Keywords
Computer Science and Digital Science
- A3.4. Machine learning and statistics
- A3.4.1. Supervised learning
- A3.4.2. Unsupervised learning
- A3.4.4. Optimization and learning
- A3.4.6. Neural networks
- A3.4.7. Kernel methods
- A3.4.8. Deep learning
- A5.3. Image processing and analysis
- A5.3.2. Sparse modeling and image representation
- A5.3.3. Pattern recognition
- A5.3.4. Registration
- A5.4.3. Content retrieval
- A5.4.4. 3D and spatio-temporal reconstruction
- A5.4.5. Object tracking and motion analysis
- A5.4.6. Object localization
- A5.9. Signal processing
- A5.9.3. Reconstruction, enhancement
- A5.9.5. Sparsity-aware processing
- A5.9.6. Optimization tools
- A6.1. Methods in mathematical modeling
- A6.1.1. Continuous Modeling (PDE, ODE)
- A6.1.2. Stochastic Modeling
- A6.3.1. Inverse problems
Other Research Topics and Application Domains
- B1.1. Biology
- B1.1.3. Developmental biology
- B2.6. Biological and medical imaging
1 Team members, visitors, external collaborators
Research Scientists
- Xavier Descombes [Team leader, Inria, Senior Researcher, HDR]
- Florence Besse [CNRS, Senior Researcher]
- Laure Blanc-Féraud [CNRS, Senior Researcher, HDR]
- Luca Calatroni [CNRS, Researcher]
- Eric Debreuve [CNRS, Researcher, HDR]
- Grégoire Malandain [Inria, Senior Researcher, HDR]
- Caroline Medioni [CNRS, Researcher]
PhD Students
- Asma Chalabi [Univ Côte d'Azur]
- Cedric Dubois [Univ Côte d'Azur]
- Sarah Laroui [Univ Côte d'Azur, until Nov 2021]
- Bastien Laville [Univ Côte d'Azur]
- Marta Lazzaretti [Università degli studi di Genova, From November 2021, Joint PhD supervision between Department of Mathematics of University of Genova (Supervisor: Prof. Claudio Estatico) and UCA]
- Imane Lboukili [Johnson & Johnson, from Nov 2021]
- Alexandre Martin [Inria, from Nov 2021]
- Clara Sanchez [Univ Côte d'Azur]
- Vasiliki Stergiopoulou [CNRS]
- Rudan Xiao [Univ Côte d'Azur]
Interns and Apprentices
- Mayeul Cachia [Univ Côte d'Azur, from May 2021 until Nov 2021]
- Morgane Fierville [Univ Côte d'Azur, until Jun 2021]
- Oumaima Laguili [CNRS, from Jun 2021 until Sep 2021]
- Youness Mellak [Inria, from Mar 2021 until Aug 2021]
- Giuseppe Recupero [Università degli studi di Bologna, Université Côte d'Azur, from Apr 2021 until Jul 2021]
- Rim Rekik Dit Nekhili [Inria, from Mar 2021 until Aug 2021]
Administrative Assistant
- Isabelle Strobant [Inria]
External Collaborator
- Sébastien Schaub [CNRS]
2 Overall objectives
Morpheme is a joint project between INRIA, CNRS and the University of Côte d'Azur (UCA); Signals and Systems Laboratory (I3S) (UMR 6070); the Institute for Biology of Valrose (iBV) (CNRS/INSERM).
The scientific objectives of Morpheme are to characterize and model the development and the morphological properties of biological structures from the cell to the supra-cellular scale. Being at the interface between computational science and biology, we plan to understand the morphological changes that occur during development combining in vivo imaging, image processing and computational modeling.
The morphology and topology of mesoscopic structures, indeed, do have a key influence on the functional behavior of organs. Our goal is to characterize different populations or development conditions based on the shape of cellular and supra-cellular structures, including micro-vascular networks and dendrite/axon networks. Using microscopy or tomography images, we plan to extract quantitative parameters to characterize morphometry over time and in different samples. We will then statistically analyze shapes and complex structures to identify relevant markers and define classification tools. Finally, we will propose models explaining the temporal evolution of the observed samples. With this, we hope to better understand the development of normal tissues, but also characterize at the supra-cellular level different pathologies such as the Fragile X Syndrome, Alzheimer or diabetes.
3 Research program
3.1 Research program
The recent advent of an increasing number of new microscopy techniques giving access to high throughput screenings and micro or nano-metric resolutions provides a means for quantitative imaging of biological structures and phenomena. To conduct quantitative biological studies based on these new data, it is necessary to develop non-standard specific tools. This requires using a multi-disciplinary approach. We need biologists to define experiment protocols and interpret the results, but also physicists to model the sensors, computer scientists to develop algorithms and mathematicians to model the resulting information. These different expertises are combined within the Morpheme team. This generates a fecund frame for exchanging expertise, knowledge, leading to an optimal framework for the different tasks (imaging, image analysis, classification, modeling). We thus aim at providing adapted and robust tools required to describe, explain and model fundamental phenomena underlying the morphogenesis of cellular and supra-cellular biological structures. Combining experimental manipulations, in vivo imaging, image processing and computational modeling, we plan to provide methods for the quantitative analysis of the morphological changes that occur during development. This is of key importance as the morphology and topology of mesoscopic structures govern organ and cell function. Alterations in the genetic programs underlying cellular morphogenesis have been linked to a range of pathologies.
Biological questions we will focus on include:
- what are the parameters and the factors controlling the establishment of ramified structures? (Are they really organize to ensure maximal coverage? How are genetic and physical constraints limiting their morphology?),
- how are newly generated cells incorporated into reorganizing tissues during development? (is the relative position of cells governed by the lineage they belong to?)
Our goal is to characterize different populations or development conditions based on the shape of cellular and supra-cellular structures, e.g. micro-vascular networks, dendrite/axon networks, tissues from 2D, 2D+t, 3D or 3D+t images (obtained with confocal microscopy, video-microscopy, photon-microscopy or micro-tomography). We plan to extract shapes or quantitative parameters to characterize the morphometric properties of different samples. On the one hand, we will propose numerical and biological models explaining the temporal evolution of the sample, and on the other hand, we will statistically analyze shapes and complex structures to identify relevant markers for classification purposes. This should contribute to a better understanding of the development of normal tissues but also to a characterization at the supra-cellular scale of different pathologies such as Alzheimer, cancer, diabetes, or the Fragile X Syndrome. In this multidisciplinary context, several challenges have to be faced. The expertise of biologists concerning sample generation, as well as optimization of experimental protocols and imaging conditions, is of course crucial. However, the imaging protocols optimized for a qualitative analysis may be sub-optimal for quantitative biology. Second, sample imaging is only a first step, as we need to extract quantitative information. Achieving quantitative imaging remains an open issue in biology, and requires close interactions between biologists, computer scientists and applied mathematicians. On the one hand, experimental and imaging protocols should integrate constraints from the downstream computer-assisted analysis, yielding to a trade-off between qualitative optimized and quantitative optimized protocols. On the other hand, computer analysis should integrate constraints specific to the biological problem, from acquisition to quantitative information extraction. There is therefore a need of specificity for embedding precise biological information for a given task. Besides, a level of generality is also desirable for addressing data from different teams acquired with different protocols and/or sensors. The mathematical modeling of the physics of the acquisition system will yield higher performance reconstruction/restoration algorithms in terms of accuracy. Therefore, physicists and computer scientists have to work together. Quantitative information extraction also has to deal with both the complexity of the structures of interest (e.g., very dense network, small structure detection in a volume, multiscale behavior, ) and the unavoidable defects of in vivo imaging (artifacts, missing data, ). Incorporating biological expertise in model-based segmentation methods provides the required specificity while robustness gained from a methodological analysis increases the generality. Finally, beyond image processing, we aim at quantifying and then statistically analyzing shapes and complex structures (e.g., neuronal or vascular networks), static or in evolution, taking into account variability. In this context, learning methods will be developed for determining (dis)similarity measures between two samples or for determining directly a classification rule using discriminative models, generative models, or hybrid models. Besides, some metrics for comparing, classifying and characterizing objects under study are necessary. We will construct such metrics for biological structures such as neuronal or vascular networks. Attention will be paid to computational cost and scalability of the developed algorithms: biological experimentations generally yield huge data sets resulting from high throughput screenings. The research of Morpheme will be developed along the following axes:
- Imaging: this includes i) definition of the studied populations (experimental conditions) and preparation of samples, ii) definition of relevant quantitative characteristics and optimized acquisition protocol (staining, imaging, ) for the specific biological question, and iii) reconstruction/restoration of native data to improve the image readability and interpretation.
- Feature extraction: this consists in detecting and delineating the biological structures of interest from images. Embedding biological properties in the algorithms and models is a key issue. Two main challenges are the variability, both in shape and scale, of biological structures and the huge size of data sets. Following features along time will allow to address morphogenesis and structure development.
- Classification/Interpretation: considering a database of images containing different populations, we can infer the parameters associated with a given model on each dataset from which the biological structure under study has been extracted. We plan to define classification schemes for characterizing the different populations based either on the model parameters, or on some specific metric between the extracted structures.
- Modeling: two aspects will be considered. This first one consists in modeling biological phenomena such as axon growing or network topology in different contexts. One main advantage of our team is the possibility to use the image information for calibrating and/or validating the biological models. Calibration induces parameter inference as a main challenge. The second aspect consists in using a prior based on biological properties for extracting relevant information from images. Here again, combining biology and computer science expertise is a key point.
4 Application domains
Among the applications addressed by Morpheme team we can cite:
- Kidney cancer classification from histological images
- IMP-RNA granules detection and classification from confocal image
- Extra-cellular matrix detection and characterization from confocal images
- Axon growth modeling
- Glial cell detection and characterization for the study of high-fat diets
- Death time detection and type classification of cells in microscopy time-lapses
- Morphogenesis and embryogenesis
- Numerical super-resolution techniques
- Design and numerical validation of non-smooth optimization algorithms with applications to biomedical imaging
- Statistical and learning-based approaches for parameter selection in imaging inverse problems
- Interpretation of contrast- and orientation-based visual illusions
5 Highlights of the year
5.1 Awards
- Luca Calatroni was awarded the Young Investigator Training Program 2020 in the occasion of the Italian biannual congress of applied mathematics SIMAI 2020+21 which took place in Parma from August 30 till September 2 2021.
- Arne Bechensteen was awarded in 2021 of the first prize of the doctoral school ED STIC mention automatic Signal and Image Processing for his PhD thesis untitled "3D fluorescence microscopy reconstruction using TIRF-MA imaging".
- Laure Blanc-Féraud has been elevated IEEE Fellow 2022, in december 2021.
- Laure Blanc-Féraud has a chair of 3IA Côte d'Azur (2019-2024).
6 New software and platforms
Let us describe new/updated software.
6.1 New software
6.1.1 Astec
-
Name:
Adaptative Segmentation and Tracking of Embryonic Cells
-
Keywords:
3D, 4D, Data fusion, Image segmentation, Fluorescence microscopy, Morphogenesis, Embryogenesis
-
Scientific Description:
ASTEC stands for Adaptive Segmentation and Tracking of Embryonic Cells, and was first developed during L. Guignard PhD thesis, "Quantitative analysis of animal morphogenesis: from high-throughput laser imaging to 4D virtual embryo in ascidians, Léo Guignard, 2015". It was later published in "Contact area–dependent cell communication and the morphological invariance of ascidian embryogenesis, Léo Guignard at al., Science 2020"
-
Functional Description:
This software suite aims at providing quantitative analysis of multi-angle acquisitions of SPIM images, and the segmentation of the temporal series of 3D images, together with quantitative informations.
- URL:
- Publications:
-
Contact:
Grégoire Malandain
-
Participants:
Patrick Lemaire, Leo Guignard, Emmanuel Faure, Gaël Michelin
-
Partners:
CRBM - Centre de Recherche en Biologie cellulaire de Montpellier, LIRMM
6.1.2 sparse_constraint_relax
-
Name:
sparse_constraint_relaxation
-
Keywords:
Optimization, Sparse regularization, Super-resolution
-
Functional Description:
The optimization of a L2 term under a L0 term constraint is made by relaxation of the initial functional with a continuous exact functional (with same global minimizers). Numerical algorithm is coded for super-resolution for Single Molecule localization microscopy (deconvolution and super-resolution on sparse molecules). We minimize the squared norm and the relaxation Q(x), using Nonmonotone Accelerated Proximal gradient algorithm which was presented in Accelerated proximal gradient methods for nonconvex programming by Li, Huan and Lin, Zhouchen in Advances in neural information processing systems. In order to do so, we use gradient of the square norm and the proximal of Q(x). There are two functions for the proximal operator, and reccomend the fast one for the calculations, but the normal one to better understand what the program is doing. A failsafe function is added to ensure that we always obtain a k-sparse solution. The file uses a simulated acquistion, Testimage.
- URL:
-
Authors:
Arne Henrik Bechensteen, Laure Blanc-Féraud, Gilles Aubert
-
Contact:
Arne Henrik Bechensteen
-
Partner:
I3S
6.1.3 CoBic-PeBic
-
Name:
CoBic-and-PeBic-SMLM
-
Keywords:
Sparse regularization, Optimization, Super-resolution
-
Functional Description:
The code is dedicated to sparse L0 optimization in the case of deconvolution and super-resolution for Single Molecule localization microscopy. The sparsity term is reformulated using an auxiliary variable, and the final cost function is biconvex. We propose two algorithms CoBic (Constrained Biconvex) and PeBic (Penalized Biconvex) algorithm for SMLM. CoBic is the reformulated constrained L2_L0 problem and PeBic is the reformulated penalized L2_L0 problem. Both with the positivity constraint. More information is the article https://hal-anr.archives-ouvertes.fr/hal-02556389v1
-
Release Contributions:
first version
- URL:
-
Authors:
Arne Henrik Bechensteen, Laure Blanc-Féraud, Gilles Aubert
-
Contact:
Arne Henrik Bechensteen
-
Partner:
I3S
6.1.4 COL0RME
-
Name:
COvariance-based l0 super-Resolution Microscopy with intensity Estimation
-
Keywords:
Super-resolution, Optimization, Fluorescence microscopy, Sparsity
-
Functional Description:
The code is dedicated to the super-resolution method COL0RME (COvariance-based l0 super-Resolution Microscopy with intensity Estimation) that improves the spatio-temporal resolution of images acquired by common fluorescent microscopes and conventional blinking or flucutating fluorophores. The method is composed of two steps: the former where both the emitters' independence and the sparse distribution of the fluorescent molecules are exploited to provide an accurate localization, the latter where real intensity values are estimated given the computed support. More information is the articles: https://hal.archives-ouvertes.fr/hal-02979332, https://hal.archives-ouvertes.fr/hal-029793
-
Release Contributions:
First version
- URL:
- Publications:
-
Authors:
Vasiliki Stergiopoulou, Laure Blanc-Féraud, Luca Calatroni, Jose Henrique De Morais Goulart
-
Contact:
Vasiliki Stergiopoulou
-
Partner:
I3S
7 New results
7.1 A scaled, inexact and adaptive FISTA algorithm for strongly convex optimization
Participants: Luca Calatroni.
This work has been carried out in collaboration with S. Rebegoldi (University of Florence).
In 35, we consider a variable metric and inexact version of the FISTA-type algorithm studied, e.g., by 39 for the minimization of the sum of two (possibly strongly) convex functions. The proposed algorithm is combined with an adaptive (non-monotone) backtracking strategy, which allows for the adjustment of the algorithmic step-size along the iterations in order to improve the convergence speed. We prove a linear convergence result for the function values, which depends on both the strong convexity parameters of the two functions and the upper and lower bounds on the spectrum of the variable metric operators. We validate the proposed algorithm, named Scaled Adaptive GEneralized FISTA (SAGE-FISTA), on exemplar image denoising and deblurring problems where edge-preserving Total Variation (TV) regularization is combined with Kullback-Leibler-type fidelity terms, as it is common in applications where signal-dependent Poisson noise is assumed in the data. We report the results obtained in this example in Figure 1 where convergence rates, computational times and Lipschitz constant estimations are compared both for monotone and non-monotone backtrcking.
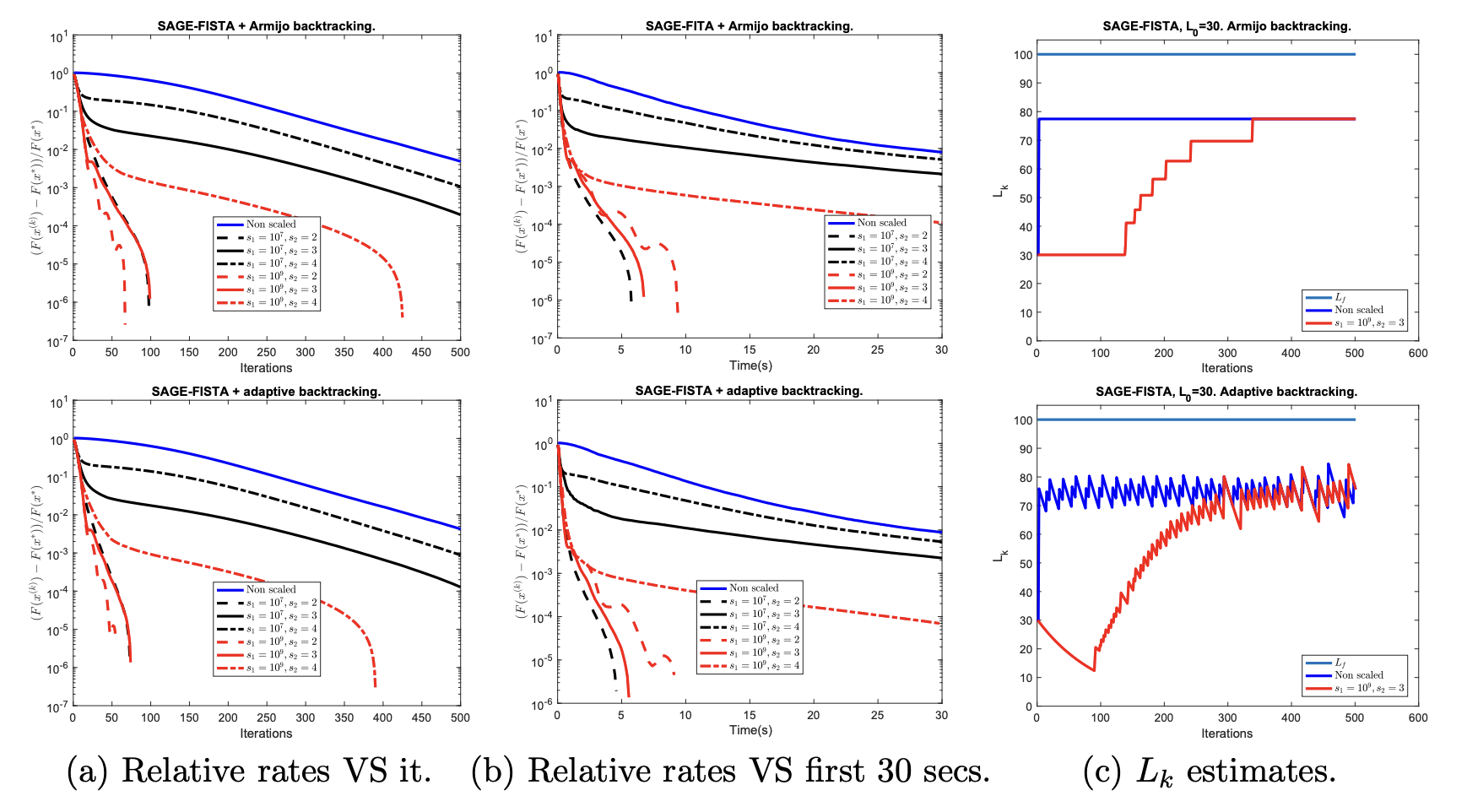
Convergence rates in function value and Lipschitz constant estimate for strongly convex inexact FISTA with scaling and monotone (first row) and adaptive (second row) backtracking. Reference problem: TV deblurring problem with ℓ2\ell _2 fidelity.
The manuscript is currently at the second stage of review for SIAM journal on Optimization.
7.2 Efficient optimisation of Poisson-type modelling of SMLM data
Participants: Luca Calatroni, Marta Lazzaretti.
This work has been carried out in collaboration with C. Estatico (DIMA, University of Genova) and S. Rebegoldi (University of Florence).
In 25, we proposed a continuous non-convex variational model for the analysis of Single Molecule Localisation Microscopy (SMLM) data in the presence of Poisson noise. Inspired by previous work 49, 42 we consider, in particular, a variation of the Continuous Exact (CEL0) penalty recently introduced to relax the - problem where a weighted- data fidelity is considered to model signal-dependent Poisson noise. For improving upon the numerical solution of the associated minimisation problem, we consider an iterative reweighted (IRL1) strategy and proposed in 28 a scaled version of the well-known FISTA algorithm is proposed. The proposed weighted-CEL0 (wCEL0) model improves the results obtained by CEL0 and the use of the scaled FISTA algorithm reduces significantly the computational efforts.
7.3 Proximal algorithms in
Participants: Luca Calatroni, Marta Lazzaretti.
This work has been carried out in collaboration with C. Estatico (DIMA, University of Genova).
In this project, we have worked on the development of forward-backward algorithm in variable exponent Lebesgue spaces , which are spaces defined by a point-wise variable Lebesgue measurable function , used as exponent, instead of a constant exponent . These spaces are (non-Hilbertian) Banach spaces, endowed with space variant geometrical properties which are useful in adaptive regularization techniques and used for the solution of ill-posed inverse problems. We analysed, in particular, two instances (primal and dual) of proximal gradient algorithms, where the proximal step, rather than depending on the natural (non-separable) norm, is defined in terms of its modular function, which, thanks to its separability, allows for the efficient computation of algorithmic iterates. Convergence in function values has been proved for both algorithms, with convergence rates depending on problem/space smoothness. To show the effectiveness of the proposed modelling, some numerical tests have been carried out for exemplar deconvolution and mixed noise removal problems. Future work shall address the crucial question on how the map shall be chosen in order to adapt to local signal/image prior contents. Moreover, it would be interesting to find a strategy to incorporate extrapolation/acceleration in order to improve convergence speed which, in practice, is particularly slow for primal algorithms.
The outcome of this project is the manuscript 32 which has been recently sent for publication to SIAM journal of Scientific Computing.
7.4 Residual whiteness principle for automatic parameter selection in super-resolution problems
Participants: Luca Calatroni.
In collaboration with A. Lanza, M. Pragliola, F. Sgallari (University of Bologna).
In 26, 33 we propose an automatic parameter selection strategy for the problem of image super-resolution for images corrupted by blur and additive white Gaussian noise with unknown standard deviation. The proposed approach exploits the structure of both the down-sampling and the blur operators in the frequency domain and computes the optimal regularisation parameter as the one optimising a suitable residual whiteness measure. Computationally, the proposed strategy relies on the fast solution of generalised Tikhonov - problems as proposed in previous works. These problems naturally appear as substeps of the Alternating Direction Method of Multipliers (ADMM) optimisation approach used to solve super-resolution problems with non-quadratic and often non-smooth, sparsity-promoting regularisers both in convex and in non-convex regimes. After detailing the theoretical properties defined in the frequency domain which allow to express the whiteness functional in a compact way, we report an exhaustive list of numerical experiments proving the effectiveness of the proposed approach for different type of problems, in comparison with well-known parameter selection strategy such as, e.g., the discrepancy principle, see, e.g., Figure 2, left, for graphical representation where the proposed whiteness measure (black line) is plotted and its minimum is compared with the one achieved by the optimisation of the standard discrepancy-principle (pink line). Analogously, in Figure 2, fight, the optimal PSNR and SSIM values are showed.
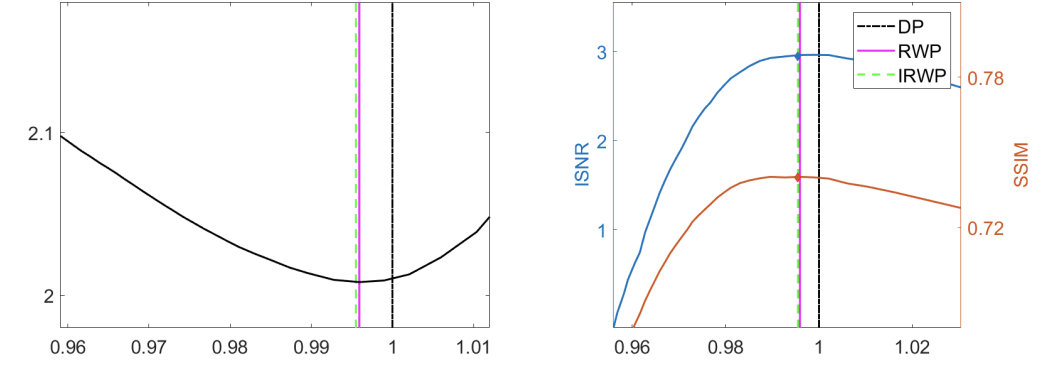
In 26, the approach is applied to - super-resolution problems. This work has been published to the SSVM 2021 conference proceedings. In 33, the approach is extended to non-smooth optimisation models and embedded within an ADMM optimisation strategy. This latter work is an invited submission for publication in the Journal of Mathematical Imaging and Vision.
7.5 On and beyond Total Variation regularisation in imaging: the role of space variance
Participants: Luca Calatroni.
In collaboration with A. Lanza, M. Pragliola, F. Sgallari (University of Bologna).
Over the last decades a plethora of variational regularisation models for image reconstruction has been proposed and thoroughly inspected by the applied mathematics community. Among them, the pioneering prototype often taught and learned in basic courses in mathematical image processing is the celebrated Rudin-Osher-Fatemi (ROF) model which relies on the minimisation of the edge-preserving Total Variation (TV) semi-norm as regularisation term. Despite its (often limiting) simplicity, this model is still very much employed in many applications and used as a benchmark for assessing the performance of modern learning-based image reconstruction approaches, thanks to its thorough analytical and numerical understanding. Among the many extensions to TV proposed over the years, a large class is based on the concept of space variance. Space-variant models can indeed overcome the intrinsic inability of TV to describe local features (strength, sharpness, directionality) by means of an adaptive mathematical modelling which accommodates local regularisation weighting, variable smoothness and anisotropy, see Figure 3 for an illustration of the many different Weighted (WTV), Weighted- variations (WTV) and Weighted Directional TV (DTWV) regularisers at different points. Those ideas can further be cast in the flexible Bayesian framework of generalised Gaussian distributions and combined with maximum likelihood and hierarchical optimisation approaches for efficient hyper-parameter estimation. In this work, we review and connect the major contributions in the field of space-variant TV-type image reconstruction models, focusing, in particular, on their Bayesian interpretation which paves the way to new exciting and unexplored research directions.
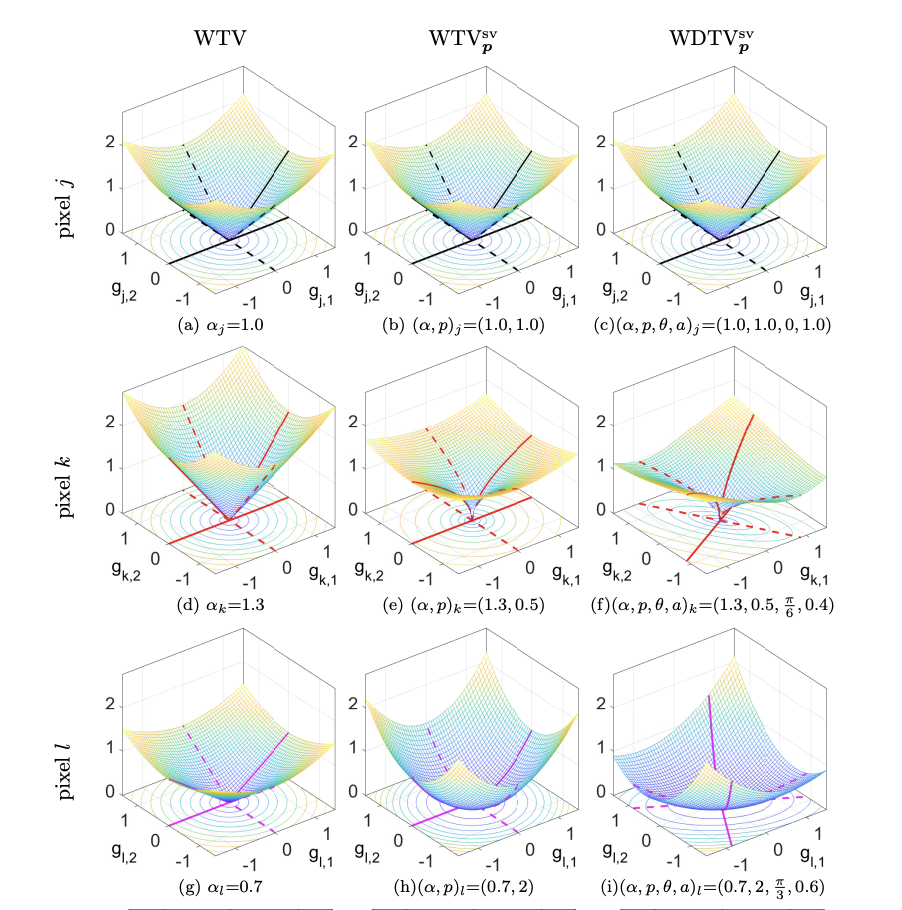
Weighted TV (first column), weighted TVp_p (second column) and weighted directional TVp_p penalty functions.
The outcome of this work 6 is currently under the second stage of review for SIAM Review.
7.6 gradient-based super-resolution for image partitioning
Participants: Luca Calatroni.
In collaboration with P. Cascarano, E. L. Piccolomini, D. Mylonopoulos (University of Bologna).
In 11, we consider a variational model for single-image super-resolution based on the assumption that the gradient of the target image is sparse. We enforce this assumption by considering both an isotropic and an anisotropic regularisation on the image gradient combined with a quadratic data fidelity, similarly as studied for signal recovery problems. For the numerical realisation of the model, we propose a novel efficient ADMM splitting algorithm whose substeps solutions are computed efficiently by means of hard-thresholding and standard conjugate-gradient solvers. We test our model on highly-degraded synthetic and real-world data and quantitatively compare our results with several sparsity-promoting variational approaches as well as with state-of-the-art deep-learning techniques. Our experiments show that thanks to the smoothing on the gradient, the super-resolved images can be used to improve the accuracy of standard segmentation algorithms for applications like QR codes, land-cover classification problems and cell detection as, e.g. in Figure 4. We compare the result obtained with the proposed penalised model with the ones obtained by an analogous constrained model, similarly to the one considered in 48.
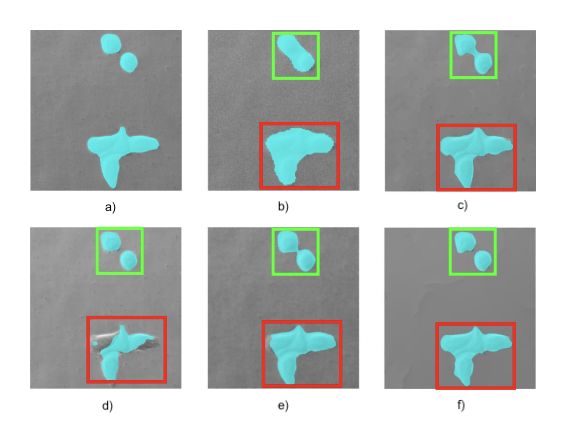
Cell detection via moprhological operations from noisy and low-resolution data. a) Ground truth b) Detection from noisy and LR data c) Detection after anisotropic TV1/2^{1/2} super-resolution d) Detection after application of IRCNN e) Detection after reconstruction through dictionary learning and sparse representation SR f) Detection after ℓ0\ell _0 gradient-based SR (proposed).
This work has been published in the journal IEEE Transactions on Computational Imaging 11. A MATLAB software with well-documented guide has been sent for publication to Image Processing On Line (IPOL) and it's currently at the second stage of review.
7.7 Non-linear image osmosis for shadow removal
Participants: Luca Calatroni, Giuseppe Recupero.
In collaboration S. Morigi (University of Bologna), S. Parisotto (Fitzwilliam museum & University of Cambridge).
We studied the images osmosis model, which is a linear drift-diffusion PDE particularly useful in image processing with applications such as shadow removal, compact data representation and cloning. It is known that in shadow removal applications the osmosis model causes diffusion and is unable to preserve the underlying features in the pixels at the border of the shadow. Hence, in order to improve the results, we started developing a non-linear isotropic variation of the original osmosis model. We investigated the theoretical properties and the possible discretizations of the model showing significant improvements with respect to the standard, linear, one. In Figure 5 a comparison between linear and non-linear modelling is showed.
![]() |
![]() |
![]() |
![]() |
Ground truth | Shadowed image | Linear osmosis | Proposed |
A journal paper on the outcomes of this project is being written and will be soon submitted for publication to a journal of mathematical imaging.
7.8 Local estimation of regularization parameters in inverse problems by deep learning
Participants: Rim Rekik Dit Nekhili, Luca Calatroni, Xavier Descombes.
The estimation of regularization parameters in the context of inverse problems is crucial for many different applications. More or less sophisticated and most often iterative approaches make it possible by encoding some (generally unknown) information on the data such as the noise distribution and/or the desired image to compute. For most of these approaches, the estimation is performed globally and, as such, does not take into account the different amount of regularization needed in different image regions. Deep learning approaches have proven to be very effective for many data and image analysis problems and often achieved unprecedented results in the context of digital image processing. In this project, a pixel-wise map of parameters is estimated by means of a convolutional network by considering the different patches centered in each of the pixels of the given noisy and blurry image.
In this project we have first constructed an image database by corrupting initial images with several types and levels of noise. We then tested several convolutional neural network architectures with different convolutional layers, max pooling and flattening layers and for different choices of batch-sizes, learning rates and epochs, and selected the one providing the least RMSE. The resulting CNN has a very simple architecture: one single convolutional layer followed by a max pooling and flattening layer and completed by a dense layer. By means of the proposed architecture we locally estimate the hyperparameter associated with a variationnal denoising model, the learning set being constructed by maximizing the SNR of the denoised result. The first results give a proof of concept of the deep learning approach for locally estimating parameters (see figure 6).
Next steps will consist of comparing the obtained results with statistical approaches and of addressing the case of deconvolution.
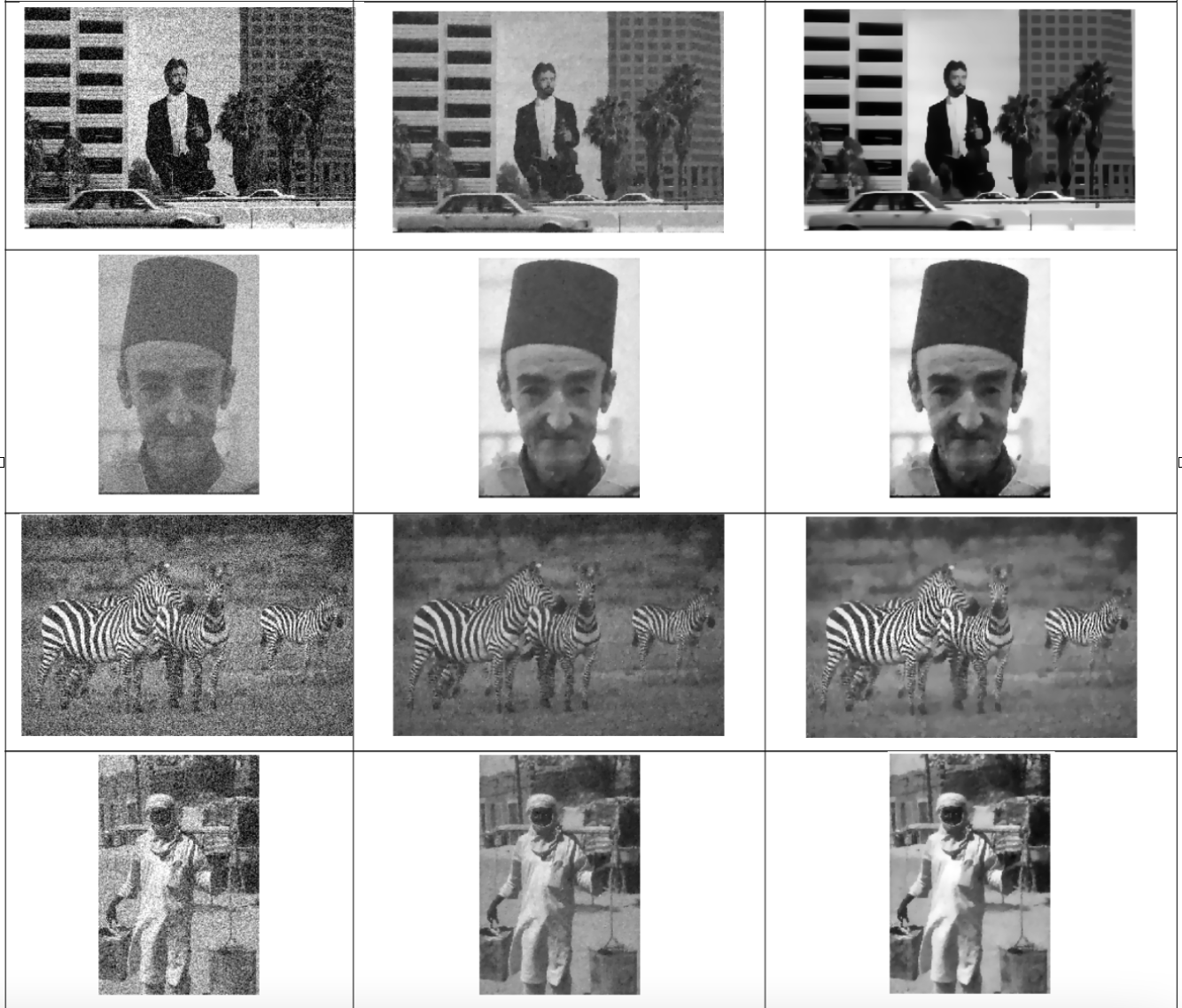
7.9 Covariance-based super-Resolution Microscopy with intensity Estimation and automatic parameter estimation (COL0RME)
Participants: Luca Calatroni, Laure Blanc-Féraud, Vasiliki Stergiopoulou, Sébastien Schaub.
This work has been carried out in collaboration with J. H de Goulart (INP Toulouse/IRIT).
Super-resolution light microscopy overcomes the physical barriers due to light diffraction. However, state-of-the-art approaches require specific and often demanding acquisition conditions to achieve adequate levels of both spatial and temporal resolution. Analyzing the stochastic fluctuations of the fluorescent molecules provides a solution to the aforementioned limitations. Based on this idea, we proposed COL0RME 7, 22, a method for COvariance-based super-Resolution Microscopy with intensity Estimation, which achieves good spatio-temporal resolution by solving a sparse optimization problem in the covariance domain, discuss automatic parameter selection strategies, estimates background and noise statistics and includes a final estimation step where intensity information is retrieved, see Figure 7 for a schematic representation. Several numerical results both on synthetic and real fluorescent microscopy images and several comparisons with state-of-the art approaches have been done. Our results show that COL0RME outperforms competing methods exploiting analogously temporal fluctuations; in particular, it achieves better localization, reduces background artifacts and avoids fine parameter tuning.
As future research directions, we are interested in the investigation of learning approaches such as, for instance, unrolled deep networks such as, e.g., the Learned SPARCOM algorithm proposed in 41. Algorithm unrolling methods connect the iterative algorithms that are widely used in image processing and the deep neural networks 47
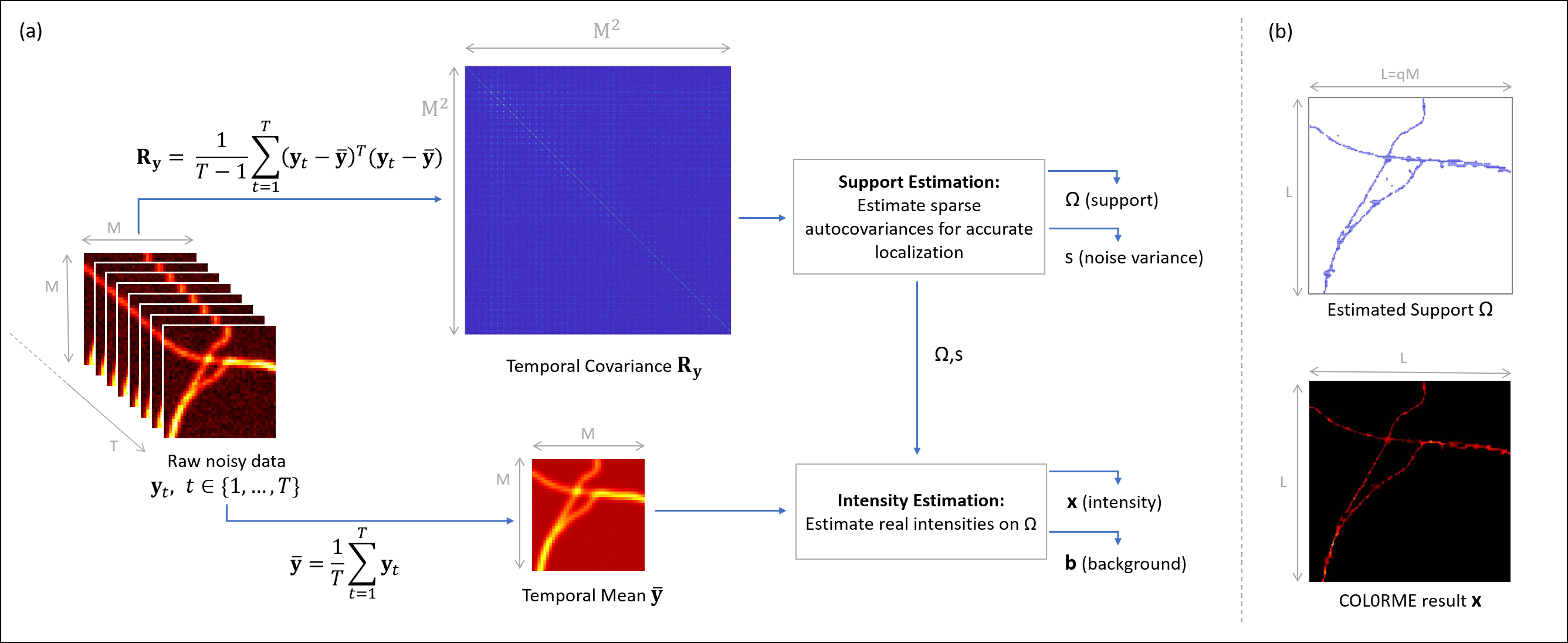
Principles of COL0RME. (a) An overview of the two steps (Support Estimation and Intensity Estimation) by visualizing the inputs/outputs of each, as well as the interaction between them. (b) The two main outputs of COL0RME are: the support Ω⊂ℝL2\Omega \subset \mathbb {R}^{L^2} containing the locations of the fine-grid pixels with at least one fluorescent molecule, and the intensity 𝐱∈ℝL2\mathbf {x} \in \mathbb {R}^{L^2} whose non-null values are estimated only on Ω\Omega .
This appeared in the proceedings of the IEEE ISBI 2021 conference 7. A longer version of this work has been sent for publication to the Biological Imaging journal 22.
Vasiliki Stergoipoulou's work is supported by the French Government through the 3IA Côte d’Azur Investments in the Future project managed by the National Research Agency (ANR) with the reference number ANR-19-P3IA-0002.
7.10 3D-COL0RME
Participants: Luca Calatroni, Laure Blanc-Féraud, Vasiliki Stergiopoulou, Sébastien Schaub.
We proposed a 3D super-resolution approach to improve spatial resolution in Total Internal Reflectance Fluorescence (TIRF) imaging applications. Our approach, called 3D-COL0RME (3D - Covariance-based super-Resolution Microscopy with intensity Estimation) improves both lateral and axial resolution by combining sparsity-based modelling for precise molecule localisation and intensity estimation in the lateral plane with a 3D reconstruction procedure in the axial one using Multi-Angle TIRF (MA-TIRF). Differently from state-of-the-art approaches, 3D-COL0RME does not require the use of special equipment as it can be used with standard fluorophores. The proposed methods has been validated on simulated MA-TIRF blinking-type data and on challenging real MA-TIRF acquisitions, showing significant resolution improvements.
This appeared has been sent for publication to the proceedings of the ISBI 2022 conference 38.
Vasiliki Stergoipoulou's work is supported by the French Government through the 3IA Côte d’Azur Investments in the Future project managed by the National Research Agency (ANR) with the reference number ANR-19-P3IA-0002.
7.11 Adversarial learning for super resolution microscopy
Participants: Mayeul Cachia, Laure Blanc-Féraud, Luca Calatroni, Sébastien Schaub.
Fluorescence microscopy is a well known method to observe specific proteins, marking them with fluorescent molecules. However, the resolution of this method is limited by the diffraction of light in the optical system. In practice it is not possible to observe details below 200nm in the lateral plane.
The goal of super-resolution is to overcome this limitation. There exist many methods for super resolution but most of them are based on specific setup or molecules. The idea was to propose a numerical method both data-driven and model-based inspired from CryoGAN 44 and MSR-GAN 51 but adapted to super-resolution context. The first step was to model the direct problem of microscope acquisition and implement a reliable simulator. This component generates from a discrete distribution of sources a range of corresponding coarse images we could get from the real microscope. Since emission and absorption of photons is a random process, especially when there are only a few photons, this simulator cannot be deterministic. These simulated images should be then compared with real images from the microscope.
Computing a super-resolved image means computing a fine estimation of the distribution of sources. Thus we update the input of the simulator until the output is distributed like the real images from the microscope. This optimization process aims to minimize the distance between the real images and the simulated images depending on the input of the simulator. It can be considered as an adversarial learning problem similar to the training of a specific well-chosen generative adverse network (GAN). The idea is to train a neural net called discriminator to evaluate the simulator output. The discriminator is trained to distinguish the real images from the simulated ones while the simulator should generate images each step nearer from the real images. So these two components have conflicting goals and this kind of double optimisation is said to be adverse.
The main difficulty is to set the hyperparameters of the algorithm. It could be interesting to understand more precisely their respective influence on the results. Despite this limitation this new method for super-resolution yields promising results on both synthetic data and ostreopsis experimental images, see Figure 8. It seems to be robust enough to generalize to other data. In the general context of inverse problems it provides a new validation of the CryoGAN and MSR-GAN approach and let us suppose this approach could be easily adapted to other inverse problems.
![]() |
![]() |
7.12 Off-the-grid dynamic super-resolution for fluorescence microscopy
Participants: Bastien Laville, Laure Blanc-Féraud, Gilles Aubert.
Off-the-grid variational methods recover Radon measures, elements of the Banach from a stack of noisy altered measurements on a compact . The thesis consists in the investigation and the development of new gridless methods in static or dynamic settings, with applications in biomedical imaging. As of today:
- we implemented several algorithms for off-the-grid methods taken from the literature in a efficient and user-friendly Python module;
- we developed a new gridless method in a dynamical context. Through the definition of new operator and variational problem, we worked on the localisation of spikes with time-dependent amplitude (and static position), a proxy of the SOFI problem. See Figure 9.
Ongoing project consists in investigating a new approach to extend the off-the-grid theory (right now limited to points) to the reconstruction of more exotic structures like curves, from both theoretical and numerical standpoints;
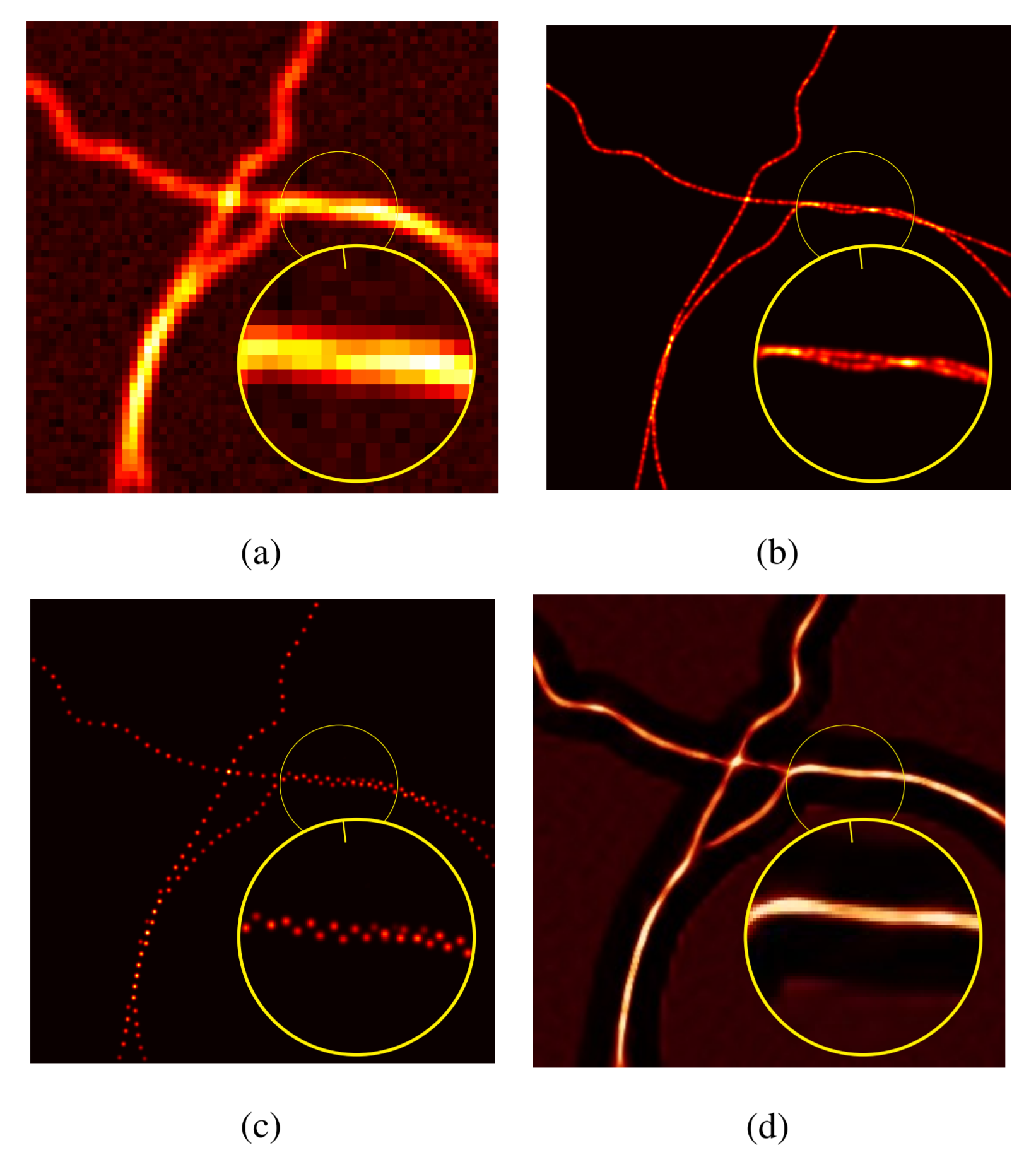
We have published a review paper in Journal of Imaging 17 and a preprint accepted in IEEE ICASSP 2022 'Off-the-grid covariance-based super-resolution fluctuation microscopy'. See 31
7.13 Correcting estimations of the copepod volume from 2-dimensional images
Participants: Cédric Dubois, Eric Debreuve.
This work was carried out in collaboration with Jean-Olivier Irisson (LOV, Villefranche-sur-mer, France).
Accurate plankton biomass estimations are essential to study marine ecological processes and biogeochemical cycles. This is particularly true for copepods, which dominate mesozooplankton. Such estimations can efficiently be computed from organism volume estimated from images. However, imaging devices only provide 2D projections of 3D objects. The classical procedures to retrieve volumes, based on the Equivalent Spherical Diameter (ESD) or the best-fitting ellipse, are biased. We developed a method to correct these biases. First a new method aims to estimate the organism body area through ellipse fitting (see Figure 10, left image). Then, the body of copepods is modeled as an ellipsoid whose 2D silhouette is mathematically derived. Samples of copepod bodies are simulated with realistic shapes/sizes and random orientations. Their total volume is estimated from their silhouettes using the two classical methods and a correction factor is computed, relative to the known, total, volume. On real data, individual orientations and volumes are unknown but the correction factor still holds for the total volume of a large number of organisms. The correction is around -20% for the ESD method and +10% for the ellipse method. When applied to a database of 150,000 images of copepods captured by the Underwater Vision Profiler, the corrections decreased the gap between the two methods by a factor of 54 (see Figure 10, right image). Additionally, the same procedure is used to evaluate the consequence of the bias in the estimation of individual volumes on the slopes of Normalized Biovolume Size Spectra (NBSS) and show that they are not sensitive to the bias. This work is being reviewed by the journal “Limnology and Oceanography: Methods” (Wiley).
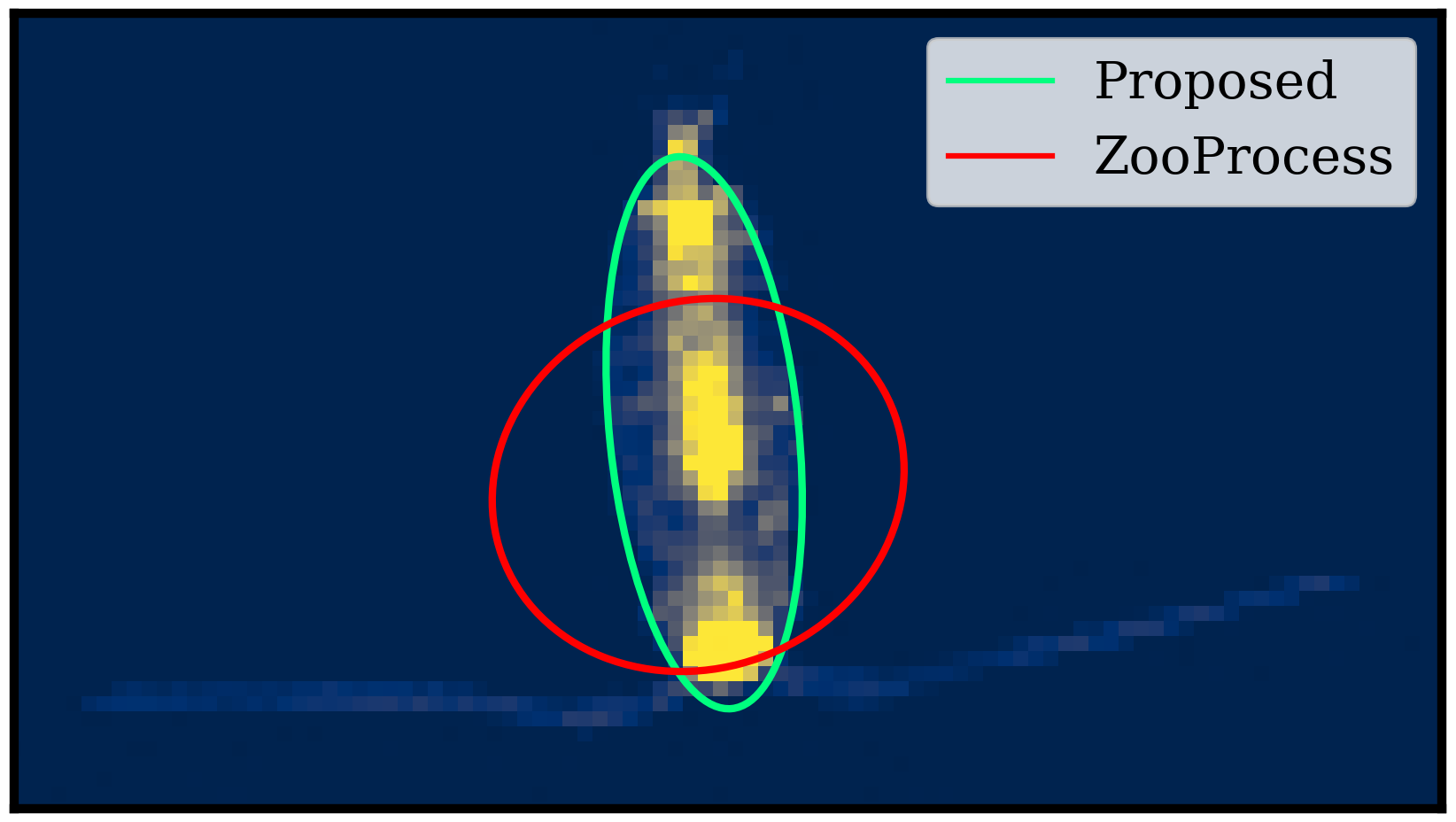
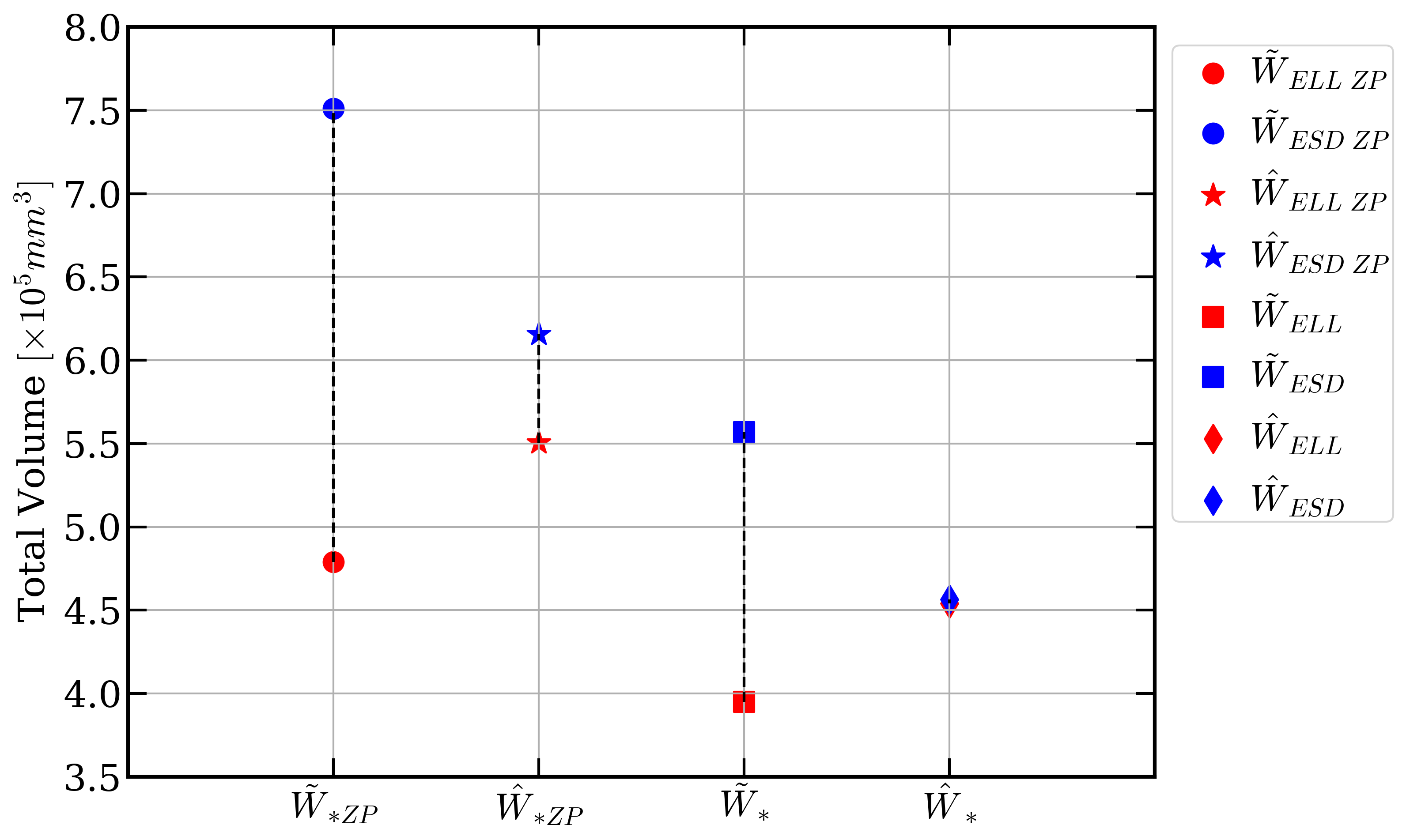
7.14 Graphical User Interface for the image processing pipeline of the NutriMorph project
Participants: Thissas Benkaroun, Eric Debreuve.
This work was made in collaboration with Clara Sanchez and Carole Rovère, IPMC, Sophia Antipolis, France.
Overweight and obesity are major public health issues affecting respectively 39% and 13% of the world population (From World Health Organization, 2016). They constitute prominent risks for numerous chronic diseases, including diabetes, cardiovascular diseases, and cancer. Studies in animal models and humans reveal that excess fat diets promote both a peripheral chronic inflammation and a hypothalamic neuroinflammation, which possibly leads to feeding behavior deregulation. Ascertaining whether the inhibition of early activation of two major brain cells involved in feeding behavior (glial cells, more specifically astrocytes and microglia) in the hypothalamus could prevent obesity would offer new prospects for therapeutic treatments. To understand the mechanisms pertaining to obesity-related neuroinflammatory response, we developed a fully automated algorithm, NutriMorph. Although some algorithms were developed in the past decade to detect and segment neural cells, they are highly specific, not fully automatic, and do not provide the desired morphological analysis. Our algorithm cope with these issues and performs the analysis of cells images (here, microglia of the hypothalamic arcuate nucleus), and the morphological clustering of these cells through statistical analysis and machine learning. However, graphical user interfaces at key steps of the pipeline are actually helpful to tune the parameters of the algorithm on a couple of images before running the analyses in batch mode on a whole dataset. Therefore, we developed such an interface for the soma detection task, allowing to check and select the cells for further analysis (see Figure 11).
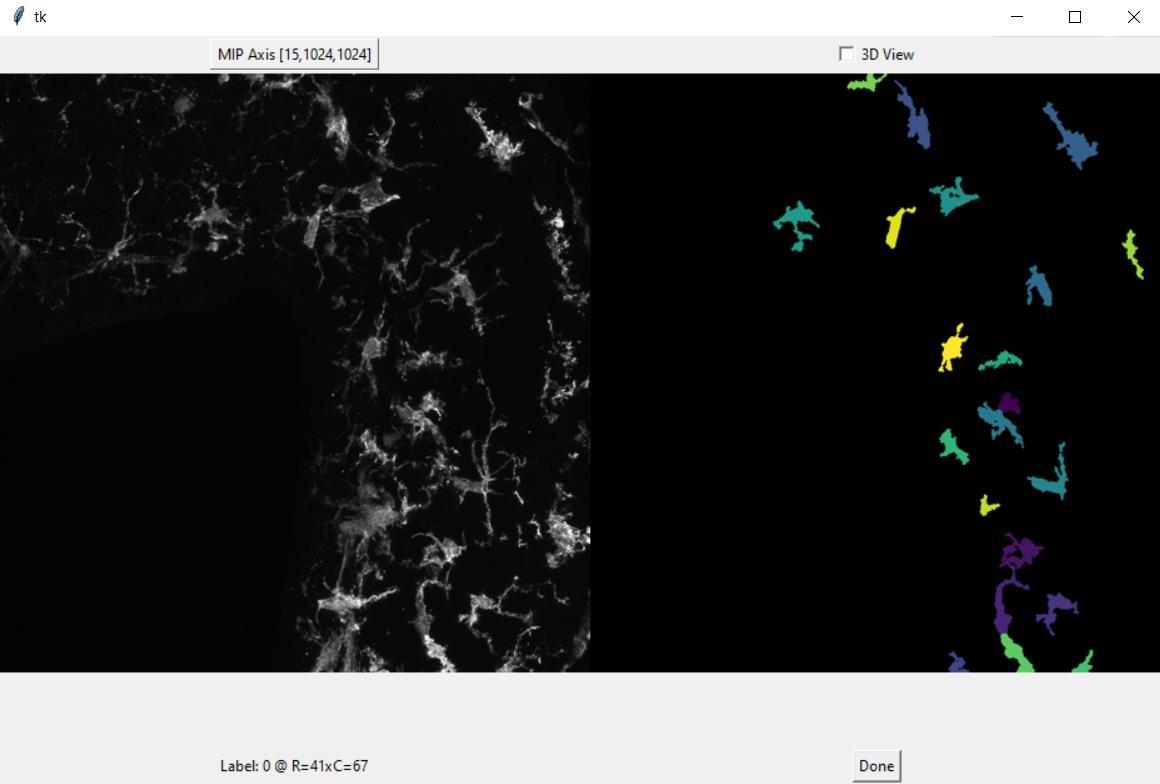
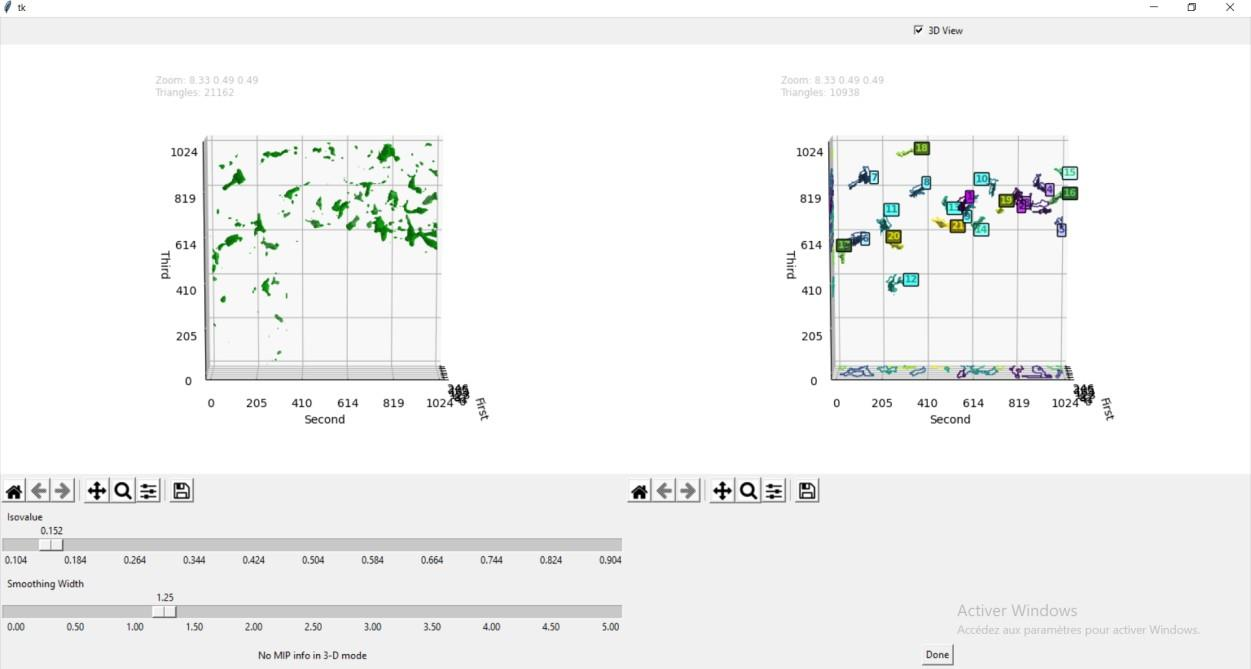
7.15 Dynamic analysis framework to detect cell division and death events in live-cell imaging without specific event markers
Participants: Asma Chalabi, Eric Debreuve.
This work was made in collaboration with Jérémie Roux, Ircan, Nice, France.
The detection of cell division and death events is essential for the understanding of tumor cells responses to cancer therapeutics and to obtain robust metrics of pharmacodynamics. Knowing precisely when these events occur in a live-cell experiment allows to study the relative contribution of different drug effects, such as cytotoxic or cytostatic effects, on a cell population. Yet, classical methods require event-specific dyes (or markers) and measure cell viability as an end-point assay with whole population counts where the proliferation rates can only be estimated when both viable and dead cells are labeled simultaneously. As an alternative, we are developing a dynamic analysis framework for detecting single cell events in marker-free time-lapse microscopy experiments of drug pharmacological profiling. Cell tracking is performed using a basic approach which has been adapted to account for cell divisions. Cell divisions are detected topologically on the tree-shaped cell trajectories produced by the tracking step. A pattern matching procedure is then applied to the cell intensity entropy to filter out spurious divisions arising from debris tracking after cell death. Cell death are detected topologically as trajectories ending before the last time-lapse frame, or using pattern matching on cell intensity entropy (see Figure 12).
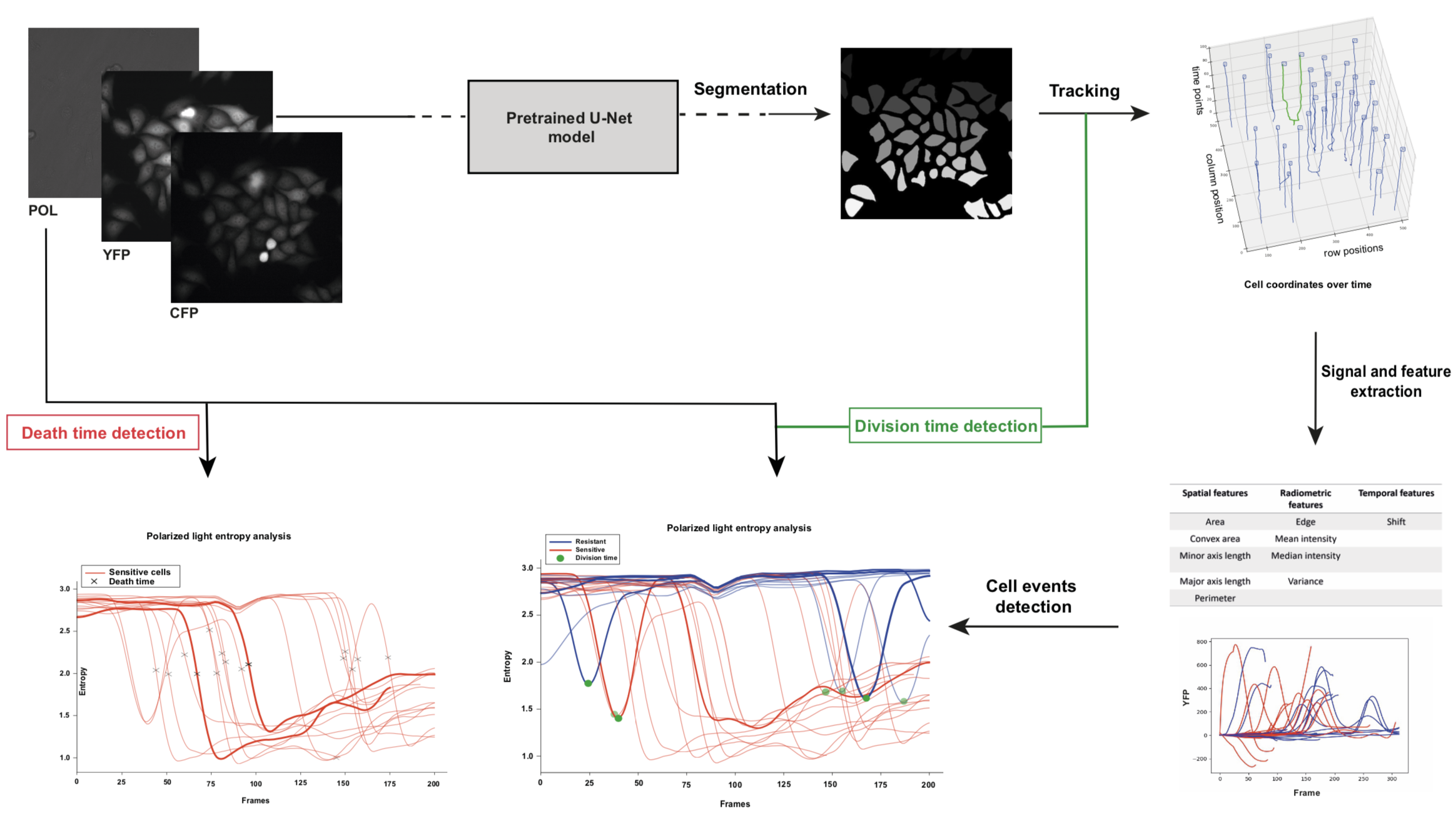
7.16 Optimal transport for classification
Participants: Sarah Laroui, Eric Debreuve, Xavier Descombes.
This work was carried out in collaboration with Aurelia Vernay (Bayer) as part of a contract with Bayer.
We have developed an alternative classification approach based on optimal transport whose strength lies in its ability to consider the geometry of the sample distribution. We proposed to transform the data so they follow a simple model (in practice a Gaussian model), the complexity of the data then being "hidden" in the transport transformation. This approach also offers an original way to estimate the probability density function (PDF) underlying a population sample. For that, we used optimal transport (OT) to transform one distribution into another at a lower cost. In our case, we transform the starting data into data that follows a normal distribution. Thus, for each class, we generate a Gaussian with the same Mean and variance as the starting distribution. We learn the transport between source and target samples. Then we transport the samples via the learned transport, and we calculate source sample PDF values using the position of the transported samples and the parameters (mean and variance) of the class distribution. An extrapolation function is then learned based on the class samples and the recovered PDFs. Finally, we obtain for each class, a model that gives the PDFs of any sample (see figure 13.)
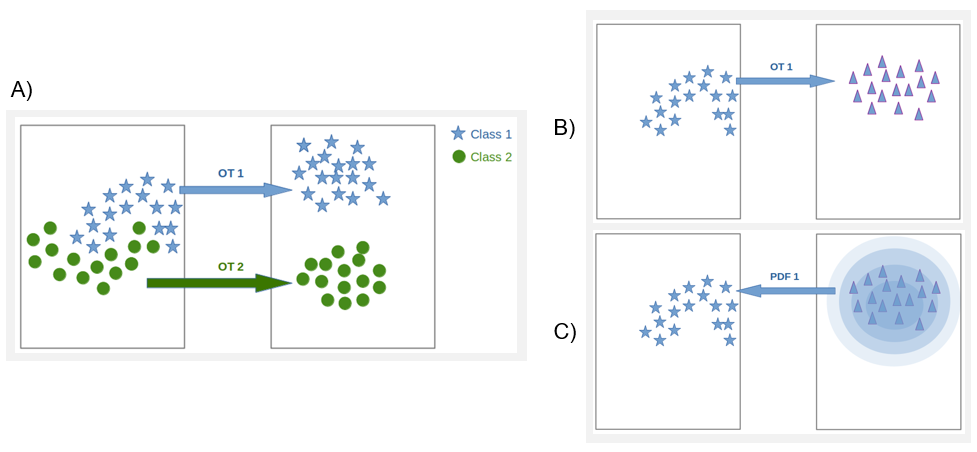
7.17 Fungus growth model
Participants: Sarah Laroui, Eric Debreuve, Xavier Descombes.
This work is made in collaboration with Aurelia Vernay (Bayer) as part of a contract with Bayer.
To model the growth of the Botrytis cinerea fungus, we consider a fungus as a tree structure whose growth is an iterative process for which some events must be defined, and the probability of their occurrence computed. We first acquired several microscopy time lapses of growing fungi. The first step is to segment the fungi. We used a Canny-based procedure. After segmentation, we corrected the masks according to an inclusion hypothesis (fungi do not move and can only grow). Then, the fungi are tracked over time using the classical overlapping hypothesis. Finally, we extracted the fungi skeletons, converted them to a graph, and computed three parameters per fungus: the total length of the skeleton and the number of primary and terminal branches. From these data, we studied the length parameter evolution over time, and chose an exponential law as the length evolution model. The law coefficients were estimated independently for each fungus using the least square method, and their respective distribution was computed. This allows to generate realistic random length evolutions. We also studied the branch creation events over time. We concluded that this event occurrences do not exhibit any particular trend. The proposed fungus growth simulator represents a fungus by a tree graph whose first node corresponds to the initial spore. A branch is composed of one or more edges. The fungus growth is generated by successive creations of edges without thickness notion. At each time (or iteration), the graph branches lengthen by an additional edge. Additionally, a new branch can be created, either from a terminal node (split at the ending point of a branch) or from the initial (spore) node, by adding a new edge according to a Bernoulli law. Each of these additions of edges relies on two parameters: a length and an angle. The length depends on coefficients drawn according to the distributions previously estimated in the exponential law context, and on the number of edges to be created. The angle is drawn randomly within fixed intervals (see Figure 14).
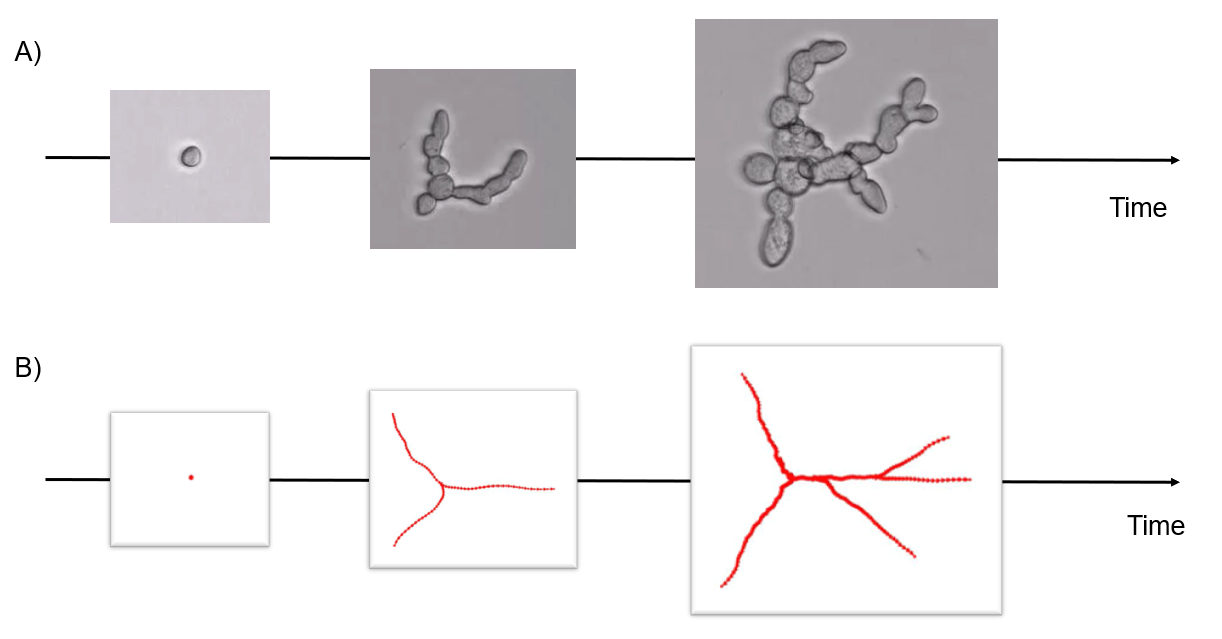
7.18 Parametric shapes segmentation using deep learning
Participants: Oumaima Laguili, Sarah Laroui, Xavier Descombes.
In this project, we aim at identifying parametric objects in an image using a deep learning approach. The goal is to detect and segment a collection of objects. In first step, we have simulated a database of noisy images containing disks or ellipses. We first have considered a circle detection CNN architecture on an image containing one disk. We have then applied a transfer learning scheme where the convolutional layers were frozen to generalize the network to the ellipse case. The next step is to address a collection of objects. We considered the Faster RCNN network to draw a bounding box around each object. We can see that the Faster RCNN is able to desambiguate clusters of objects by drawing a bounding box around each individual oject, preventing the use of non overlapping prior (see figure 15). We then extract the image patch corresponding to each bounding box and compare two strategies. First we segment the ellipse using the ellipse detection CNN and we compare the result with an ellipse fitting algorithm. This two steps framework extract clustered objects model by ellipses from images. The next work will consist in a transfer learning step to address real data.
![]() |
![]() |
Objects detection by bounding boxes using faster RCNN.
7.19 Uveitis detection from OCT using deep learning
Participants: Youness Mellak, Xavier Descombes.
This work has been carried out in collaboration with Prof. Alin Achim from Bristol university.
Uveitis is an inflammatory disease that affect the eye and is considered a leading cause of vision loss in young and old people around the world. Experts confirm that early and accurate detection of vitreous inflammation can potentially reduce the rate of blindness. In this work, we have defined a CNN-based approach that allows the detection of the disease, even in early stages, i.e. retinas in day 2 of the development of the disease (see figure 16), furthermore we applied an explainable AI method (Grad-CAM) to help the clinicians to know where the disease is located and why the algorithm gives a prediction. The performances obtained are of the order of 80% in distinguishing whether the 2D image corresponds to an inflamated retina, on the different phases of the disease.
Often in clinical routine, specialists need statistical tools that can quantify the distribution of particles in diseased eyes. Since the particles size is small and annotating them with bounding boxes or masks is costly in terms of time and money, we present an automatic method to segment small particles based on a weak annotation (points). It is based on FCN8 architecture with a loss function containing 4 terms written as follows:
These terms include an image term which tends to put objects on bright areas, a consistency term between the annotations and the detected points, a consistency term between the watershed segmentation obtained from the markers given respectively by the annotations and by the detection and a last term preventing from false detections.
After having detected the particles, we consider their localization with respect to the retina and analyze their distribution using the K-Ripley function. We thus show a clustering effect that may reflect a local inflammation of the retina near the optical nerve(see figure 17). Further work will consist of grading the pathology.
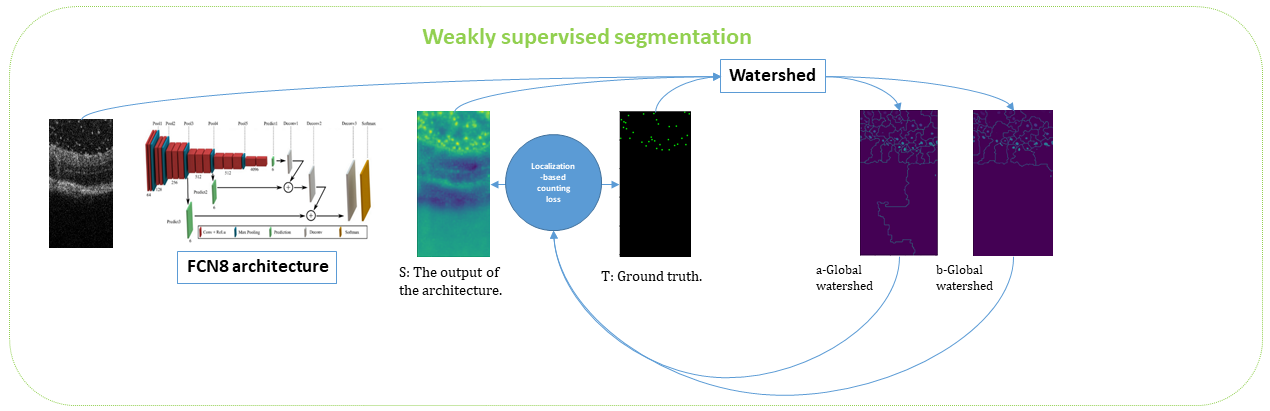
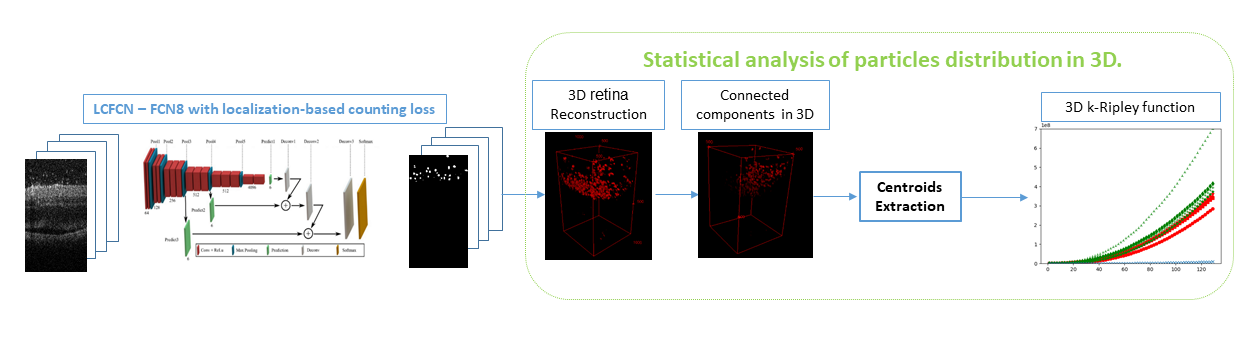
7.20 Simultaneous vascular network segmentation and classification using a multi-tasks semi-supervised neural network
Participants: Rudan Xiao, Xavier Descombes.
This work was carried out in collaboration with Damien Ambrosetti, Nice CHU.
Vascular network analysis is crucial to define the tumoral architecture and then diagnose the Renal Cell Carcinoma (RCC) subtypes. However, automatic vascular network segmentation from histopathological images is still a challenge due to the background complexity. We propose a method that reduces reliance on labeled data through semi-supervised learning (SSL). Additionally, considering the correlation between tumor classification and vascular segmentation, we propose a multi-task learning (MTL) model, shown in Fig. 18, which can simultaneously segment the vascular network using SSL and predict the tumor class in a supervised context. This multi-task learning procedure offers an end-to-end machine learning solution to jointly perform vascular network segmentation and tumor classification. We computed the ratio of miss-detected vessels (MV), the ratio of falsely detected vessels (FV) and the global performance index (IV) to evaluate our segmentation results. Fig. 19 shows the segmentation results of the proposed MTL and Tab. 1 gives a quantitative evaluation.
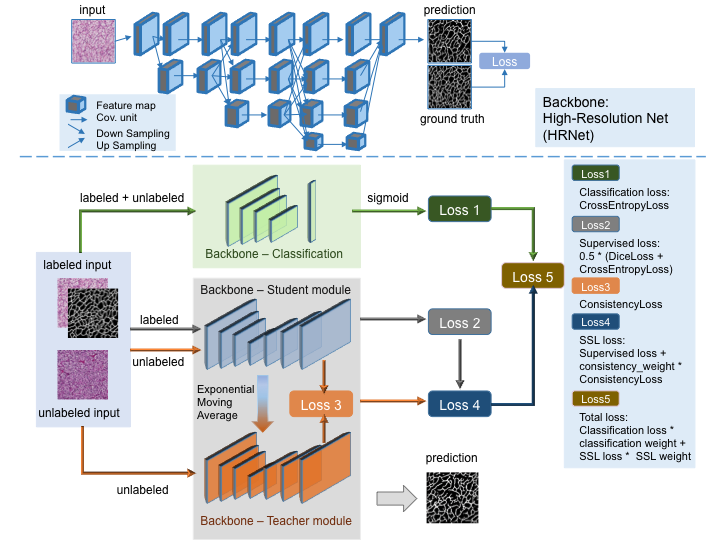
Proposed multi-task learning model architecture.
Proposed multi-task learning model architecture.
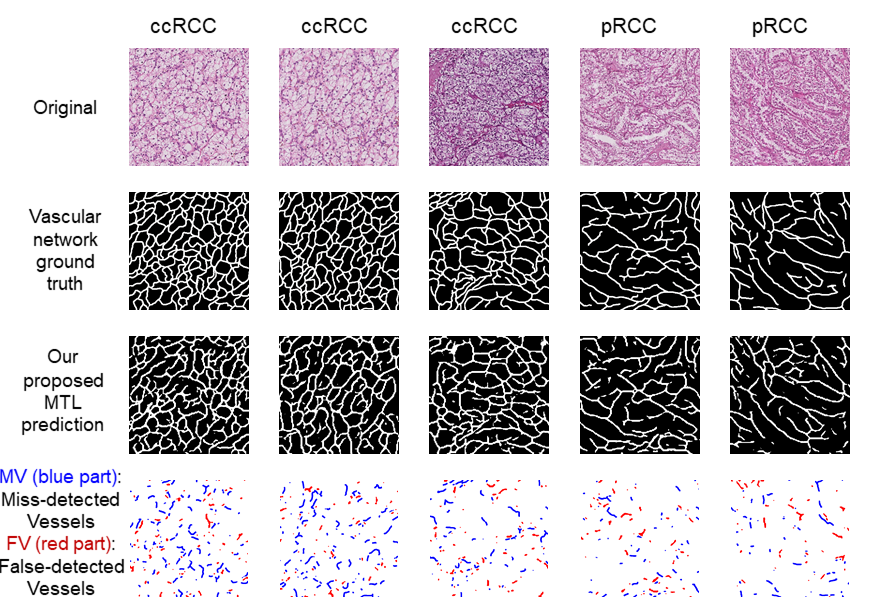
Segmentation results of the proposed model.
Segmentation results of the proposed model.
val | test | |||||
Supervised | MV | FV | IV | MV | FV | IV |
single-task | 0.247 | 0.162 | 0.796 | 0.323 | 0.118 | 0.780 |
multi-task | 0.275 | 0.131 | 0.797 | 0.302 | 0.136 | 0.781 |
Semi-Supervised | MV() | FV() | IV() | MV() | FV() | IV() |
single-task | 0.282 | 0.103 | 0.807 | 0.331 | 0.191 | 0.740 |
multi-task | 0.225 | 0.149 | 0.813 | 0.219 | 0.176 | 0.803 |
7.21 RCC classification using a deep neural decision tree
Participants: Xavier Descombes.
This work was carried out in collaboration with Francesco Ponzio from Politechnico di Torino (Italy) and Damien Ambrosetti from Nice CHU.
Renal cell carcinoma (RCC), which typically emerges from the renal tubules, is currently categorized into five main histological sub-types: clear cell, papillary, chromophobe, collecting duct, and unclassified RCC. Among them, the three most common RCCs types are clear cell, papillary, and chromophobe, including 70% to 80%, 14% to 17%, and 4% to 8% of all RCCs, respectively. Collecting duct carcinoma is the most uncommon class of RCC (1%), and unclassified RCCs gathers those alien types which do not fit, morphologically or cytogenetically, into any of the above four sub-types. The CNN (Convolutional Neural Network) appears to be a successful approach for image classification purposes. However it leads to data-hungry supervised algorithms, requiring ten of thousands of annotated images to be proficiently trained. This aspect is still an open issue that limits CNN usability in the everyday clinical setting. Transfer learning (TL), which leverages knowledge from related domains (called source domains) to facilitate the learning in a new domain (called target domain), is known to be a good solution to this problem. Originally defined as a research problem in machine learning that focuses on storing knowledge gained while solving one problem and applying it to a different but related problem, TL was initially considered capable of shifting and reusing knowledge between two similar/related domains of interest. For instance, using features embedding extracted from a nudity detection model into a new facial recognition task. Nonetheless, debate continues about the practicability of TL-based embedding in contexts distant from the original training domain, or in presence of a limited number of annotated samples. In this regard, the term negative transfer (NT) specifically refers to situations where the source knowledge can have a negative impact on the target learner, causing reduced learning performance in the target domain.
In this study, we applied several TL-based and full-trained state-of-the-art CNNs in the RCC sub-typing task. We show how both these learning paradigms failed, and, specifically, how TL led to NT, producing not satisfactory performance in the target domain. As a matter of fact, RCC sub-typing task includes those conditions known to be cumbersome even for TL-based solutions: a complex classification task, well-known to be arduous also for skilled pathologists showing a limited number of annotated samples. Our results proved on the one hand that canonical TL from general propose images, and even from other histological source domains, fail, inducing NT. We proposed a hybrid approach, leveraging a combination of TL and expert knowledge, that we named as DeepRCCTree, which was able to outperform several state-of-the-art CNNs in subtyping the RCC cancer (see figure 20). The different binary classification tasks, included in this decision tree, are directly inspired by the histopathologist practice.
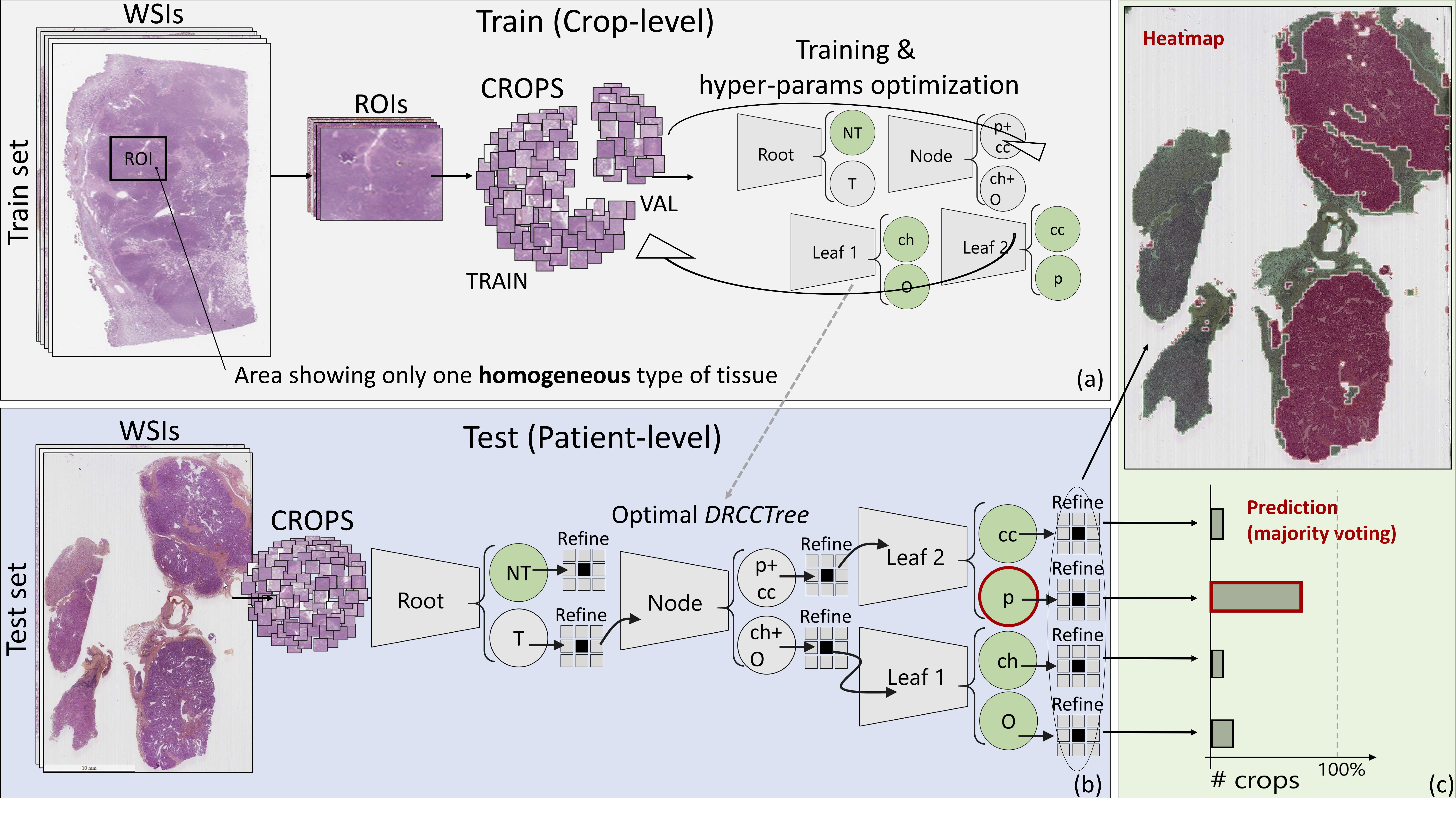
Expert-based decision tree based for histopathological image classification.
Expert-based decision tree based for histopathological image classification.
7.22 Analysis and characterization of the tissue structure of the epidermis from confocal imaging
Participants: Imane Lboukili, Xavier Descombes.
This work was carried out in collaboration with Georgios Stamatas (Johnson & Johnson Consumer Health R&D) as part of a collaboration contract with Johnson & Johnson.
We propose an automated approach to identify cells on a Reflectance Confocal Microscopy (RCM) image of skin. The study of RCM images provides information on the topological and geometrical properties of the epidermis. These properties change in each layer of the epidermis, with age and with certain skin conditions. RCM can also reveal dynamic changes to the epidermis structure in response to stimuli, as it enables repetitive sampling of the skin without damaging the tissue. Studying RCM images requires manual identification of each cell to derive geometrical and topological information, which is time-consuming and subject to human error. More insights could be derived from these data if not for the tediousness of their manual segmentation, highlighting the need for an automated cell identification method. Our automated method of cell identification on RCM images follows three general steps, (1) identifying the region of interest on the RCM image by removing any areas not containing cells, (2) identifying cells within the region of interest using different filters, and (3) rebuilding the identified cells. Pre-processing is required before each one of these steps. Accuracy assessment is complicated on real RCM images as an absolute ground truth doesn’t exist due to inter-expert variability. To assess the accuracy of our results more accurately and guide parametrization we create synthetic images similar to RCM images in their elements and noise. The obtained segmentation results show that identification of cells on RCM images is possible (see Figure 21).
A patent has been submitted for this work.
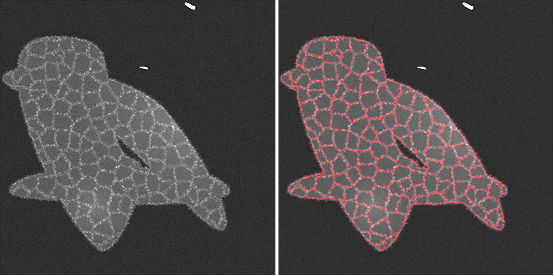
Synthetic RCM image (left) and obtained automatic cells detection of its cells (right).
Synthetic RCM image (left) and obtained automatic cells detection of its cells (right).
7.23 Classification of particle trajectories of the left-right organizer (LRO) in the zebrafish embryo
Participants: Morgane Fierville, Xavier Descombes.
This project has been conducted in collaboration with M. Fürthauer (iBV) and supported by an UCA Academy 2 project.
In this project we study the laterality of organs in the zebrafish embryo and more particularly the breaking of the right-left symmetry. This phenomenon occurs in a cavity called the Kupffer vesicle (KV) which contains particles moving in a flow. We can observe that in mutant embryos (Myo1D gene mutation), we have a disturbance of the flow in the cavity, which generates a defect of the laterality of the organs.
This year we first created velocity maps to validate the orientation of the flow in the cavity (circular in anti-clockwise). We recovered trajectory positions that were obtained through the semi-automatic tracking of exogenous particles (fluorescent beads) developed last year(see Morpheme 2020 activity report). We extrapolated the trajectories using the sliding window principle to obtain velocities at all points of the KV. We then considered a diffusion model using these velocity maps to estimate the associated parameters. This model is then used to simulate trajectories inside the cavity. These simulations lead to a reliable estimate of the density of particles in the different angular sectors of the KV and of the distribution of hitting points on the cavity membrane.
We then develop an automatic pipeline to accurately detect and track endogenous particles to avoid the injection of fluorescent beads which is invasive and can disturb the cavity flow (see Fig. 22). We first removed the heterogeneous non-stationary background from the images by substracting a local time mean. Then, we projected the image sequences into a space to observe the trajectories as tubular structures. We enhanced the contrast of linear structures (Gabor and Sato filters) in this representation. Finally, we thresholded, skeletonized and projected back the images in the initial plane to obtain the position of the trajectories over time.
Endogenous particles trajectories are sparse motivating the use of simulated additional trajectories to compute the particle density statistics.
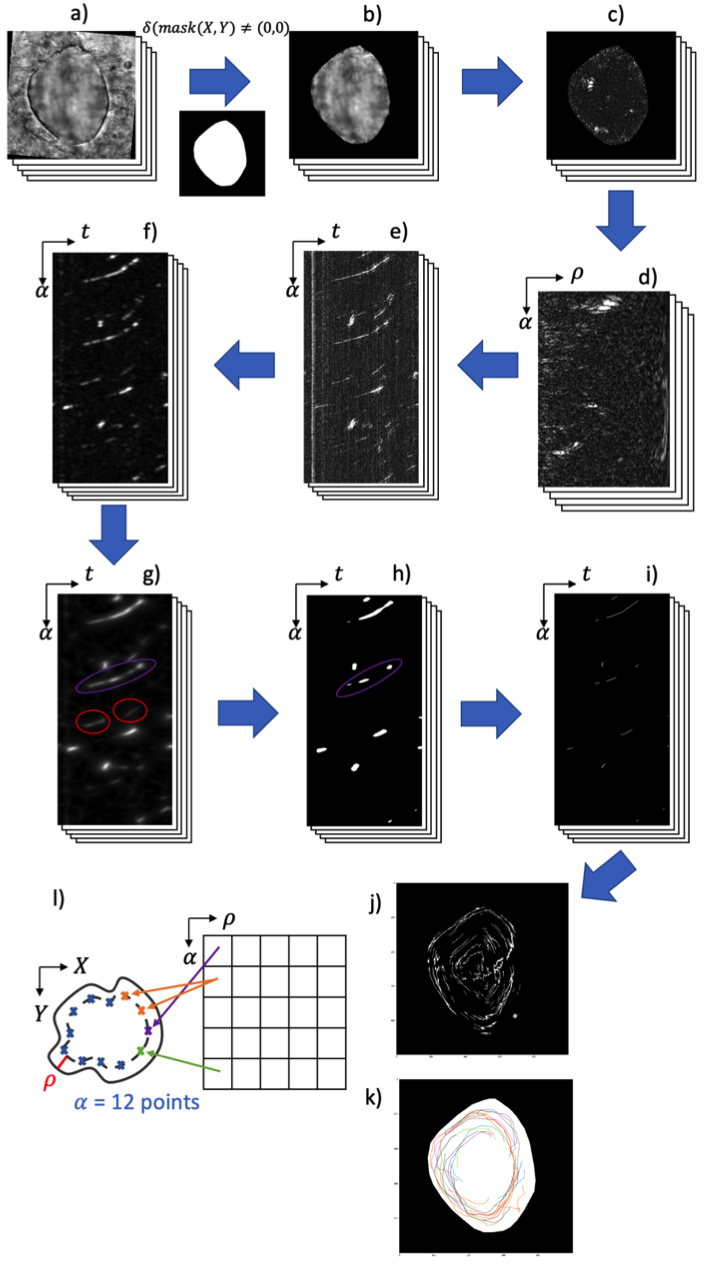
Image processing pipeline for endogenous particle detection. a) 3D image sequences; b) KV outlined; c) image after deletions of the KV background; d) transformations in (ρ,α,z)(\rho , \alpha , z); e) visualization of linear structures in (α,z)(\alpha , z) ; f) directional filtering with Gabor (frequency = 0.2, θ=45o\theta = 45^{o}); g) filtering with Sato 2D (σ∈[5,20])(\sigma \in [5, 20]) ; h) binarization with Yen method ; i) 3D skeletonization; j) transformation into (x,y,z)(x, y, z) and maximum intensity projection (MIP) of trajectories; k) visualization of the manually detected trajectories; l) diagram for the transformation into (ρ,α,z)(\rho , \alpha , z).
7.24 Robust plant cell tracking
Participants: Grégoire Malandain.
This work was carried out in collaboration with Manuel Petit, Guillaume Cerutti and Christophe Godin from Mosaic Inria team, within the IPL Naviscope (section 9.3.5).
Automatic tracking of cell deformation during development using time-lapse confocal microscopy is a challenging task. In plant cell tissues, large deformations and several division cycles can occur between two consecutive time-points making the image registration and tracking procedure particularly difficult. Here, we propose an iterative approach where an initial registration transformation and cell-to-cell mapping are alternatively refined using high-confidence associations selected on the basis of a geometric context preservation score. The method, evaluated on a long time-lapse series of floral meristem, clearly demonstrates its superiority over a non-iterative approach. In addition, we show that the geometric context preservation score can be used to define a lineage quality assessment metric that makes it possible for an expert to provide locally nudges to finalize the lineage detection if necessary in a semi-automatic way.
7.25 Ascidian embryo cell naming
Participants: Grégoire Malandain.
This work has been carried out in collaboration with Killian Biasuz, Benjamin Gallean and Patrick Lemaire from CRBM, Montpellier, within the ANR Cell Whisper (section 9.3.4).
Since the pioneering work of Conklin 40, it is admitted that ascidian exhibited a stereotyped development in the first stages of their embryogenesis. As a consequence, embryo cells can be unambiguously canonically named after their fate: this provides a common reference space into which population of inviduals can be projected and compared (see Fig. 23). Moreover, this stereotypy also provides a means to name a new sequence from already named ones.
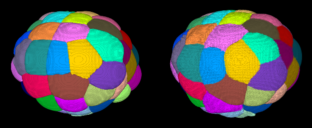
Two ascidian embryos with cells colored according to their name: this emphasize the development stereotypy.
Two ascidian embryos with cells colored according to their name: this emphasize the development stereotypy.
In 5, we proposed a pipeline to segment and track the embryo cells over several generations from a temporal sequence of light-sheet microscopy 3D images. This provides not only the cell segmentations and lineage but also numerous quantitative measurements (volume, contact surfaces). This work resulted in 10 developing embryos almost entirely named (through visual inspection), or atlases.
We hypothetized that the development stereotypy induces cell neighborhood invariance. Thus the cell neighborhood can be viewed as a cell fingerprint. The definition of cell-to-cell as well as division-to-division distances allows then to name a newly acquired embryo from one or several atlases. As a side result, it also provides means to investigate the developmental variability at a cellular level.
7.26 Morphogenesis of the sea urchin embryo
Participants: Grégoire Malandain.
This work is made in collaboration with Barthélémy Delorme and Matteo Rauzi (iBV, Nice).
The project aims at understanding the mechanisms driving the larval gut formation in the embryo of the sea urchin Paracentrotus lividus 45.
We use the Astec pipeline (see section 6.1.1 and 5) to process several sets of data and performed targeted quantifications to improve our understanding of the archenteron formation.
The sea urchin embryo is composed of a simple epithelium – a layer of mechanically coupled cells – forming a hollow, bell-shaped organism. At a given point of the embryo - known as the vegetal pole, the epithelium folds and elongates concomitantly to form the rudiment of a tube, the archenteron (Figure 24).
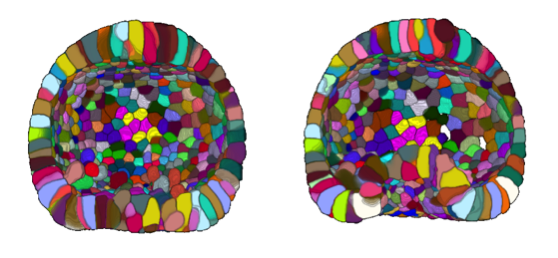
One mechanism commonly found in various model systems that drives tissue folding is apical constriction: a group of cells reduce their surfaces on one side and expand on the opposite side 46. In the sea urchin embryo, a ring of apically constricted cells is indeed present during archenteron invagination (blue cluster on Figure 25 A). Nevertheless, we also imaged and analyzed a mutant that does not display archenteron invagination: the ring-shaped cluster of apically constricted cells is present too (cf Figure 25 B), with similar characteristics (number of cells and level of constriction) (cf Figure 25 C&D). Hereby we demonstrate for the first time that apical constriction is not sufficient to drive archenteron folding in the sea urchin embryo.
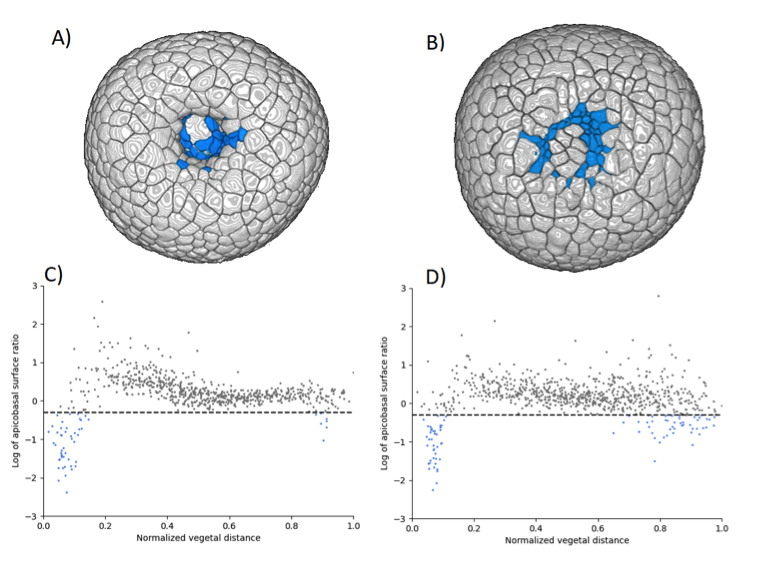
Various mechanisms may drive tissue elongation; nevertheless, neighbor exchange via cell intercalation is often found to be a key factor of extension in morphogenetic processes. The 3D segmentations allowed us to systematically track cell intercalation (cf Figure 26 A-D). We could thus demonstrate that, from the onset of archenteron invagination, the tissue elongates via cell intercalations mainly located in the region surrounding the vegetal pole (cf Figure 26 E).
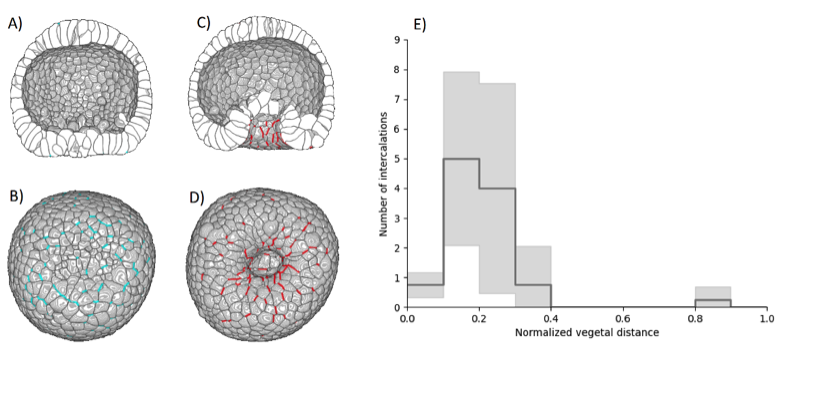
8 Bilateral contracts and grants with industry
8.1 Bilateral contracts with industry
- Bayer CropScience, Lyon: 39-month companion contract (Aug 2018 – Oct 2021) for the Cifre PhD thesis of Sarah Laroui.
- Johnson & Johnson, Santé Beauté France, Paris: 36-month companion contract (Jan 2021 – Dec 2023) for the PhD thesis of Imane Lboukili.
9 Partnerships and cooperations
9.1 International initiatives
9.1.1 CNRS IEA project VaMOS
Participants: Luca Calatroni.
This project is in collaboration with Simone Rebegoldi (University of Florence).
The purpose of this project is the design novel sparse optimisation approaches for image microscopy inverse problems. To do that, the following challenges will be addressed
- The design of new models defined in terms of tailor-made continuous and non-convex sparsity-promoting regularisations as well as non-Gaussian data terms (Poisson, Gauss + Poisson...);
- The design and theoretical analysis of new inexact and variable metric algorithms solving the models above and their MATLAB open-source implementation;
- The validation of the models and algorithms above on synthetic and real fluorescence microscopy data.
The use of inexact and variable-metric approaches for imaging inverse problems has gained a lot of attention in the recent years at an international level, but it has not been sufficiently explored by the French imaging community. Along with his collaborator S. Bonettini (UniMoRe, Italy), secondary partner of the project, S. Rebegoldi is an expert on the topic whose expertise could boost the French research activity on the topic with new ideas as well as establish long-lasting collaborations. The project will last till October 2022.
9.2 European initiatives
9.2.1 FP7 & H2020 projects
Luca Calatroni is local PI and WP coordinator of the H2020 RISE project NoMADS on Nonlocal methods for arbitrary data sources.
9.3 National initiatives
9.3.1 Labex SIGNALIFE
The Morpheme team belongs to the Labex (Laboratory of Excellence) Signalife. This Labex embeds four biology institutes in Nice, Sophia Antipolis and two Inria teams.
9.3.2 ANR JCJC RUBIN-VASE
Participants: Luca Calatroni.
This project is in collaboration with Dario Prandi (L2S)[PI], Valentina Franceschi (UniPD), Laurent Perrinet (INT) and Giuseppina Turco (LLF, Paris).
In this project we will design novel variational models defined in suitable functional spaces to translate the neurobiological principle of sensory efficient representation into mathematical terms. This will be done by addressing the following three different problems:
- The modelling of neuronal processes in V1, especially through the study of hallucinatory patterns;
- The design of a neuro-inspired framework for sound processing and speech reconstruction;
- The comparison between neurobiological models and (deep) data-driven ones, notably relying on ideas borrowed from the fields of sparse sensing and predictive coding.
Our goal is to formulate new effective reference models adapted to describe both visual and auditory perceptual phenomena, overcoming the limitations associated to WC equations and L+NL models.
This project will be carried out by coupling in parallel the development of rigorous analytical theories with their numerical and experimental validation. It features a focused team of young researchers with a synergy of combined expertise in variational methods, signal processing, optimisation, control theory, sparse coding, computational neurosciences, and speech analysis. The project will last till October 2023.
9.3.3 ANR MORPHEUS
Participants: Xavier Descombes [PI], Grégoire Malandain.
In this project we propose to use the cutting-edge organoid technology to test the toxicity of endocrine disruptors (EDCs) on human organs. The aim is to develop computational tools and models to allow the use of organoid technology for EDC toxicity testing. The project is thus divided in two main objectives: to build up and analyze a phenotypic landscape of EDC effect on organoid and to develop explicative or predictive models for their growth.The first goal is to define and construct a phenotypic map of organoids, modelled as graphs (the nodes representing the cells and edges adjacency between them) for classifying EDCs families. The second is to classify organoid growth trajectories on this map. We will consider two organoid models, gastruloids and prostate organoids. to derive the phenotypic map we will combine a graph representation and a deep learning approach. The deep learning approach will be considered for its discriminating properties whereas a correspondence between the bottleneck layer of the chosen neural network and the stratified graph space will bring some explicability to the derived classification.This 4-years project started in november 2021 and is leaded by X. Descombes. It involves 3 groups : C3M (S. Clavel, Nice), Metatox, Inserm (X. Coumoul, Paris) and Morpheme.
9.3.4 ANR Cell Whisper
Participants: Grégoire Malandain.
Successful embryogenesis requires the differentiation of the correct cell types, in defined numbers and in appropriate positions. In most cases, decisions taken by individual cells are instructed by signals emitted by their neighbours. A surprisingly small set of signalling pathways is used for this purpose. The FGF/Ras/ERK pathway is one of these and mutations in some of its individual components cause a class of human developmental syndromes, the RASopathies. Our current knowledge of this pathway is, however, mostly static. We lack an integrated understanding of its spatio-temporal dynamics and we can imperfectly explain its highly non-linear response to a graded increase in input stimulus.
This systems biology project combines advanced quantitative live imaging, pharmacological/optogenetics perturbations and computational modelling to address 3 major unanswered questions, each corresponding to a specific aim:
- Aim 1: What is the spatio-temporal dynamic of intracellular signal transduction in response to FGF (Fibroblast Growth Factor) ?
- Aim 2: What is the molecular basis of the switch-like response to graded extracellular signals?
- Aim 3: Can the results be integrated into a predictive computational model of the pathway?
Through this approach, in a simplified model system, we hope to gain an integrated view of the spatio-temporal dynamics of this pathway and of its robustness to parameter variations. Participants are CRBM (Montpellier), LIRMM (Montpellier), MOSAIC (INRIA Lyon) and Morpheme.
9.3.5 Inria Large-scale initiative Naviscope
Participants: Grégoire Malandain.
This action gathers the expertise of seven Inria research teams (Aviz, Beagle, Hybrid, Morpheme, Parietal, Serpico and Mosaic) and other groups (MaIAGE, INRAE, Jouy-en-Josas and UMR 144, Institut Curie Paris) and aimed at developing original and cutting-edge visualization and navigation methods to assist scientists, enabling semi-automatic analysis, manipulation, and investigation of temporal series of multi-valued volumetric images, with a strong focus on live cell imaging and microscopy application domains. More precisely, the three following challenges will be addressed:
- Novel machine learning methods able to detect the main regions of interest, and automatic quantification of sparse sets of molecular interactions and cell processes during navigation to save memory and computational resources.
- Novel visualization methods able to encode 3D motion/deformation vectors and dynamics features with color/texture-based and non-sub-resolved representations, abstractions, and discretization, as used to show 2D motion and deformation vectors and patterns.
- Effective machine learning-driven navigation and interaction techniques for complex functional 3D+Time data enabling the analysis of sparse sets of localized intra-cellular events and cell processes (migration, division, etc.).
9.4 Regional initiatives
9.4.1 UCA Arch-AI-story/Imag'In (PRIME CNRS)
Participants: Luca Calatroni, Laure Blanc-Féraud.
The project involves a large network of research scientists and is led by I. Théry-Parisot (CEPAM).
It focuses on the analysis of high-resolution imaging data (2D, 3D, Tomography) for the characterisation of objects at different scales such as frescoes, manuscripts, biological archives (wood, bones) and geological findings (pottery). It focuses in particular on the exploitation of the potential of applied mathematiques and artificial intelligence to Archeology and Art history. The use of tools coming from Artificial Intelligence and, in particular, of advanced imaging techniques is expected to improve the interpretation of historical and archaeological data. One specific aspect is also the make available the large databases to the AI communities.
The working package Imag'In on imaging of this project (led by Luca Calatroni and Rosa Maria Dessí, CEPAM) received the PRIME label by the CNRS (see miti.cnrs.fr/projet-multi-quipe/imagin/).
9.4.2 UCA project - Left-Right Organizer
Participants: Morgane Fierville, Xavier Descombes.
In this project we study the left-right organizer (LRO) of the zebrafish which is involved in the organ laterality. The zebrafish LRO is a vesicle in which a flow is produced by cillia beats. To study this flow we consider trajectories of endogenous and/or exogenous particles . In a previous work we have constructed a pipeline to detect the vesicles. During this new project, the goal was to develop a statistical framework to characterize the flow first by computing mean and variance velocity maps in the vesicle. We then considered a diffusion process whose parameters were estimated from the these maps. This model allows simulating new trajectories. From these simulations statistical features, such as the particles density or the distribution of hitting events on the vesicle membrane, are computed.
The project is a collaboration with M. Furthauer from iBV (Nice).
10 Dissemination
10.1 Promoting scientific activities
10.1.1 Scientific events: organisation
General chair, scientific chair
- The Morpheme group has organized the IEEE ISBI conference (International Symposium on Biomedical Imaging) with Laure Blanc-Féraud as General Chair (co-chair : F. Peyrin, INSERM, Lyon Univ.) and Grégoire Malandain as Financial Chair, with almost 800 registrations, 13-16 April.
- Laure Blanc-Féraud was scientific co-organizator of the summer school Peyresq 2021 on "Signal and Image Processing for the co-design of innovative imaging systems: physics, mathematics, algorithms", 20-26 June.
Member of the organizing committees
- Luca Calatroni has been co-organiser of mini-symposia at the following international conferences conferences: SMB 2021 (workshop Recent advances in mathematical neuroscience: cortically inspired models for vision and synaptic plasticity), ICMNS 2021 (workshop The mathematics of vision: from cortical-inspired modelling to perception), SIAM OP21 (workshop: ), IFIP 2021 (workshop: Bilevel Optimisation Approaches for Image and Data Analysis).
- Luca Calatroni has been co-organiser of the workshop Advanced optimization for inverse problems & applications to image microscopy, which took place at the University of Florence on November 22-23 2021. Co-organiser: S. Rebegoldi (University of Florence).
- Luca Calatroni has been on January 20 co-organiser of the Virtual kick-off Mathematics of the Italian Imaging, Vision & Applications (MIVA) UMI group. Co-organisers: M. Donatelli (UnInsubria), S. Morigi (UniBo), M. Prato (UniMoRe), M. Santacesaria (UniGe, MaLGA).
- Luca Calatroni has been co-organiser of the SIAM Imagine & Inverse Problems (IMAGINE) virtual series from January till December 2021. Co-organisers: E. Bonnetier (UGA), R. Chan (CityU), F. Santosa (JHU), C.-B. Schönlieb (University of Cambrige), M. NG (HKU), J. Müller (CSU).
10.1.2 Scientific events: selection
Reviewer
10.1.3 Journal
Member of the editorial boards
- Luca Calatroni is Topic Editor of the MDPI Journal of Imaging.
- Luca Calatroni is chief Guest Editor of the Cambridge Elements series on Non-local data interactions: foundations & applications from the Cambridge University Press.
- Laure Blanc-Féraud is Area Editor for the Feature articles of IEEE Signal Processing Magazine.
- Laure Blanc-Féraud is member of the editorial board of the encyclopedia SCIENCES of ISTE/Wiley, responsable of the Image Domain with about fifteen books to be published.
- Laure Blanc-Féraud was member of the editorial board of the book "Le corps en image" to appear at CNRS editions (with Emmanuel Caruyer, Hervé Liebgott and Christian Jutten).
- Xavier Descombes is associate editor of Digital Signal Processing journal.
Reviewer - reviewing activities
- Luca Calatroni has been reviewer for: SIAM Journal on Imaging Sciences - SIAM Journal on Optimization - Journal of Mathematical Imaging and Vision - Medical Image Analysis - Inverse problems & imaging - Computers and Mathematics with Applications - Applied Mathematical Modelling - Eletronic Transactions on Numerical Analysis - Signal processing: Image communication - Applied Mathematics and Computation - Journal of Imaging.
- Eric Debreuve has been reviewer for: Digital Signal Processing (Elsevier) - Entropy (MDPI).
- Xavier Descombes has been reveiwer for MeDdIA (Medical Image Analysis).
10.1.4 Invited talks
- Luca Calatroni has been invited to present his work at the following international conferences: SIAM LA2021, ICSSA 2021, IFIP 2021 (2 talks), SIMAI 2021 (2 talks).
- Luca Calatroni has been invited speaker at the Workshop Optimization techniques for Inverse Problems on September 6-7 2021.
- Luca Calatroni has been invited to present his work in the occasion of the following seminars: OneWorld IMAGINE webinars, IRIT SC seminars (University of Toulouse), SNAP seminars (University of Naples), ModeMat seminars (ESN), FIM seminars (UniMoRe), Numerical Analysis Seminars (UniFe), Numerical Analysis Seminars (UniBo), McTAO seminars (Inria SAM).
- Laure Blanc-Féraud has been invited speaker at the workshop Advanced optimization methods for inverse problems and applications to image microscopy Florence, Italy, 22-23 November 2021 and the AI/Bio workshop, one-day online workshop of three Lyon's federations, 1 October, 2021.
- Laure Blanc-Féraud has been Keynote Speaker at the IEEE International Conference on Antenna Measurements and Applications (CAMA), Antibes Juan-les-pins, 15-17 November 2021.
- Xavier Descombes has presented the Morpheus project during the annual C45 ANR meeting for Laureat presentation, 7 December, 2021.
- Marta Lazzaretti has been invited to present her work at the following conferences: PRIMO workshop, YAMC 2021 conference, SIMAI 2021, SSVM 2021 (poster), ISBI 2021 (poster).
- Vasilina Stergioupolou has been invited to present her work at the following conferences: 3IA PhD/post-doc seminars, 3IA scientific days (poster), ISBI 2021 (poster).
10.1.5 Leadership within the scientific community
- Luca Calatroni is the responsible of the Italian group on the Mathematics of Imaging, Vision and Applications (MIVA), together with M. Donatelli (UnInsubria), S. Morigi (UniBo), M. Prato (UniMoRe), M. Santacesaria (UniGe, MaLGA).
- Luca Calatroni is P.I. of the GdR ISIS project "SParse & non-convex optimisation for Learning of INverse image microscopy problems" (SPLIN), 7k€, for the period 2021-2023.
- Luca Calatroni is co P.I. (together with S. Rebegoldi) of the CNRS IEA project "Variable-Metric & inexact sparse Optimisation for Super-resolution microscopy" (VaMOS), 12 k€, for the period 2021-2023.
- Luca Calatroni is co P.I. (together with R. M. Dessì) of the CNRS PRIME project "Transformation and destruction processes of painted images" (Imag'In), UCA, 16 k€, for the period 2021-2023.
- Luca Calatroni is work package leader (together with R. M. Dessì) of the project "Applied mathematics and artificial intelligence potential for archaeology and art history" (Arch-AI-story), P.I.: Isabelle Thery-Parisot, UCA.
- Luca Calatroni is work package leader and local PI of the H2020 RISE project “Nonlocal methods for arbitrary data sources" (NoMADS).
- Laure Blanc-Féraud is member of the IEEE BioImaging and Signal Processing technical committee.
- Laure Blanc-Féraud is responsible of the axis 3 on IA for Biology and biology for IA of the 3IA Côte d'Azur Institute and member of the scientific committee of 3IA Côte d'Azur Institute.
- Xavier Descombes is member of the scientific council of Labex Signalife.
- Xavier Descombes is P.I. of the ANR project Morpheus, 657 k€ for the period 11/21-10/24.
- Xavier Descombes is co P.I. of the UCA project "Left Right Organizer", 24 k€ for 2021.
- Grégoire Malandain is member of the IEEE Biomedical Imaging and Image Processing technical committee.
- Grégoire Malandain is member of the scientific committee of the MATHNUM department of INRAE.
10.1.6 Scientific expertise
- Luca Calatroni has been evaluator of research grant proposals for the Progetti di Ricerca d'Interesse Nazionale (PRIN) projects, Italy.
- Luca Calatroni has been evaluator of a post-doctoral application for the Research Foundation Flanders (FWO) projects, Belgium.
- Laure Blanc-Féraud has been evaluator of research grant proposals for the Progetti di Ricerca d'Interesse Nazionale (PRIN) projects, Italy.
- Laure Blanc-Féraud has been part of the HCERES evaluation committee of the GREYC Laboratory and Normastic federation.
- Xavier Descombes has been a reviewer for a NSERC (Canada) computer science project and for an ANR project.
- Xavier Descombes has served as an external reviewer for an ANR project.
- Xavier Descombes is an expert for French Research Ministry to evaluate companies CIR ("Crédit Impôt Recherche").
10.1.7 Research administration
- Laure Blanc-Féraud was part of the selection committee for a professor position in Machine Learning at University Côte d'Azur.
- Laure Blanc-Féraud is member of the CAP (Comission Administrative Paritaire) at CNRS for the "chargés de recherche".
- Laure Blanc-Féraud is member of the board of the Academy 1 of the Idex UCA.
10.2 Teaching - Supervision - Juries
10.2.1 Teaching
- Licence: Florence Besse, Subcellular RNA targeting, 4h, L3, ENS Ulm, France
- Master: Florence Besse, Neurobiologie cellulaire et moléculaire, 6h, M1/2, Université Côte d'Azur, France
- Master: Florence Besse, Outils génétiques pour l’étude des circuits neuronaux, 4h, M1/2, Université Côte d'Azur, France
- Master: Florence Besse, Transport axonal, 2h, M1/2, Université Côte d'Azur, France
- Master: Laure Blanc-Féraud, module Machine Learning for Image processing and module Applied AI of master SD, 12h Eq. TD, Niveau M2, Université Côte d’Azur, France.
- Master: Eric Debreuve, Scientific image processing and Machine learning, Master SVS, 15h EqTD, M1 + M2, Université Côte d'Azur, France
- Master: Xavier Descombes, Traitement d’images, Analyse de données, Techniques avancées de traitement d’images, 10h Eq. TD, Niveau M2, ISAE, France.
- Master: Xavier Descombes, Polytech Sophia, master, 9h Eq. TD, Niveau M2, Université Côte d’Azur, France.
- Master: Xavier Descombes, Bio-imagerie, master IRIV, 6h Eq. TD, Niveau M2, Université de Strasbourg, France
- Master: Xavier Descombes, Analyse d’images, master GBM, 9h Eq. TD, Niveau M2, Université Côte d’Azur, France.
- Master: Xavier Descombes, Traitement d'images scientifiques, master SVS, 15h Eq. TD Niveau M2, Université Côte d'Azur.
- Master: Xavier Descombes, Artificial Intelligence for Histopathologist, master oncology, 3h Eq. TD, Niveau M2, Université Côte d'Azur.
- Master/Ingénieur: Sarah Laroui, Data Science, M2/Ingénieur 5, Niveau 5eme année ingénieur, 7.5h Eq. TD, Polytech Nice Sophia - Univ Côte d'Azur , France.
- IUT 1ere année: Sarah Laroui, Acquisition et codage de l'information, niveau 1ère Année DUT RT, 13.5h Eq. TD, Institut Universitaire de Technologie (IUT) Sophia, France.
- PhD course Peyresq summer school: Luca Calatroni, Bilevel optimisation for hyperparameter estimation in imaging inverse problems, 2h, virtual, France.
- Master: ECUE histoire de l'histoire de l'art: Luca Calatroni, Where arts & mathematics meet, niveau M1, 4h, Université Côte d'Azur, France.
- DUT : Cédric Dubois, Programmation, 26h TD, IUT QLIO, Qualité, Logistique Industrielle et Organisation, 2ième année (alternants), Université Côte d'Azur, France. Dans le cadre d'un DCME (64h Eq. TD annuelles).
- DUT : Cédric Dubois, Chaîne de transmission numérique 38h Eq. TD, IUT RT, Réseaux et Télécommunications, 1ère année, Université Côte d'Azur, France. Dans le cadre d'un DCME (64h Eq. TD annuelles).
- MSc Data Science: Vasiliki Stergiopoulou, Machine Learning in Image Analysis (2h).
- BUT RT Signaux et Systèmes pour les transmission (R205): Vasiliki Stergiopoulou, Numérisation de l'information (R206) (in total 60h).
- Licence in Mathematics, University of Genova: Marta Lazzaretti, tutoring classes in Algebra I, Calculus, Linear Algebra and Analytical Geometry (in total 30h).
- Polytech L2 : Bastien Laville, Automatic, 39 hours (2nd year PeiP, equivalent to L2) Polytech Sophia Antipolis, UCA.
- Polytech L3 : Bastien Laville, mathematics, 15 hours, L3 Polytech Nice Sophia Antipolis, UCA.
10.2.2 Supervision
- Luca Calatroni and Laure Blanc-Féraud have supevised the internship of Mayeul Cachia on the development of GANs for super-resolution microscopy, from May till November 2021.
- Luca Calatroni and Xavier Descombes have supervised the internship of Rim Rekik Dit Nekhili on the development of CNNs for learning image regularization models from March till July 2021.
- Luca Calatroni has supervised (with S. Morigi, University of Bologna) the internship of Giuseppe Recupero on the development of non-linear differential models for shadow removal, from April till July 2021.
- Xavier Descombes has supervised the internship of Youness Mellak, with Professor Alin Achim from Bristol University, on Retina OCt analyzis and classification for early diagnosis of uveitis, March till July 2021.
- Xavier Descombes and Sarah Laroui have supervised the internship of Oumayma Aguili on Image Segmentation using Caricature, appliction to phyto-pathogeneous fungi, March till July 2021.
- Xavier Descombes has supervised the internship of Morgane Fierville on modeling particles trajectories in zebrafish left-right organizer, january till June 2021.
- Xavier Descombes has supervised the internship of Marina Battlo Ruis on nuclei detection from histopathological images, january till march 2021.
- Eric Debreuve has supervised the 2-month internship of Thissas Benkaroun, Master 1, on the development of a Graphical User Interface for the image processing pipeline of the NutriMorph project.
- Eric Debreuve was supervisor and Xavier Descombes co-supervisor of Sarah Laroui's PhD.
- Eric Debreuve is supervisor of Cedric Dubois's PhD (in collaboration with J.-O. Irisson, LOV, Villefranche-sur-mer).
- Eric Debreuve is co-supervisor of Asma Chalabi's PhD (supervised by J. Roux, Ircan, Nice).
- Eric Debreuve is co-supervisor of Clara Sanchez's PhD (supervised by C. Rovère, IPMC, Sophia Antipolis).
- Xavier Descombes is supervisor of Rudan Xiao's PhD (in collaboratiopn with D. Ambrosetti, Nice Hospital).
- Xavier Descombes is supervisor of Imane Lboukili's PhD (in collaboration with G. Stamatas at Johnson & Johnson).
- Laure Blanc-Féraud is supervisor and Luca Calatroni cosupervisor of Vasiliki Stergiopoulou's PhD.
- Laure Blanc-Féraud is supervisor and Gilles Aubert cosupervisor of Bastien Laville's PhD.
- Luca Calatroni is supervisor of Marta Lazzaretti's PhD (co-tutelle with professor Claudio Estatico, University of Genova, Italy).
- Grégoire Malandain is co-supervisor of Manuel Petit's PhD (also co-supervised by C. Godin, Mosaic, Lyon).
10.2.3 Juries
- Laure Blanc-Féraud was president of 2 PhD juries and one HDR jury and she was reviewer for two HDR.
- Xavier Descombes was reviewer of one PhD jury and member of a second one.
10.3 Popularization
- Rudan Xiao took part in "Fête de la Science" in Nice in October.
11 Scientific production
11.1 Major publications
-
1
articleA continuous relaxation of the constrained
problem.Journal of Mathematical Imaging and VisionJanuary 2021 - 2 articleDetecting and quantifying stress granules in tissues of multicellular organisms with the Obj.MPP analysis tool.TrafficJuly 2019
- 3 articleMultiple objects detection in biological images using a marked point process framework.Methods2016
- 4 articleFibronectin Extra Domains tune cellular responses and confer topographically distinct features to fibril networks.Journal of Cell ScienceFebruary 2021
- 5 articleContact area-dependent cell communication and the morphological invariance of ascidian embryogenesis.ScienceJuly 2020
- 6 miscOn and beyond Total Variation regularisation in imaging: the role of space variance.April 2021
- 7 miscCOL0RME: Super-resolution microscopy based on sparse blinking fluorophore localization and intensity estimation.August 2021
11.2 Publications of the year
International journals
International peer-reviewed conferences
Conferences without proceedings
Scientific book chapters
Reports & preprints
11.3 Cited publications
- 39 articleA Fast Iterative Shrinkage-Thresholding Algorithm for Linear Inverse Problems.SIAM Journal on Imaging Sciences212009, 183-202
- 40 bookThe organization and cell-lineage of the ascidian egg / by Edwin G. Conklin..Philadelphia :[Academy of Natural Sciences],1905, 182URL: http://www.biodiversitylibrary.org/item/24005
- 41 inproceedingsLSPARCOM: Deep Unfolded Super-Resolution Microscopy.Imaging and Applied Optics CongressOptical Society of America2020, JW5A.5URL: http://opg.optica.org/abstract.cfm?URI=AOMS-2020-JW5A.5
- 42 inproceedingsHigh density molecule localization for super-resolution microscopy using CEL0 based sparse approximation.Biomedical Imaging (ISBI 2017), 2017 IEEE 14th International Symposium onIEEE2017, 28--31
- 43 articleDigital zooplankton image analysis using the ZooScan integrated system.Journal of Plankton Research32303 2010, 285--303URL: https://doi.org/10.1093/plankt/fbp124
- 44 articleCryoGAN: A New Reconstruction Paradigm for Single-Particle Cryo-EM Via Deep Adversarial Learning.IEEE Transactions on Computational Imaging72021, 759-774
- 45 articleGastrulation in the sea urchin embryo: a model system for analyzing the morphogenesis of a monolayered epithelium..Dev Growth Differ464August 2004, 309-26
- 46 articleApical constriction: themes and variations on a cellular mechanism driving morphogenesis..Development14110May 2014, 1987-98
- 47 articleAlgorithm Unrolling: Interpretable, Efficient Deep Learning for Signal and Image Processing.IEEE Signal Processing Magazine3822021, 18-44
-
48
article
Gradient Projection.IEEE Transactions on Image Processing2642017, 1554-1564 -
49
articleA Unified View of Exact Continuous Penalties for
Minimization.SIAM Journal on Optimization2732017, 2034--2060 - 50 articleImage Super-Resolution Via Sparse Representation.IEEE Transactions on Image Processing19112010, 2861-2873
- 51 inproceedingsMSR-GAN: Multi-Segment Reconstruction via Adversarial Learning.ICASSP 2021 - 2021 IEEE International Conference on Acoustics, Speech and Signal Processing (ICASSP)2021, 5115-5119
- 52 inproceedingsLearning Deep CNN Denoiser Prior for Image Restoration.2017 IEEE Conference on Computer Vision and Pattern Recognition (CVPR)2017, 2808-2817