Keywords
Computer Science and Digital Science
- A2.3.1. Embedded systems
- A3.4.1. Supervised learning
- A3.4.2. Unsupervised learning
- A3.4.3. Reinforcement learning
- A3.4.5. Bayesian methods
- A3.4.6. Neural networks
- A3.4.7. Kernel methods
- A3.4.8. Deep learning
- A5.4.1. Object recognition
- A5.4.5. Object tracking and motion analysis
- A5.4.6. Object localization
- A5.9.2. Estimation, modeling
- A5.9.4. Signal processing over graphs
- A5.9.6. Optimization tools
- A9.3. Signal analysis
Other Research Topics and Application Domains
- B3.3.1. Earth and subsoil
- B3.3.2. Water: sea & ocean, lake & river
- B3.3.3. Nearshore
- B3.4. Risks
- B3.5. Agronomy
- B3.6. Ecology
- B8.3. Urbanism and urban planning
- B8.4. Security and personal assistance
1 Team members, visitors, external collaborators
Research Scientist
- Josiane Zerubia [Team leader, Inria, Senior Researcher, HDR]
Post-Doctoral Fellows
- Camilo Gabriel Aguilar Herrera [Inria]
- Bilel Kanoun [Univ. Côte d'Azur (until 14/07/21), Inria (from 15/07/21)]
PhD Students
- Jules Mabon [Inria]
- Martina Pastorino [Inria and Genoa University (DITEN) Italy]
Interns and Apprentices
- Erick Takács [Inria and Szeged University (RGVC), Intern, until 31/01/21]
Administrative Assistant
- Nathalie Bellesso [Inria]
2 Overall objectives
The AYANA AEx is an interdisciplinary project using knowledge in stochastic modeling, image processing, artificial intelligence, remote sensing and embedded electronics/computing. The aerospace sector is expanding and changing ("New Space"). It is currently undergoing a great many changes both from the point of view of the sensors at the spectral level (uncooled IRT, far ultraviolet, etc.) and at the material level (the arrival of nano-technologies or the new generation of "Systems on chips" (SoCs) for example), that from the point of view of the carriers of these sensors: high resolution geostationary satellites; Leo-type low-orbiting satellites; or mini-satellites and industrial cube-sats in constellation. AYANA will work on a large number of data, consisting of very large images, having very varied resolutions and spectral components, and forming time series at frequencies of 1 to 60 Hz. For the embedded electronics/computing part, AYANA will work in close collaboration with specialists in the field located in Europe, working at space agencies and/or for industrial contractors.
3 Research program
3.1 FAULTS R GEMS: Properties of faults, a key to realistic generic earthquake modeling and hazard simulation
Decades of research on earthquakes have yielded meager prospects for earthquake predictability: we cannot predict the time, location and magnitude of a forthcoming earthquake with sufficient accuracy for immediate societal value. Therefore, the best we can do is to mitigate their impact by anticipating the most “destructive properties” of the largest earthquakes to come: longest extent of rupture zones, largest magnitudes, amplitudes of displacements, accelerations of the ground. This topic has motivated many studies in last decades. Yet, despite these efforts, major discrepancies still remain between available model outputs and natural earthquake behaviors. An important source of discrepancy is related to the incomplete integration of actual geometrical and mechanical properties of earthquake causative faults in existing rupture models. We first aim to document the compliance of rocks in natural permanent damage zones. These data –key to earthquake modeling– are presently lacking. A second objective is to introduce the observed macroscopic fault properties –compliant permanent damage, segmentation, maturity– into 3D dynamic earthquake models. A third objective is to conduct a pilot study aiming at examining the gain of prior fault property and rupture scenario knowledge for Earthquake Early Warning (EEW). This research project is partially funded by the ANR Fault R Gems, whose PI is Prof. I. Manighetti from Geoazur. Two sucessive postdocs (Barham Jafrasteh and Bilel Kanoun) have worked on this research topic funded by UCA-Jedi.
3.2 Probabilistic models on graphs and machine learning in remote sensing applied to natural disaster response
We currently develop novel probabilistic graphical models combined with machine learning in order to manage natural disasters such as earthquakes and flooding. The first model will introduce a semantic component to the graph at the current scale of the hierarchical graph, and will necessitate a new graph probabilistic model. The quad-tree proposed by Ihsen Hedhli in AYIN team in 2016 is no longer fit to resolve this issue. Applications from urban reconstruction or reforestation after natural disasters will be achieved on images from Pleiades optical satellites (provided by the French Space Agency, CNES) and CosmoSKyMed radar satellites (provided by the Italian Space Agency, ASI). This project is conducted in partnership with the University of Genoa (Prof. G.Moser and Prof. S. Serpico) via the co-supervision of a PhD student, financed by the Italian government. The PhD student, Martina Pastorino, has worked with Josiane Zerubia and Gabriele Moser in 2020 during her double Master degree at both University Genoa and IMT Atlantique. She started her PhD in co-supervision between University of Genoa DITEN (Prof. Moser) and Inria (Prof. Zerubia) in November 2020.
3.3 Marked point process models for object detection and tracking in temporal series of high resolution images
The model proposed by Paula Craciun's PhD thesis in 2015 in AYIN team, supposed the speed of tracked objects of interest in a series of satellite images to be quasi-constant between two frames. However this hypothesis is very limiting, particularly when objects of interest are subject to strong and sudden acceleration or deceleration. The model we proposed within AYIN team is then no longer viable. Two solutions will be considered within AYANA team : either a generalist model of marked point processes (MPP), supposing an unknown and variable velocity, or a multi-model of MPPs, simpler with regard to the velocity that can have a very limited amount of values (ie, quasi-constant velocity for each MPP). The whole model will have to be redesigned, and is to be tested with data from a constellation of small satellites, where the objects of interest can be for instance "speed-boats" or "go-fast cars". Some comparaisons with deep learning based methods belonging to Airbus Defense and Space (Airbus DS) will be done. Then this new model should be brought to diverses platforms (ground based or on-board). The modeling and ground-based application part will be studied within a PhD thesis (Jules Mabon), and the on-board version will be developed as part of a postdoctoral project (Camilo Aguilar-Hererra), both being conducted in partnership with Airbus DS, and the company Erems for the onboard hardware. This project is financed by BPI France within the LiChIE contract. This research project has started in 2020 for a duration of 4 years.
4 Application domains
Our research is applied within all Earth observation domains such as: urban planning, precision farming, natural disaster management, geological features detection, geospatial mapping, and security management.
5 Highlights of the year
5.1 Awards
- Martina Pastorino received the Prix d'excellence from Université Côte d’Azur (France) on December 10, 2021.
- Martina Pastorino received the best student paper award at IGARSS’21 6, and IEEE GRSS Mikio Takagi Student Prize in July 16 2021.
- Martina Pastorino received the Prize for Talent and Merit from Orientamenti 2021, Regione Liguria (Italy)
- Josiane Zerubia received the Doctor Honoris Causa from the University of Szeged (Hungary) on November 13, 2021.
6 New results
6.1 Multisensor and multiresolution remote sensing image classification through a causal hierarchical markov framework and decision tree ensembles
Participants: Martina Pastorino, Josiane Zerubia.
External collaborators: Gabriele Moser [Genoa University, DITEN, Professor], Sebastiano Serpico [Genoa University, DITEN, Professor], Ihsen Hedhli [Laval University, IID, Research scientist].
Keywords: image processing, stochastic models, optimization, multimodal data fusion.
This work consisted in the development of a hierarchical probabilistic graphical model to tackle joint classification of multiresolution and multisensor remote sensing images of the same scene. This problem is crucial in the study of satellite imagery in, particular for data fusion. Due to the heterogeneity of high or very high resolution (HR/VHR) remote sensing imagery, including optical (e.g., Pléiades, WorldView-3, SPOT-6/7) and synthetic aperture radar (SAR; e.g., COSMO-SkyMed Second Generation, TerraSAR-X, RADARSAT Constellation) data, a challenging problem is the development of image classifiers that can exploit information from multimodal input observations. The proposed framework consisted of a hierarchical Markov model with a quadtree structure to model information contained in different spatial scales, a planar Markov model to account for contextual spatial information at each resolution, and decision tree ensembles for pixelwise modeling. This probabilistic graphical model and its topology are especially fit for application to VHR image data. The theoretical properties of the proposed model were analyzed: the causality of the whole framework has been mathematically proved, granting the use of time-efficient inference algorithms based on the Marginal Posterior Mode (MPM) criterion, which are non-iterative when applied to quadtree structures. This is mostly advantageous for classification methods linked to multiresolution tasks formulated on hierarchical Markov models. Within the proposed framework, two multimodal classification algorithms were developed, that incorporate Markov mesh and spatial Markov chain concepts. The results obtained in the experimental validation conducted with two datasets containing VHR multispectral, panchromatic, and radar satellite images, verifies the effectiveness of the proposed framework (Figures 1 and 2). The proposed approach was compared to previous methods that are based on alternate strategies for multimodal fusion 23 and a journal paper was published in Remote Sensing 2.
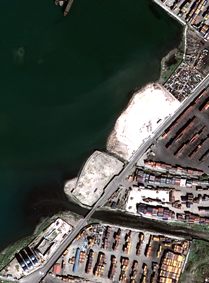
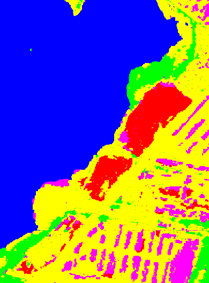
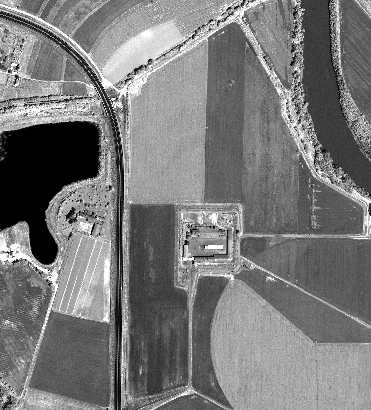
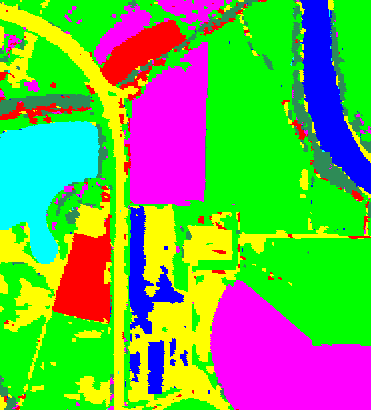
6.2 Semantic segmentation of remote sensing images combining hierarchical probabilistic graphical models and deep convolutional neural networks
Participants: Martina Pastorino, Josiane Zerubia.
External collaborators: Gabriele Moser [Genoa University, DITEN, Professor], Sebastiano Serpico [Genoa University, DITEN, Professor].
Keywords: image processing, stochastic models, deep learning, fully convolutional networks, multiscale analysis.
The work carried out consisted in the development of a novel method to deal with the semantic labelling of very high resolution (VHR) remote sensing data. Recent advances in deep learning, especially deep convolutional neural networks and fully convolutional networks (FCNs), have shown outstanding performances in this task. However, as for other methods, the map accuracy depends on the quantity and quality of ground truth (GT) used to train them. Having densely annotated data (i.e., a detailed, pixel-level GT) allows obtaining effective models, but requires high efforts in annotation. GTs related to real applications, such as remote sensing, are almost never exhaustive, they are spatially sparse and typically do not represent the spatial boundaries between the classes. Models trained with sparse maps usually produce results with poor geometric fidelity. This significantly affects the accuracy of the classification, and it is a major challenge in the development of deep neural networks for remote sensing. At the same time, probabilistic graphical models (PGMs) have sparked even more interest in the past few years, because of the ever-growing availability of VHR data and the correspondingly increasing need for structured predictions. The objective of this research work has been to combine different ideas from these approaches (deep learning and stochastic models) to develop novel methods for remote sensing image classification.
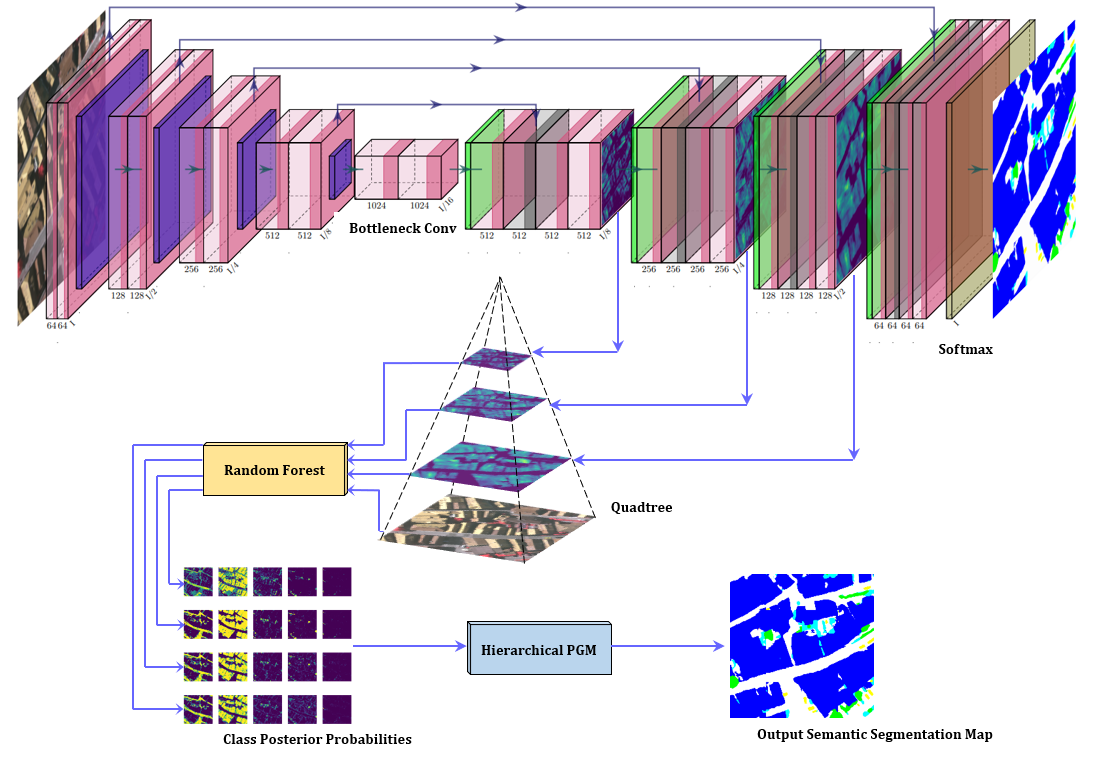
The logic was to take advantage of the spatial modeling capabilities of hierarchical PGMs to mitigate the impact of incomplete GTs and obtain accurate classification results. In particular, the study focuses on the possibility of exploiting the intrinsically multiscale nature of FCNs, to integrate them with hierarchical Markov models. This approach made use of a quadtree to model the interactions of the pixels, inter-layer and intra-layer. The technique is defined by three methodological components: an FCN, a hierarchical PGM and an ensemble learning approach to link the representation extracted from the FCN and the Bayesian inference structure of the PGM (Figure 3). The marginal posterior mode (MPM) criterion for inference was used in the proposed framework.
In order to develop a refined pipeline mixing deep learning and PGMs for meeting the rising need for accurate semantic mapping in remote sensing images, this work studied well-renowned deep learning architectures, i.e., U-Net, SegNet, HRNet, and DeepLabV3+. Experimental validation was conducted with the ISPRS 2D Semantic Labelling Challenge dataset, which includes satellite images of the cities of Vaihingen and Potsdam in Germany. These datasets are "ideal": the class label is known for all pixels in the images, a condition which is rarely available in realistic applications, as already mentioned before, where the objective is to generate accurate land cover or land use maps using fewer training samples. Therefore, the FCN has been further trained with a dataset with several modifications, aimed at approximating the most common GTs found in real remote sensing applications (which are far from being spatially exhaustive). The purpose is to evaluate the capabilities of the developed method in making improvements in terms of classification accuracy.
The results (Figures 4 and 5) are significant, as the proposed methodology has a higher producer accuracy (the map accuracy from the point of view of the map maker) compared to the standard FCNs considered, especially when the input training data are scarce and approach the real-world GTs available for land-cover mapping applications.
The proposed technique was compared to the recently proposed “FESTA” method 15, where the problem of FCN training with an incomplete GT is addressed through a loss term that favors regularization in the spatial and feature domains and with a multiscale feature fusion method making use of multiscale information through the concatenation of feature maps associated with different scales 18.
Three conference papers were published in EUSIPCO’21 5, IGARSS‘21 6, and ORASIS’2021 8, and a journal paper was accepted in December for publication in IEEE Transactions on Geoscience and Remote Sensing in 2022 3. In the future, the idea is to integrate the proposed method with transfer learning and test it with various datasets related to real world applications, such as disaster management, with different complexities and characteristics, to deepen the generalization capabilities of the proposed approach and compare them with those of a standard FCN in the same framework.
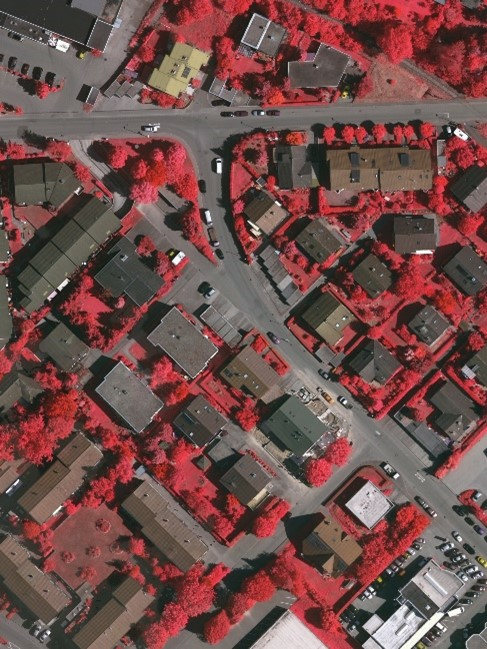
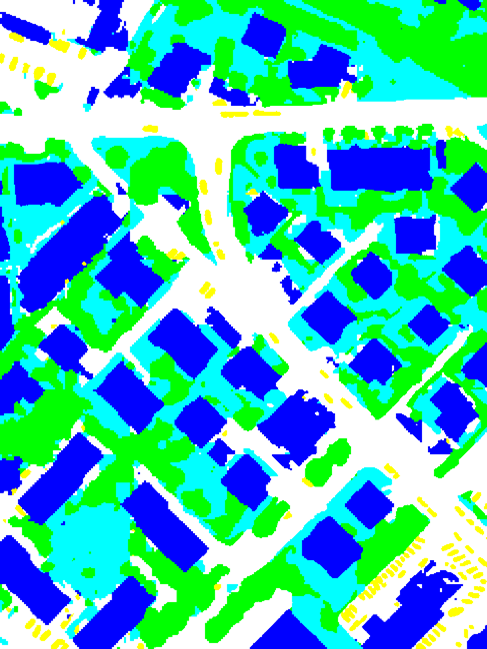
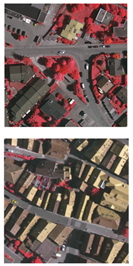
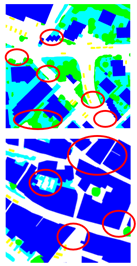
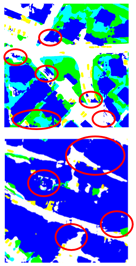
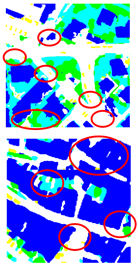
6.3 Change detection in multi-temporal remote sensing imagery using statistical and deep learning approaches
Participants: Erik Takács, Josiane Zerubia.
External collaborators: Zoltan Kato [Szeged University, RGVC, Professor].
Keywords: change detection, image segmentation, deep learning, remote sensing.
This project aimed at designing a deep neural network for change detection. As a premise, we considered the problem as a segmentation task; computing the difference of two remote sensing images which have different domains and using a threshold method to get a change map. However this would have risen some false positives, such as illumination changes and other natural changes that are not of interest to us. In order to solve this problem we used deep neural networks to get a change map which only includes the relevant changes. Our implementation consisted of two encoders with the two images as input, a feature difference layer which takes as input the outputs of the two encoders and outputs a deep feature difference and lastly a decoder which constructed the change map (see Figure 6). In order to segment the changed and unchanged areas we used the binary cross-entropy as loss function since the change detection task can be considered as a pixel wise binary classification between two classes: changed or unchanged. As we needed a large dataset for the segmentation, we used the public Change Detection Dataset (CDD) which consists of 16000 image pairs (256x256 pixels) and their associated ground truth . We trained our model on 5000 image pairs as a training set and 1500 image pairs as a validation set because of tight memory capacity. We evaluated different deep learning models 13, 19, 20 on public datasets.
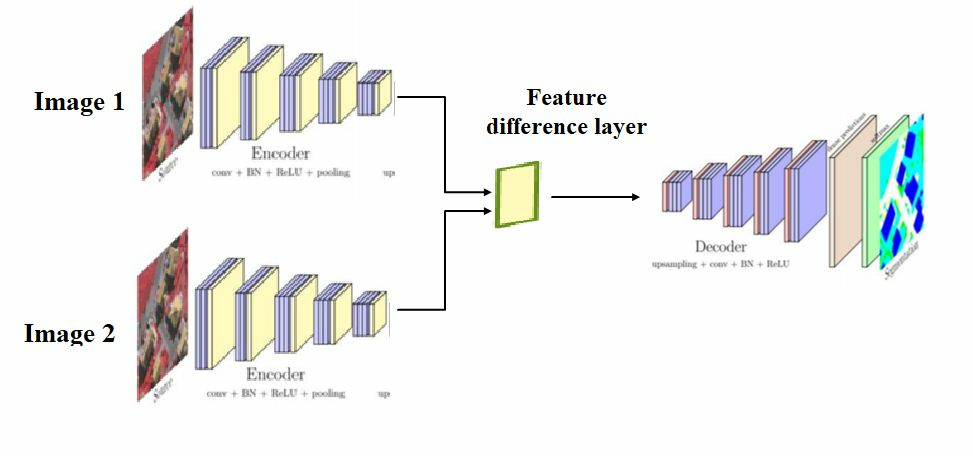
6.4 Extraction of curvilinear structure networks in image data using an innovative deep learning approach
Participants: Bilel Kanoun, Josiane Zerubia.
External collaborators: Isabelle Manighetti [OCA, Géoazur, Senior Physicist].
Keywords: curvilinear feature extraction, machine learning, VHR optical imagery, remote sensing.
Fractures and faults are ubiquitous on Earth and contribute to a number of important processes: small scale fractures control the permeability of rock reservoirs, and larger-scale faults may produce damaging earthquakes. Therefore, identifying and mapping fractures and faults are important in geosciences, especially in earthquake hazard and geological reservoir studies. However, the delineation of fractures and faults is a challenging task due to complexity of the faults. So far, mapping fractures and faults has been done manually in optical images of the Earth surface, yet it is time consuming and it requires an expertise that may not be available. To this purpose, the idea is to explore deep learning approaches for the automatic extraction of fractures and faults. Building upon a recent prior study namely TM-MRef model 22, we developed a deep learning approach, called BK-MRef (paper submitted to ICASSP 2022), based on a variant of a U-Net neural network, and applied it to automate fracture and fault mapping in optical images and topographic data. We performed the training of the model with a realistic knowledge of fracture and fault uneven distributions and trends (a “balanced training”), using a loss function, called the focal loss 17 , that “down-weights” the most represented features (not-a-fault) to focus on the less represented but most meaningful features (the fracture/fault pixels). In addition, we proceeded the study with another loss function that operates at both pixel and larger scales through a combined use of weighted binary Cross-entropy (wBCE) 14 and Jaccard loss 16, noted as combined loss L”. Such a loss function greatly improves the predictions, both quantitatively (see ROC curves in Figure 7) and qualitatively (see Figure 8). As we applied the model to a site different from those used for training, we have demonstrated it enhanced generalization capacity.
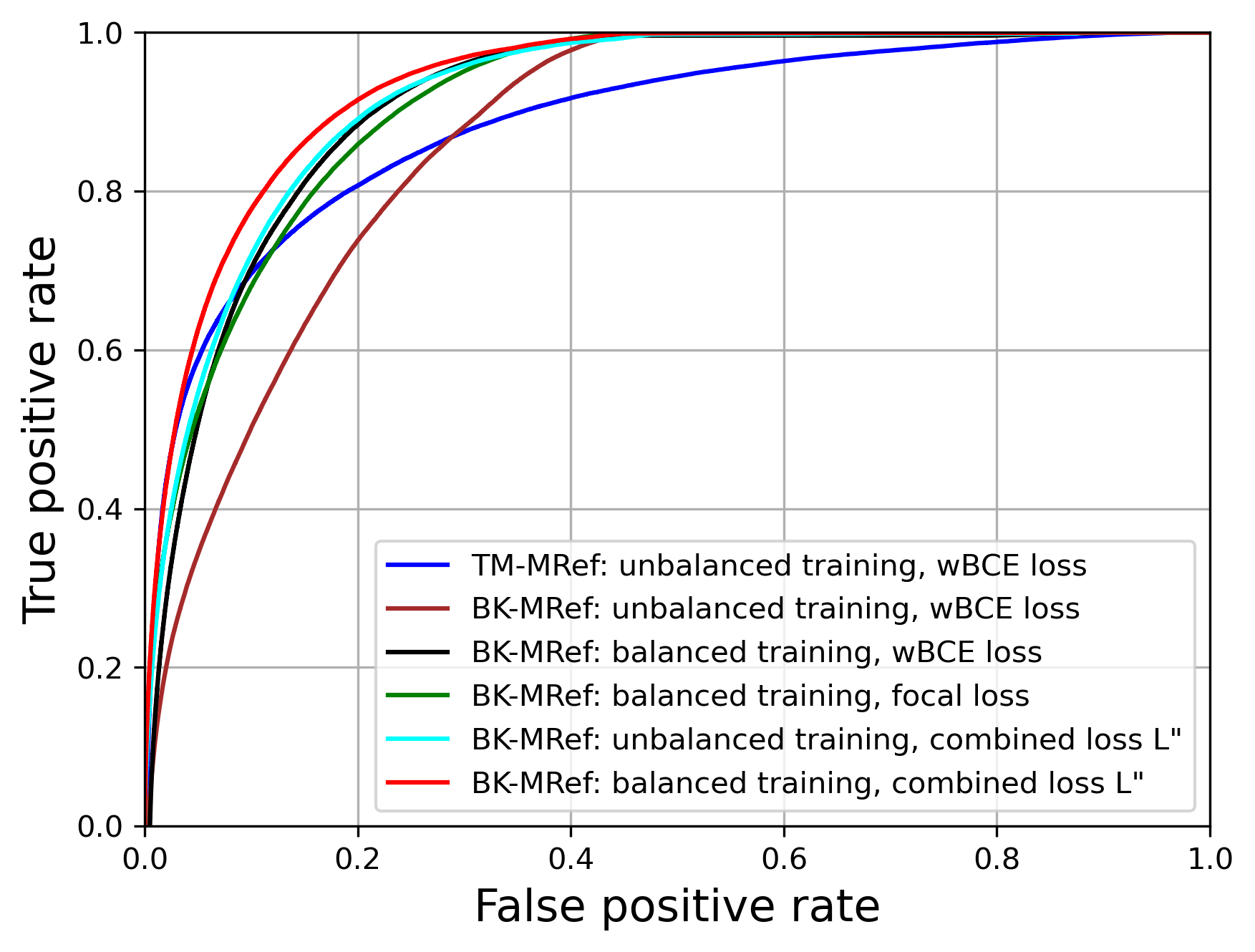
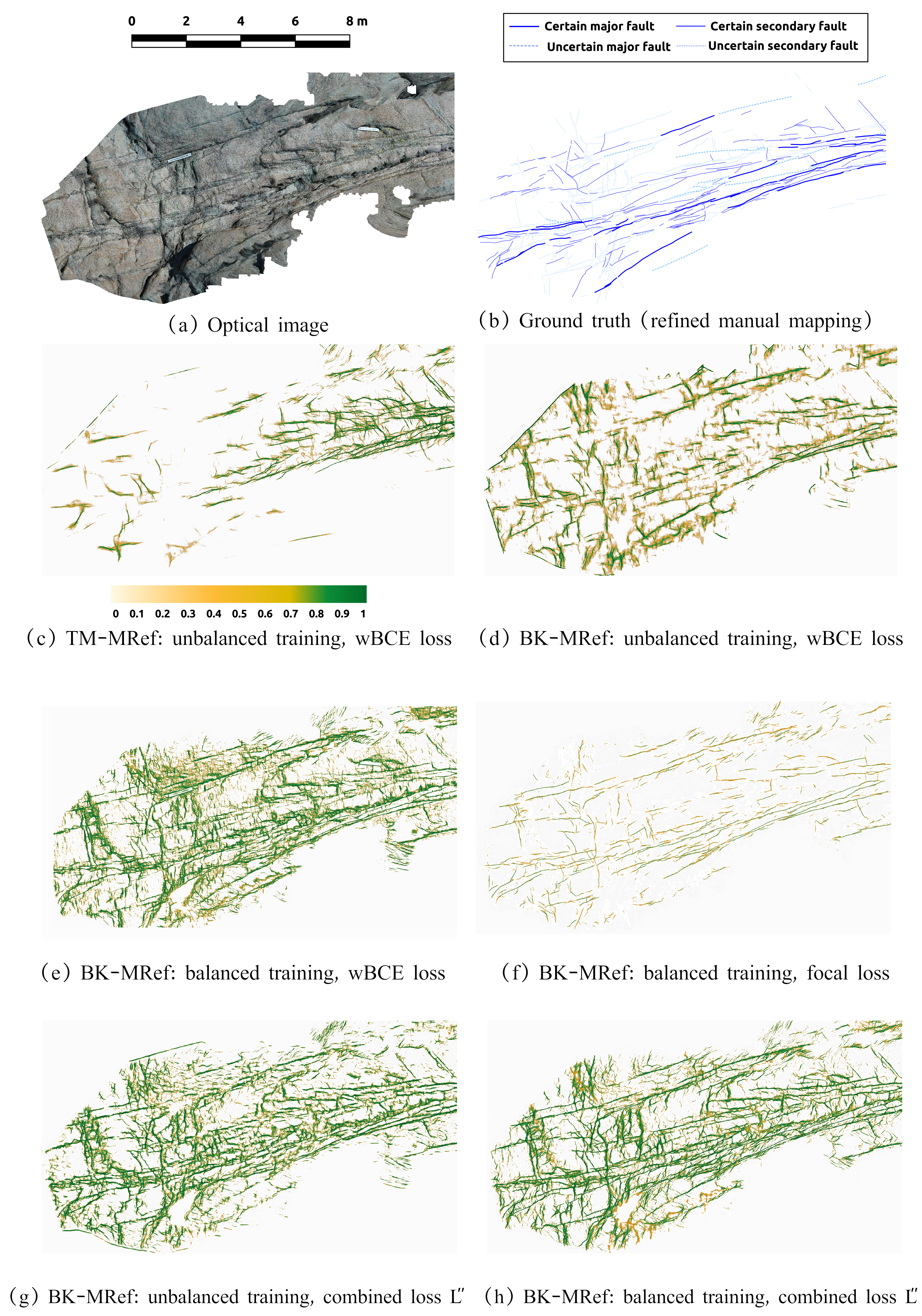
Predictions in the test zone with the different approaches. (a) Optical ortho-image of the test zone, (b) Manual expert mapping of fracture and fault traces (refined mapping), (c) Predictions using TM-MRef model and (d, e, f, g, h) variants of BK-MRef model.
6.5 Combining Stochastic geometry and deep learning for small objects detection in remote sensing datasets
Participants: Jules Mabon, Josiane Zerubia.
External collaborators: Mathias Ortner [Airbus Defense and Space, Senior Data Scientist].
Keywords: object detection, deep learning, fully convolutional networks, stochastic models.
Unmanned aerial vehicles and low-orbit satellites, including cubesats, are increasingly used for wide area surveillance, which results in large amounts of multi-source data that have to be processed and analyzed. These sensor platforms capture vast ground areas at frequencies varying from 1 to 60 Hz depending on the sensor. The number of moving objects in such data is typically very high, accounting for up to thousands of objects. On one hand, stochastic geometry has proven to be extremely powerful for capturing object positions within images using a prior model. For instance, it is possible to introduce a regularizing term that accounts for relative positions of objects (see previous research results in both AYIN and ARIANA teams), in order to represent specific patterns. One major drawback of this approach is that the amount of marks that can be handled has to be limited in order to avoid an explosion of the problem dimensionality. Moreover, parameter optimization is more complex when objects have complex shapes. On the other hand, deep learning approaches have largely proven over the recent years to be extremely efficient in building representations that match object signatures. In this research project, we aim at improving on the previous point process models for multiple objects tracking developed in AYIN team by Paula Craciun 10, 11, 12. We first tackled the problem of static detection. We propose to merge both approaches and build a stochastic point process model using a data term learned through a convolutional neural network (CNN); more specifically a Unet architecture, allowing for dense predictions on any image size. This approach allows us to leverage the generalization capabilities of neural networks while regularizing the results through prior energies in the point process model. The availability of detection map sourced from the CNN -used for the data term of the point process- allows us to leverage a birth map within the sampling procedure thus reduce the inference time. Our overall goal is to intertwine the sampling and learning procedure as much as feasible, in order to avoid the ad-hoc tweaking of parameters that is often necessary with point process modelizations. We perform tests of our CNN and point process joint model on the Cars Overhead With Context (COWC) dataset, subsampled at a spatial resolution of 50 cm per pixel (see Figure 9). In that first approach we consider a non-marked point process, i.e. objects are modeled as a simple set of coordinates (our current works focus on adding marks to this approach among other things). This work has been in part presented and published at the ORASIS 2021 conference in Toulouse 7. Airbus DS has been providing the data within the LiChIE contract funded by BPI France.
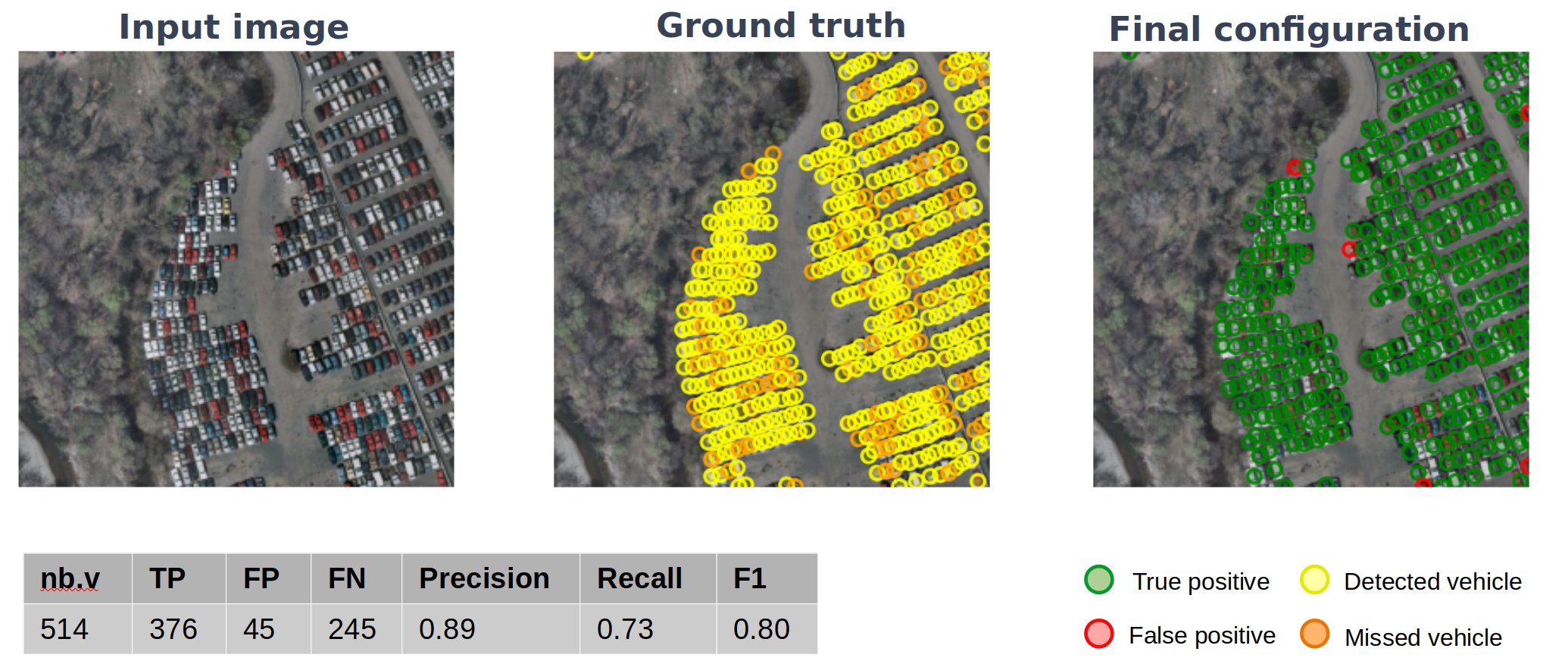
6.6 Small Object Detection and Tracking in Satellite Videos with Neural Networks and Random Finite Sets
Participants: Camilo Aguilar, Josiane Zerubia.
External collaborators: Mathias Ortner [Airbus Defense and Space, Senior Data Scientist].
Keywords: object detection, object tracking, convolutional neural networks, Random Finite Sets,.
This work presents a novel object detection and tracking approach for satellite videos. Specifically, the project focuses on tracking moving ground vehicles in low-resolution images (1 m/pixel). The main challenge arises from detecting and tracking dense populations of small objects in cluttered scenes. Vehicles span few pixels of width and height, lack distinctive features, and are often surrounded by texture rich backgrounds. We propose to use the 3-frame difference algorithm to extract motion information and rough target locations. We refine detection results with a patch-based convolutional neural network (CNN) focused on small regions containing moving targets. The patch-based CNN obtains accurate object locations, filters false detections, and discriminates nearby targets. Fig. 10 shows object detection results for the 3-frame difference and the advantage of using a CNN over 3-frame difference to refine the results.
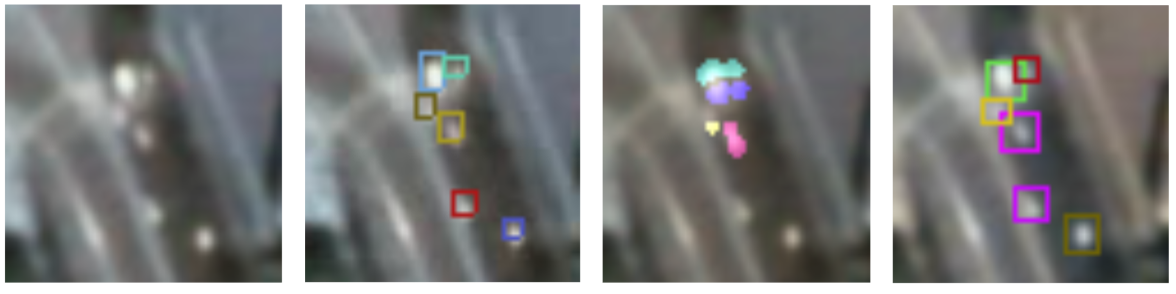
Additionally, we use the random finite set (RFS) 21 framework to extract temporal multi-object states and their uncertainty. The RFS approach provides a robust statistical formulation to infer multi-object posteriors by modeling object states and measurements as sets of finite dimensions. Using the RFS framework, we employ a modified version of the Gaussian mixture probability hypothesis density (GM-PHD) 25 filter to obtain object state estimates and object tracks. The GM-PHD filter offers a computationally feasible approximation of the multi-object posterior at the expense of assuming Gaussian noise and object linear motion. We further modify the GM-PHD to deal with unknown target appearing locations and to keep track of targets trajectories. Fig. 11 shows the tracks obtained by using the proposed modified GM-PHD filter.
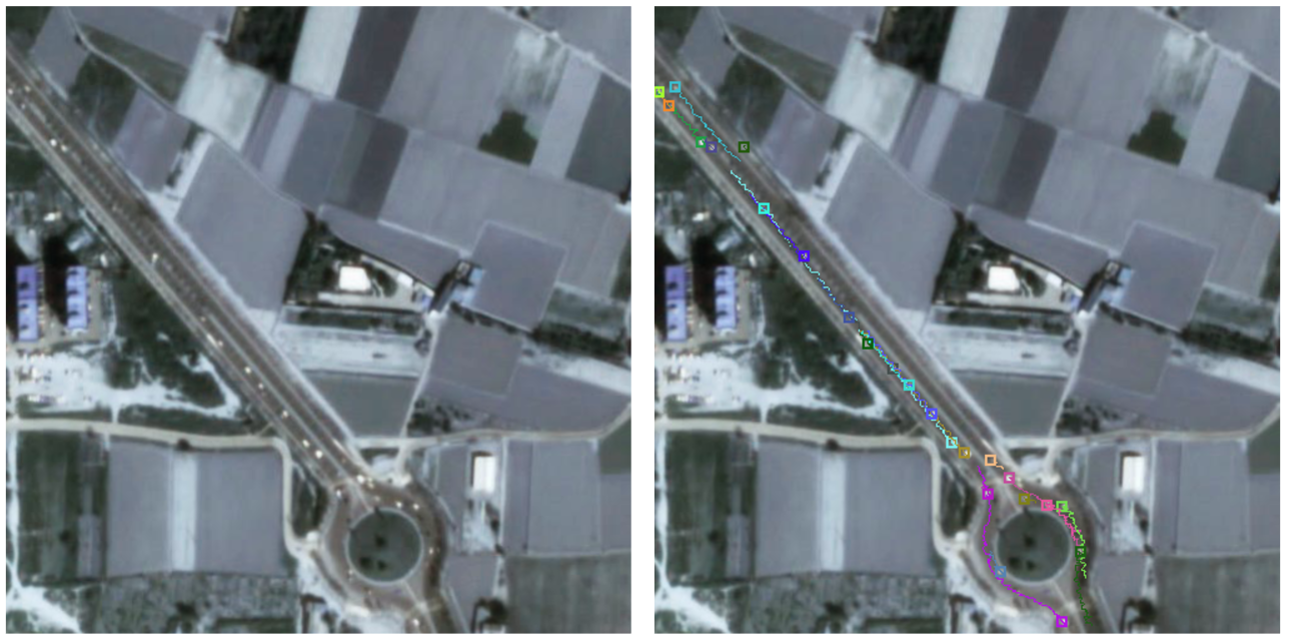
Our results show competitive scores across detection and tracking metrics and better performance than recent competing methods. These have been published in MLSP 2021 4 and submitted to Frontiers in Signal Processing in 2021 (under minor revisions in January 2022). However, satellite object tracking requires constant improvements to reduce the gap between computational requirements and accuracy. Currently, our work involves developing better and computationally feasible approximations for the RFS posterior using state-of-the-art filters 26 and novel CNN architectures 24.
7 Bilateral contracts and grants with industry
7.1 Bilateral contracts with industry
7.1.1 LiChIE contract with Airbus Defense and Space funded by BPI France
Participants: Camilo Aguilar, Jules Mabon, Josiane Zerubia.
External collaborators: Mathias Ortner [Airbus Defense and Space, Senior Data Scientist].
Automatic object detection and tracking on sequences of images taken from various constellations of satellites.
8 Partnerships and cooperations
8.1 International research visitors
8.1.1 Visits of international scientists
Other international visits to the team
Prof. Joseph Francos
Participants: Joseph Francos [Ben Gurion University, Professor], Josiane Zerubia, Bilel Kanoun, Jules Mabon.
-
Status
Professor
-
Institution of origin:
Ben Gurion University
-
Country:
Israel
-
Dates:
November 8 to 10
-
Context of the visit:
long term collaboration between Joseph Francos and Josiane Zerubia
-
Mobility program/type of mobility:
research stay
8.1.2 Visits to international teams
Research stays abroad
Josiane Zerubia
Participants: Josiane Zerubia [Szeged University, Professor], Zoltan Kato [Szeged University, Professor], Peter Horvath [BIOMAG, Biological Research Center Szeged, Group Leader], Tamas Sziranyi [SZTAKI Institute, Head of research laboratory], Csaba Benedek [GeoComp, SZTAKI Institute, Group Leader].
-
Visited institution:
University of Szeged and SZTAKI Institute
-
Country:
Hungary
-
Dates:
November 11 to 19
-
Context of the visit:
Doctor Honoris Causa ceremony and long term collaboration between Zoltan Kato, Tamas Sziranyi, Csaba Benedek and Josiane Zerubia
8.2 National initiatives
AYANA team is part of the ANR project FAULTS R GEMS (2017-2023, PI Geoazur) dedicated to realistic generic earthquake modeling and hazard simulation.
8.3 Regional initiatives
Isabelle Manighetti (OCA, Geoazur), and Josiane Zerubia (Inria-SAM) were co-PI of a UCA-AI grant (2020-mid 2021) of UCA-Jedi Idex to fund a postdoc, complementary to the ANR FAULTS R GEMS project.
9 Dissemination
9.1 Promoting scientific activities
9.1.1 Scientific events: organisation
General chair, scientific chair
- Josiane Zerubia was Technical Chair of the conference EUSIPCO‘21 in Dublin, Ireland (virtual), with Prof. Sabrina Greco and Prof. Stephen McLaughlin.
Member of the organizing committees
- Josiane Zerubia is part of the organizing committee and member of the National Scientific Committee of the ISPRS congress 2020 (digital), 2021 (virtual) and 2022 (in person), in Nice, France.
9.1.2 Scientific events: selection
Member of the conference program committees
- Josiane Zerubia was part of the conference program committee of SPIE Remote Sensing’21 (Madrid, Spain, virtual).
Reviewer
- Josiane Zerubia was a reviewer for the conferences IEEE ICASSP’21, IEEE EMBC’21, IEEE ICIP'21, IEEE/EURASIP EUSIPCO'21, SPIE Remote Sensing’21 and ISPRS Congress 2021.
9.1.3 Journal
Member of the editorial boards
- Josiane Zerubia is a member of the editorial boards of IEEE SPMag (2018- ), and Fondation and Trend in Signal Processing (2007- ).
Reviewer - reviewing activities
- Josiane Zerubia was a reviewer for IEEE SPMag and a lead guest editor for a special issue of IEEE SPMag.
9.1.4 Invited talks
- Josiane Zerubia gave two talks at Szeged Univ. (Computer Science Department) on November 16 and at SZTAKI Institute in Budapest on November 18 about "Hierarchical Probabilistic Graphical Models and Neural Networks for Remote Sensing Image Classification: Application to Natural Disasters and Urban Studies"
- Martina Pastorino gave a seminar about “Satellite Imagery of the Territory”, at UniTE, University of Genoa, on April 29 2021.
- Camilo Aguilar Herrera has been invited to present his work at MLSP 2021
- Jules Mabon has been invited to present his work at ORASIS 2021
- Martina Pastorino has been invited to present her work at IGARSS 2021, EUSIPCO 2021, ORASIS 2021
9.1.5 Leadership within the scientific community
- Josiane Zerubia is IEEE (2002- ), EURASIP (2019- ) and IAPR Fellow (2020- )
- Josiane Zerubia is member of the Teaching Board of the Doctoral School STIET at University of Genoa, Italy (2018- )
- Josiane Zerubia is member of the Scientific Council of GdR Geosto (Stochastic Geometry) from CNRS (2021- )
9.1.6 Scientific expertise
- Josiane Zerubia is member of the IEEE SPS Awards Board (2020-2022)
- Josiane Zerubia is member of IAPR Fellow Committee (2021-2022)
- Josiane Zerubia is member of JIVP EURASIP journal Best Paper Award Committee in 2021
9.1.7 Research administration
- Josiane Zerubia is member of various committees at Inria-SAM (CEP, CC, CB)
9.2 Teaching - Supervision - Juries
9.2.1 Teaching
- Masters: Josiane Zerubia , Remote Sensing, 13.5h eq. TD (9h of lectures), Master RISKS, Université Côte d'Azur, France (2019-2021). This course was given to Masters students .
- Masters: Martina Pastorino did Teaching support activities (TD 45h) for the course of Machine Learning for Pattern Recognition (laboratories), University of Genoa, (2020-2021).
9.2.2 Supervision
- Josiane Zerubia is supervising two postdocs, two PhD students and one Master student within the AYANA team.
9.2.3 Juries
- Josiane Zerubia was a member a PhD thesis committee at Purdue University (USA) in December 2021
9.3 Popularization
9.3.1 Articles and contents
- Josiane Zerubia was part of the WHAT06 "inspire me" project (Métiers/Aérospatiale, in French)
9.3.2 Interventions
- Josiane Zerubia participated on Dec 2, 2021 to the day “Girls, Mathematics and Computer Science” at Lycée Dumont d’Urville in Toulon (83). She talked in front of 138 female students to encourage them to follow a scientific cursus leading to technical jobs.
- Martina Pastorino was part of Notte dei Talenti, Orientamenti 2021, Primocanale.it, on regional TV, Genoa (Italy), on november 17, 2021.
- Josiane Zerubia participated to activities of both Femmes et Sciences and Terra Numerica in 2021, throughout the year via emails and websites.
10 Scientific production
10.1 Major publications
- 1 inbookHierarchical Markov random fields for high resolution land cover classification of multisensor and multiresolution image time series.2Change Detection and Image Time Series AnalysisSupervised MethodsISTE-WileyDecember 2021, 1-27
10.2 Publications of the year
International journals
International peer-reviewed conferences
National peer-reviewed Conferences
Scientific book chapters
10.3 Cited publications
- 10 inproceedingsIntegrating RJMCMC and Kalman filters for multiple object tracking.GRETSI -- Traitement du Signal et des ImagesLyon, FranceSeptember 2015
- 11 inproceedingsJoint detection and tracking of moving objects using spatio-temporal marked point processes.IEEE Winter Conference on Applications of Computer VisionHawaii, United StatesJanuary 2015
- 12 phdthesisStochastic geometry for automatic multiple object detection and tracking in remotely sensed high resolution image sequences.Université Nice Sophia AntipolisNovember 2015
- 13 articleUnsupervised Deep Slow Feature Analysis for Change Detection in Multi-Temporal Remote Sensing Images.IEEE Transactions on Geoscience and Remote Sensing57122019, 9976-9992
- 14 articleThe Real-World-Weight Cross-Entropy Loss Function: Modeling the Costs of Mislabeling.IEEE Access82020, 4806-4813
- 15 articleSemantic Segmentation of Remote Sensing Images With Sparse Annotations.IEEE Geoscience and Remote Sensing Letters2021, 1-5URL: https://dx.doi.org/10.1109/LGRS.2021.3051053
- 16 articleThe distribution of the flora in the Alpine zone.1.New Phytologist1121912, 37-50URL: https://nph.onlinelibrary.wiley.com/doi/abs/10.1111/j.1469-8137.1912.tb05611.x
- 17 inproceedingsFocal Loss for Dense Object Detection.Proceedings of the IEEE International Conference on Computer Vision (ICCV)2017, 2980-2988URL: https://openaccess.thecvf.com/content_iccv_2017/html/Lin_Focal_Loss_for_ICCV_2017_paper.html
- 18 inproceedingsLight-Weight Attention Semantic Segmentation Network for High-Resolution Remote Sensing Images.IGARSS 2020 - IEEE International Geoscience and Remote Sensing SymposiumWaikoloa, HI, USASep 2020, 2595-2598URL: https://dx.doi.org/10.1109/IGARSS39084.2020.9324723
- 19 articleCode-Aligned Autoencoders for Unsupervised Change Detection in Multimodal Remote Sensing Images.arXiv preprint arXiv:2004.070112020
- 20 articleDeep Image Translation with an Affinity-Based Change Prior for Unsupervised Multimodal Change Detection.arXiv preprint arXiv:2001.042712020
- 21 bookStatistical Multisource-Multitarget Information Fusion.USAArtech House, Inc.2007
- 22 articleAutomatic Fault Mapping in Remote Optical Images and Topographic Data With Deep Learning.Journal of Geophysical Research: Solid Earth1264e2020JB021269 2020JB0212692021, e2020JB021269URL: https://agupubs.onlinelibrary.wiley.com/doi/abs/10.1029/2020JB021269
- 23 articleA causal hierarchical Markov framework for the classification of multiresolution and multisensor remote sensing images.ISPRS Ann. of Photogramm., Remote Sens., Spat. Inf. Sci.3Aug 2020, 269-277URL: https://hal.inria.fr/hal-02982420
- 24 inproceedingsAttention is All you Need.Advances in Neural Information Processing Systems30Curran Associates, Inc.2017, 5998-6008URL: https://proceedings.neurips.cc/paper/2017/file/3f5ee243547dee91fbd053c1c4a845aa-Paper.pdf
- 25 articleThe Gaussian Mixture Probability Hypothesis Density Filter.IEEE Transactions on Signal Processing5412 2006, 4091 - 4104
- 26 articleAn Efficient Implementation of the Generalized Labeled Multi-Bernoulli Filter.IEEE Transactions on Signal Processing6582017, 1975-1987