette.
Oticon Medical, Vallauris, France, is co-funding the PhD work of Zihao Wang which aims at developing robust medical image algorithms for cochlea image segmentation.
8.1.3 Accenture Labs
Participants: Marco Lorenzi, Yann Fraboni.
Accenture Labs, Sophia Antipolis, France, is funding the CIFRE PhD work of Yann Fraboni, which aims at investigating the problem of bias and fairness in federated learning applications.8.1.4 Microport CRM
Participants: Maxime Sermesant, Gaetan Desrues.
Microport CRM is a cardiac impantable device company, and is funding the PhD work of Gaëtan Desrues, which aims at using cardiac electromechanical modelling for cardiac resynchronisation therapy planning.8.1.5 Spin-off company inHEART
Participants: Maxime Sermesant.
inHEART2 is a spin-off of the Epione team and IHU Liryc founded in 2017. inHEART provides a service to generate detailed anatomical and structural meshes from medical images, that can be used during ablation interventions. inHEART received 2 awards, one from Aquitaine region and one i-LAB from the BPI. It raised 3.2 million euros in 2020. It currently employs 19 people.
9 Partnerships and cooperations
9.1 International initiatives
9.1.1 Participation in other International Programs
Participants: Xavier Pennec, Marco Lorenzi, Hervé Delingette, Maxime Sermesant, Nicholas Ayache, Irene Balelli.
Department of Computer Science, University of Copenhagen, DK
Xavier Pennec is collaborating with Pr. Stefan Sommer on stochastic and sub-Riemannian approached to statistics, in the framework of his ERC G-statistics and of the jointly advised PhD thesis of Morten Akhoj Pedersen.
University College London (UCL), London, UK
Marco Lorenzi is collaborator of the COMputational Biology in Imaging and geNEtics (COMBINE) group within the Centre for Medical Image Computing (CMIC) of UCL, and with the UCL Institute of Ophtalmology. His collaboration is on the topic of spatio-temporal analysis of medical images, with special focus on brain imaging analysis and biomarker development. He is also collaborating with the “Progression Over Neurodegenerative Disorders” (POND) group (Prof. Daniel Alexander) for developing new computational models and techniques for learning characteristic patterns of disease progression using large longitudinal clinical data sets, with special focus on dementias.
Imaging Genetics Center (IGC), University of Southern California (USC), CA, USA and Illinois Institute of Technology (IIT, IL, USA)
Marco Lorenzi is currently collaborator of IGC and IIT for the investigation of the complex relationship between brain atrophy and genetics in Alzheimer's disease, in particular for demonstrating the effectiveness of multivariate statistical models in providing a meaningful description of the relationship between genotype and brain phenotype.
Laboratory of Neuroimaging of Aging (LANVIE), Faculty of Medicine, Geneva University Hospitals (HUG)
Marco Lorenzi collaborates with the LANVIE laboratory led by Prof. Giovanni B. Frisoni. The collaboration consists in developing and translating novel approaches for disease progression modeling in neurodegenerative disorders, such as Alzheimer's disease. St Thomas' Hospital, King's College London, United Kingdom
Laboratory of Physics of Fluids, University of Twente, NL
Herve Delingette is collaborating with Assistant Professor Guillaume Lajoinie, on the topics of Deep Learning for ultrasound imaging in the framework of the BoostUrCareer Cofund program and the thesis of Hari Sreedhar.
Other International Hospitals
Collaborations with several other European hospitals have been established through the European projects VP2HF, MD PAEDIGREE, SysAFib and with BarcelonaBeta research centre for Alzheimer.
9.2 International research visitors
Pr Sébastien Ourselin visited our team in Nov. 2021 in the context of his international Chair at the 3IA Côte d'Azur.
9.2.1 Visits of international scientists
Sarang Joshi
-
Status:
Professor
-
Institution of origin:
SCI Institute, Univ. Utah, Salt-lake City
-
Country:
USA
-
Dates:
15-11 to 20-11-2021
-
Context of the visit:
visit of the G-Statistics team.
-
Mobility program/type of mobility:
invitation.
9.3 European initiatives
9.3.1 FP7 & H2020 projects
ERC G-Statistics
-
Title:
Geometric Statistics
-
Type:
ERC
-
Program:
H2020
-
Duration:
2018-2023
-
Inria contact:
Xavier Pennec
-
Coordinator:
Inria
-
Summary:
G-Statistics aims at exploring the foundations of statistics on non-linear spaces with applications in the Life Siences. Invariance under gauge transformation groups provides the natural structure explaining the laws of physics. In life sciences, new mathematical tools are needed to estimate approximate invariance and establish general but approximate laws. Rephrasing Poincaré: a geometry cannot be more true than another, it may just be more convenient, and statisticians must find the most convenient one for their data. At the crossing of geometry and statistics, G-Statistics aims at grounding the mathematical foundations of geometric statistics and to exemplify their impact on selected applications in the life sciences.
So far, mainly Riemannian manifolds and negatively curved metric spaces have been studied. Other geometric structures like quotient spaces, stratified spaces or affine connection spaces naturally arise in applications. G-Statistics will explore ways to unify statistical estimation theories, explaining how the statistical estimations diverges from the Euclidean case in the presence of curvature, singularities, stratification. Beyond classical manifolds, particular emphasis will be put on flags of subspaces in manifolds as they appear to be natural mathematical object to encode hierarchically embedded approximation spaces.
In order to establish geometric statistics as an effective discipline, G-Statistics will propose new mathematical structures and characterizations of their properties. It will also implement novel generic algorithms and illustrate the impact of some of their efficient specializations on selected applications in life sciences. Surveying the manifolds of anatomical shapes and forecasting their evolution from databases of medical images is a key problem in computational anatomy requiring dimension reduction in non-linear spaces and Lie groups. By inventing radically new principled estimations methods, we aim at illustrating the power of the methodology and strengthening the “unreasonable effectiveness of mathematics” for life sciences.
ERC ECSTATIC
-
Title:
Electrostructural Tomography – Towards Multiparametric Imaging of Cardiac Electrical Disorders
-
Type:
ERC
-
Program:
H2020
-
Duration:
2017 - 2022
-
Inria contact:
Maxime Sermesant
-
Coordinator:
U. Bordeaux
-
Summary:
Cardiac electrical diseases are directly responsible for sudden cardiac death, heart failure and stroke. They result from a complex interplay between myocardial electrical activation and structural heterogeneity. Current diagnostic strategy based on separate electrocardiographic and imaging assessment is unable to grasp both these aspects. Improvements in personalized diagnostics are urgently needed as existing curative or preventive therapies (catheter ablation, multisite pacing, and implantable defibrillators) cannot be offered until patients are correctly recognized.
ECSTATIC aims at achieving a major advance in the way cardiac electrical diseases are characterized and thus diagnosed and treated, through the development of a novel non-invasive modality (Electrostructural Tomography), combining magnetic resonance imaging (MRI) and non-invasive cardiac mapping (NIM) technologies.
The approach will consist of: (1) hybridising NIM and MRI technologies to enable the joint acquisition of magnetic resonance images of the heart and torso and of a large array of body surface potentials within a single environment; (2) personalising the inverse problem of electrocardiography based on MRI characteristics within the heart and torso, to enable accurate reconstruction of cardiac electrophysiological maps from body surface potentials within the 3D cardiac tissue; and (3) developing a novel disease characterisation framework based on registered non-invasive imaging and electrophysiological data, and propose novel diagnostic and prognostic markers.
This project will dramatically impact the tailored management of cardiac electrical disorders, with applications for diagnosis, risk stratification/patient selection and guidance of pacing and catheter ablation therapies. It will bridge two medical fields (cardiac electrophysiology and imaging), thereby creating a new research area and a novel semiology with the potential to modify the existing classification of cardiac electrical diseases.
SimCardioTest
-
Title: Simulation of Cardiac Devices
Drugs for in-silico Testing and Certification -
Type:
Resarch and Innovation Action
-
Program:
H2020
-
Duration:
2021-2024
-
Inria contact:
Maxime Sermesant
-
Coordinator:
Inria
-
Summary:
Computer modelling and simulation have the power to increase speed and reduce costs in most product development pipelines. The EU-funded SimCardioTest project aims to implement computer modelling, simulation and artificial intelligence to design and test cardiac drugs and medical devices. Scientists will establish a platform for running in silico trials and obtaining scientific evidence based on controlled investigations. The simulation of disease conditions and cohort characteristics has the potential to overcome clinical trial limitations, such as under-representation of groups. It also reduces the size and duration of human clinical trials as well as animal testing, and offers robust, personalised information. Leveraging in silico technology in healthcare will expedite product and drug certification and offer patients the best possible care.
9.4 National initiatives
Consulting for Industry
- Nicholas Ayache has been a scientific consultant for the company Mauna Kea Technologies (Paris) until 31 Dec 2021. He joined the Scientific Advisory Board of Caranx Medical in Oct 2021.
- Marco Lorenzi was a scientific consultant for the company MyDataModels (Sophia Antipolis), and for the company Flexper (Sophia Antipolis).
- Maxime Sermesant is a scientific advisor for the company inHEART (Bordeaux).
Institute 3IA Côte d'Azur
-
The 3IA Côte d'Azur 3ia.univ-cotedazur.eu/ is one of the four "Interdisciplinary Institutes of Artificial Intelligence" that were created in France in 2019. Its ambition is to create an innovative ecosystem that is influential at the local, national and international levels, and a focal point of excellence for research, education and the world of AI.
-
Epione is heavily involved in this institute since its 5 permanents researchers (N. Ayache, H. Delingette, M. Lorenzi, M. Sermesant and X.Pennec) are chair holders in this institute, and N. Ayache serves as scientific director. H. Delingette and N. Ayache are members of its scientific committee.
Funded projects
- Hervé Delingette is among the main investigators of the DAICAP project (2020-2022, 300k€) selected by the Health Data Hub, the Grand Défi « Amélioration des diagnostics médicaux par l’Intelligence Artificielle », and Bpifrance in July 2020. That project aims to develop an algorithm able to produce a standardised MRI scan report to improve the early detection of prostate cancer.
- Marco Lorenzi is principal investigator of the ANR JCJC project Fed-BioMed (2020-2023, 196k€).
Collaboration with national hospitals
The Epione project team collaborates with the following 3 French IHU (University Hospital Institute): the IHU-Strasbourg (Pr J. Marescaux and L. Soler) on image-guided surgery, the IHU-Bordeaux (Pr M. Haïssaguere and Pr P. Jaïs) on cardiac imaging and modeling and the IHU-Pitié Salpétrière (Dr. O. Colliot and S. Durrleman) on neuroimaging.
The Epione project team is involved in the following research projects with the Assistance Publique des Hôpitaux de Paris (AP-HP) : NHANCE project on abdominal ultrasound image analysis with Dr Anne-Laure Rousseau (Hospital St-Louis), PAIMRI project on prostate cancer detection with Pr Raphaele Renard-Penna (Hospital La Pitié Salpêtrière), CLARITI project on PET-CT anomaly detection with Pr Florent Besson (Hospital Kremlin Bicêtre), PET-CT lesion detection with Dr Paul Blanc-Durand (Hospital Henri Mondor).
We also have long term collaborations with the CHU Nice and Centre Antoine Lacassagne in Nice.
9.5 Regional initiatives
- N. Ayache and P. Robert are principal investigators of the project MNC3 (Médecine Numérique, Cerveau, Cognition, Comportement) funded by Idex Jedi UCA (2017-2021, 450k€). M. Lorenzi (Inria) actively participates to the supervision of this project with the help of V. Manera (ICP).
- Hervé Delingette is the principal investigator of the LungMark project funded by Idex UCA JEDI (2018-2021).
- Hervé Delingette is the principal investigator of the CIMPLE project, funded by Idex UCA JEDI (2018-2021), the region PACA and Oticon Medical. The region PACA and Oticon Medical are co-funding the Phd of Zihao Wang.
- Marco Lorenzi and Hervé Delingette received funding for a PhD salary from UCA under the European Program BoostUrCareer (Marie Sklodowska-Curie agreement 847581).
- Maxime Sermesant is principal investigator of the project "The Digital Heart" and the innovation action "Digital Heart Phantom" with General Electrics, funded by Idex UCA JEDI. These projects gather the local cardiac research in academia, clinics and industry.
10 Dissemination
Participants: Nicholas Ayache, Irene Balelli, Hervé Delingette, Marco Lorenzi, Xavier Pennec, Maxime Sermesant.
10.1 Promoting scientific activities
10.1.1 Scientific events: organisation
General chair, scientific chair
- Marco Lorenzi was Area Chair of the Conferences “Conference on Computer Vision and Pattern Recognition” (CVPR 2021) and Medical Image Computing and Computer Assisted Intervention (MICCAI 2021).
- M. Sermesant was a co-chair of the MICCAI 2021 Workshop Statistical Atlases and Computational Models of the Heart (STACOM 2021), which was held virtually in September 2021.
Member of the organizing committees
- Nicholas Ayache was a member of the organizing committee of the 4th Conference on Medical Imaging in the era of AI, held at the Brain Institute in Paris in June 2021.
- Hervé Delingette is a member of the organizing committee of the 2021 "SophI.A. summit" a scientific event that was held virtually and face to face in Sophia Antipolis from Nov. 15th till Nov. 17th 2021.
- Marco Lorenzi is part of the organizing committee of the Winter School AI4Health, co-organised by the Health Data Hub, 3IA Côte d'Azur, PRAIRIE (Paris), and MIAI (Grenoble).
- Marco Lorenzi is organiser of the Special Session “Security and Fairness in Collaborative Healthcare Data Analysis”, presented at the International Symposium of Biomedical Imaging (ISBI) 2021.
- Marco Lorenzi is co-organiser of the Tutorial “Neurological Disease Progression Modelling”, presented at the International Symposium of Biomedical Imaging (ISBI) 2021.
- Marco Lorenzi is co-organiser of the Tutorial “Disease progression modeling with cross-sectional and longitudinal data”, presented at the International Conference Medical Image Computing and Computer Assisted Intervention (MICCAI) 2021.
10.1.2 Scientific events: selection
Member of the conference program committees
- Hervé Delingette is a Program Committee member of the 2022 AAAI conference focusing on various aspects of artificial intelligence.
- X. Pennec was a member of the program committee of the Geometric Science of Information (GSI 2021) and the ICPR W. on Manifold Learning from Euclid to Riemann (Manlearn, Milan, January 2021).
Reviewer
- H. Delingette was a reviewer for the Conference on Computer Vision and Pattern Recognition (CVPR'21), the International Conference on Learning Representations (ICLR 2022), the International Symposium on Biomedical Imaging (ISBI'21).
- M. Lorenzi was a reviewer for the conferences Neural Information Processing Systems (NeurIPS 2021), International Conference on Machine Learning (ICML 2021), International Conferene on Artificial Intelligence and Statistics (AISTATS 2022), Medical Image Computing and Computer Aided Intervention (MICCAI 2021), International Conference on Learning Representations (ICLR 2021), Information Processing in Medical Imaging (IPMI 2021), International Joint Conference on Artificial Intelligence (IJCAI 2021), Alzheimer's Association International Conference (AAIC 2021)
- X. Pennec was reviewer for the Information Processing in Medical Imaging (IPMI 2021) Conference.
- M. Sermesant was a reviewer for Medical Image Computing and Computer Aided Intervention (MICCAI 2021), the MICCAI workshop STACOM and the Computing in Cardiology conference.
10.1.3 Journal
Member of the editorial boards
- N. Ayache is the co-founder and the Co-Editor in Chief with J. Duncan (Professor at Yale) of Medical Image Analysis journal. This scientific journal was created in 1996 and is published by Elsevier.
- N. Ayache is a member of the editorial board of the following journal: Journal of Computer Assisted Surgery (Wiley).
- H. Delingette is a member of the editorial board of the journal Medical Image Analysis (Elsevier).
- M. Lorenzi was member of the editorial board of the journal Medical Image Analysis (Elsevier), and of Scientific Reports (Nature Publishing Group); he is also member of the Board of Statisticians of the Journal of Alzheimer’s Disease (IOS Press).
- X. Pennec is a member of the editorial board of the journal Medical Image Analysis (MedIA, Elsevier), of the International Journal of Computer Vision (IJCV, Springer), and of the Journal of Mathematical Imaging and Vision (JMIV, Springer).
- I. Strobant is editorial coordinator for Medical Image Analysis, Elsevier (since october 2001).
Reviewer - reviewing activities
- H. Delingette was a reviewer for the following journals: Medical Image Analysis (Elsevier), IEEE Transactions on Pattern Analysis and Machine Intelligence, Pattern Recognition.
- M. Lorenzi was a reviewer for the following journals: Journal of Alzheimer's Disease, Medical Image Analysis, IEEE Transactions on Medical Imaging, NeuroImage, International Journal of Computer Vision, Scientific Reports.
- X. Pennec was a reviewer for the following journals: PLOS One, Bernoulli, SIAM Journal on Mathematics of Data Science (SIMODS), SIAM Journal on Matrix Analysis and Applications (SIMAX), Information Geometry, Medical Image Analysis.
- M. Sermesant was a reviewer for the following journals: Journal of Machine Learning Research, Journal of the American College of Cardiology, IEEE Transactions on Medical Imaging, IEEE Transactions on Biomedical Engineering, Medical Image Analysis and Computers in Biology and Medecine.
10.1.4 Invited talks
- Nicholas Ayache gave the following plenary invited talks:
- AI4Health - Winter School of HDH, January 4th, 2021 (visio);
- French-German International Symposium, May 10th, 2021 (visio);
- International Steven Hoogendijk Award Lecture, Rotterdam City Hall, October 1st, 2021;
- Sofcot Forum (Othopedic surgery) in Paris, November 9th, 2021;
- International 3IA Prairie Workshop in Paris, November 10th, 2021;
- Hervé Delingette gave the following invited talks:
- University of Twente (NL) colloquium on Image analysis, February 19th, 2021;
- 5th ACE Impact Workshop, April 29th, 2021;
- EuroBiomed colloquium, on October 11th, 2021.
- Marco Lorenzi gave the following invited talks:
- keynote at the MICCAI Workshop on Distributed And Collaborative Learning 2021, October 1st, 2021;
- IEEE EMBS Public Forum on Data Science and Engineering in Healthcare, February 10th, 2021.
- X. Pennec gave the following invited talks:
- BIRS-CMO Workshop Geometry & Learning from Data, Casa Mathematica de Oaxaca, Mexico, October 24-29, 2021;
- Algebraic and Combinatorial Perspectives in Mathematical Sciences (ACPMS) European Seminar, Mai 28th, 2021;
- Manifold Learning from Euclid to Riemann, Workshop in conjunction with the 25th ICPR, Milan, Italy, January 11th, 2021.
10.1.5 Scientific expertise
- N. Ayache is a member of the following scientific committees:
- 2021- Scientific Committee of the French Health Data Hub;
- 2016 -: Scientific advisory committee for Région Ile de France (20 members);
- 2010 -: Scientific Advisory Boards in London (ICL,KCL).
- H. Delingette is a member of the scientific committee of the institute 3IA Côte d'Azur and was reviewer of the funding agency ANR (Agence Nationale de la Recherche, France), a reviewer of the PEPR Santé Numérique for the UDICE consortium. He was the lead participant in the French-canadian round table on AI for health care in March 25th.
- M. Lorenzi was reviewer of the funding agency ANR (Agence Nationale de la Recherche, France), and of the Fondation France Alzheimer (France).
- X. Pennec was a panel member for the Collaborative Research in Computational Neuroscience (CRCN) (joint call from NSF (US), NIH (US), ANR (FR), BMBF (DE), BSF (US-IL), NICT (JP)) and of the Inria International Chair committee.
10.1.6 Research administration
- Nicholas Ayache is the scientific director of the 3IA Côte d'Azur since its creation in April 2019.
- Hervé Delingette is a representive of Inria at the Federation Hospitalo-Universitaire Oncoage led by the CHU Nice. He was nominated as one of the 3 scientific directors of the IDEX program UCA JEDI under the direction of the president of the Université Côte d'Azur. He is an administrator of the Groupement de Coopération Sanitaires (GCS) CARES involving the Université Côte d'Azur and the 3 local hospitals (CHU Nice, Centre Antoine Lacassagne, Fondation Lenval).
- Hervé Delingette is a member of the committee of coordination between Université Côte d'Azur and Inria which supervises the strategic agreement between the two entities.
- Marco Lorenzi is a member of the local steering committee of the technological platforms (Comités Scientifiques de Pilotage des Plateformes) in charge of Cluster, Grid, Cloud, and HPC technologies. He is supervisor representative of the BoostUrCAreer Doctoral Program of Université Côte d'Azur.
- Marco Lorenzi is part of the working group of the PEPR Santé Numerique (axis “Secure, safe, and fair machine learning for healthcare” and “MultiScale AI for SingleCell-Based Precision Medicine”).
- Xavier Pennec is co-director of the Ecole doctorale STIC of Université Côte d'Azur. He is a member of the committee of EDSTIC, of the Doctoral follow-up Committee (CSD) at Inria Sophia Antipolis, and participated to the PhD fellowship granting committees of EDSTIC, Cofund at UCA, CORDI at Inria. He is a member of the "Comité de la Recherche Biomédicale en Santé Publique (CRBSP)" of the Nice University Hospital (CHU). At University Côte d'Azur /UCA JEDI, he is a member of the executive committee of the Academy 4 (Living systems Complexity and diversity), of the Scientific committee of the Academy 2 (Complex Systems), and of the Advanced Research Program Committee. At the national level, X. Pennec is a member of the Evaluation Committee of Inria. As such, he participated in 2021 to the promotion commitees for CRHC, DR1, DR0, to the PEDR committe and to the CRCN recruitment committee at Inria Bordeaux.
- Maxime Sermesant is an elected member of the Inria Sophia Antipolis research centre committee.
10.2 Teaching - Supervision - Juries
10.2.1 Teaching
- Master: H. Delingette and X. Pennec, Introduction to Medical Image Analysis, 21h course (28.5 ETD), Master 2 MVA, ENS Saclay, France.
- Master: M. Lorenzi and I. Balelli, Bayesian Learning, 30h course, Master Data Science, Univ. Côte d'Azur, France.
- Master : H. Delingette, 1h, Challenges in AI, University Diploma for AI in Healthcare, Univ. Côte d'Azur, France.
- Master: M. Lorenzi, Model Selection and Resampling Methods, 30h course, Master Data Science, Univ. Côte d'Azur, France.
- Master: I. Balelli, Modeling of biological systems, 10h ETD, Master 2 BIM, Univ. Côte d'Azur, France.
- M. Lorenzi and I. Balelli presented a one-day hands-on session on the software Fed-BioMed to the Winter School AI4Health (HDH and 3IA), and to the Inria DFKI Summer School.
10.2.2 Supervision: defended PhDs
- Clément Abi Nader, Modelling and simulating the progression of Alzheimer's disease through the analysis of multi-modal neuroimages and clinical data, Université Côte d'Azur. Defended on June 15th, 2021. Directed by Nicholas Ayache and Philippe Robert, and co-supervised by Marco Lorenzi.
- Luigi Antelmi, Statistical learning on heterogeneous medical data with bayesian latent variable models : application to neuroimaging dementia studies, Université Côte d'Azur. Defended on July 7th, 2021. Directed by Nicholas Ayache and Philippe Robert, and co-supervised by Marco Lorenzi.
- Benoit Audelan, Probabilistic segmentation modelling and deep learning-based lung cancer screening, Université Côte d'Azur. Defended on July 22nd, 2021. Directed by Hervé Delingette. Received second prize from the doctoral school EDSTIC.
- Tania-Marina Bacoyannis, Cardiac Imaging and Machine Learning for Electrostructural Tomography, Université Côte d'Azur. Defended on December 17th, 2021. Co-directed by Maxime Sermesant and Hubert Cochet (IHU Liryc).
- Jaume Banus Cobo, Linking cardiovascular pathologies and neurodegeneration with a combined biophysical and statistical methodology, Université Côte d'Azur. Defended on May 26th, 2021. Directed by Maxime Sermesant and co-supervised by Marco Lorenzi.
- Nicolas Guigui, Computational methods for statistical estimation on Riemannian manifolds and application to cardiac deformations, Université Côte d'Azur. Defended on November 18, 2021. Directed by Xavier Pennec 58.
- Zihao Wang, Deep generative learning for medical data processing, analysis and modeling: application to cochlea CT imaging, Université Côte d'Azur. Defended on Sept. 17th, 2021. Directed by Hervé Delingette.
10.2.3 Supervision: ongoing PhDs
- Paul Blanc-Durand, Deep neural networks in Nuclear Medicine, Université Paris Descartes, co-supervised by Hervé Delingette
- Mikael Chelli, AI for Othopedic Surgery, Centre hospitalier universitaire de Nice. Started in August 2019. Co-directed by Nicholas Ayache, Hervé Delingette and Jean Chaoui (Imascap).
- Hind Dadoun, AI-Based Real Time Diagnosis of Abdominal Ultrasound, Université Côte d'Azur. Started in December 2019. Co-directed by Nicholas Ayache and Hervé Delingette.
- Gaëtan Desrues, 3D electromechanical cardiac modelling for heart failure patients stratification and prediction of cardiac resynchronisation therapy response, Université Côte d'Azur. Started in 2020. Directed by Maxime Sermesant.
- Zhijie Fang, Robust Multimodal Non rigid image registration based on machine learning, Université Côte d'Azur. Started in 2020. Co-directed by Hervé Delingette and Nicholas Ayache.
- Yann Fraboni, Bias in Federated Learning, Université Côte d'Azur. Started in 2020. Directed by Marco Lorenzi.
- Dimitri Hamzaoui, AI-Based Diagnosis of Prostate Cancer from Multiparametric MRI, Université Côte d'Azur. Started in 2020. Co-directed by Nicholas Ayache and Hervé Delingette.
- Josquin Harrison, Medical Imaging and learning for the prediction of strokes in the case of atrial fibrillation, Université Côte d'Azur. Started in 2020. Directed by Maxime Sermesant.
- Etrit Haxholli, Exploring latent dynamical models for failure prediction in time-series of high-dimensional and heterogeneous data, Université Côte d'Azur. Started in 2020. Directed by Marco Lorenzi.
- Florent Jousse, Analyse statistique de forme, de déformations et d'apparences de surfaces anatomiques pour l'aide à la dermatologie et à la chirurgie plastique. Started in 2019. Cifre fellowship with Quantificare. Co-directed by Xavier Pennec and Hervé Delingette.
- Victoriya Kashtanova, Learning Cardiac 3D Electromechanical Dynamics with PDE-based Physiological Constraints for Data-Driven Personalized Predictions in Cardiology, Université Côte d'Azur. Started in 2020. Co-directed by Maxime Sermesant and Patrick Gallinari (Sorbonne University, LIP6, Paris).
- Huiyu Li, Anonymisation and protection of medical data based on deep neural networks. Université Côte d'Azur. Started in 2020. Co-directed by Hervé Delingette and Nicholas Ayache.
- Buntheng Ly, Cardiac Image Analysis for Sudden Cardiac Death Prediction, Université Côte d'Azur. Started in 2019. Co-directed by Maxime Sermesant and Hubert Cochet (IHU Liryc).
- Elodie Maignan, Geometric learning of manifolds, Université Côte d'Azur. Started in 2020. Co-directed by Xavier Pennec and Alain Trouvé (ENS Paris-Saclay).
- Morten Pedersen, Lie group action, approximate invariance and Sub-Riemannian geometry in statistics, Université Côte d'Azur and University of Copenhagen. Started in 2020. Co-directed by Xavier Pennec and Stefan Sommer (Univ Copenhagen, DK).
- Santiago Smith Silva Rincon, Federated learning of biomedical data in large-scale networks of multicentric imaging-genetics information, Université Côte d'Azur. Started in 2020. Co-directed by Marco Lorenzi and Barbara Bardoni (INSERM)
- Hari Sreedhar, Learning-based detection and classification of thyroid nodules from ultrasound images, Université Côte d'Azur. Started in 2020. Co-directed by Hervé Delingette and Charles Raffaelli (CHU-Nice).
- Riccardo Taiello, Data Structuration and Security in Large-Scale Collaborative Healthcare Data Analysis, Université Côte d'Azur. Started in 2021. Co-supervised by Melek Önen (EURECOM) and Olivier Humbert (Centre Antoine Lacassagne).
- Yann Thanwerdas, Statistical Dimension Reduction in Non-Linear Manifolds for Brain Shape Analysis, Connectomics & Brain-Computer Interfaces, Université Côte d'Azur. Started in 2019. Directed by Xavier Pennec.
- Paul Tourniaire, AI-based selection of imaging and biological markers predictive of therapy response in lung cancer, Université Côte d'Azur. Started in 2019. Co-directed by Nicholas Ayache and Hervé Delingette.
- Yingyu Yang, Artificial Intelligence for Automatic Cardiac Function Analysis: Multimodal and Biophysical Approach with Application to a Portable Imaging Device, Université Côte d'Azur. Started in 2020. Co-directed by Maxime Sermesant and Pamela Moceri (CHU-Nice)
10.2.4 Juries
- H. Delingette was PhD jury member for the thesis of Z. Wang (UCA, Thesis Director), of B. Audelan (UCA, Thesis Director), of Theo Estienne (Ecole Centrale Paris, reviewer). He was a member of the medicine thesis committee of Dr A. Berembaum (Faculté de médecine Paris) and Dr Karime Wu (Faculté de médecine Paris).
- M. Lorenzi was PhD jury Member for the thesis of Vikram Venkatraghavan (VUMC, NL), Arnaud Fernandez (IPMC, UCA), Julien Audibert (EURECOM), Radia Zeghari (Institut Claude Pompidou), Rosa Candela (EURECOM), Gia-Lac Tran (EURECOM).
- X. Pennec was reviewer for the HDR of Salem Said (Univ. Bordeaux), and member of PhD jury of Nicolas Guigui (Univ. Côte d'Azur).
10.3 Popularization
- Hervé Delingette participated to a TV program on Azur TV on Feb. 25th dedicated to "AI in healtcare".
- Hervé Delingette and Hind Dadoun participated to the "Fête de la science" by presenting the concept of AI in healthcare to high school students at the "Maison de l'IA" in Sophia Antipolis on Oct. 5th.
11 Scientific production
11.1 Major publications
- 1 miscClustered Sampling: Low-Variance and Improved Representativity for Clients Selection in Federated Learning.May 2021
- 2 articleNumerical Accuracy of Ladder Schemes for Parallel Transport on Manifolds.Foundations of Computational MathematicsJune 2021
- 3 articleLearning a Generative Motion Model from Image Sequences based on a Latent Motion Matrix.IEEE Transactions on Medical ImagingFebruary 2021
- 4 articleApplications of artificial intelligence in cardiovascular imaging.Nature Reviews CardiologyMarch 2021
- 5 articleBayesian Logistic Shape Model Inference: application to cochlear image segmentation.Medical Image AnalysisOctober 2021
11.2 Publications of the year
International journals
- 6 articleSimulating the outcome of amyloid treatments in Alzheimer's Disease from multi-modal imaging and clinical data.Brain CommunicationsFebruary 2021
- 7 articleSimulAD: A dynamical model for personalized simulation and disease staging in Alzheimer's disease.Neurobiology of Aging2022
- 8 articleRobust Bayesian fusion of continuous segmentation maps.Medical Image AnalysisMarch 2022
- 9 articleDeep Learning Formulation of ECGI Integrating Image & Signal Information with Data-driven Regularisation.EP-Europace23Supplement_1March 2021, i55-i62
- 10 articleA Probabilistic Framework for Modeling the Variability Across Federated Datasets of Heterogeneous Multi-View Observations.Information processing in medical imaging : proceedings of the ... conference.2021
- 11 articleBiophysics-based statistical learning: Application to heart and brain interactions.Medical Image Analysis72August 2021
- 12 articleFully automatic segmentation of diffuse large B cell lymphoma lesions on 3D FDG-PET/CT for total metabolic tumour volume prediction using a convolutional neural network.European Journal of Nuclear Medicine and Molecular Imaging2021, 1362-1370
- 13 articleRobust joint registration of multiple stains and MRI for multimodal 3D histology reconstruction: Application to the Allen human brain atlas.Medical Image AnalysisOctober 2021
- 14 articleDetection, Localization, and Characterization of Focal Liver Lesions in Abdominal US with Deep Learning.Radiology: Artificial Intelligence2022
- 15 articleDecoding genetic markers of multiple phenotypic layers through biologically constrained Genome-to-Phenome Bayesian Sparse Regression.Frontiers in Molecular Medicine2021
- 16 articleNiPTUNE: an automated pipeline for noninvasive prenatal testing in an accurate, integrative and flexible framework.Briefings in BioinformaticsSeptember 2021
- 17 articleDirection-dependent decomposition of 3D right ventricular motion: beware of approximations.Journal of The American Society of Echocardiography3422021, 201-203
- 18 articleInvestigating hypotheses of neurodegeneration by learning dynamical systems of protein propagation in the brain.NeuroImage235July 2021, 117980
- 19 articleNumerical Accuracy of Ladder Schemes for Parallel Transport on Manifolds.Foundations of Computational MathematicsJune 2021
- 20 articleProstate volume prediction on MRI: tools, accuracy and variability.European RadiologyFebruary 2022
- 21 articleAutomatic Zonal Segmentation of the Prostate from 2D and 3D T2-weighted MRI and Evaluation for Clinical Use.Journal of Medical Imaging2022
- 22 articleLearning a Generative Motion Model from Image Sequences based on a Latent Motion Matrix.IEEE Transactions on Medical ImagingFebruary 2021
- 23 articleCinE caRdiac magneTic resonAnce to predIct veNTricular arrhYthmia (CERTAINTY).Scientific Reports111November 2021
- 24 articleClassifying histograms of medical data using information geometry of beta distributions.IFAC-PapersOnLineJune 2021
- 25 articleAdditional prognostic value of echocardiographic follow-up in pulmonary hypertension - role of 3D right ventricular area strain.European Heart Journal - Cardiovascular Imaging2021
- 26 articleValue of 3D right ventricular function over 2D assessment in acute pulmonary embolism.Echocardiography2021
- 27 articleChallenge of prostate MRI segmentation on T2-weighted images: inter-observer variability and impact of prostate morphology.Insights into Imaging121June 2021
- 28 articleAssociation of Immunosuppression and Viral Load With Subcortical Brain Volume in an International Sample of People Living With HIV.JAMA Network Open41January 2021, e2031190
- 29 articleApplications of artificial intelligence in cardiovascular imaging.Nature Reviews CardiologyMarch 2021
- 30 articleBayesian Logistic Shape Model Inference: application to cochlear image segmentation.Medical Image Analysis75October 2021, 102268
- 31 articleInner-ear Augmented Metal Artifact Reduction with Simulation-based 3D Generative Adversarial Networks.Computerized Medical Imaging and Graphics93October 2021, 101990
- 32 articleJitter Aware Economic PDN Optimization with a Genetic Algorithm.IEEE Transactions on Microwave Theory and TechniquesJune 2021
- 33 articleDeep reinforcement learning in medical imaging: A literature review.Medical Image Analysis73October 2021, 102193
International peer-reviewed conferences
- 34 inproceedingsCombining Bayesian and Deep Learning Methods for the Delineation of the Fan in Ultrasound Images.ISBI 2021 - 18th IEEE International Symposium on Biomedical ImagingNice, FranceApril 2021
- 35 inproceedingsPersonal-by-design: a 3D Electromechanical Model of the Heart Tailored for Personalisation.FIMH 2021 - 11th International Conference on Functional Imaging and Modeling of the HeartStanford, CA, United StatesJune 2021
- 36 inproceedingsInvestigation of the impact of normalization on the study of interactions between myocardial shape and deformation.FIMH 2021 - 11th International Conference on Functional Imaging and Modeling of the HeartStanford, United StatesJune 2021, In press
- 37 inproceedingsParallel Transport on Kendall Shape Spaces.GSI 2021 - 5th conference on Geometric Science of Information12829Lecture Notes in Computer ScienceParis, FranceSpringerJuly 2021, 103-110
- 38 inproceedingsCardiac Motion Modeling with Parallel Transport and Shape Splines.ISBI 2021 - 18th IEEE International Symposium on Biomedical ImagingIEEE 18th International Symposium on Biomedical Imaging (ISBI), 2021Nice, FranceIEEEApril 2021, pp. 1394-1397
- 39 inproceedingsA reduced parallel transport equation on Lie Groups with a left-invariant metric.GSI 2021 - 5th conference on Geometric Science of Information12829Lecture Notes in Computer ScienceParis, FranceSpringer, ChamJuly 2021, 119-126
- 40 inproceedingsPhase-independent Latent Representation for Cardiac Shape Analysis.MICCAI 2021 - 24th International Conference on Medical Image Computing and Computer Assisted Intervention12906LNCS - Lecture Notes in Computer ScienceStrasbourg, FranceSeptember 2021
- 41 inproceedingsGeodesic squared exponential kernel for non-rigid shape registration.FG 2021 - IEEE International Conference on Automatic Face and Gesture RecognitionJODHPUR, IndiaDecember 2021
- 42 inproceedingsEP-Net 2.0: Out-of-Domain Generalisation for Deep Learning Models of Cardiac Electrophysiology.Functional Imaging and Modeling of the Heart; Functional Imaging and Modeling of the HeartFIMH 2021 - 11th International Conference on Functional Imaging and Modeling of the Heart12738Lecture Notes in Computer ScienceStanford, CA (virtual), United StatesSpringer International PublishingJune 2021, 482-492
- 43 inproceedingsScar-Related Ventricular Arrhythmia Prediction from Imaging Using Explainable Deep Learning.FIMH 2021 - 11th International Conference on Functional Imaging and Modeling of the Heart12738Lecture Notes in Computer ScienceStanford, United StatesSpringer International PublishingJune 2021, 461-470
- 44 inproceedingsGeodesics and Curvature of the Quotient-Affine Metrics on Full-Rank Correlation Matrices.GSI 2021 - 5th conference on Geometric Science of Information12829Proceedings of Geometric Science of InformationParis, FranceSpringer, ChamJuly 2021, 93-102
- 45 inproceedingsAttention-based Multiple Instance Learning with Mixed Supervision on the Camelyon16 Dataset.Proceedings of Machine Learning ResearchMICCAI Workshop on Computational Pathology156Proceedings of Machine Learning ResearchStrasbourg, FrancePMLRSeptember 2021, 216-226
- 46 inproceedingsOne-shot Learning Landmarks Detection.The MICCAI workshop on Data Augmentation, Labeling, and Imperfections13003Lecture Notes in Computer Science (LNCS)strasbourg, FranceSpringerSeptember 2021, 163-172
National peer-reviewed Conferences
- 47 inproceedingsAutomatic classification of multispectral eye fundus images using deep learning.Libro de Resúmenes RNO2021Online, SpainNovember 2021
Conferences without proceedings
- 48 inproceedingsValidation of lung nodule detection a year before diagnosis in NLST dataset based on a deep learning system.ERS 2021 - European Respiratory Society International CongressVirtual, United KingdomSeptember 2021
- 49 inproceedingsClustered Sampling: Low-Variance and Improved Representativity for Clients Selection in Federated Learning.International Conference on Machine Learningonline, FranceJuly 2021
- 50 inproceedingsFree-rider Attacks on Model Aggregation in Federated Learning.AISTATS 2021 - 24th International Conference on Artificial Intelligence and StatisticsSan Diego, United StatesApril 2021
- 51 inproceedingsShape Constraints in Deep Learning for Robust 2D Echocardiography Analysis.FIMH 2021 - 11th International Conference on Functional Imaging and Modeling of the HeartStanford, United StatesJune 2021
Scientific book chapters
- 52 inbookForeword.Digital Anatomy - Applications of Virtual, Mixed and Augmented RealityHuman–Computer Interaction SeriesSpringer NatureMay 2021
- 53 inbookStatistical analysis of organs' shapes and deformations: the Riemannian and the affine settings in computational anatomy.Digital Anatomy - Applications of Virtual, Mixed and Augmented RealityHuman–Computer Interaction SeriesSpringer NatureMay 2021
Doctoral dissertations and habilitation theses
- 54 thesisModelling and simulating the progression of Alzheimer's disease through the analysis of multi-modal neuroimages and clinical data.Université Côte d'AzurJune 2021
- 55 thesisStatistical learning on heterogeneous medical data with bayesian latent variable models : application to neuroimaging dementia studies.Université Côte d'AzurJuly 2021
- 56 thesisProbabilistic segmentation modelling and deep learning-based lung cancer screening.Université Côte d'AzurJuly 2021
- 57 thesisHeart & Brain. Linking cardiovascular pathologies and neurodegeneration with a combined biophysical and statistical methodology.Université Côte d'AzurMay 2021
- 58 thesisComputational methods for statistical estimation on Riemannian manifolds and application to the study of the cardiac deformations.Université Côte d'AzurNovember 2021
- 59 thesisDeep generative learning for medical data processing, analysis and modeling: application to cochlea ct imaging.Inria - Sophia Antipolis; Université côte d'azurSeptember 2021
Reports & preprints
- 60 miscCombining Multi-Task Learning and Multi-Channel Variational Auto-Encoders to Exploit Datasets with Missing Observations -Application to Multi-Modal Neuroimaging Studies in Dementia.May 2021
- 61 miscThe Measurement and Analysis of Shapes.February 2022
- 62 miscParameter estimation in nonlinear mixed effect models based on ordinary differential equations: An optimal control approach.September 2021
- 63 miscOn The Impact of Client Sampling on Federated Learning Convergence.December 2021
- 64 miscICLR 2021 Challenge for Computational Geometry & Topology: Design and Results.December 2021
- 65 miscSmaller limbic structures are associated with greater immunosuppression in over 1000 HIV-infected adults across five continents: Findings from the ENIGMA-HIV Working Group.January 2021
- 66 miscO(n)-invariant Riemannian metrics on SPD matrices.September 2021
- 67 miscThe geometry of mixed-Euclidean metrics on symmetric positive definite matrices.November 2021
- 68 miscTheoretically and computationally convenient geometries on full-rank correlation matrices.January 2022
- 69 miscAttention for Image Registration (AiR): an unsupervised Transformer approach.May 2021
- 70 miscQuasi-Symplectic Langevin Variational Autoencoder.June 2021
Other scientific publications
- 71 miscGraph-Valued Models for Dimensionality Reduction and Regression.Online, United StatesAugust 2022
11.3 Other
Patents
- 72 patentRisk prediction for sudden cardiac death from image derived cardiac motion and structure features.US20210059612A1United StatesMarch 2021
- 73 patentPatient specific risk prediction of cardiac events from image-derived cardiac function features.US20210057104A1United StatesFebruary 2021