Keywords
Computer Science and Digital Science
- A3.2.6. Linked data
- A3.3.2. Data mining
- A3.3.3. Big data analysis
- A5.3.3. Pattern recognition
- A5.4.1. Object recognition
- A5.4.3. Content retrieval
- A5.7. Audio modeling and processing
- A5.7.1. Sound
- A5.7.3. Speech
- A5.8. Natural language processing
- A9.2. Machine learning
- A9.3. Signal analysis
- A9.4. Natural language processing
Other Research Topics and Application Domains
- B9. Society and Knowledge
- B9.3. Medias
- B9.6.10. Digital humanities
- B9.10. Privacy
1 Team members, visitors, external collaborators
Research Scientists
- Laurent Amsaleg [Team leader, CNRS, Senior Researcher, HDR]
- Ioannis Avrithis [Inria, Advanced Research Position, until Jun 2021, HDR]
- Vincent Claveau [CNRS, Researcher, HDR]
- Teddy Furon [Inria, Researcher, HDR]
- Guillaume Gravier [CNRS, Senior Researcher, HDR]
Faculty Members
- Ewa Kijak [Univ de Rennes I, Associate Professor]
- Simon Malinowski [Univ de Rennes I, Associate Professor]
- Pascale Sébillot [INSA Rennes, Professor, HDR]
PhD Students
- Benoit Bonnet [Inria]
- Antoine Chaffin [Imatag, CIFRE]
- Deniz Engin [InterDigital, CIFRE]
- Julie Fournier [Univ de Rennes I, from Feb 2021]
- Marzieh Gheisari Khorasgani [Inria, until Mar 2021]
- Yann Lifchitz [Groupe SAFRAN, CIFRE, until Feb 2021]
- Thibault Maho [Inria]
- Cyrielle Mallart [Ouest-France Quotidien, CIFRE, until Nov 2021]
- Duc Hau Nguyen [CNRS]
- Raquel Pereira De Almeida [Université pontificale catholique du Minas Gerais Brésil]
- Samuel Tap [Zama Sas, CIFRE]
- Karim Tit [Thalès, CIFRE]
- Francois Torregrossa [Pages Jaunes, CIFRE]
- Shashanka Venkataramanan [Inria]
- Hanwei Zhang [Inria, until Mar 2021]
Technical Staff
- Guillaume Le Noe-Bienvenu [CNRS, Engineer, until Nov 2021]
Interns and Apprentices
- Hugo Thomas [CNRS, from May 2021 until Jul 2021]
Administrative Assistant
- Aurélie Patier [Univ de Rennes I]
Visiting Scientists
- Filippos Bellos [National and Kapodistrian University of Athens, Jan 2021]
- Josu Ircio Fernandez [Center for Technological Research Spain, Jan 2021]
- Michalis Lazarou [Imperial College London, Jan 2021]
2 Overall objectives
2.1 Context
Linkmedia is concerned with the processing of extremely large collections of multimedia material. The material we refer to are collections of documents that are created by humans and intended for humans. It is material that is typically created by media players such as TV channels, radios, newspapers, archivists (BBC, INA, ...), as well as the multimedia material that goes through social-networks. It has images, videos and pathology reports for e-health applications, or that is in relation with e-learning which typically includes a fair amount of texts, graphics, images and videos associating in new ways teachers and students. It also includes material in relation with humanities that study societies through the multimedia material that has been produced across the centuries, from early books and paintings to the latest digitally native multimedia artifacts. Some other multimedia material are out of the scope of Linkmedia, such as the ones created by cameras or sensors in the broad areas of video-surveillance or satellite images.
Multimedia collections are rich in contents and potential, that richness being in part within the documents themselves, in part within the relationships between the documents, in part within what humans can discover and understand from the collections before materializing its potential into new applications, new services, new societal discoveries, ... That richness, however, remains today hardly accessible due to the conjunction of several factors originating from the inherent nature of the collections, the complexity of bridging the semantic gap or the current practices and the (limited) technology:
- Multimodal: multimedia collections are composed of very diverse material (images, texts, videos, audio, ...), which require sophisticated approaches at analysis time. Scientific contributions from past decades mostly focused on analyzing each media in isolation one from the other, using modality-specific algorithms. However, revealing the full richness of collections calls for jointly taking into account these multiple modalities, as they are obviously semantically connected. Furthermore, involving resources that are external to collections, such as knowledge bases, can only improve gaining insight into the collections. Knowledge bases form, in a way, another type of modality with specific characteristics that also need to be part of the analysis of media collections. Note that determining what a document is about possibly mobilizes a lot of resources, and this is especially costly and time consuming for audio and video. Multimodality is a great source of richness, but causes major difficulties for the algorithms running analysis;
- Intertwined: documents do not exist in isolation one from the other. There is more knowledge in a collection than carried by the sum of its individual documents and the relationships between documents also carry a lot of meaningful information. (Hyper)Links are a good support for materializing the relationships between documents, between parts of documents, and having analytic processes creating them automatically is challenging. Creating semantically rich typed links, linking elements at very different granularities is very hard to achieve. Furthermore, in addition to being disconnected, there is often no strong structure into each document, which makes even more difficult their analysis;
- Collections are very large: the scale of collections challenges any algorithm that runs analysis tasks, increasing the duration of the analysis processes, impacting quality as more irrelevant multimedia material gets in the way of relevant ones. Overall, scale challenges the complexity of algorithms as well as the quality of the result they produce;
- Hard to visualize: It is very difficult to facilitate humans getting insight on collections of multimedia documents because we hardly know how to display them due to their multimodal nature, or due to their number. We also do not know how to well present the complex relationships linking documents together: granularity matters here, as full documents can be linked with small parts from others. Furthermore, visualizing time-varying relationships is not straightforward. Data visualization for multimedia collections remains quite unexplored.
2.2 Scientific objectives
The ambition of Linkmedia is to propose foundations, methods, techniques and tools to help humans make sense of extremely large collections of multimedia material. Getting useful insight from multimedia is only possible if tools and users interact tightly. Accountability of the analysis processes is paramount in order to allow users understanding their outcome, to understand why some multimedia material was classified this way, why two fragments of documents are now linked. It is key for the acceptance of these tools, or for correcting errors that will exist. Interactions with users, facilitating analytics processes, taking into account the trust in the information and the possible adversarial behaviors are topics Linkmedia addresses.
3 Research program
3.1 Scientific background
Linkmedia is de facto a multidisciplinary research team in order to gather the multiple skills needed to enable humans to gain insight into extremely large collections of multimedia material. It is multimedia data which is at the core of the team and which drives the design of our scientific contributions, backed-up with solid experimental validations. Multimedia data, again, is the rationale for selecting problems, applicative fields and partners.
Our activities therefore include studying the following scientific fields:
- multimedia: content-based analysis; multimodal processing and fusion; multimedia applications;
- computer vision: compact description of images; object and event detection;
- machine learning: deep architectures; structured learning; adversarial learning;
- natural language processing: topic segmentation; information extraction;
- information retrieval: high-dimensional indexing; approximate k-nn search; embeddings;
- data mining: time series mining; knowledge extraction.
3.2 Workplan
Overall, Linkmedia follows two main directions of research that are (i) extracting and representing information from the documents in collections, from the relationships between the documents and from what user build from these documents, and (ii) facilitating the access to documents and to the information that has been elaborated from their processing.
3.3 Research Direction 1: Extracting and Representing Information
Linkmedia follows several research tracks for extracting knowledge from the collections and representing that knowledge to facilitate users acquiring gradual, long term, constructive insights. Automatically processing documents makes it crucial to consider the accountability of the algorithms, as well as understanding when and why algorithms make errors, and possibly invent techniques that compensate or reduce the impact of errors. It also includes dealing with malicious adversaries carefully manipulating the data in order to compromise the whole knowledge extraction effort. In other words, Linkmedia also investigates various aspects related to the security of the algorithms analyzing multimedia material for knowledge extraction and representation.
Knowledge is not solely extracted by algorithms, but also by humans as they gradually get insight. This human knowledge can be materialized in computer-friendly formats, allowing algorithms to use this knowledge. For example, humans can create or update ontologies and knowledge bases that are in relation with a particular collection, they can manually label specific data samples to facilitate their disambiguation, they can manually correct errors, etc. In turn, knowledge provided by humans may help algorithms to then better process the data collections, which provides higher quality knowledge to humans, which in turn can provide some better feedback to the system, and so on. This virtuous cycle where algorithms and humans cooperate in order to make the most of multimedia collections requires specific support and techniques, as detailed below.
Machine Learning for Multimedia Material.
Many approaches are used to extract relevant information from multimedia material, ranging from very low-level to higher-level descriptions (classes, captions, ...). That diversity of information is produced by algorithms that have varying degrees of supervision. Lately, fully supervised approaches based on deep learning proved to outperform most older techniques. This is particularly true for the latest developments of Recurrent Neural Networkds (RNN, such as LSTMs) or convolutional neural network (CNNs) for images that reach excellent performance 66. Linkmedia contributes to advancing the state of the art in computing representations for multimedia material by investigating the topics listed below. Some of them go beyond the very processing of multimedia material as they also question the fundamentals of machine learning procedures when applied to multimedia.
- Learning from few samples/weak supervisions. CNNs and RNNs need large collections of carefully annotated data. They are not fitted for analyzing datasets where few examples per category are available or only cheap image-level labels are provided. Linkmedia investigates low-shot, semi-supervised and weakly supervised learning processes: Augmenting scarce training data by automatically propagating labels 69, or transferring what was learned on few very well annotated samples to allow the precise processing of poorly annotated data 78. Note that this context also applies to the processing of heritage collections (paintings, illuminated manuscripts, ...) that strongly differ from contemporary natural images. Not only annotations are scarce, but the learning processes must cope with material departing from what standard CNNs deal with, as classes such as "planes", "cars", etc, are irrelevant in this case.
- Ubiquitous Training. NN (CNNs, LSTMs) are mainstream for producing representations suited for high-quality classification. Their training phase is ubiquitous because the same representations can be used for tasks that go beyond classification, such as retrieval, few-shot, meta- and incremental learning, all boiling down to some form of metric learning. We demonstrated that this ubiquitous training is relatively simpler 69 yet as powerful as ad-hoc strategies fitting specific tasks 83. We study the properties and the limitations of this ubiquitous training by casting metric learning as a classification problem.
- Beyond static learning. Multimedia collections are by nature continuously growing, and ML processes must adapt. It is not conceivable to re-train a full new model at every change, but rather to support continuous training and/or allowing categories to evolve as the time goes by. New classes may be defined from only very few samples, which links this need for dynamicity to the low-shot learning problem discussed here. Furthermore, active learning strategies determining which is the next sample to use to best improve classification must be considered to alleviate the annotation cost and the re-training process 73. Eventually, the learning process may need to manage an extremely large number of classes, up to millions. In this case, there is a unique opportunity of blending the expertise of Linkmedia on large scale indexing and retrieval with deep learning. Base classes can either be "summarized" e.g. as a multi-modal distribution, or their entire training set can be made accessible as an external associative memory 89.
- Learning and lightweight architectures. Multimedia is everywhere, it can be captured and processed on the mobile devices of users. It is necessary to study the design of lightweight ML architectures for mobile and embedded vision applications. Inspired by 93, we study the savings from quantizing hyper-parameters, pruning connections or other approximations, observing the trade-off between the footprint of the learning and the quality of the inference. Once strategy of choice is progressive learning which early aborts when confident enough 74.
- Multimodal embeddings. We pursue pioneering work of Linkmedia on multimodal embedding, i.e., representing multiple modalities or information sources in a single embedded space 87, 86, 88. Two main directions are explored: exploiting adversarial architectures (GANs) for embedding via translation from one modality to another, extending initial work in 88 to highly heterogeneous content; combining and constraining word and RDF graph embeddings to facilitate entity linking and explanation of lexical co-occurrences 63.
- Accountability of ML processes. ML processes achieve excellent results but it is mandatory to verify that accuracy results from having determined an adequate problem representation, and not from being abused by artifacts in the data. Linkmedia designs procedures for at least explaining and possibly interpreting and understanding what the models have learned. We consider heat-maps materializing which input (pixels, words) have the most importance in the decisions 82, Taylor decompositions to observe the individual contributions of each relevance scores or estimating LID 50 as a surrogate for accounting for the smoothness of the space.
- Extracting information. ML is good at extracting features from multimedia material, facilitating subsequent classification, indexing, or mining procedures. Linkmedia designs extraction processes for identifying parts in the images 79, 80, relationships between the various objects that are represented in images 56, learning to localizing objects in images with only weak, image-level supervision 82 or fine-grained semantic information in texts 61. One technique of choice is to rely on generative adversarial networks (GAN) for learning low-level representations. These representations can e.g. be based on the analysis of density 92, shading, albedo, depth, etc.
- Learning representations for time evolving multimedia material. Video and audio are time evolving material, and processing them requests to take their time line into account. In 75, 59 we demonstrated how shapelets can be used to transform time series into time-free high-dimensional vectors, preserving however similarities between time series. Representing time series in a metric space improves clustering, retrieval, indexing, metric learning, semi-supervised learning and many other machine learning related tasks. Research directions include adding localization information to the shapelets, fine-tuning them to best fit the task in which they are used as well as designing hierarchical representations.
Adversarial Machine Learning.
Systems based on ML take more and more decisions on our behalf, and maliciously influencing these decisions by crafting adversarial multimedia material is a potential source of dangers: a small amount of carefully crafted noise imperceptibly added to images corrupts classification and/or recognition. This can naturally impact the insight users get on the multimedia collection they work with, leading to taking erroneous decisions e.g.
This adversarial phenomenon is not particular to deep learning, and can be observed even when using other ML approaches 55. Furthermore, it has been demonstrated that adversarial samples generalize very well across classifiers, architectures, training sets. The reasons explaining why such tiny content modifications succeed in producing severe errors are still not well understood.
We are left with little choice: we must gain a better understanding of the weaknesses of ML processes, and in particular of deep learning. We must understand why attacks are possible as well as discover mechanisms protecting ML against adversarial attacks (with a special emphasis on convolutional neural networks). Some initial contributions have started exploring such research directions, mainly focusing on images and computer vision problems. Very little has been done for understanding adversarial ML from a multimedia perspective 60.
Linkmedia is in a unique position to throw at this problem new perspectives, by experimenting with other modalities, used in isolation one another, as well as experimenting with true multimodal inputs. This is very challenging, and far more complicated and interesting than just observing adversarial ML from a computer vision perspective. No one clearly knows what is at stake with adversarial audio samples, adversarial video sequences, adversarial ASR, adversarial NLP, adversarial OCR, all this being often part of a sophisticated multimedia processing pipeline.
Our ambition is to lead the way for initiating investigations where the full diversity of modalities we are used to work with in multimedia are considered from a perspective of adversarial attacks and defenses, both at learning and test time. In addition to what is described above, and in order to trust the multimedia material we analyze and/or the algorithms that are at play, Linkmedia investigates the following topics:
- Beyond classification. Most contributions in relation with adversarial ML focus on classification tasks. We started investigating the impact of adversarial techniques on more diverse tasks such as retrieval 49. This problem is related to the very nature of euclidean spaces where distances and neighborhoods can all be altered. Designing defensive mechanisms is a natural companion work.
- Detecting false information. We carry-on with earlier pioneering work of Linkmedia on false information detection in social media. Unlike traditional approaches in image forensics 64, we build on our expertise in content-based information retrieval to take advantage of the contextual information available in databases or on the web to identify out-of-context use of text or images which contributed to creating a false information 76.
- Deep fakes. Progress in deep ML and GANs allow systems to generate realistic images and are able to craft audio and video of existing people saying or doing things they never said or did 72. Gaining in sophistication, these machine learning-based "deep fakes" will eventually be almost indistinguishable from real documents, making their detection/rebutting very hard. Linkmedia develops deep learning based counter-measures to identify such modern forgeries. We also carry on with making use of external data in a provenance filtering perspective 81 in order to debunk such deep fakes.
- Distributions, frontiers, smoothness, outliers. Many factors that can possibly explain the adversarial nature of some samples are in relation with their distribution in space which strongly differs from the distribution of natural, genuine, non adversarial samples. We are investigating the use of various information theoretical tools that facilitate observing distributions, how they differ, how far adversarial samples are from benign manifolds, how smooth is the feature space, etc. In addition, we are designing original adversarial attacks and develop detection and curating mechanisms 50.
Multimedia Knowledge Extraction.
Information obtained from collections via computer ran processes is not the only thing that needs to be represented. Humans are in the loop, and they gradually improve their level of understanding of the content and nature of the multimedia collection. Discovering knowledge and getting insight is involving multiple people across a long period of time, and what each understands, concludes and discovers must be recorded and made available to others. Collaboratively inspecting collections is crucial. Ontologies are an often preferred mechanism for modeling what is inside a collection, but this is probably limitative and narrow.
Linkmedia is concerned with making use of existing strategies in relation with ontologies and knowledge bases. In addition, Linkmedia uses mechanisms allowing to materialize the knowledge gradually acquired by humans and that might be subsequently used either by other humans or by computers in order to better and more precisely analyze collections. This line of work is instantiated at the core of the iCODA project Linkmedia coordinates.
We are therefore concerned with:
- Multimedia analysis and ontologies. We develop approaches for linking multimedia content to entities in ontologies for text and images, building on results in multimodal embedding to cast entity linking into a nearest neighbor search problem in a high-dimensional joint embedding of content and entities 86. We also investigate the use of ontological knowledge to facilitate information extraction from content 63.
- Explainability and accountability in information extraction. In relation with ontologies and entity linking, we develop innovative approaches to explain statistical relations found in data, in particular lexical or entity co-occurrences in textual data, for example using embeddings constrained with translation properties of RDF knowledge or path-based explanation within RDF graphs. We also work on confidence measures in entity linking and information extraction, studying how the notions of confidence and information source can be accounted for in knowledge basis and used in human-centric collaborative exploration of collections.
- Dynamic evolution of models for information extraction. In interactive exploration and information extraction, e.g., on cultural or educational material, knowledge progressively evolves as the process goes on, requiring on-the-fly design of new models for content-based information extractors from very few examples, as well as continuous adaptation of the models. Combining in a seamless way low-shot, active and incremental learning techniques is a key issue that we investigate to enable this dynamic mechanisms on selected applications.
3.4 Research Direction 2: Accessing Information
Linkmedia centers its activities on enabling humans to make good use of vast multimedia collections. This material takes all its cultural and economic value, all its artistic wonder when it can be accessed, watched, searched, browsed, visualized, summarized, classified, shared, ... This allows users to fully enjoy the incalculable richness of the collections. It also makes it possible for companies to create business rooted in this multimedia material.
Accessing the multimedia data that is inside a collection is complicated by the various type of data, their volume, their length, etc. But it is even more complicated to access the information that is not materialized in documents, such as the relationships between parts of different documents that however share some similarity. Linkmedia in its first four years of existence established itself as one of the leading teams in the field of multimedia analytics, contributing to the establishment of a dedicated community (refer to the various special sessions we organized with MMM, the iCODA and the LIMAH projects, as well as 70, 71, 67).
Overall, facilitating the access to the multimedia material, to the relevant information and the corresponding knowledge asks for algorithms that efficiently search collections in order to identify the elements of collections or of the acquired knowledge that are matching a query, or that efficiently allow navigating the collections or the acquired knowledge. Navigation is likely facilitated if techniques are able to handle information and knowledge according to hierarchical perspectives, that is, allow to reveal data according to various levels of details. Aggregating or summarizing multimedia elements is not trivial.
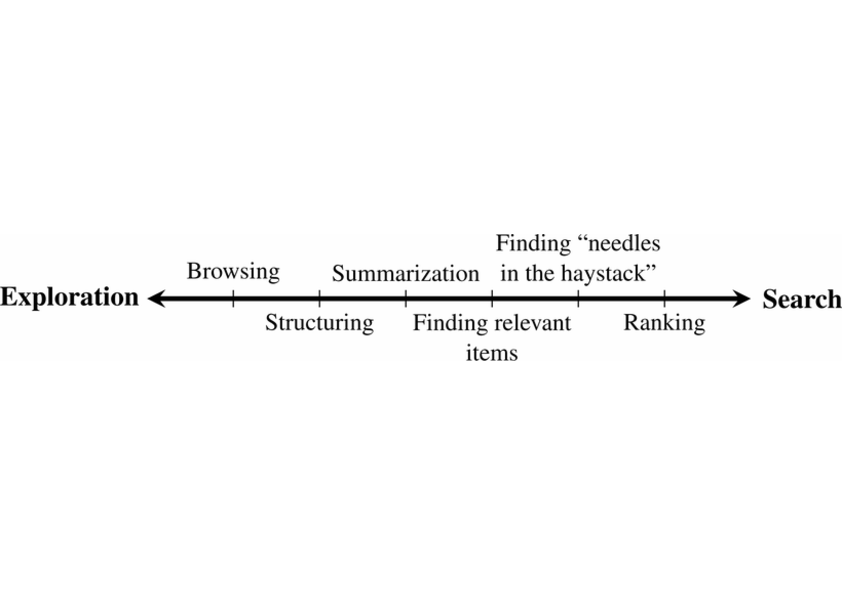
Three topics are therefore in relation with this second research direction. Linkmedia tackles the issues in relation to searching, to navigating and to summarizing multimedia information. Information needs when discovering the content of a multimedia collection can be conveniently mapped to the exploration-search axis, as first proposed by Zahálka and Worring in 91, and illustrated by Figure 1 where expert users typically work near the right end because their tasks involve precise queries probing search engines. In contrast, lay-users start near the exploration end of the axis. Overall, users may alternate searches and explorations by going back and forth along the axis. The underlying model and system must therefore be highly dynamic, support interactions with the users and propose means for easy refinements. Linkmedia contributes to advancing the state of the art in searching operations, in navigating operations (also referred to as browsing), and in summarizing operations.
Searching.
Search engines must run similarity searches very efficiently. High-dimensional indexing techniques therefore play a central role. Yet, recent contributions in ML suggest to revisit indexing in order to adapt to the specific properties of modern features describing contents.
-
Advanced scalable indexing. High-dimensional indexing is one of the foundations of Linkmedia. Modern features extracted from the multimedia material with the most recent ML techniques shall be indexed as well. This, however, poses a series of difficulties due to the dimensionality of these features, their possible sparsity, the complex metrics in use, the task in which they are involved (instance search,
-nn, class prototype identification, manifold search 69, time series retrieval, ...). Furthermore, truly large datasets require involving sketching 53, secondary storage and/or distribution 52, 51, alleviating the explosion of the number of features to consider due to their local nature or other innovative methods 68, all introducing complexities. Last, indexing multimodal embedded spaces poses a new series of challenges. - Improving quality. Scalable indexing techniques are approximate, and what they return typically includes a fair amount of false positives. Linkmedia works on improving the quality of the results returned by indexing techniques. Approaches taking into account neighborhoods 62, manifold structures instead of pure distance based similarities 69 must be extended to cope with advanced indexing in order to enhance quality. This includes feature selection based on intrinsic dimensionality estimation 50.
- Dynamic indexing. Feature collections grow, and it is not an option to fully reindex from scratch an updated collection. This trivially applies to the features directly extracted from the media items, but also to the base class prototypes that can evolve due to the non-static nature of learning processes. Linkmedia will continue investigating what is at stake when designing dynamic indexing strategies.
Navigating.
Navigating a multimedia collection is very central to its understanding. It differs from searching as navigation is not driven by any specific query. Rather, it is mostly driven by the relationships that various documents have one another. Relationships are supported by the links between documents and/or parts of documents. Links rely on semantic similarity, depicting the fact that two documents share information on the same topic. But other aspects than semantics are also at stake, e.g., time with the dates of creation of the documents or geography with mentions or appearance in documents of some geographical landmarks or with geo-tagged data.
In multimedia collections, links can be either implicit or explicit, the latter being much easier to use for navigation. An example of an implicit link can be the name of someone existing in several different news articles; we, as humans, create a mental link between them. In some cases, the computer misses such configurations, leaving such links implicit. Implicit links are subject to human interpretation, hence they are sometimes hard to identify for any automatic analysis process. Implicit links not being materialized, they can therefore hardly be used for navigation or faceted search. Explicit links can typically be seen as hyperlinks, established either by content providers or, more aligned with Linkmedia, automatically determined from content analysis. Entity linking (linking content to an entity referenced in a knowledge base) is a good example of the creation of explicit links. Semantic similarity links, as investigated in the LIMAH project and as considered in the search and hyperlinking task at MediaEval and TRECVid, are also prototypical links that can be made explicit for navigation. Pursuing work, we investigate two main issues:
- Improving multimodal content-based linking. We exploit achievements in entity linking to go beyond lexical or lexico-visual similarity and to provide semantic links that are easy to interpret for humans; carrying on, we work on link characterization, in search of mechanisms addressing link explainability (i.e., what is the nature of the link), for instance using attention models so as to focus on the common parts of two documents or using natural language generation; a final topic that we address is that of linking textual content to external data sources in the field of journalism, e.g., leveraging topic models and cue phrases along with a short description of the external sources.
- Dynamicity and user-adaptation. One difficulty for explicit link creation is that links are often suited for one particular usage but not for another, thus requiring creating new links for each intended use; whereas link creation cannot be done online because of its computational cost, the alternative is to generate (almost) all possible links and provide users with selection mechanisms enabling personalization and user-adaptation in the exploration process; we design such strategies and investigate their impact on exploration tasks in search of a good trade-off between performance (few high-quality links) and genericity.
Summarizing.
Multimedia collections contain far too much information to allow any easy comprehension. It is mandatory to have facilities to aggregate and summarize a large body on information into a compact, concise and meaningful representation facilitating getting insight. Current technology suggests that multimedia content aggregation and story-telling are two complementary ways to provide users with such higher-level views. Yet, very few studies already investigated these issues. Recently, video or image captioning 90, 85 have been seen as a way to summarize visual content, opening the door to state-of-the-art multi-document text summarization 65 with text as a pivot modality. Automatic story-telling has been addressed for highly specific types of content, namely TV series 57 and news 77, 84, but still need a leap forward to be mostly automated, e.g., using constraint-based approaches for summarization 54, 84.
Furthermore, not only the original multimedia material has to be summarized, but the knowledge acquired from its analysis is also to summarize. It is important to be able to produce high-level views of the relationships between documents, emphasizing some structural distinguishing qualities. Graphs establishing such relationships need to be constructed at various level of granularity, providing some support for summarizing structural traits.
Summarizing multimedia information poses several scientific challenges that are:
- Choosing the most relevant multimedia aggregation type: Taking a multimedia collection into account, a same piece of information can be present in several modalities. The issue of selecting the most suitable one to express a given concept has thus to be considered together with the way to mix the various modalities into an acceptable production. Standard summarization algorithms have to be revisited so that they can handle continuous representation spaces, allowing them to benefit from the various modalities 58.
- Expressing user’s preferences: Different users may appreciate quite different forms of multimedia summaries, and convenient ways to express their preferences have to be proposed. We for example focus on the opportunities offered by the constraint-based framework.
- Evaluating multimedia summaries: Finding criteria to characterize what a good summary is remains challenging, e.g., how to measure the global relevance of a multimodal summary and how to compare information between and across two modalities. We tackle this issue particularly via a collaboration with A. Smeaton at DCU, comparing the automatic measures we will develop to human judgments obtained by crowd-sourcing.
- Taking into account structuring and dynamicity: Typed links between multimedia fragments, and hierarchical topical structures of documents obtained via work previously developed within the team are two types of knowledge which have seldom been considered as long as summarization is concerned. Knowing that the event present in a document is causally related to another event described in another document can however modify the ways summarization algorithms have to consider information. Moreover the question of producing coarse-to-fine grain summaries exploiting the topical structure of documents is still an open issue. Summarizing dynamic collections is also challenging and it is one of the questions we consider.
4 Application domains
4.1 Asset management in the entertainment business
Media asset management—archiving, describing and retrieving multimedia content—has turned into a key factor and a huge business for content and service providers. Most content providers, with television channels at the forefront, rely on multimedia asset management systems to annotate, describe, archive and search for content. So do archivists such as the Institut National de l'Audiovisuel, the bibliothèque Nationale de France, the Nederlands Instituut voor Beeld en Geluid or the British Broadcast Corporation, as well as media monitoring companies, such as Yacast in France. Protecting copyrighted content is another aspect of media asset management.
4.2 Multimedia Internet
One of the most visible application domains of linked multimedia content is that of multimedia portals on the Internet. Search engines now offer many features for image and video search. Video sharing sites also feature search engines as well as recommendation capabilities. All news sites provide multimedia content with links between related items. News sites also implement content aggregation, enriching proprietary content with user-generated content and reactions from social networks. Most public search engines and Internet service providers offer news aggregation portals. This also concerns TV on-demand and replay services as well as social TV services and multi-screen applications. Enriching multimedia content, with explicit links targeting either multimedia material or knowledge databases is central here.
4.3 Data journalism
Data journalism forms an application domain where most of the technology developed by Linkmedia can be used. On the one hand, data journalists often need to inspect multiple heterogeneous information sources, some being well structured, some other being fully unstructured. They need to access (possibly their own) archives with either searching or navigational means. To gradually construct insight, they need collaborative multimedia analytics processes as well as elements of trust in the information they use as foundations for their investigations. Trust in the information, watching for adversarial and/or (deep) fake material, accountability are all crucial here.
5 Social and environmental responsibility
5.1 Impact of research results
Social biases in text generation.
Recent advances in the domain of text generation allow realistic text-based interaction with a computer. These systems rely on complex neural architectures that leverage very large amount of training texts collected the Web. The problem is that these texts contains unwanted biases (sexism, racism, harmful language...) that are sometimes even amplified by the training procedure. Curating the training texts once for all is not feasible due to the complexity of defining a priori what is relevant or not at the training time. Our work on controlled generation 35, 43 takes another point of view and tries to impose constraints at the inference time. This work aims at making the text generation respect application-specific conditions with the help of a simple classifier.
6 Highlights of the year
- The very first papers of two starting ph.D. students, Thibault Maho and Karim Tit, have been accepted to the two first-ranked Computer Science conferences (ranking from research.com), IEEE/CVF CVPR and NeuIPS.
- Teddy Furon was keynote speaker at IEEE Workshop on Information Forensics and Security.
- Best student paper award at WI-IAT for François Torregrossa 32.
- Linkmedia was in charge of representing the lab at the "Fête de la science" in October 2021.
7 New software and platforms
7.1 New software
7.1.1 SurFree
-
Name:
A fast surrogate-free black-box attack against classifier
-
Keywords:
Computer vision, Classification, Cyber attack
-
Scientific Description:
Machine learning classifiers are critically prone to evasion attacks. Adversarial examples are slightly modified inputs that are then misclassified, while remaining perceptively close to their originals. Last couple of years have witnessed a striking decrease in the amount of queries a black box attack submits to the target classifier, in order to forge adversarials. This particularly concerns the blackbox score-based setup, where the attacker has access to top predicted probabilites: the amount of queries went from to millions of to less than a thousand.
This paper presents SurFree, a geometrical approach that achieves a similar drastic reduction in the amount of queries in the hardest setup: black box decision-based attacks (only the top-1 label is available). We first highlight that the most recent attacks in that setup, HSJA, QEBA and GeoDA all perform costly gradient surrogate estimations. SurFree proposes to bypass these, by instead focusing on careful trials along diverse directions, guided by precise indications of geometrical properties of the classifier decision boundaries. We motivate this geometric approach before performing a head-to-head comparison with previous attacks with the amount of queries as a first class citizen. We exhibit a faster distortion decay under low query amounts (few hundreds to a thousand), while remaining competitive at higher query budgets.
Paper : https://arxiv.org/abs/2011.12807
-
Functional Description:
This software is the implementation in python of the attack SurFree. This is an attack against a black-box classifier. It finds an input close to the reference input (Euclidean distance) yet not classified with the same predicted label as the reference input. This attack has been tested against image classifier in computer vision.
- URL:
-
Authors:
Thibault Maho, Erwan Le Merrer, Teddy Furon
-
Contact:
Thibault Maho
7.1.2 AML
-
Name:
Asymmetric Metric Learning
-
Keywords:
Knowledge transfer, Metric learning, Image retrieval
-
Functional Description:
This is the official code and a set of pre-trained models that enable the reproduction of the results of our paper https://hal.inria.fr/hal-03047591.
- URL:
-
Contact:
Ioannis Avrithis
7.1.3 DAL
-
Name:
Rethinking Deep Active Learning
-
Keywords:
Active Learning, Deep learning
-
Functional Description:
This is the official code that enables the reproduction of the results of our paper https://hal.inria.fr/hal-02372102.
- URL:
-
Contact:
Ioannis Avrithis
7.1.4 HyperStoryLines
-
Keywords:
Data visualization, Hypergraphs
-
Scientific Description:
HyperStorylines is a technique that generalizes Storylines to visualize the evolution of relationships involving multiple types of entities such as, for example, people, locations, and companies. Datasets which describe such multi-entity relationships are often modeled as hypergraphs, that can be difficult to visualize, especially when these relationships evolve over time. HyperStorylines builds upon Storylines, enabling the aggregation and nesting of these dynamic, multi-entity relationships.
-
Functional Description:
A visualization tool to represent relationships among different types of entities, forming a hypergraph, and that evolve over time.
- URL:
- Publication:
-
Contact:
Vanessa Pena Araya
-
Participants:
Vanessa Pena Araya, Anastasia Bezerianos, Emmanuel Pietriga, Rim Hajri, Laurent Amsaleg
7.1.5 RoBIC
-
Name:
A benchmark suite for assessing classifiers robustness
-
Keywords:
Adversarial attack, Classification, Deep learning
-
Functional Description:
From a dataset of test images annotated with ground truth (like ImageNet), the benchmark leads two attacks to delude the classifier over this set of images and compute some statistics gauging the robustness of the classifier.
- URL:
-
Contact:
Thibault Maho
8 New results
8.1 Extracting and Representing Information
8.1.1 Training Object Detectors from Few Weakly-Labeled and Many Unlabeled Images
Participants: Zhaohui Yang [Key Laboratory of Machine Perception, Beijing], Miaojing Shi [King‘s College, London], Chao Xu [Key Laboratory of Machine Perception, Beijing], Vittorio Ferrari [Google Research, Zurich], Yannis Avrithis.
Weakly-supervised object detection attempts to limit the amount of supervision by dispensing the need for bounding boxes, but still assumes image-level labels on the entire training set. In this work, we study the problem of training an object detector from one or few images with image-level labels and a larger set of completely unlabeled images 11. This is an extreme case of semi-supervised learning where the labeled data are not enough to bootstrap the learning of a detector. Our solution is to train a weakly-supervised student detector model from image-level pseudo-labels generated on the unlabeled set by a teacher classifier model, bootstrapped by region-level similarities to labeled images. Building upon the recent representative weakly-supervised pipeline PCL, our method can use more unlabeled images to achieve performance competitive or superior to many recent weakly-supervised detection solutions.
8.1.2 Asymmetric metric learning for knowledge transfer
Participants: Mateusz Budnik, Yannis Avrithis.
Knowledge transfer from large teacher models to smaller student models has recently been studied for metric learning, focusing on fine-grained classification. In this work, focusing on instance-level image retrieval, we study an asymmetric testing task, where the database is represented by the teacher and queries by the student. Inspired by this task, we introduce asymmetric metric learning, a novel paradigm of using asymmetric representations at training. This acts as a simple combination of knowledge transfer with the original metric learning task. We systematically evaluate different teacher and student models, metric learning and knowledge transfer loss functions on the new asymmetric testing as well as the standard symmetric testing task, where database and queries are represented by the same model. We find that plain regression is surprisingly effective compared to more complex knowledge transfer mechanisms, working best in asymmetric testing. Interestingly, our asymmetric metric learning approach works best in symmetric testing, allowing the student to even outperform the teacher 13. Our implementation is publicly available.8.1.3 Local Propagation for Few-Shot Learning
Participants: Yann Lifchitz [Safran, Paris], Yannis Avrithis [Safran, Paris], Sylvaine Picard [Safran, Paris].
The challenge in few-shot learning is that available data is not enough to capture the underlying distribution. To mitigate this, two emerging directions are (a) using local image representations, essentially multiplying the amount of data by a constant factor, and (b) using more unlabeled data, for instance by transductive inference, jointly on a number of queries. In this work (24), we bring these two ideas together, introducing local propagation. We treat local image features as independent examples, we build a graph on them and we use it to propagate both the features themselves and the labels, known and unknown. Interestingly, since there is a number of features per image, even a single query gives rise to transductive inference. As a result, we provide a universally safe choice for few-shot inference under both non-transductive and transductive settings, improving accuracy over corresponding methods. This is in contrast to existing solutions, where one needs to choose the method depending on the quantity of available data.8.1.4 Rethinking deep active learning: Using unlabeled data at model training
Participants: Oriane Siméoni [Valeo], Mateusz Budnik, Yannis Avrithis, Guillaume Gravier.
Active learning typically focuses on training a model on few labeled examples alone, while unlabeled ones are only used for acquisition. In this work we depart from this setting by using both labeled and unlabeled data during model training across active learning cycles. We do so by using unsupervised feature learning at the beginning of the active learning pipeline and semi-supervised learning at every active learning cycle, on all available data. The former has not been investigated before in active learning, while the study of latter in the context of deep learning is scarce and recent findings are not conclusive with respect to its benefit. Our idea is orthogonal to acquisition strategies by using more data, much like ensemble methods use more models. By systematically evaluating on a number of popular acquisition strategies and datasets, we find that the use of unla-beled data during model training brings a surprising accuracy improvement in image classification, compared to the differences between acquisition strategies. We thus explore smaller label budgets, even one label per class 29.8.1.5 Few-Shot Few-Shot Learning and the role of Spatial Attention
Participants: Yann Lifchitz [Safran, Paris], Yannis Avrithis [Safran, Paris], Sylvaine Picard [Safran, Paris].
Few-shot learning is often motivated by the ability of humans to learn new tasks from few examples. However, standard few-shot classification benchmarks assume that the representation is learned on a limited amount of base class data, ignoring the amount of prior knowledge that a human may have accumulated before learning new tasks. At the same time, even if a powerful representation is available, it may happen in some domain that base class data are limited or non-existent. This motivates us to study a problem where the representation is obtained from a classifier pre-trained on a large-scale dataset of a different domain, assuming no access to its training process, while the base class data are limited to few examples per class and their role is to adapt the representation to the domain at hand rather than learn from scratch. We adapt the representation in two stages, namely on the few base class data if available and on the even fewer data of new tasks. In doing so, we obtain from the pre-trained classifier a spatial attention map that allows focusing on objects and suppressing background clutter. This is important in the new problem, because when base class data are few, the network cannot learn where to focus implicitly. We also show in 23that a pre-trained network may be easily adapted to novel classes, without meta-learning.8.1.6 Detecting Human-Object Interaction with Mixed Supervision
Participants: Suresh Kumaraswamy [King's college, London], Miaojing Shi [King's college, London], Ewa Kijak.
Human object interaction (HOI) detection is an important task in image understanding and reasoning. It is in a form of HOI triplet human, verb, object, requiring bounding boxes for human and object, and action between them for the task completion. In other words, this task requires strong supervision for training that is however hard to procure. A natural solution to overcome this is to pursue weakly-supervised learning, where we only know the presence of certain HOI triplets in images but their exact location is unknown. Most weakly-supervised learning methods do not make provision for leveraging data with strong supervision, when they are available; and indeed a naive combination of this two paradigms in HOI detection fails to make contributions to each other. In this regard we propose in 19 a mixed-supervised HOI detection pipeline: thanks to a specific design of momentum-independent learning that learns seamlessly across these two types of supervision. Moreover, in light of the annotation insufficiency in mixed supervision, we introduce an HOI element swapping technique to synthesize diverse and hard negatives across images and improve the robustness of the model. Our method is evaluated on the challenging HICO-DET dataset. It performs close to or even better than many fully-supervised methods by using a mixed amount of strong and weak annotations; furthermore, it outperforms representative state of the art weakly and fully-supervised methods under the same supervision.8.1.7 Attaques et défenses de réseaux de neurones profonds : le cas de la classification d’images
Participants: Hanwei Zhang, Teddy Furon, Laurent Amsaleg, Yannis Avrithis.
L’apprentissage automatique utilisant des réseaux neuronaux profonds appliqués à la reconnaissance d’images fonctionne extrêmement bien. Néanmoins, il est possible de modifier intentionnellement et très légèrement les images, modifications quasi invisibles à nos yeux, pour leurrer le système de classification et lui faire classer dans une catégorie visuelle erronée tel ou tel contenu. Ce chapitre dresse un panorama de ces attaques intentionnelles, mais aussi des mécanismes de défense visant à les déjouer 37.8.1.8 Traçage de traîtres
Participant: Teddy Furon.
Dans 36 nous présentons le problème du traçage de traîtres et la solution la plus efficace connue, les codes de Tardos. Les codes traçants constituent une surcouche applicative à la couche de transmission par tatouage : un identifiant est généré pour chaque utili- sateur, puis inséré par tatouage dans un document confidentiel à partager. Le chapitre met avant tout l’accent sur la modélisation de la collusion lorsque plusieurs traîtres mélangent leurs copies. Grâce à ce modèle, les mathématiques (statistiques, théorie de l’information) nous donnent les limites fondamentales de cet outil.8.1.9 Detecting Fake News Conspiracies with Multitask and Prompt-Based Learning
Participants: Cheikh Brahim El Vaigh [Shaman], Thomas Girault [Ouest-France], Cyrielle Mallart, Duc Hau Nguyen.
We present in 17 our participation to the task of fake news conspiracy theories detection from tweets. We rely on a variant of BERT-based classification approach to devise a first classification method for the three different tasks. Moreover, we propose a multitask learning approach to perform the three different tasks at once. Finally, we developed a prompt-based approach to generate classifications thanks to a TinyBERT pre-trained model. Our experimental results show the multitask model to be the best on the three tasks.8.1.10 On the hidden treasure of dialog in video question answering
Participants: Deniz Engin [InterDigital Communications], François Schnitzler [InterDigital Communications], Ngoc Duong, Yannis Avrithis.
High-level understanding of stories in video such as movies and TV shows from raw data is extremely challenging. Modern video question answering (VideoQA) systems often use additional human-made sources like plot synopses, scripts, video descriptions or knowledge bases. In this work, we present a new approach to understand the whole story without such external sources 18. The secret lies in the dialog: unlike any prior work, we treat dialog as a noisy source to be converted into text description via dialog summarization, much like recent methods treat video. The input of each modality is encoded by transformers independently, and a simple fusion method combines all modalities, using soft temporal attention for localization over long inputs. Our model outperforms the state of the art on the KnowIT VQA dataset by a large margin, without using question-specific human annotation or humanmade plot summaries. It even outperforms human evaluators who have never watched any whole episode before.8.1.11 Iterative label cleaning for transductive and semi-supervised few-shot learning
Participants: Michalis Lazarou, Tania Stathaki, Yannis Avrithis.
Few-shot learning amounts to learning representations and acquiring knowledge such that novel tasks may be solved with both supervision and data being limited. Improved performance is possible by transductive inference, where the entire test set is available concurrently, and semi-supervised learning, where more unlabeled data is available. Focusing on these two settings, we introduce a new algorithm that leverages the manifold structure of the labeled and unlabeled data distribution to predict pseudo-labels, while balancing over classes and using the loss value distribution of a limited-capacity classifier to select the cleanest labels, iteratively improving the quality of pseudo-labels. Our solution described in 20 surpasses or matches the state of the art results on four benchmark datasets, namely miniImageNet, tieredImageNet, CUB and CIFAR-FS, while being robust over feature space pre-processing and the quantity of available data.8.1.12 Forensics Through Stega Glasses: the Case of Adversarial Images
Participants: Benoît Bonnet [CRIStAL - Centre de Recherche en Informatique, Signal et Automatique de Lille - UMR 9189], Teddy Furon [CRIStAL - Centre de Recherche en Informatique, Signal et Automatique de Lille - UMR 9189], Patrick Bas [CRIStAL - Centre de Recherche en Informatique, Signal et Automatique de Lille - UMR 9189].
This paper explores the connection between forensics, counterforensics, steganography and adversarial images 12. On the one hand, forensicsbased and steganalysis-based detectors help in detecting adversarial perturbations. On the other hand, steganography can be used as a counterforensics strategy and helps in forging adversarial perturbations that are not only invisible to the human eye but also less statistically detectable. This work explains how to use these information hiding tools for attacking or defending computer vision image classification. We play this cat and mouse game using both recent deep-learning content-based classifiers, forensics detectors derived from steganalysis, and steganographic distortions dedicated to color quantized images. It turns out that crafting adversarial perturbations relying on steganographic perturbations is an effective counter-forensics strategy.8.1.13 Generating Adversarial Images in Quantized Domains
Participants: Benoît Bonnet [CRIStAL - Centre de Recherche en Informatique, Signal et Automatique de Lille - UMR 9189], Teddy Furon [CRIStAL - Centre de Recherche en Informatique, Signal et Automatique de Lille - UMR 9189], Patrick Bas [CRIStAL - Centre de Recherche en Informatique, Signal et Automatique de Lille - UMR 9189].
Many adversarial attacks produce floating-point tensors which are no longer adversarial when converted to raster or JPEG images due to rounding. This paper 2 proposes a method dedicated to quantize adversarial perturbations. This "smart" quantization is conveniently implemented as versatile post-processing. It can be used on top of any white-box attack targeting any model. Its principle is tantamount to a constrained optimization problem aiming to minimize the quantization error while keeping the image adversarial after quantization. A Lagrangian formulation is proposed and an appropriate search of the Lagrangian multiplier enables to increase the success rate. We also add a control mechanism of the8.1.14 SurFree: a fast surrogate-free black-box attack
Participants: Thibault Maho [Wide], Teddy Furon [Wide], Erwan Le Merrer [Wide].
Machine learning classifiers are critically prone to evasion attacks. Adversarial examples are slightly modified inputs that are then misclassified, while remaining perceptively close to their originals. Last couple of years have witnessed a striking decrease in the amount of queries a black box attack submits to the target classifier, in order to forge adversarials. This particularly concerns the black-box score-based setup, where the attacker has access to top predicted probabilites: the amount of queries went from millions of to less than a thousand. This paper presents SurFree, a geometrical approach that achieves a similar drastic reduction in the amount of queries in the hardest setup 5, 26: black box decision-based attacks (only the top-1 label is available). We first highlight that the most recent attacks in that setup, HSJA, QEBA and GeoDA all perform costly gradient surrogate estimations. SurFree proposes to bypass these, by instead focusing on careful trials along diverse directions, guided by precise indications of geometrical properties of the classifier decision boundaries. We motivate this geometric approach before performing a head-to-head comparison with previous attacks with the amount of queries as a first class citizen. We exhibit a faster distortion decay under low query amounts (few hundreds to a thousand), while remaining competitive at higher query budgets.8.1.15 RoBIC: A benchmark suite for assessing classifiers robustness
Participants: Thibault Maho [Wide], Benoît Bonnet [Wide], Teddy Furon [Wide], Erwan Le Merrer [Wide].
Many defenses have emerged with the development of adversarial attacks. Models must be objectively evaluated accordingly. This paper systematically tackles this concern by proposing a new parameter-free benchmark we coin RoBIC 25. RoBIC fairly evaluates the robustness of image classifiers using a new half-distortion measure. It gauges the robustness of the network against white and black box attacks, independently of its accuracy. RoBIC is faster than the other available benchmarks. We present the significant differences in the robustness of 16 recent models as assessed by RoBIC. We make this benchmark publicly available for use and contribution on gitlab.8.1.16 Patch Replacement: A Transformation-based Method to Improve Robustness against Adversarial Attacks
Participants: Hanwei Zhang, Yannis Avrithis, Teddy Furon, Laurent Amsaleg.
Deep Neural Networks (DNNs) are robust against intra-class variability of images, pose variations and random noise, but vulnerable to imperceptible adversarial perturbations that are well-crafted precisely to mislead. While random noise even of relatively large magnitude can hardly affect predictions, adversarial perturbations of very small magnitude can make a classifier fail completely. To enhance robustness, we introduce in 34 a new adversarial defense called patch replacement, which transforms both the input images and their intermediate features at early layers to make adversarial perturbations behave similarly to random noise. We decompose images/features into small patches and quantize them according to a codebook learned from legitimate training images. This maintains the semantic information of legitimate images, while removing as much as possible the effect of adversarial perturbations. Experiments show that patch replacement improves robustness against both white-box and gray-box attacks, compared with other transformation-based defenses. It has a low computational cost since it does not need training or fine-tuning the network. Importantly, in the white-box scenario, it increases the robustness, while other transformation-based defenses do not.8.1.17 Efficient Statistical Assessment of Neural Network Corruption Robustness
Participants: Karim Tit [SimSmart], Teddy Furon [SimSmart], Mathias Rousset [SimSmart].
In 30 we quantify the robustness of a trained network to input uncertainties with a stochastic simulation inspired by the field of Statistical Reliability Engineering. The robustness assessment is cast as a statistical hypothesis test: the network is deemed as locally robust if the estimated probability of failure is lower than a critical level. The procedure is based on an Importance Splitting simulation generating samples of rare events. We derive theoretical guarantees that are non-asymptotic w.r.t. sample size. Experiments tackling large scale networks outline the efficiency of our method making a low number of calls to the network function.8.1.18 Évaluation statistique efficace de la robustesse de classifieurs
Participants: Karim Tit [SimSmart], Teddy Furon [SimSmart], Mathias Rousset [SimSmart], Louis-Marie Traonouez [Thalès, Rennes].
Nous proposons de quantifier la robustesse d'un classifieur aux incertitudes d'entrée avec une simulation stochastique 31. L'évaluation de la robustesse est présentée comme un test d'hypothèse : le classifieur est considéré comme localement robuste si la probabilité de défaillance estimée est inférieure à un niveau critique. La procédure est basée sur une simulation d'Importance Splitting générant des échantillons d'événements rares. Nous dérivons des garanties théoriques non-asymptotiques par rapport à la taille de l'échantillon. Des expériences portant sur des classifieurs à grande échelle mettent en évidence l'efficacité de notre méthode.8.1.19 Probabilistic forecasting of seasonal time series Combining clustering and classification for forecasting
Participants: Colin Leverger [Orange, Rennes], Thomas Guyet [AgroCampus Ouest, Rennes], Simon Malinowski [Orange, Lannion], Vincent Lemaire [Orange, Lannion], Alexis Bondu [Orange, Paris], Laurence Rozé [Lacodam], Alexandre Termier [Lacodam], Régis Marguerie [Orange, Rennes].
In this article (22), we propose a framework for seasonal time series probabilistic forecasting. It aims at forecasting (in a probabilistic way) the whole next season of a time series, rather than only the next value. Probabilistic forecasting consists in forecasting a probability distribution function for each future position. The proposed framework is implemented combining several machine learning techniques 1) to identify typical seasons and 2) to forecast a probability distribution of the next season. This framework is evaluated using a wide range of real seasonal time series. On the one side, we intensively study the alternative combinations of the algorithms composing our framework (clustering, classification), and on the other side, we evaluate the framework forecasting accuracy. As demonstrated by our experiences, the proposed framework outperforms competing approaches by achieving lower forecasting errors.8.1.20 Impact of Data Cleansing for Urban Bus Commercial Speed Prediction
Participants: Gauthier Lyan [Diverse], David Gross-Amblard [Druid], Jean-Marc Jézéquel [Diverse], Simon Malinowski.
Les systèmes d'information pour les transports publics (SITP) sont largement répandus et utilisés par les services de bus publics dans nombre de villes à travers le monde. Ces systèmes recueillent des informations sur les trajets, les arrêts de bus, la vitesse des bus, la fréquentation, etc. Ces données massives constituent une source d'information intéressante pour les outils prédictifs d'apprentissage automatique. Cependant, elles souffrent le plus souvent de déficiences qualitatives, dues à des ensembles de données multiples aux structures multiples, à des infrastructures différentes utilisant des technologies incompatibles, à des erreurs humaines ou à des défaillances matérielles. Dans cet article (8), nous examinons l'impact du nettoyage des données sur une tâche classique d'apprentissage automatique : la prédiction de la vitesse commerciale des bus urbains. Nous montrons que des règles de gestion et de qualité simples et spécifiques au transport peuvent améliorer considérablement la qualité des données, alors que des règles plus sophistiquées tendent à offrir peu d'améliorations tout en ayant un coût de calcul élevé.8.1.21 A survey on training and evaluation of word embeddings
Participants: François Torregrossa [SoLocal, Boulogne-Billancourt], Robin Allesiardo [SoLocal, Boulogne-Billancourt], Vincent Claveau [SoLocal, Boulogne-Billancourt], Nihel Kooli [SoLocal, Boulogne-Billancourt], Guillaume Gravier.
Word Embeddings have proven to be effective for many Natural Language Processing tasks by providing word representations integrating prior knowledge. In this article, we focus on the algorithms and models used to compute those representations and on their methods of evaluation. Many new techniques were developed in a short amount of time and there is no unified terminology to emphasize strengths and weaknesses of those methods. Based on the state of the art, we propose a thorough terminology to help with the classification of these various models and their evaluations. We also provide comparisons of those algorithms and methods, highlighting open problems and research paths, as well as a compilation of popular evaluation metrics and datasets. This survey (10) gives: 1) an exhaustive description and terminology of currently investigated word embeddings, 2) a clear segmentation of evaluation methods and their associated datasets, and 3) high-level properties to indicate pros and cons of each solution.8.1.22 Unsupervised Tree Extraction in Embedding Spaces for Taxonomy Induction
Participants: François Torregrossa [SoLocal, Boulogne-Billancourt], Robin Allesiardo [SoLocal, Boulogne-Billancourt], Vincent Claveau, Guillaume Gravier.
Exposing latent structure (graph, tree...) of data is a major challenge to deal with the web of data. Today's embedding techniques incorporate any data source (noisy graphs, item similarities, plain text) into continuous vector spaces that are typically used as input to classifier. In 32, we are dealing with the opposite task: finding structures (taxonomies) from embedded data. We provide an original unsupervised methodology for taxonomy induction by directly searching for graph structures preserving pairwise distances between items. Contrary to the state-of-the-art (SOTA), our approach does not require to train classifiers; it is also more versatile as it can be applied to any embedding (eg. word embedding, similarity embedding like space-time local embedding...). On standard benchmarks and metrics, our approach yields SOTA performance. As another contribution, we propose better evaluation metrics for taxonomy induction, leveraging graph kernel similarities and edit distance, showing that the structures of our predicted taxonomies are significantly closer to the ground-truth than SOTA solutions.8.1.23 Active Learning for Interactive Relation Extraction in a French Newspaper’s Articles
Participants: Cyrielle Mallart [Sipa Ouest-France, Rennes], Michel Le Nouy [Sipa Ouest-France, Rennes], Guillaume Gravier, Pascale Sébillot.
Relation extraction is a subtask of natural language processing that has seen many improvements in recent years, with the advent of complex pre-trained architectures. Many of these state-of-the-art approaches are tested against benchmarks with labelled sentences containing tagged entities, and require important pretraining and fine-tuning on task-specific data. However, in a real use-case scenario such as in a newspaper company mostly dedicated to local information, relations are of varied, highly specific type, with virtually no annotated data for such relations, and many entities co-occur in a sentence without being related. We question the use of supervised state-of-the-art models in such a context, where resources such as time, computing power and human annotators are limited. To adapt to these constraints, we experiment with an active-learning based relation extraction pipeline, consisting of a binary LSTM-based lightweight model for detecting the relations that do exist, and a state-of-the-art model for relation classification. We compare several choices for classification models in this scenario, from basic word embedding averaging, to graph neural networks and Bert-based ones, as well as several active learning acquisition strategies, in order to find the most cost-efficient yet accurate approach in our French largest daily newspaper company's use case (27).8.2 Accessing Information
8.2.1 HyperStorylines: Interactively untangling dynamic hypergraphs – GO TO ACCESSING
Participants: Vanessa Pena Araya [Ilda], Tong Xue [Ilda], Emmanuel Pietriga [Ilda], Laurent Amsaleg [Ilda], Anastasia Bezerianos [Ilda].
We present in 9 the design and evaluation of HyperStorylines, a technique that generalizes Storylines to visualize the evolution of relationships involving multiple types of entities such as, for example, people, locations, and companies. Datasets which describe such multi-entity relationships are often modeled as hypergraphs, that can be difficult to visualize, especially when these relationships evolve over time. HyperStorylines builds upon Storylines, enabling the aggregation and nesting of these dynamic, multi-entity relationships. We report on the design process of HyperStorylines, which was informed by discussions and workshops with data journalists; and on the results of a comparative study in which participants had to answer questions inspired by the tasks that journalists typically perform with such data. We observe that although HyperStorylines takes some practice to master, it performs better for identifying and characterizing relationships than the selected baseline visualization (PAOHVis) and was preferred overall.8.2.2 Generating artificial texts for query expansion
Participant: Vincent Claveau.
A well-known way to improve the performance of document retrieval is to expand the user's query. Several approaches have been proposed in the literature, and some of them are considered as yielding state-of-the-art results. In this series of papers (15, 16, 45), we explore the use of text generation to automatically expand the queries. We rely on a well-known neural generative model, GPT-2, that comes with pre-trained models for English but can also be fine-tuned on specific corpora. Through different experiments, we show that text generation is a very effective way to improve the performance of an IR system, with a large margin (+10% MAP gains), and that it outperforms strong baselines also relying on query expansion (LM+RM3). This conceptually simple approach can easily be implemented on any IR system thanks to the availability of GPT code and models.8.2.3 Generating artificial texts as substitution or complement of training data
Participants: Vincent Claveau, Antoine Chaffin, Ewa Kijak.
The quality of artificially generated texts has considerably improved with the advent of transformers. The question of using these models to generate learning data for supervised learning tasks naturally arises. In this series of papers 14, 44, this question is explored under 3 aspects: (i) are artificial data an efficient complement? (ii) can they replace the original data when those are not available or cannot be distributed for confidentiality reasons? (iii) can they improve the explainability of classifiers? Different experiments are carried out on Web-related classification tasks – namely sentiment analysis on product reviews and Fake News detection – using artificially generated data by fine-tuned GPT-2 models. The results show that such artificial data can be used in a certain extend but require pre-processing to significantly improve performance. We show that bag-of-word approaches benefit the most from such data augmentation.
8.2.4 PPL-MCTS: Constrained Textual Generation Through Discriminator-Guided Decoding
Participants: Vincent Claveau, Antoine Chaffin, Ewa Kijak.
Large pre-trained language models (LM) based on Transformers allow to generate very plausible long texts. In this series of papers 35, 43, we explore how this generation can be further controlled to satisfy certain constraints (eg. being non-toxic, positive or negative, convey certain emotions, etc.) without fine-tuning the LM. Precisely, we formalize constrained generation as a tree exploration process guided by a discriminator that indicates how well the associated sequence respects the constraint. Using a discriminator to guide this generation, rather than fine-tuning the LM, in addition to be easier and cheaper to train, allows to apply the constraint more finely and dynamically. We propose several original methods to search this generation tree, notably the Monte Carlo Tree Search (MCTS) which provides theoretical guarantees on the search efficiency, but also simpler methods based on re-ranking a pool of diverse sequences using the discriminator scores. These methods are evaluated on two types of constraints and languages: review polarity and emotion control in French and English. We show that MCTS achieves state-of-the-art results in constrained generation, without having to tune the language model, in both tasks and languages. We also demonstrate that our other proposed methods based on re-ranking can be really effective when diversity among the generated propositions is encouraged.
8.2.5 A Study of the Plausibility of Attention between RNN Encoders in Natural Language Inference
Participants: Duc Hau Nguyen, Guillaume Gravier, Pascale Sébillot.
Attention maps in neural models for NLP are appealing to explain the decision made by a model, hopefully emphasizing words that justify the decision. While many empirical studies hint that attention maps can provide such justification from the analysis of sound examples, only a few assess the plausibility of explanations based on attention maps, i.e., the usefulness of attention maps for humans to understand the decision. These studies furthermore focus on text classification. In this paper (28), we report on a preliminary assessment of attention maps in a sentence comparison task, namely natural language inference. We compare the cross-attention weights between two RNN encoders with human-based and heuristic-based annotations on the eSNLI corpus. We show that the heuristic reasonably correlates with human annotations and can thus facilitate evaluation of plausible explanations in sentence comparison tasks. Raw attention weights however remain only loosely related to a plausible explanation.8.2.6 Understanding the phenomenology of reading through modeling
Participants: Alessio Antonini [OU - The Open University, Milton Keynes], Mari Carmen Suárez-Figueroa [OEG - Ontology Engineering Group, Madrid], Alessandro Adamou [INSIGHT - Insight Centre for Data Analytics, Galway], Francesca Benatti [OU - The Open University, Milton Keynes], François Vignale [3L.AM - Langues, Littératures, Linguistique des universités d'Angers et du Mans], Guillaume Gravier [University of Turin], Lucia Lupi [University of Turin].
Large scale cultural heritage datasets and computational methods for the humanities research framework are the two pillars of Digital Humanities, a research field aiming to expand humanities studies beyond specific sources and periods to address macroscope research questions on broad human phenomena. In this regard, the development of machine-readable semantically enriched data models based on a cross-disciplinary "language" of phenomena is critical for achieving the interoperabil-ity of research data. This contribution reports, documents, and discusses the development of a model for the study of reading experiences as part of the EU JPI-CH project Reading Europe Advanced Data Investigation Tool (READ-IT). Through the discussion of the READ-IT ontology of reading experience, this contribution will highlight and address three challenges emerging from the development of a conceptual model for the support of research on cultural heritage. Firstly, this contribution (7) addresses modelling for multidisciplinary research. Secondly, this work addresses the development of an ontology of reading experience, under the light of the experience of previous projects, and of ongoing and future research developments. Lastly, this contribution addresses the validation of a conceptual model in the context of ongoing research, the lack of a consolidated set of theories and of a consensus of domain experts.8.2.7 Utilisation d'approches automatiques pour la reconnaissance des expériences de lecture–"Je pense que ça traite d'expérience de lecture, à voir ... " : retour sur une expérience d'annotation collaborative
Participants: Guillaume Le Noé Bienvenu [3L.AM - Langues, Littératures, Linguistique des universités d'Angers et du Mans], François Vignale [3L.AM - Langues, Littératures, Linguistique des universités d'Angers et du Mans], Guillaume Gravier, Pascale Sébillot.
Ces communications (21, 33) ont pour but de présenter le rôle des techniques relevant de l'intelligence artificielle et du traitement du langage naturel dans la mise au point d'algorithmes de détection semi-automatique des expériences de lecture développés dans le cadre du projet READ-IT. Au cours des dernières décennies, les connaissances sur l'histoire des pratiques de lecture ont considérablement augmenté au sujet des usages et des habitudes mais des questions fondamentales demeurent, telles que le "pourquoi" et le "comment" on lit. Grâce à l'exploration de sources numériques à la recherche de témoignages d'expériences de lecture, le projet READ-IT (Reading Europe Advanced Data Investigation Tool) vise à mieux comprendre ces phénomènes. Ce projet financé par le Joint Programming Initiative for Cultural Heritage (2018-2021) associe 5 partenaires de 4 pays (France, Royaume-Uni, Pays-Bas, République Tchèque).
9 Bilateral contracts and grants with industry
9.1 Bilateral contracts with industry
CIFRE PhD: Incremental dynamic construction of knowledge bases from text mining
Participants: Guillaume Gravier, Cyrielle Mallart, Pascale Sébillot.
Duration: 3 years, started in Dec. 2018
CIFRE PhD: Embedding heterogeneous data for directory search
Participants: Vincent Claveau, Guillaume Gravier, François Torregrossa.
Duration: 3 years, started in Dec. 2018
CIFRE PhD: Few shot learning for object recognition in aerial images
Participants: Yannis Avrithis, Yann Lifchitz.
Duration: 3 years, started in March 2018
CIFRE PhD: Deep Learning and Homomorphic encryption
Participants: Teddy Furon, Samuel Tap.
Duration: 3 years, started in December 2020
CIFRE PhD: Robustness of machine learning against uncertainties
Participants: Teddy Furon, Mathias Rousset, Karim Tit.
Duration: 3 years, started in December 2020
CIFRE PhD: Semantic multimodal question answering (MQA) in domestic environments
Participants: Yannis Avrithis, Teddy Furon, Deniz Engin.
Duration: 3 years, started in September 2020
CIFRE PhD: Multimodal detection of fake news
Participants: Vincent Claveau, Ewa Kijak, Antoine Chaffin.
Duration: 3 years, started in November 2020
10 Partnerships and cooperations
10.1 International initiatives
10.1.1 Associate Teams in the framework of an Inria International Lab or in the framework of an Inria International Program
LOGIC
-
Title:
Learning on graph-based hierarchical methods for image and multimedia data
-
Duration:
2020 to 2022, possibly extended due to Covid
-
Coordinator:
Silvio Jamil Guimaraes (sjamil@pucminas.br)
-
Partners:
- Pontifícia Universidade Católica de Minas Gerais
-
Inria contact:
Simon Malinowski
-
Summary:
The main goal of this associate team is related to learning graph-based hierarchical methods to be applied on image and multimedia data. Regarding image data, we aim at advancing in the state-of-the-art on hierarchy of partitions taking into account aspects of efficiency, quality, hierarchies and interactivity, as well as the use of hierarchical information to help the information extraction process. Research on graph-based multimedia label/information propagation will be developed within this project along two main lines of research : (i) construction and learning of multimedia graphs and hierarchies and (ii) use of these structures to propagate information.
10.1.2 Participation in other International Programs
HIMMD
-
Title:
Hierarchical Graph-based Analysis of Image, Video and Multimedia Data
-
Partner Institution(s):
- Pontifícia Universidade Católica de Minas Gerais, Brazil
- Universidade Federal de Minas Gerais, Brazil
- Universidade Estadual de Campinas, Brazil
- LIGM/ESIEE, France
- Grenoble INP, France
- IRISA, France
-
Date/Duration:
2019–2022
10.2 European initiatives
10.2.1 Other european programs/initiatives
JPI CH READ-IT (Joint Programming Initiative on Cultural Heritage)
-
Title:
READ-IT (Reading Europe Advanced Data Investigation Tool)
-
Duration:
3.5 years, started in May 2018
-
Coordinator:
Brigitte Ouvry-Vial (brigitte.ouvry-vial@univ-lemans.fr)
-
Partners:
- Le Mans University (FR)
- CNRS-IRISA (FR)
- Open University (UK)
- Universiteit Utrecht (NL)
- Institute of Czech Litterature (CZ)
-
Inria contact:
Guillaume Gravier
-
Participants:
Vincent Claveau, Guillaume Gravier, Ewa Kijak, Suresh Kirthi Kumaraswamy, Guillaume Le Noé-Bienvenu, Pascale Sébillot
-
Summary:
READ-IT is a transnational, interdisciplinary R&D project that will build a unique large-scale, user- friendly, open access, semantically-enriched investigation tool to identify and share groundbreaking evidence about 18th-21st century Cultural Heritage of reading in Europe. READ-IT will ensure the sustainable and reusable aggregation of qualitative data allowing an in-depth analysis of the Cultural Heritage of reading. State-of-the art technology in Semantic Web and information systems will provide a versatile, end-users oriented environment enabling scholars and ordinary readers to retrieve information from a vast amount of community-generated digital data leading to new understanding about the circumstances and effects of reading in Europe.
BigScience.
LinkMedia is involved in a large international initiative aiming at building and exploring the multiple aspects of very large neural generative models. More than 600 researchers (academics and companies) are involved and a large amount of GPU hours have been granted on the Jean Zay supercomputer. LinkMedia participates in the prompt engineering and evaluation sub-tasks. More info on BigScience.
10.3 National initiatives
Chaire Security of AI for Defense Applications (SAIDA)
Participants: Teddy Furon [WIDE], Laurent Amsaleg [WIDE], Erwan Le Merrer [WIDE], Mathias Rousset [SIMSMART], Benoit Bonnet [CRIStAL - Centre de Recherche en Informatique, Signal et Automatique de Lille - UMR 9189], Thibault Maho [CRIStAL - Centre de Recherche en Informatique, Signal et Automatique de Lille - UMR 9189], Patrick Bas [CRIStAL - Centre de Recherche en Informatique, Signal et Automatique de Lille - UMR 9189], Samuel Tap, Karim Tit.
Duration: 4 years, started Sept 2020
Inria Project Lab Knowledge-driven data and content collaborative analytics (iCODA)
Participants: Laurent Amsaleg, Cheikh Brahim El Vaigh, Guillaume Gravier, Cyrielle Mallart, Pascale Sébillot.
Duration: 4.5 years, started in April 2017
ANR Archival: Multimodal machine comprehension of language for new intelligent interfaces of scientific and cultural mediation
Participants: Laurent Amsaleg, Guillaume Gravier, Duc Hau Nguyen, Pascale Sébillot.
Duration: 3.5 year, started in Dec. 2019
The multidisciplinary and multi-actor ARCHIVAL project aims at yielding collaborations between researchers from the fields of Information and Communication Sciences as well as Computer Sciences around archive value enhancing and knowledge sharing for arts, culture and heritage. The project is structured around the following questionings: What part can machine comprehension methods play towards the reinterpretation of thematic archive collections? How can content mediation interfaces exploit results generated by current AI approaches?
ARCHIVAL teams will explore heterogeneous document collection structuration in order to explicitly reveal implicit links, to explain the nature of these links and to promote them in an intelligible way towards ergonomic mediation interfaces that will guarantee a successful appropriation of contents. A corpus has been delimited from the FMSH “self-management” collection, recently awarded as Collex, which will be completed from the large Canal-U academic audiovisual portal. The analysis and enhancement of this collection is of particular interest for Humanities and Social Sciences in a context where it becomes a necessity to structurally reconsider new models of socioeconomic development (democratic autonomy, social and solidarity-based economy, alternative development,…).
ANR MEERQAT: MultimEdia Entity Representation and Question Answering Tasks
Participants: Laurent Amsaleg, Yannis Avrithis, Ewa Kijak, Shashanka Venkataramanan.
Duration: 3.5 year, started in April 2020
The overall goal of the project is to tackle the problem of ambiguities of visual and textual content by learning then combining their representations. As a final use case, we propose to solve a Multimedia Question Answering task, that requires to rely on three different sources of information to answer a (textual) question with regard to visual data as well as an external knowledge base containing millions of unique entities, each being represetd by textual and visual content as well as some links to other entities. An important work will deal with the representation of entities into a common tri-modal space, in which one should determine the content to associate to an entity to adequately represent it. The challenge consists in defining a representation that is compact (for performance) while still expressive enough to reflect the potential links between the entity and a variety of others.
MinArm: EVE3
Participants: Teddy Furon.
Duration: 3 year, started in April 2019
ANR UNLIR: Unsupervised Representation Learning for Image Recognition
Participants: Yannis Avrithis.
Duration: 4 years, started in January 2020
- Complex recognition problems such as fine grained classification (highly similar categories e.g. bird species, airplane/car models, etc.) show that state of the art DNNs are still improved by better objective functions and more discriminative intermediate representations.
- Despite progress in using less annotated data, DNN can hardly cope with learning from few examples.
- DNNs have so many parameters and complex structures that it is extremely hard to understand what happens in every layer in producing the final decision.
This project aims to address these limitations. In particular, we will work towards building networks capable of solving fine-grained visual recognition tasks. We will improve the capabilities of networks to learn from few to no data, building highly discriminative representations that can address complex recognition problems. Following that, we will provide insight on how such models take their decisions.
AID-CNRS: FakeNews
Participants: Vincent Claveau, Ewa Kijak.
Duration: 2 years, started mid-2021
11 Dissemination
11.1 Promoting scientific activities
11.1.1 Scientific events: organisation
General chair, scientific chair
- Vincent Claveau organized a workshop about "NLP for Defense" in the framework of the AID "AI for defense" event, Paris
Member of the organizing committees
- Ewa Kijak and Vincent Claveau co-organized the Madonna workshop from the GdR MaDICS, 2 days, Rennes
- Simon Malinowski co-organized the Workshop on Advanced Learning and Analytics on Temporal Data in September 2021, colocated with ECML/PKDD Conference (virtual event due do health crisis)
11.1.2 Scientific events: selection
Chair of conference program committees
- Guillaume Gravier was area chair for ACM Multimedia
- Laurent Amsaleg was area chair for ACM Multimedia
Member of the conference program committees
- Laurent Amsaleg was part of the program committee of: ACM International Conference on Multimedia, ACM International Conference on Multimedia Retrieval, Multimedia Modeling, IEEE International Conference on Multimedia & Expo, International Conference on Similarity Search and Applications
- Pascale Sébillot was part of the program committee of: Traitement Automatique des Langues Naturelles (TALN)
- Ewa Kijak was part of the program committee of: International Conference on Content-Based Multimedia Indexing
- Yannis Avrithis was part of the programm committee of ICCV, CVPR
- Simon Malinowski was a PC member for Workshop on Advanced Learning and Analytics on Temporal Data in September 2021
Reviewer
- Teddy Furon, as an IEEE IFS TC affiliate, was a reviewer for the Information Forensics and Security track in IEEE conferences like ICASSP, ICIP, and WIFS
- Vincent Claveau was a reviewer for AAAI, ACL, EMNLP, ECIR, TextMine, TALN
- Pascale Sébillot was a reviewer for IEEE International Conference on Machine Learning and Applications (ICMLA)
- Guillaume Gravier was reviewer for ACM Intl. Conf. on Multimedia Retrieval
- Yannis Avrithis was part of the programm committee of IJCV
11.1.3 Journal
Member of the editorial boards
- Pascale Sébillot is editor of the Journal Traitement Automatique des Langues (TAL)
- Pascale Sébillot is member of the editorial board of the Journal Traitement Automatique des Langues (TAL)
- Vincent Claveau is a board member of the journal TAL Traitement Automatique des Langues
- Yannis Avrithis is an associate editor of CVIU
Reviewer - reviewing activities
- Laurent Amsaleg was a reviewer for: IEEE Transactions on Information Forensics and Security, IEEE Transactions on Signal Processing, IEEE Transactions on Dependable and Secure Computing
- Teddy Furon was a reviewer for: IEEE Transactions on Information Forensics and Security, IEEE Transactions on Signal Processing, IEEE Transactions on Image Processing, IEEE Transactions on Dependable and Secure Computing, IEEE Transactions on Circuits and Systems for Video Technology, Journal of Machine Learning
- Vincent Claveau was a reviewer for: journal of Computer Science reviews, Multimedia Tools and Applications, Expert Systems with Applications, TAL Traitement Automatique des Langues
- Pascale Sébillot was a reviewer for: Traitement Automatique des Langues (TAL)
11.1.4 Invited talks
- Teddy Furon, HubDay Data Science & Artificial Intelligence (Pôle de compétitivité Systematic)
- Teddy Furon, UK/FR Defense Sector Trusted AI Workshop organized by DGA/AID and MOD/DSTL
- Teddy Furon, Journées Scientifiques Inria
- Teddy Furon, Journées Nationales du GdR Securité Informatique
- Teddy Furon, Journée annuelle du programme Confiance.ai (IRT SystemX), atelier robustesse de l'IA
- Teddy Furon, Atelier Hands-on Machine Learning for Security, CentraleSupelec Rennes
- Teddy Furon, European CyberWeek, Présentation du Centre de Compétence en Cybersécurité C3
- Teddy Furon, IEEE Workshop on Information Forensics ans Security (keynote speaker)
- Teddy Furon, Internal seminars (Thales, Amazon, Content Armor, Insa Rennes lab VADDER)
- Vincent Claveau presented LinkMedia's work on fake news detection at 2-day multidisciplinary event of Univ. Avignon
- Vincent Claveau presented LinkMedia's work on fake news detection at the IXXI seminar
- Guillaume Gravier, L'IA pour décrire, indexer et explorer des collections multimédias : comment faire sans Google ? Conférence invité d'ouverture du colloque Humanistica
- Ewa Kijak, Manipulation des images, des vidéos et fausses informations : qu’est-ce que l’IA a changé ?, Breizh Data days
- Ewa Kijak, Fake news, Deepfakes : un défi pour la recherche en informatique, Dakar Institute of Technology
- Ewa Kijak, Deep Learning Breakthrough in Computer Vision, Dakar Institute of Technology, Percepts and Concepts seminar, ENS ULM
11.1.5 Leadership within the scientific community
- Laurent Amsaleg is a member of the Steering Committee of ACM Multimedia for the 2020-2023 term
- Pascale Sébillot is a member of the board of the GDR Traitement Automatique des Langues
- Guillaume Gravier is a member of the scientific board of the GDR Traitement Automatique des Langues
- Guillaume Gravier is a referent on AI within Allistene representing the CPU
- Guillaume Gravier is the coordinator of Rennes' AI doctoral program AI4SDA
11.1.6 Scientific expertise
- Teddy Furon was an expert for Pôle d'Excellence en Cybersécurité, IDEX Grenoble CBS-MIAI.
- Teddy Furon was a member of committee ANR ASTRID AAP.
- Vincent Claveau served as expert for ANRT (CIFRE PhD funding)
- Vincent Claveau served as expert for LabEx Digicosme
- Vincent Claveau served as jury member for the AI4SDA PhD funding program
11.1.7 Research administration
- Pascale Sébillot is a deputy director of IRISA UMR 6074 since January 1st, 2021
- Pascale Sébillot is the deputy director of the Scientific Advisory Committee of IRISA UMR 6074
- Pascale Sébillot was a member of the theses advisory committee of the MathSTIC doctoral school until April 2021
- Pascale Sébillot is a member of the board of the MathSTIC doctoral school
- Teddy Furon is a member of Commission du Personnel of IRISA / Inria Rennes Bretagne Atlantique
- Teddy Furon was a member of Commission des Moyens Incitatifs for Inria Rennes Bretagne Atlantique
- Teddy Furon was the head of the Comité de sélection des délégations at Inria Rennes Bretagne Atlantique
- Guillaume Gravier is director of IRISA (UMR 6074) since Jan. 2021
- Guillaume Gravier is a member of the board of the competitvity cluster Images & Réseaux, representing CNRS
- Guillaume Gravier is a member of the AID-CNRS coordination on AI
11.2 Teaching - Supervision - Juries
11.2.1 Teaching
- Licence: Pascale Sébillot, Natural Language Processing, 9h, L3, INSA Rennes, France
- Master: Laurent Amsaleg, Bases de données avancées, 25h, M2, INSA Rennes, France
- Master: Teddy Furon, Rare Event Simulations, 40h, INSA Rennes, France
- Master: Pascale Sébillot, Natural Language Processing, 6h, M1, INSA Rennes, France
- Engineering school: Vincent Claveau, Machine Learning, 18h, 3rd year, INSA Rennes, France
- Master: Vincent Claveau, Information Retrieval, 10h, M2 MIAGE, Univ. Rennes, France
- Engineering school: Vincent Claveau, Natural Language Processing, 12h, ESIR, Univ. Rennes, France
- Licence: Guillaume Gravier, Base de données, 26h, L2, INSA Rennes
- Licence: Guillaume Gravier, Natural language processing, 12h, L3, INSA Rennes
- Licence: Guillaume Gravier, Markov models, 6h, L3, INSA Rennes
- Master: Guillaume Gravier, Natural Language Processing, 6h, M1, INSA Rennes
- Master: Guillaume Gravier, Natural Language Processing, 33h, M2, ENSAI
- Master: Ewa Kijak, Image processing, 45h, M1, ESIR, Univ. Rennes
- Master: Ewa Kijak, Supervised machine learning, 15h, M2R, Univ. Rennes
- Master: Ewa Kijak, Image classification, 27h, M1, ESIR
- Ewa Kijak is head of the Image engineering track (M1-M2) of ESIR, Univ. Rennes
- Master: Ewa Kijak, Image classification, 27h, M1, ESIR
- Master: Yannis Avrithis, Deep learning for vision, 20h, M2 SIF France
- Master: Simon Malinowski, Basics of Data Analytics for Data Science, 24h, EIT Data Science Master 1, Rennes
- Master: Simon Malinowski, Prediction Methods, 30h, M1 MIAGE and Data Science EIT Master 1, Rennes
- Master: Simon Malinowski, Statisical Data Mining, 24h, M2 MIAGE, ISTIC, Rennes
- Master: Simon Malinowski, Symbolic Data Mining, 12h, M2 MIAGE, ISTIC, Rennes
- Simon Malinowski is responsible for the Master 2 MIAGE parcours Classique
- Simon Malinowski is responsible for the M2 studies within the DataScience track of the EIT-digital master school
11.2.2 Supervision
- PhD in progress: Shashanka Venkataramanan, Metric learning for instance- and category-level visual representations. Started in December 2020. Yannis Avrithis, Ewa Kijak & Laurent Amsaleg
- PhD in progress: Benoit Bonnet, Security of DNN against white-box attacks. Started in November 2019. Teddy Furon & Patrick Bas (CNRS CRIStAL Lille)
- PhD in progress: Thibault Maho, Security of DNN against black-box attacks. Started in December 2020. Erwan Le Merrer & Teddy Furon
- PhD in progress: Karim Tit, Assessment of DNN robustness against uncertainties. Started in February 2021. Teddy Furon
- PhD in progress: Samuel Tap, Homomorphic encryption for Machine Learning. Started in March 2021. Teddy Furon
- PhD in progress: Deniz Engin, Video Query Answering in domestic environments. Started in September 2020. Yannis Avrithis & Teddy Furon
- PhD in progress: Cyrielle Mallart, Incremental dynamic construction of knowledge graphs from text mining, started December 2018, Guillaume Gravier, Michel Le Nouy (Ouest-France)& Pascale Sébillot
- PhD in progress: Duc Hau Nguyen, Multimodal space for the generation and justification of semantic links between documents, started September 2020, Guillaume Gravier & Pascale Sébillot
- PhD in progress: Antoine Chaffin, Multimodal Fake News Detection, started in October 2020, Ewa Kijak & Vincent Claveau
- PhD in progress: Mohamed Younes, Learning and simulating strategies in sports for VR training, started in December 2020, Franck Multon (Mimetic), Richard Kulpa (Mimetic), Ewa Kijak, Simon Malinowski
- PhD: Yichang Wang, Interpretable time series classification, defended September 2021, Élisa Fromont (Lacodam), Romain Tavenard (Obélix), Rémi Emonet (Jean Monnet U.), Simon Malinowski
- PhD: Cheikh Brahim El Vaigh, Incremental content to data linking leveraging ontological knowledge in data journalism 39, defended January 2021, Guillaume Gravier, Pascale Sébillot & François Goasdoué (with CEDAR, Inria team)
- PhD: Hanwei Zhang, Deep Learning in Adversarial Contexts 42, defended June 2021, Laurent Amsaleg, Yannis Avrithis & Teddy Furon
- PhD: Marzieh Gheisari Khorasgani, Secure identification in the Internet of Things 40, defended July 2021, Laurent Amsaleg & Teddy Furon
- PhD: Yann Lifchitz, Making the most of available data : representation and adaptation for few-shot image classification 41, defended April 2021, Yannis Avrithis & Sylvaine Picard (Safran)
- PhD: François Torregrossa, Hyperbolic embeddings for the represenation of words and knowledge, defended Dec. 2021, Guillaume Gravier & Vincent Claveau
11.2.3 Juries
- Laurent Amsaleg was a reviewer for Solène Bernard's PhD committee (Centrale Lille. Oct 2021)
- Teddy Furon was a reviewer for Alexandre Araujo's PhD committee (Univ. Paris Dauphine P.S.L. June 2021)
- Teddy Furon was a reviewer for Rémi Bernhard's PhD committee (Mines de St Etienne. Nov 2021)
- Teddy Furon was a member of Solène Bernard's PhD committee (Centrale Lille. Oct 2021)
- Vincent Claveau was a reviewer for Perceval Wajsburt's PhD committee (Sorbonne Université)
- Vincent Claveau was a member of Marwa Elleuch'PhD committee (Institut Polytechnique de Paris)
- Pascale Sébillot was president of Antoine Perquin's PhD committee (Université de Rennes 1).
- Pascale Sébillot was a reviewer of Aman Behre's PhD committee (Université Paris-Saclay)
- Pascale Sébillot was a reviewer of Michael Filhol's habilitation (HDR) committee (Université Paris-Saclay)
- Guillaume Gravier was reviewer for Sebastian Ferrada's PhD (UChile, Santiago de Chile)
- Guillaume Gravier was president of the jury of Hugo Talibart's PhD (Université de Rennes 1)
- Ewa Kijak was a member of Florent Guiotte's PhD committee (Université de Rennes 2)
- Ewa Kijak was a member of Sanae Bourtafass's PhD committee (Université de La Rochelle)
11.3 Popularization
11.3.1 Internal or external Inria responsibilities
Linkmedia was representing the lab IRISA and the Inria research center at "La Fête de la Science" during a week in October 2021 at Les Champs Libres, Rennes.
Vincent Claveau was in charge of organizing the first popularization event of the GdR TAL about recent advances in NLP.
11.3.2 Articles and contents
Rennes – une IA souveraine au service de la vie publique 47, Contribution au Bulletin de l'Association Française pour l'Intelligence Artificielle, AFIA.
Participants: Guillaume Gravier [Lacodam], Elisa Fromont [Lacodam], Nicolas Courty [Obélix], Teddy Furon [Sirocco], Christine Guillemot [Sirocco], Paolo Robuffo Giordano [Rainbow].
11.3.3 Interventions
Vincent Claveau was interviewed about fake news and deepfake detection by the RTS and Data Analytics Post
Ewa Kijak was interviewed about IA in fake news and deepfake detection by the RFI, Data Analytics Post, and Science et vie
Guillaume Gravier gave a webinar on AI for the competivity cluster Images & Réseaux
Laurent Amsaleg participated to two panels during the "DigitalTech" series of conferences about Fake News and Deep Fakes.
12 Scientific production
12.1 Major publications
- 1 articleHigh Intrinsic Dimensionality Facilitates Adversarial Attack: Theoretical Evidence.IEEE Transactions on Information Forensics and Security16September 2020, 1-12
- 2 articleGenerating Adversarial Images in Quantized Domains.IEEE Transactions on Information Forensics and SecurityDecember 2021
- 3 inproceedingsIndiscriminateness in representation spaces of terms and documents.ECIR 2018 - 40th European Conference in Information Retrieval10772LNCSGrenoble, FranceSpringerMarch 2018, 251-262
- 4 inproceedingsEfficient Diffusion on Region Manifolds: Recovering Small Objects with Compact CNN Representations.2017 IEEE Conference on Computer Vision and Pattern Recognition (CVPR)Honolulu, United StatesJuly 2017
- 5 inproceedingsSurFree: a fast surrogate-free black-box attack.CVPR 2021 - Conference on Computer Vision and Pattern RecognitionProc. of {IEEE} Conference on Computer Vision and Pattern Recognition, {CVPR}Virtual, FranceJune 2021, 10430--10439
- 6 articleA Crossmodal Approach to Multimodal Fusion in Video Hyperlinking.IEEE MultiMedia2522018, 11-23
12.2 Publications of the year
International journals
- 7 articleUnderstanding the phenomenology of reading through modelling.Semantic Web – Interoperability, Usability, Applicability122January 2021, 191-217
- 8 articleImpact of Data Cleansing for Urban Bus Commercial Speed Prediction.Springer Nature Computer ScienceNovember 2021, 1-11
- 9 articleHyperStorylines: Interactively untangling dynamic hypergraphs.Information VisualizationSeptember 2021, 1-21
- 10 articleA survey on training and evaluation of word embeddings.International Journal of Data Science and Analytics112February 2021, 85-103
- 11 articleTraining Object Detectors from Few Weakly-Labeled and Many Unlabeled Images.Pattern Recognition120December 2021, 108164
International peer-reviewed conferences
- 12 inproceedingsForensics Through Stega Glasses: the Case of Adversarial Images.ICPR-MMForWILD 2020 - Workshop MultiMedia FORensics in the WILDPattern Recognition: ICPR International Workshops and ChallengesMilan, ItalySpringer International PublishingJanuary 2021, 1-17
- 13 inproceedingsAsymmetric metric learning for knowledge transfer.CVPR 2021 - IEEE/CVF Conference on Computer Vision and Pattern RecognitionVirtual, FranceIEEEJune 2021, 8224-8234
- 14 inproceedingsLa génération de textes artificiels en substitution ou en complément de données d’apprentissage.Actes de la 28e Conférence sur le Traitement Automatique des Langues Naturelles : conférence principaleTALN 2021 - 28e Conférence sur le Traitement Automatique des Langues Naturelles1Lille, FranceATALA2021, 37-49
- 15 inproceedingsGenerating artificial texts for query expansion.Actes de la conférence CORIA 2021CORIA 2021 - Conférence en Recherche d’Information et ApplicationsGrenoble, FranceApril 2021, 1-16
- 16 inproceedingsNeural text generation for query expansion in information retrieval.WI-IAT 2021 - 20th IEEE/WIC/ACM International Conference on Web Intelligence and Intelligent Agent TechnologyProceedings of the WI-IAT ConferenceMelbourne, AustraliaIEEEDecember 2021, 1-8
- 17 inproceedingsDetecting Fake News Conspiracies with Multitask and Prompt-Based Learning.MediaEval 2021 - MediaEval Multimedia Evaluation benchmark. WorkshopOnline, NetherlandsDecember 2021, 1-3
- 18 inproceedingsOn the hidden treasure of dialog in video question answering.ICCV 2021 - IEEE/CVF International Conference on Computer VisionVirtual, FranceOctober 2021
- 19 inproceedingsDetecting Human-Object Interaction with Mixed Supervision.WACV 2021 - Winter Conference on Applications of Computer VisionWaikoloa / Virtual, United StatesJanuary 2021, 1-10
- 20 inproceedingsIterative label cleaning for transductive and semi-supervised few-shot learning.ICCV 2021 - IEEE/CVF International Conference on Computer VisionVirtual, FranceOctober 2021
- 21 inproceedingsUtilisation d'approches automatiques pour la reconnaissance des expériences de lecture.Humanistica 2021 - Colloque de l'Association francophone des humanités numériquesProceedings of Humanistica 202Rennes, FranceMay 2021, 81-83
- 22 inproceedingsProbabilistic forecasting of seasonal time series Combining clustering and classification for forecasting.ITISE 2021 - 7th International Conference on Time Series and ForecastingGran Canaria, SpainJuly 2021, 1-13
- 23 inproceedingsFew-Shot Few-Shot Learning and the role of Spatial Attention.ICPR 2020 - 25th International Conference on Pattern RecognitionVirtual, ItalyJanuary 2021, 1-7
- 24 inproceedingsLocal Propagation for Few-Shot Learning.ICPR 2020 - 25th International Conference on Pattern RecognitionVirtual, ItalyJanuary 2021
- 25 inproceedingsRoBIC: A benchmark suite for assessing classifiers robustness.ICIP 2021 - IEEE International Conference on Image ProcessingAnchorage, Alaska, United StatesIEEESeptember 2021, 1-5
- 26 inproceedingsSurFree: a fast surrogate-free black-box attack.CVPR 2021 - Conference on Computer Vision and Pattern RecognitionProc. of {IEEE} Conference on Computer Vision and Pattern Recognition, {CVPR}Virtual, FranceJune 2021, 10430--10439
- 27 inproceedingsActive Learning for Interactive Relation Extraction in a French Newspaper's Articles.RANLP 2021 - Recent Advances in Natural Language ProcessingProceedings of International Conference Recent Advances in Natural Language Processing RANLP 2021Online, BulgariaSeptember 2021, 886-894
- 28 inproceedingsA Study of the Plausibility of Attention between RNN Encoders in Natural Language Inference.ICMLA 2021 - 20th IEEE International Conference on Machine Learning and ApplicationsPasadena, United StatesIEEEDecember 2021, 1-7
- 29 inproceedingsRethinking deep active learning: Using unlabeled data at model training.International Conference on Pattern RecognitionMilan, ItalyJanuary 2021
- 30 inproceedingsEfficient Statistical Assessment of Neural Network Corruption Robustness.NeurIPS 2021 - 35th Conference on Neural Information Processing Systems34Advances in Neural Information Processing Systems proceedingsSydney (virtual), AustraliaDecember 2021
- 31 inproceedingsÉvaluation statistique efficace de la robustesse de classifieurs.CAID 2021 - Conference on Artificial Intelligence for DefenseActes de la conférence CAID 2021Rennes, FranceNovember 2021, 1-11
- 32 inproceedingsUnsupervised Tree Extraction in Embedding Spaces for Taxonomy Induction.WI-IAT 2021 - 20th IEEE/WIC/ACM International Conference on Web Intelligence and Intelligent Agent TechnologyProceedings of the WI-IAT ConferenceMelbourne, AustraliaIEEEDecember 2021, 1-8
- 33 inproceedings" Je pense que ça traite d'expérience de lecture,à voir ... " : retour sur une expérience d'annotation collaborative.Humanistica 2021 - Colloque annuel de l'association francophone des humanités numériquesProceedings of Humanistica 2021Rennes, FranceMay 2021, 84-85
- 34 inproceedingsPatch Replacement: A Transformation-based Method to Improve Robustness against Adversarial Attacks.ACM Multimedia, Trustworthy AI WorkshopTrustworthy AI 2021 - 1st International Workshop on Trustworthy AI for Multimedia ComputingVirtual, ChinaACMOctober 2021, 1-10
Conferences without proceedings
- 35 inproceedingsPPL-MCTS: Constrained Textual Generation Through Discriminator-Guided Decoding.CtrlGen 2021 - Workshop on Controllable Generative Modeling in Language and Vision at NeurIPS 2021virtual, United StatesDecember 2021, 1-19
Scientific book chapters
- 36 inbookTraçage de traîtres.1Sécurité multimédia. Authentification et insertion de données cachéesISTEJune 2021, 201-228
- 37 inbookAttaques et défenses de réseaux de neurones profonds : le cas de la classification d'images.Sécurité multimédia 1. Authentification et insertion de données cachéesJune 2021
Edition (books, proceedings, special issue of a journal)
- 38 proceedingsF.François De VieillevilleS.Stéphane MayA.Adrien LagrangeA.A DupuisR.Rosa RuilobaF.Fred Ngolè MboulaT.Tristan Bitard-FeildelE.Erwan NoguesC.Corentin LarrocheJ.Johan MazelS.Stephan ClémençonR.Romain BurgotA.Alric GaurierL.Louis HulotL.Léo Isaac-DogninL.Laetitia LeichtnamE.Eric TotelN.Nicolas PrigentL.Ludovic MéR.Rémi BernhardP.-A.Pierre-Alain MoëllicJ.-M.Jean-Max DUTERTREK.Katarzyna KapustaV.Vincent ThouvenotO.Olivier BettanT.Tristan CharrierL.Luc BonnafouxF.-P.Francisco-Pierre PuigQ.Quentin LhoestT.Thomas RenaultA.Adrien BenamiraB.Benoit BonnetT.Teddy FuronP.Patrick BasB.Benjamin FarcyS.Silvia Gil-CasalsJ.Juliette MattioliM.Marc FiammanteM.Marc LambertR.Roman BressonJ.Johanne CohenE.Eyke HullermeierC.Christophe LabreucheM.Michele SebagT.Thomas ThebaudA.Anthony LarcherG.Gaël Le LanN.Nouredine NourR.Reda Belhaj-SoullamiC. L.Cédric L.R. BuronA.Alain PeresF.Frédéric BarbarescoA.Antoine d’AcremontG.Guillaume QuinA.Alexandre BaussardR.Ronan FabletL.Louis Morge-RolletF.Frederic Le RoyD.Denis Le JeuneR.Roland GautierB.Benjamin CamusE.Eric MonteuxM.Mikaël VermetA.Alex GoupilleauT.Tugdual CeillierM.-C.Marie-Caroline CorbineauProceedings of the Conference on Artificial Intelligence for Defence 2020.CAID 2020 - Second Conference on Artificial Intelligence for DefenceRennes, FranceApril 2021
Doctoral dissertations and habilitation theses
- 39 thesisContent and data linking leveraging ontological knowledge in data journalism.Université Rennes 1January 2021
- 40 thesisSecure identification for the Internet of Things.Inria Rennes - Bretagne AtlantiqueJuly 2021
- 41 thesisMaking the most of available data : representation and adaptation for few-shot image classification.Université Rennes 1April 2021
- 42 thesisDeep Learning in Adversarial Context.École normale supérieure de RennesJune 2021
Reports & preprints
- 43 miscGenerating texts under constraint through discriminator-guided MCTS.November 2021
- 44 miscGenerating artificial texts as substitution or complement of training data.November 2021
- 45 miscQuery expansion with artificially generated texts.November 2021
-
46
miscNote: An alternative proof of the vulnerability of
-NN classifiers in high intrinsic dimensionality regions.January 2021
Other scientific publications
- 47 articleRennes - une IA souveraine au service de la vie publique.Bulletin de l'Association Française pour l'Intelligence Artificielle2021
12.3 Other
Scientific popularization
- 48 inproceedingsIt Takes Two to Tango: Mixup for Deep Metric Learning.ICLR 2022 - 10th International Conference on Learning RepresentationsVirtual, FranceApril 2022, 1-21
12.4 Cited publications
- 49 inproceedingsThe Vulnerability of Learning to Adversarial Perturbation Increases with Intrinsic Dimensionality.WIFS2017
- 50 inproceedingsEstimating Local Intrinsic Dimensionality.KDD2015
- 51 articlePrototyping a Web-Scale Multimedia Retrieval Service Using Spark.ACM TOMCCAP143s2018
- 52 inproceedingsScalability of the NV-tree: Three Experiments.SISAP2018
- 53 inproceedingsSketching techniques for very large matrix factorization.ECIR2016
- 54 inproceedingsConstraint Satisfaction Programming for Video Summarization.ISM2013
- 55 articleWild Patterns: Ten Years After the Rise of Adversarial Machine Learning.Pattern Recognition2018
- 56 phdthesisImage indexing and retrieval using component trees.Université de Bretagne Sud2016
- 57 phdthesisA storytelling machine? : Automatic video summarization: the case of TV series.University of Avignon, France2016
- 58 inproceedingsA Study on Multimodal Video Hyperlinking with Visual Aggregation.ICME2018
- 59 inproceedingsTime Series Retrieval using DTW-Preserving Shapelets.SISAP2018
- 60 articleAudio Adversarial Examples: Targeted Attacks on Speech-to-Text.CoRRabs/1801.019442018
- 61 inproceedingsNumerical eligibility criteria in clinical protocols: annotation, automatic detection and interpretation.AIME2017
- 62 inproceedingsImage Retrieval with Reciprocal and shared Nearest Neighbors.VISAPP2014
- 63 inproceedingsUsing Knowledge Base Semantics in Context-Aware Entity Linking.DocEng 2019 - 19th ACM Symposium on Document EngineeringBerlin, GermanyACMSeptember 2019, 1-10
- 64 bookPhoto Forensics.The MIT Press2016
- 65 articleRecent automatic text summarization techniques: a survey.Artif. Intell. Rev.4712017
- 66 bookDeep Learning.MIT Press2016
- 67 inproceedingsShaping-Up Multimedia Analytics: Needs and Expectations of Media Professionals.MMM, Special Session Perspectives on Multimedia Analytics2016
- 68 inproceedingsScaling Group Testing Similarity Search.ICMR2016
- 69 inproceedingsMining on Manifolds: Metric Learning without Labels.CVPR2018
- 70 inproceedingsA Multi-Dimensional Data Model for Personal Photo Browsing.MMM2015
- 71 inproceedingsTen Research Questions for Scalable Multimedia Analytics.MMM, Special Session Perspectives on Multimedia Analytics2016
- 72 articleDeep Video Portraits.ACM TOG2018
- 73 inproceedingsActive Learning to Assist Annotation of Aerial Images in Environmental Surveys.CBMI2018
- 74 articleIamNN: Iterative and Adaptive Mobile Neural Network for Efficient Image Classification.CoRRabs/1804.101232018
- 75 inproceedingsLearning DTW-Preserving Shapelets.IDA2017
- 76 inproceedingsContext-Aware Forgery Localization in Social-Media Images: A Feature-Based Approach Evaluation.ICIP2018
- 77 inproceedingsConnecting the dots between news articles.KDD2010
- 78 inproceedingsWeakly Supervised Object Localization Using Things and Stuff Transfer.ICCV2017
- 79 inproceedingsUnsupervised part learning for visual recognition.CVPR2017
- 80 inproceedingsMemory Vectors for Particular Object Retrieval with Multiple Queries.ICMR2015
- 81 inproceedingsProvenance filtering for multimedia phylogeny.ICIP2017
- 82 inproceedingsUnsupervised Object Discovery for Instance Recognition.WACV2018
- 83 inproceedingsDeep Metric Learning via Lifted Structured Feature Embedding.CVPR2016
- 84 inproceedingsHighly Efficient Multimedia Event Recounting from User Semantic Preferences.ICMR2014
- 85 articleShow and Tell: Lessons Learned from the 2015 MSCOCO Image Captioning Challenge.TPAMI3942017
- 86 phdthesisDeep Neural Architectures for Automatic Representation Learning from Multimedia Multimodal Data.INSA de Rennes2017
- 87 inproceedingsBidirectional Joint Representation Learning with Symmetrical Deep Neural Networks for Multimodal and Crossmodal Applications.ICMR2016
- 88 inproceedingsGenerative Adversarial Networks for Multimodal Representation Learning in Video Hyperlinking.ICMR2017
- 89 articleMemory Networks.CoRRabs/1410.39162014
- 90 inproceedingsVideo Paragraph Captioning Using Hierarchical Recurrent Neural Networks.CVPR2016
- 91 inproceedingsTowards interactive, intelligent, and integrated multimedia analytics.VAST2014
- 92 inproceedingsCrowd Counting via Scale-Adaptive Convolutional Neural Network.WACV2018
- 93 articleShuffleNet: An Extremely Efficient Convolutional Neural Network for Mobile Devices.CoRRabs/1707.010832017