Keywords
Computer Science and Digital Science
- A1.1.9. Fault tolerant systems
- A1.2.2. Supervision
- A1.2.4. QoS, performance evaluation
- A1.2.5. Internet of things
- A1.3.3. Blockchain
- A1.3.4. Peer to peer
- A1.3.5. Cloud
- A3.4.1. Supervised learning
- A3.4.2. Unsupervised learning
- A3.4.3. Reinforcement learning
- A3.4.6. Neural networks
- A3.4.8. Deep learning
- A6.1.2. Stochastic Modeling
- A6.2.3. Probabilistic methods
- A6.2.4. Statistical methods
- A6.2.6. Optimization
- A8.2.1. Operations research
- A8.6. Information theory
- A8.7. Graph theory
- A8.11. Game Theory
- A9.2. Machine learning
- A9.7. AI algorithmics
Other Research Topics and Application Domains
- B6.2.1. Wired technologies
- B6.2.2. Radio technology
- B6.2.4. Optic technology
- B6.3.2. Network protocols
- B6.3.3. Network Management
- B6.3.5. Search engines
- B6.4. Internet of things
- B9.6.3. Economy, Finance
1 Team members, visitors, external collaborators
Research Scientists
- Bruno Tuffin [Team leader, INRIA, Senior Researcher, HDR]
- Maël Le Treust [CNRS, Researcher, HDR]
- Raymond Marie [UNIV RENNES I, Emeritus, HDR]
- Gerardo Rubino [INRIA, Emeritus, HDR]
- Bruno Sericola [INRIA, Senior Researcher, HDR]
Faculty Members
- Soraya Ait Chellouche [UNIV RENNES I, Associate Professor]
- Yann Busnel [IMT ATLANTIQUE, Professor, HDR]
- Yassine Hadjadj-Aoul [UNIV RENNES I, Professor, HDR]
- Sofiene Jelassi [UNIV RENNES I, Associate Professor]
- Patrick Maillé [IMT ATLANTIQUE, Professor, HDR]
- César Viho [UNIV RENNES I, Professor, from Sep 2022, HDR]
Post-Doctoral Fellow
- Abdellah Kaci [UNIV RENNES I, from May 2022]
PhD Students
- Zahra Al Attar [IMT Atlantique, co-supervised by a team member]
- Ahcene Boumhand [Orange until , CIFRE]
- Abdelmounaim Bouroudi [NOKIA BELL LABS]
- Nicolas Charpenay [Université de Rennes 1, co-supervised by team a member]
- Khalil El Houssni [IMT ATLANTIQUE]
- Sid Ali Hamideche [NOKIA BELL LAB until Jul. 2023, CIFRE]
- Ali Hodroj [Université Saint Joseph de Beyrouth]
- Soumeya Kaada [NOKIA, until Nov 2022]
- Léo Lavaur [IMT Atlantique, co-supervised by a team member]
- Pierre-Marie Lechevalier [IMT Atlantique, co-supervised by a team member ]
- Fatima Zahra Mardi [Université Moulay Ismail Meknès, Maroc, from Dec 2022, Doctorante en mobilité]
- Antoine Rebstock [IMT ATLANTIQUE]
- Amine Rguez [EXFO SOLUTIONS]
Technical Staff
- Ghina Dandachi [INRIA, Engineer]
- Antoine Lesieur [INRIA, Engineer, from Nov 2022]
- Mohammed Islam Naas [INRIA, Engineer, from Nov 2022]
Interns and Apprentices
- Kaouther Boulaouinat [Université Saint Joseph de Beyrouth, until Jun 2022]
- Hibat Djecta [Ecole Nationale Supérieure d’Informatique (ESI), Alger, Algérie]
- Florent Dufay [Inria, from Jun 2022 until Sep 2022]
- Imene Goucem [Université Mouloud Mammeri de Tizi Ouzou, from Jun 2022 until Oct 2022]
Administrative Assistant
- Gwenaelle Lannec [INRIA]
Visiting Scientists
- Soledad Collazo [UBA (Université de Buenos Aires, Argentine), projet Climate AmSud « ClimateDL »]
- Jorge Graneri [Université de la République, Montevideo, Uruguay, from Jul 2022 until Aug 2022, Projet MASC (Mathematical Algorithms for Semantic Cognition)]
- Pablo Rodriguez [Université de la République, Montevideo, Uruguay, from Jul 2022 until Jul 2022]
2 Overall objectives
Networks are omnipresent and increasingly complex, and require an efficient management of their operations. The ERMINE team designs and analyzes procedures and policies for efficiently managing network operations, but also works on the required measurement and monitoring of performance metrics. Our specific and original management activity focuses on network economics, regulation, and automated decision making. In terms of needed measurement, we make use of standard modeling and performance analysis techniques, but also carry out direct measurements to be analyzed statistically. A cross-cutting research axis for both management and measuring is artificial intelligence. Our activity is a trade-off between methodological/mathematical developments and practical implementations.
3 Research program
3.1 Context
Telecommunication networks are ubiquitous in our daily life. The network ecosystem and its infrastructure are increasingly complex with more and more involved actors and technologies which have to interoperate. This complexity is illustrated in Figure 1, where
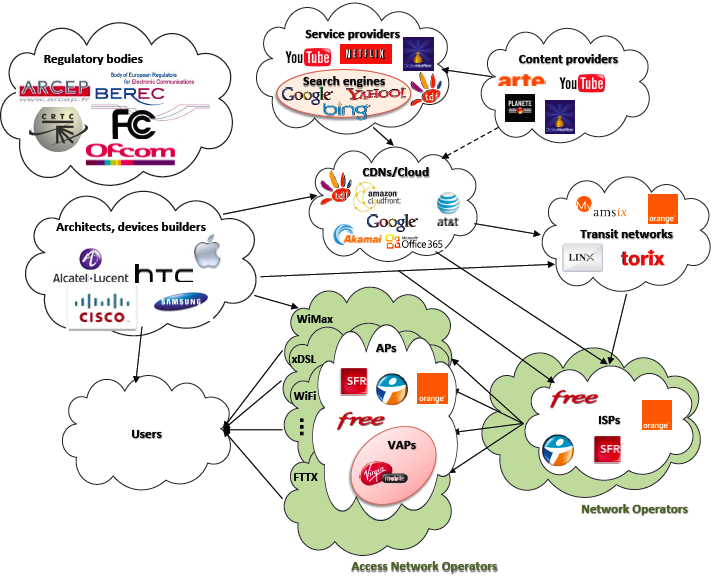
Description of the various actors on the ICT (for Information & Communications Technology) ecosystem
the basic network paradigm with network operators simply sending content from/to end users has moved to a topology with many players having different roles. A complete telecommunications system has to integrate traditional networks capabilities but also all other innovative Internet services into a single service platform. Selfishness of actors, heterogeneity of requests and technologies, interoperability of services and automation of decisions are major challenges to be tackled.
ERMINE deals with network operations management and the required associated monitoring and measurements and performance analysis. Operation network management means for us performance-based management, fault analysis, or the design of procedures and strategies for resource provisioning and quality-of-service fulfillment. On this management side, we aim at looking at the whole topic of networks economics, studying the best economic strategies of actors of the digital economy, their interactions and the potential need for regulation. We also want to address resource management through artificial intelligence for automated decision as another main research direction. On the measurement side, monitoring services of all sorts is a major challenge for regulatory bodies to ensure a fair and legal behavior of providers, and is also of primary importance for providers themselves to optimize decisions; this often requires new modeling, analysis and artificial intelligence tools. This is detailed below.
3.2 Managing network operations
The heterogeneity and complexity of the ICT (for Information & Communications Technology) world bring a number of challenges to network operations management. ERMINE addresses key issues for network management: i) the challenges of ICT economics; ii) network control and interoperability iii) automated decisions through AI.
ICT is omnipresent in our modern society, and the economy has gone beyond the industrial economy to the Internet and ICT economy. Thanks to hyper-connectivity, there are now lots of opportunities for innovation. As of May 2020, among the top-10 most valuable companies worldwide, seven are ICT companies, trusting the places from 2 to 8 (Microsoft, Apple Inc., Amazon Inc., Alphabet Inc., Facebook, Alibaba Group, Tencent). Internet Service Providers (ISPs), Content Delivery Networks (CDNs) and cloud providers, social network actors, all services and content providers are among actors needing a business model as profitable as possible. Designing and analyzing such business models and their acceptance by end users are issues to be addressed, leading to challenges in terms of outcome for all players (who act in most cases selfishly) and in terms of benefits for society. On that last point, regulators have to determine rules that actors need to follow in order to avoid harmful behaviors and maximize social welfare. The issues we address include: the design of economic rules for new services, the analysis of the impact of players decisions and interactions, and the potential design of rules or incentives from regulatory bodies leading to the most adequate (social) outcome. One of the frameworks to be used is that of game theory, and in particular of mechanism design.
There has been a metamorphosis in the last few years on the management of network operations, driven by the virtualization of networks and services. This evolution allows to meet the needs in terms of the dynamic scaling of infrastructures and the agility of the decision-making, namely, a necessary prerequisite for reducing operating costs as well as improving return on investment. These developments are radically changing the way services are managed, as they become more complex (i.e., services in the form of graphs, micro-services, etc.), their expectations can be very diverse and strategies for their placement could consider a single domain or spanning across several domains, whether cooperative or not. Thus, the operation of the network becomes extremely difficult and requires not only optimization of resources but also economic considerations, especially when management involves several domains. We believe that automated management and control is the key direction for an efficient solution. We deal with the automation of the network by contributing to ongoing standardization efforts, notably by the “Zero touch network & Service Management” (ZSM) group of the ETSI (the European Telecommunications Standards Institute), and through the elaboration of solutions based on the most recent advances in machine learning techniques, and in particular in deep reinforcement learning. Some of the challenges we tackle include dynamic placement of complex and constrained – QoS/QoE – services (i.e., network slicing), automatic service scaling, congestion control in the context of new generation networks, including IoT networks (e.g., massive Machine-Type Communications (mMTC)). Different aspects are taken into account when developing the various solutions, including reliability, resilience, guaranteed performance (i.e., deterministic networks), but also the energy efficiency on which the viability of the latter depends. Ensuring the performance of the solutions when using machine learning techniques is, however, a major problem that is poorly addressed in the literature. We address it by proposing techniques that offer at least similar performances to heuristics. Indeed, even if heuristics can be very efficient, they often have, however, some limitations. One of these is the blocking at the level of local minima. AI techniques can address this issue encountered in combinatorial problems (of the NP-hard type, such as the travelling salesman one). They sometimes yield near-optimal results. AI techniques have the additional advantage of being able to learn by interacting with the environment, and can therefore find solutions that heuristics could not.
3.3 Measuring
A proper management of network services and operations cannot be effective without measurement/monitoring and without the analysis of relevant metrics. Indeed, decision making cannot be realized without knowledge and data on the past, present and even future status of the activity. This is typically the case for agile capacity planning and resource sharing for which usage needs to be computed and even predicted. This is a challenge for which tools coming from AI are very promising. Another relevant example of challenge is measurements for regulation purposes, in relation with our activity on network economics: defining regulation rules means designing measurement procedures to verify that the rules are followed. It is for example required to monitor a neutral behavior of ISPs as expected from the Net neutrality principles. While there exist a few tools towards that goal, they are actually all devoted to very specific hindrances to neutrality (blocking, degradation) for specific types of flows or traffic; a major challenge is to detect any type of non-authorized behavior. Many other actors of the ICT economy, if not all, are barely monitored while an unfair behavior could be seen as harming end users and society. To name some noticeable examples, we can cite CDNs which cache some content at the edge of the network and could unfairly propose a better quality to selected customers, or search engines who can prioritize the web. In all those cases, different and specific techniques have to be designed to analyze and detect unexpected behaviors.
Similarly, managing resources and operations calls for evaluating (measuring) the impact of decisions to determine the most appropriate ones. Modeling and performance evaluation techniques are appropriate and useful solutions at a low cost, without the need to build and run the real system. While we have in the group a long experience on performance evaluation, new challenges keep popping up due to new types of services translated into new problems to be modeled and analyzed through performance evaluation tools, and adaptations or extensions of existing techniques for better decision making. They usually require to develop new appropriate network metrics to assess network service operations effectiveness. Illustrative examples are blockchains. The popularity of blockchain lies in the introduction of the concept of a public distributed ledger shared by all participants of the system without relying on a centralized authority. This distributed ledger records all the transactions between parties efficiently and in a verifiable and permanent way. In order to enjoy higher throughput and self-adaptivity to transactions demand in ICT, it requires the development of a new architecture and thus specific modeling and analysis techniques.
3.4 Research axes
Our activity is organized in five subtopics (or axes). The first two axes are on network management, and the next two are orthogonal on the associated and required measurements. Axis 5 on AI is transverse. The axes and their intersections are described in Figure 2.
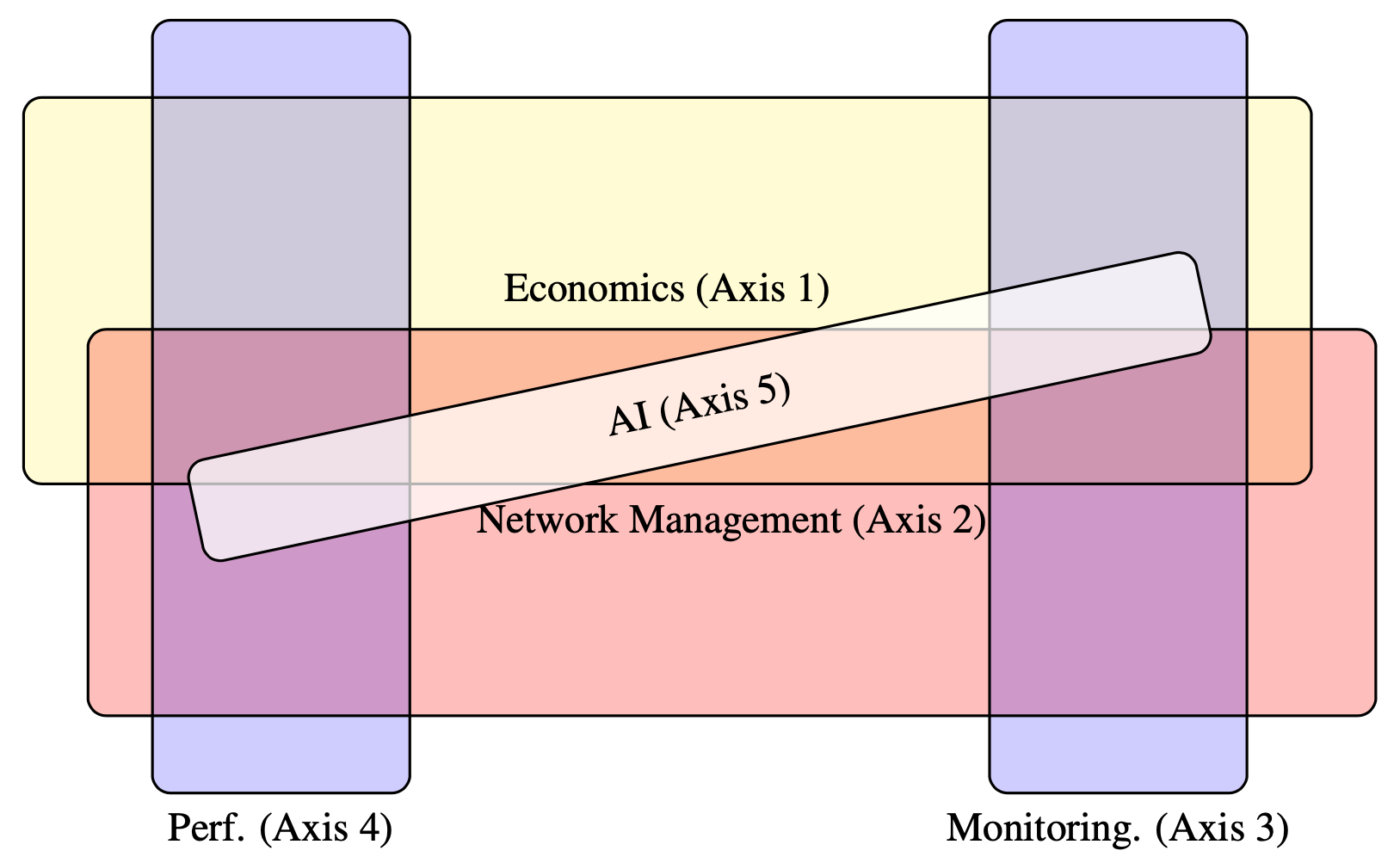
The five research axes: two on network management, two orthogonal on measurements and transverse on AI, with intersections
As a brief description:
- Network Economics. The digital economy has gained and keeps gaining in scale, scope, and significance. The ecosystem is quickly evolving and one of our main goals is to answer all questions related to the Internet & digital economy that pop up in line with what we started to do, and to be reactive to the news in that domain. We want to address issues concerning Internet resource allocation and pricing models, the economics of services, issues with vertical integration, the economics of structuring platforms, as well as economics and regulation (including network neutrality).
- Managing next generation networks & massive IoT communication. One of the fundamental challenges in the traffic management of new and emerging networking activities is to describe, analyze and control heterogeneous resources and complex services, under energy constraints. While AI is a main research direction to address this problem, there exist many other possibilities. The main questions of interest include the automation of infrastructure management (a typical example being network slicing) or the massive access in IoT networks.
- Measuring, monitoring & regulation. With the rapid evolution of networks, many needs are appearing for the design of measurement techniques associated with new services, but also for regulators to monitor network's activity. Measurements are of two possible categories: passive, monitoring existing traffic, or active, i.e., based on injecting traffic to investigate the network behavior. The goal of this research direction is to develop practical network operations measurement techniques, from the two different points of view: operators (for a better management) and regulators (for monitoring).
- Measuring performance metrics based on models. Measuring performances has to be done not only to observe and monitor directly a service like in previous subsection, but also at different phases such as at conception or to propose enhancements. Indeed, network services management and decision making cannot be applied efficiently without a valid and accurate evaluation of performance metrics through the construction and analysis of models. We are carrying out such evaluations.
- Artificial Intelligence (AI) in networking. AI is a transverse research axis, since it is used for both management and measuring. We are not only users of the technology but also interested in the methodology itself, that is, we also develop new techniques, based on our networking problems but usable outside. This concerns mainly supervised learning, in particular time series forecasting using this type of learning, and reinforcement learning. From the point of view of the tools, our skills and work are essentially related to Random Neural Networks, Reservoir Computing and deep neural architectures in Reinforcement Learning.
4 Application domains
Our global research effort concerns networking problems.
The need to support services requiring increasingly high throughput and extremely short latencies in 5G and Beyond 5G (B5G) networks is causing a major transformation of operators' infrastructure. The latter are increasingly being virtualized and expanded to be as close as possible to service consumers, in particular through the development of edge computing. Operators should increasingly collaborate to respond optimally to demands (i.e., cross-domain networks), and eventually converge their networks (i.e., networks' federation). The ultimate solution to this growing complexity is to move towards a fully automated network (i.e., zero-touch network). Our efforts will be dedicated, not on architectural improvements, but on the development of algorithms to manage these infrastructures in an automated way. The efficient and automatic placement of dynamic network services (i.e., network slicing), accommodating simultaneously a wide range of services, is certainly one of the most important concept to move towards zero-touch networks. Similarly, the limited radio resources, the random access to the medium and the spectrum sharing between different applications are critical issues in the IoT context. The focus is not only on 5G and beyond-5G networks, but also on unlicensed networks spectrum such as LoRaWan (for Long Range Wide Area Network; to enable IoT devices to communicate over long distances with minimal battery usage). In LoRaWan networks, the management challenges are even more important given their inherent characteristics, where access is not regulated at all, as it is the case in cellular networks like NB-IoT (for Narrowband Internet of Things).
Still on the management side, with the telecommunications ecosystem quickly evolving, one of our main goals is to answer all questions related to the Internet & digital economy that pop up in line with what we started to do, and to be reactive to the news in that domain to help economic actors and society. Typical and illustrative current questions requiring reactivity are: Is USA repealing neutrality good for some actors in the world, what reactions would be beneficial? Is free roaming in Europe bad for network providers? Is the 2019 Orange-TF1 argument part of the neutrality debate? Should search engines be regulated? Etc. Our goal is mainly to help regulators define proper rules, but we also aim at helping economic actors in decision making (economic choices of CDNs, search engines, etc.) and study their interactions.
On the measurement side, when developing practical network operations measurement techniques, we are interested in two different points of view: operators (for a better management) and regulators (for monitoring). Many of the techniques developed at Ermine are related to the analysis of complex systems in general, not only in telecommunications. For instance, our Monte Carlo methods for analyzing rare events have been used by different industrial partners, some of them in networking but recently also by companies building transportation systems.
5 Highlights of the year
- We published a book on neutrality issues in ICT 35.
- Mael Le Treust got his Habilitation à diriger les recherches (HDR) on the 29th of November 2022.
- Yassine Hadjadj-Aoul became a full Professor at the University of Rennes.
5.1 Awards
- Paper 20 was finalist for best contributed theoretical paper at the Winter Simulation Conference (the main event devoted to simulation).
- Paper 28 won the best paper award at the 14th IFIP Wireless Communications and Networking Conference (WMNC'2022).
- Paper 23 won the best short paper award at the IEEE CloudNet international conference (CloudNet'2022).
6 New software and platforms
6.1 New software
6.1.1 SNIDE
-
Name:
Search Non neutratlIty DEtection
-
Keywords:
Search Engine, Statistic analysis
-
Scientific Description:
The goal of this tool is, for a search, to collect the ordered lists from the main search engines, to compare them, to perform statistical tests to point out potential outliers, and to propose two meta search engines reducing biases.
-
Functional Description:
Different search engines provide different outputs for the same keyword. This may be due to different definitions of relevance, to different ranking aggregation methods, and/or to different knowledge/anticipation of users’ preferences, but rankings are also suspected to be biased towards own content, which may be prejudicial to other content providers.
-
Contact:
Bruno Tuffin
-
Participants:
Patrick Maillé, Bruno Tuffin, Guillermo Andrade Barroso
-
Partner:
IMT Atlantique
7 New results
7.1 Network Economics
Participants: Patrick Maillé, Bruno Tuffin, Maël Le Treust.
The major part of our activity on network economics was focusing in 2022 on network neutrality and its consequences.
As a main event, we have published a book on the topic 35. This book discusses the pros and cons of ICT neutrality. It tries to be as objective as possible from arguments of proponents and opponents, this way enabling readers to build their own opinion. It presents the history of the ongoing network neutrality debate, the various concepts it encompasses, and also some mathematical developments illustrating optimal strategies and potential counter-intuitive results. It discusses the related issues of fairness, freedom, and economic efficiency, among others, and illustrating the complexity of confronting those high-level considerations with the constantly evolving technical realities. It then extends the discussion to connected ICT domains. The book thus touches issues related to history, economics, law, networking, and mathematics.
A specific model is designed in 44 to simultaneously encompass several scenarios regarding the content-providing market structure: a monopolistic content provider, competitive ones, and a vertically-integrated one facing a non vertically-integrated competitor. We each time compare the outputs when non-neutral, weak neutral or strong neutral policies are applied, in order to get insights regarding the possible consequences of each type of neutrality regulation.
As a major issue, slicing is seen as one of the key characteristics of 5G and beyond networks but seems in apparent contradiction with neutrality principles promoted worldwide. We discuss in 43 the two contradictory but considered compulsory notions and propose how they can be accommodated. The general issue of accommodating neutrality principles and next generation networks is discussed in 50.
Also related to the net neutrality debate is the search neutrality debate, referring to possible biases in search engine's ranking results. The economic incentives for implementing such biases (e.g., to favor content from related entities) are indeed considerable. In 6, we develop a model to analyze those incentives and a tool to detect suspect behavior. To investigate notable differences among search engines (SEs), we formalize a definition of score, as the average displayed interest/visibility of the positions obtained by that page at SEs weighted by the click-through-rate of the position. As a consequence, we are also able to compute a score for each SE and compare SEs for a given keyword and for a population of keywords. We then develop a statistical methodology to identify potential bias based on Dixon’s Q test and propose two meta-engines: The first one, called consensus SE, ranks links according to the weighted Borda scores (average visibility among SEs); the second one is following the majority judgment rule expected to limit the imlpact of outliers of considering the median of scores instead of the mean.
Finally, we discuss in 48 the recent declaration of the European Commissioner Thierry Breton asking for the main platforms to participate to the network infrastructure, which can be seen as a surprising backdoor neutrality repeal. With respect to all our previous work, we add here the point of view of experts in law.
On a different network-economics subject, we have continued working on pricing mechanisms for resource allocation, in particular auction mechanisms for spectrum allocations in 5G networks, under the Licensed Shared Access (LSA) framework. In 2 we design an incentive-compatible scheme that improves the use of existing spectrum in dynamic settings. The mechanisms proposed in the literature for the LSA context were one-shot auction mechanisms. In our work, we first show how to increase the performance of those auctions (in terms of revenue, efficiency and fairness of the allocation) while preserving truthful bidding, by splitting spectrum and converting single block auctions into multi-block auctions. Simulation results illustrate how appropriately choosing the number of blocks allows to increase the aforementioned metrics. Second, we show how to convert one-shot mechanisms to equivalent ascending mechanisms (in terms of allocations and payments) so that we add transparency and privacy to the auction.
Information is crucial in decision making problems. The information asymmetries in Game Theory raise new scenarios and new open questions, also in Information Theory. In 45, we investigate the vector-valued extension of the Witsenhausen counterexample setup by using a coordination coding scheme. In 27 and in 41, we develop several graph theoretical tools in order to investigate the zero-error source coding problem with side information at the decoder. In the habilitation thesis 39, we present Information-Theoretic tools that bring new insights into Game Theoretical problems, such as the coordination of players’ actions and the processing of strategic information. Although Information Theory and Game Theory have distinct fields of application, these two theories rely on similar mathematical tools. Such problems arise for the design of autonomous devices and for the study of the behavior of rational agents. New generations of communication networks involve devices that take decisions with greater autonomy. These machines observe their environment, interact, cooperate with other machines or with humans, adapt dynamically to their environment and to the topology of the network.
7.2 Managing next generation networks & massive IoT communication
Participants: Soraya Ait Chellouche, Yann Busnel, Yassine Hadjadj-Aoul, Patrick Maillé, Fatima Zahra Mardi.
Fleet of drones coordination.
Decentralization and hybridization of distributed systems has given birth to edge and fog computing. At the same time, artificial intelligence and self-adaptive mechanisms have allowed great progress in the autonomy of networks. In 51, we look at the need for self-organization, where local decisions give rise to globally consistent system behaviors. We present two algorithms for (1) target discovery following a disaster, using autonomous drones and for (2) extending the lifespan of an IoT network.
The problem of the lifetime of connected objects, in most use cases (Industrial Internet of Things (IIoT), disaster management, etc.) is also an essential element of the proposed solutions. Radio frequency (RF) harvesting of sensor batteries, even if attractive, does not scale up if it has to be done by human operators, and becomes impossible if the objects are located in unreachable places. An innovative solution consists of using fleets of drones to take care of this regular recharge. We focus in 15 on the self-organised deployment of a fleet of drones to solve this problem, taking into account the multiple constraints involved. We propose a two-step optimization framework based on an optimal orchestration solution to reduce the recharging time of a complete sensor system, by optimizing the number of drones, the overall flight time and their energy consumption.
Optimization of Massive-IoT emission strategies
In today's monitoring solutions, each application involves custom deployment and requires significant configuration efforts to accommodate sensor changes. In contrast, in 29, 46, a massive deployment of battery-powered sensors is considered and we propose a more versatile monitoring solution that is not tied to physical device deployment. We characterize a monitoring strategy by formally defining a function that modifies the transmission period of a sensor that has just transmitted. Such a function can be customized to manage the tradeoff between overall monitoring accuracy and sensor energy consumption through two parameters, namely the target emission period and the number of jointly used sensors.
In the specific context when IoT traffic is mixed with video traffic further optimizations are particularly required. Indeed, the traditional way of storing and processing data in the Cloud cannot satisfy many latency critical applications. This is why Fog computing emerged as a continuum infrastructure from the Cloud to end-user devices. Misplacing data in such an infrastructure results in high latency, and consequently increases the penalty for Internet Service Providers (ISPs) incurred by violating the service level agreement (SLA). In past studies, two issues have been investigated separately: the IoT data placement and the streaming cache placement. In 25, we address those issues in a unique model with the aim to minimize the penalty for ISPs incurred by the SLA violation and maximize storage resource usage. We subdivided each Fog node storage space into a storage part and a cache part. First, our model consists in placing IoT data in the storage part of Fog nodes, and then placing streaming data in the cache part of these nodes. The novelty of our model is the flexibility it offers for managing the cache volume, which can, adaptively, spill on the free part dedicated to IoT data. Experiments show that using our model makes it possible to reduce the streaming data penalty of the ISP's SLA violation by more than 47% on average.
Network slicing for IoT networks
The new emerging technologies enable the appearance of the 5G system and beyond that offers a plethora of services and target new verticals. One of these verticals is the large-scale IoT that is expected to be used everywhere in our daily lives. The traffic would likely be increased due to these emerging verticals. To overcome such challenges, network slicing and softwarization will play a crucial role in addressing these requirements and ensuring SLAs. Thus, there is a need to provide efficient and flexible network slice management mechanisms to handle the hurdles that come with the emerging industrial verticals. In 19, we suggest a centralized coalition game-based network slicing strategy to manage LoRa nodes efficiently. According to the K-means clustering algorithm, the proposed solution is deployed within clustered players to maximize reliability while ensuring the SLA of the LoRa slices. Simulation results clearly show that our proposed approach improves Packets' Success Rate (PSR), Network Energy Consumption (NEC), and guarantees prioritization between slices.
On the other hand, the significant deployment of LoRaWAN networks is increasingly questioning its ability to support service differentiation, in addition to the massive amount of devices 42. The few existing attempts to implement service differentiation suffer from a lack of scalability and do not meet the qualitative criteria of the services, since without admission control there is no way to restrain the devices from transmitting. In 13, we propose a scalable probabilistic approach that not only enables an efficient sharing of LoRaWan access networks between different services/slices, but more importantly allows achieving the objectives of the supported services through the integration of an admission control. Since the derivation of devices' repartition probabilities is a very complex problem, we propose an evolutionary algorithm to derive them efficiently. The obtained results clearly show the ability of the proposed solution to efficiently utilize the scarce radio resources, while achieving the qualitative objectives of the prioritized services.
Service placement in the cloud-edge continuum
The increasing trend of IoT and its wide adoption by industries led to the emergence of the edge computing paradigm. The idea is to leverage the computing resources at the edge of the network, close to end users and data production and consumption, to allow low latency and better bandwidth utilization. Indeed, currently, applications offload tasks to remote cloud data centers to get processing and storage support. However, the centralize approach of cloud computing is not suitable for lower latency applications and can induce heavy load on the network infrastructure. Offloading tasks to the edge of the network represents thus a promising distributed computing model that can efficiently support the delay-constrained and resource-hungry applications and services. However, extending cloud computing to the Edge of the network raises new resource allocation and service placement challenges. Indeed, at the Edge, resources are usually limited and heterogeneous especially when dealing with mobile resources. In such a context, reliability becomes a major issue to consider when placing network services. Motivated by this, we work on genetic algorithm-based service placements that consider both the heterogeneity and scarceness of resources at the edge and the link reliability.
Besides, when users move, their services and communications may also suffer long down times that can violate the latency requirements’ of the consumed services, when not completely disconnected. Considering mobility, which represents a real trend in service consumption nowadays, is then critical when placing services. We conduct studies on the MIT reality mining dataset which give us confidence in the high predictability of the user mobility. Based on the mobility historical information of users, their POI and the staying times at these POI, we propose a service placement that aims to reduce service migration and thus experienced down time by users while keeping as objectives to satisfy the response time required by in the SLA and efficiently operating load balancing between the hosting edge servers.
7.3 Measuring, monitoring & regulation
Participants: Yassine Hadjadj-Aoul, Antoine Lesieur, Patrick Maillé, Bruno Tuffin, Zahraa El Attar.
Our activity on monitoring has focused in 2022 on specific contexts but notice that we still work on network neutrality monitoring, starting to develop a demonstrator of the efficiency of existing tools which will help to suggest improvements.
Search neutrality monitoring. The search neutrality debate stresses the need for methodologies and tools to verify bias in search engine rankings and analyze their potential impact. We have developed in 6 a simple yet effective framework comparing the results of existing search engines. We present statistical tests based on outlier detection pointing out potential biases, and introduce two meta engines aiming at reducing bias. All this is implemented in a publicly-available tool SNIDE. Thanks to extensive studies on campaigns of most dialed keywords, we compare the results of all SEs (not only our meta-engines, but also the “regular” ones). We are able to compute and compare expected scores over searches, but also their distributions, in order to see similarities, highlight if they use close algorithms, and compute the frequencies of potential bias to emphasize how often they can be trusted. The tool is described for a large public in 49.
Data-driven network monitoring. Similarly, monitoring network performance becomes crucial today since it allows content providers to ensure a good quality of their services by identifying the root causes of service degradation. Also, it gives the end-user a better understanding of the performance they have (state of the networks). A widely used monitoring technique involves performing measurements from within the browser in an effort to capture the network status as close as possible; we talk about Web-based network monitoring. Many Web measurement tools have recently been proposed, however, most of these tools either have a high computational cost or exaggeratedly consume data. In 24, we propose a lightweight solution able to estimate the underlying network status accurately and perform Web troubleshooting in order to detect anomalies. We develop and implement a distributed system that collects measurements at both levels: browser and network. Then, we build an original network monitoring framework based on Bayesian Gaussian Mixture Models (BGMM) coupled with an algorithm to detect in real time the occurrence of anomalies. We follow a browser-based passive measurement and data-driven approach to derive our inference models, which leads to an efficient Web browsing troubleshooting solution.
7.4 Measuring performance metrics based on models
Participants: Bruno Tuffin, Gerardo Rubino, Sofiene Jelassi, Bruno Sericola.
Our work on performance analysis in 2022 can be decomposed in simulation, with application to resilience, transient analysis, with application to protocols, and distributed systems; with application to blockchain.
Monte Carlo simulation.
We edited a Festchrift in the honor of Pierre L'Ecuyer 34 (see also 36), a former member of Dionysos project-team (which was preceding Ermine) and a top scientist in the fields of stochastic modeling, simulation, and operations research.
On simulation, studying rare events is a critical issue on which we pursue an activity. For a regenerative process for example, we propose in 20 various estimators of the density function of the cumulative reward up to hitting a rarely visited set of states. The approaches exploit existing weak-convergence results for the hitting time distribution, and we apply simulation (often with previously developed importance samplers for estimating the mean) to estimate parameters of the limiting distribution. We also combine these ideas with kernel methods. For the estimation of the cumulative distribution function, 37 examines how randomized quasi-Monte Carlo (RQMC) techniques can help to reduce the variance of the estimators. Estimating hitting-time properties entails simulating a stochastic (here Markov) process, for which the so-called array-RQMC method is suited. In 26 we describe new techniques we proposed for estimating the probability that the sum of nonnegative independent and identically distributed random variables falls below a given threshold, in the rare event case, when this probability is extremely small. We discuss how to improve the classical exponential twisting technique, by means of appropriate Important Sampling schemes. Specifically, we describe how using a well-chosen Gamma distribution provides such an improvement.
We continued to work on flow reliability problems, where the goal is to evaluate the probability that a given transportation network is able to transport at least a specific amount of flow, under possible failures of its components. We describe in 1 a methodology that can efficiently estimate this reliability metric when components' failures are not necessarily independent. In that paper we describe a Splitting–based Monte Carlo method that uses the Marshall–Olkin copula for the case of non independent components. This proposal operates on the so-called Destruction Process because the Marshall–Olkin copula model over networks is quite related —and somehow similar— to that scheme, which is unusual but here necessary. In 32 we present these developments and discuss several possible extensions to other contexts.
As a specific application, a 5G Radio Access Network (5G-RAN) can be disturbed or shutdown due to a variety of failures, in spite of advanced optimization techniques and self-healing methods. Recently, operators started to take an interest in improving the resilience of communication networks with adaptive compensation techniques. However, for the sake of effective and efficient resilience management, it is vital to be able to measure current and prospective resiliency levels of a given 5G-RAN using relevant and explicit metrics. In 16 we perform an analysis and a quantification of 5G-RAN resilience using a coverage indicator. We model the network coverage using Continuous Time Markov Chains where coverage status is characterized with multiple states defined with Reference Signal Received Power (RSRP) signal. The proposed Markov model is analytically studied allowing to perform quantitative analysis, predict coverage outage and provide resilience quantification. Using our model, we conduct numerical analysis and Monte Carlo explorations of several usage scenarios and propose a resilience framework to show the usability of our proposed approach.
In 38 we consider static models in network reliability. The analysis of these models is in general #P-complete, and Monte Carlo remains the only effective approach. We underline the interest in moving from the typical binary world where components and systems are either up or down, to a multi-variate one, where the up state is decomposed into several performance levels. This is also called a performability view of the system. We then propose a different view of Monte Carlo procedures, where instead of trying to reduce the variance of the estimators, we focus on their time complexities. We discuss the ability of the mentioned approach for quickly estimating resilience, together with variations of it. We also discuss another side effect of the sampling technique proposed in the text, the possibility of easily computing the sensitivities of these metrics with respect to the individual reliabilities of the components.
We also worked in 9 on so-called generalized likelihood ratio (GLR) method, a gradient estimation method for handling discontinuities for a wide scope of sample performances. We put the GLR methods from previous works into a single framework, simplified regularity conditions for justifying unbiasedness of GLR, and relaxed some of those conditions that are difficult to verify in practice. Moreover, we combined GLR with conditional Monte Carlo methods and RQMC methods to reduce the variance.
A discussion on sufficient conditions for the validity of a confidence interval for RQMC methods is presented in 30.
Transient analysis.
In recent works, we proposed a matrix transformation that we call the exponential-dual, which we used to find closed-forms of some matrix exponentials. The initial motivation of this work was the transient analysis of basic Markovian models, mainly in queueing. Using this transformation in the particular case of transition rate matrices, corresponding to these queuing models, allowed to efficiently apply combinatorial techniques to expressions of the transient distributions of the number of customers in the system at an arbitrary point in time. This could also be done for some infinite models (infinite matrices). In 31 we present the discrete counterpart of this idea, the power-dual of a matrix, which completes the picture and which is strongly related to the exponential-dual concept. The power-dual appears when we work with the power of a given matrix. These ideas are basically extensions (to arbitrary linear systems of Ordinary Differential Equations) of Siegmund’s duality, defined on continuous time Markov processes.
Network protocols sometimes make use of random walks (i.e., token circulation) on networks, to explore networks to collect information from them and to make systems reliable. Important performance measures of these protocols such as the search time and the time to visit all sites obviously depend on some quality measures of random walks such as the hitting and the cover times. The cover time of a discrete-time homogenous Markov chain or of a random walk the time needed to visit all the states of the process. We analyze in 47 both the distribution and the moments of the cover time and we are interested in exact results instead of asymptotic values of the mean cover time which are generally considered in the literature. We first obtain several general results on the hitting time and the cover time of a subset of the state space both in terms of distribution and moments. These results are then applied to particular graphs namely the generalized cycle graph, the complete graph and the generalized path graph.
Measuring the performance of distributed systems.
Randomized rumor spreading or gossiping is an important communication mechanism that allows the dissemination of information in large-scale and open networks. A large-scale and open network comprises a collection of sequential computing entities (e.g., processes, processors, nodes, agents, peers) that join and leave the system at any time, and communicate with one another by exchanging messages. In contrast to reliable communication broadcasts which must provide agreement on the broadcast value with possibly additional ordering guarantees on the delivery of updates from different sources, a randomized rumor spreading procedure provides reliability only with some probability. A randomized spreading rumor protocol describes the rules required for one or more pieces of information known by an arbitrary node in the network to be spread to all the nodes of the network. We propose and analyze in 10 a new asynchronous rumor spreading protocol to deliver a rumor to all the nodes of a large-scale distributed network. This spreading protocol relies on what we call a -pull operation, with . Specifically a -pull operation consists, for an uninformed node , in contacting other nodes at random in the network, and if at least one of them knows the rumor, then node learns it. We perform a thorough study (expected value, variance, limiting distribution as ) of the total number of -pull operations needed for all the nodes to learn the rumor. Finally, we show that when , our new protocol requires less operations than the traditional 2-push-pull and 2-push protocols by using stochastic dominance arguments.
This analysis has been extended in 40 to deal with the continuous-time case. Specifically, a local clock following an exponential distribution with rate is associated with each uninformed node of the system. Each time the clock of an uniformed node rings, this node contacts , with , distinct nodes, chosen at random uniformly among the other nodes. If at least one of these contacted nodes knows the rumor, the initiator of the -pull operation learns the rumor and clears its clock. We perform a detailed study in continuous-time of the total time needed for all the n nodes to learn the rumor. The analysis of the -pull rumor spreading in continuous-time is much more complicated than the one in discrete-time and needs more refined results.
7.5 Artificial Intelligence (AI) in networking
Participants: Yann Busnel, Yassine Hadjadj-Aoul, Gerardo Rubino, César Viho.
Tools of Machine Learning for Networking.
The Echo State Network (ESN) is a class of Recurrent Neural Network with a large number of hidden-hidden weights (in the so-called reservoir). Canonical ESNs and their variations have recently received significant attention due to their remarkable success in the modeling of non-linear dynamical systems. The reservoir is randomly connected with fixed weights that don't change in the learning process, only the weights from reservoir to output are trained. Since the reservoir is fixed during the training procedure, we may wonder if the computational power of the recurrent structure is fully harnessed. In 11 we propose a new computational model of the ESN type, that represents the reservoir weights in the Fourier space and performs a fine-tuning of these weights applying genetic algorithms in the frequency domain. The main interest is that this procedure will work in a much smaller space compared to the classical ESN, thus providing a dimensionality reduction transformation of the initial method. The proposed technique allows us to exploit the benefits of the large recurrent structure avoiding the training problems of gradient-based method.
Learning from non-stationary data presents several new challenges. Among them, a significant problem comes from the sudden changes in the incoming data distributions, the so-called concept drift. Several concept drift detection methods exist, generally based on distances between distributions, either arbitrarily selected or context-dependent. Our paper 21 presents a straightforward approach for detecting concept drift based on a weighted dissimilarity metric over posterior probabilities. We also evaluate the performance of three well-known dissimilarity metrics when used by the proposed approach. Experimental evaluation has been done over ten datasets with injected sudden drifts in a binary classification context. Our results first suggest choosing the Kullback-Leibler divergence, and second, they show that our drift detection procedure based on dissimilarity measures is pretty efficient.
Nowadays, Machine Learning (ML) tools are commonly used in every area of science or technology. Networking is not an exception, and we find ML all over the research activities in most fields composing the domain. In 22, we briefly describe a set of research activities we have developed along several years around several pretty different families of problems, using ML methods. They concern (i) the automatic and accurate real time measure of the Quality of Experience of an application or service built on top of the Internet around the transport of video or audio content (e.g. video streaming, IP telephony, video-conferencing, etc.), (ii) network tomography (measuring on the edges to infer values inside the network), (iii) time series forecasting in several contexts, in particular concept drift detection or anomalies detection, and (iv) service placements in Software Defined Networks, a central problem in 5G and B5G technologies. The corresponding ML tools are mainly Supervised Learning and Reinforcement Learning, even if we are currently using Unsupervised Learning in recent activities of point (i).
Random Neural Networks are a class of Neural Networks coming from Stochastic Processes and, in particular, from Queuing Models. They have some nice properties and they have reached good performances in several application areas. They are, in fact, queuing systems seen as Neural machines, and the two uses (probabilistic models for the performance evaluation of systems, or learning machines similar as the other more standard families of Neural Networks) refer to the same mathematical objects. They have the appealing that, as other special models that are unknown for most experts in ML, their testing in and/or adaptation to the many areas where standard ML techniques have obtained great successes is totally open. In 52 we underline the reasons that make them at least extremely interesting. We then describe some of their successful applications. We focus on learning, but we also mention other uses of these models in performance evaluation, in the analysis of biological systems, and in optimization.
AI-based Context-awareness in Future Mobile Networks.
During the last decades, different studies highlighted the benefits of acquiring channel state cognition based on the environmental context of mobile users or devices. Thanks to this cognition, cellular networks can optimize themselves and personalize the delivered services and in turn, offer a better quality of experience to users. In collaboration with Nokia Bell lab, we are investigating solutions to improve the decision process in SONs (Self Organized Networks), in a 5G environment and beyond, by introducing external knowledge through personalization. We proposed a system for profiling mobile network user behavior using artificial intelligence methods. This knowledge is then used to configure and optimize the mobile network according to the needs of each user while better managing resources. This year was dedicated to further improve the performance of user environment detection methods. We first studied the detection of the real-life mobile user environment using context aware detection via multi-task learning (MTL). We proposed models that are able to automatically detect up to eight distinct real-life user environments. We also improved the detection accuracy with the assistance of the mobility state profiling task Using MTL, the task of detecting environment corresponds to simultaneously answering the questions: "how and where mobile user consumes mobile services?". We build models using real-life radio data which is already available in network. Results support our claim to detect several environment classes in network infrastructure with improved UED accuracy 7.
We have also investigated the detection of mobile users' environment, in real-life situations, using ML classification methods on time series data. To attain the highest accuracy, while using limited length of time series, we propose using a heuristic method to account for the typical user behavior when he or she changes environment. For this, a new module called User Behavioral Optimizer, is investigated and combined with time series models. Experiments done using real radio data show that ML on time series data using our behavioral optimizer and heuristic allows improving the indoor/outdoor detection with high accuracy (with F1 - score up to around 94.8%) 28.
Federated Learning for Collaborative Security.
In 2016, Google introduced the concept of Federated Learning (FL), enabling collaborative ML. FL does not share local data but ML models, offering applications in diverse domains. Our study 5 focuses on the application of FL to Intrusion Detection Systems (IDSs). There, common criteria to compare existing solutions are missing. In particular, we have shown: (i) how FL-based IDSs are used in different domains; (ii) what differences exist between architectures; (iii) the state of the art of FL-based IDS. With a structured literature survey, this work identifies the relevant state of the art in FL-based intrusion detection from its creation in 2016 until 2021. It provides a reference architecture and a taxonomy to serve as guidelines to compare and design FLbased IDSs.
On the other hand, literature shows that trust typically relies on knowledge about the communication partner. Federated learning allows collaborators to share ML models without revealing secrets, as only the abstract models and not the data used for their creation is shared. A fundamental challenge, however, is determining how much trust is justified for each contributor to collaboratively optimize the joint models. Trust also depends on how much an aggregated model contributes to the objectives of a party. For example, a model trained for an OT system is typically useless for monitoring IT systems. Our work 18 shows directions how heterogeneous distributed data sources could be integrated using federated learning methods.
Internet of Things and Deep Learning for e-Health.
Cough is a symptom in over a hundred respiratory diseases. The audio features in cough signals contain erudition about the predicament of the respiratory system. Using deep learning or signal processing, these features can be used to build an effective disease prediction system. Our work 4 categorizes and reviews the current progress on cough audio analysis for the classification of pulmonary diseases. It also explores potential future issues in research. Additionally, it proposes a model for the classification of ten serious pulmonary ailments commonly seen in Indian adolescents.
ML-based Network Slicing.
Network slicing is seen as one of the key building blocks in the evolution towards fully autonomous networks, a concept referred to as “zero touch networks”. It mainly consists in a dynamic deployment (i.e., placement) of services, regardless of their constraints, in a substrate network, whether physical or virtual. However, the Virtual Network Embedding (VNE) algorithms used for network slices placement generally follow a static operating mechanism, which obstructs the controller from optimally using the available physical resources resulting in inferior embedding strategies and less robust decisions.
We propose in 33, 23 a highly reliable solution for systematically placing network services, touching the optimal results while maintaining the scalability, making it suitable for online scenarios with strict time constraints. We organized our solution as a Branch and Bound search structure, which leverages Artificial Intelligence (AI) search strategies (Especially A-Star) to address the placement problem.
In the recent literature, some reinforcement learning algorithms have been conceived to dynamically update the decision-making strategies, although the time required to learn the optimal strategy is usually costly. In 14, we propose the use of Monte Carlo techniques to improve the decision of Deep Reinforcement Learning (DRL) strategies in dynamic virtual network embedding problems. The proposed solution, named DQMC, is based on a combination of deep Q-learning and a Monte Carlo (MC) approach. The idea here is to learn to generate, using a Deep Q-Network (DQN), a distribution of the placement solution, on which a MC-based search technique is applied. This makes the agent's exploration of the solution space more efficient, and achieves a faster convergence of the placement decision, and thus a safer learning. As the learning is costly in time and computing resources, and sensitive to changes in the network, we suggest, in 3 a control-theory-based techniques to dynamically leverage exploration in DQMC. This leads to fast, efficient, and sober learning compared to a Monte Carlo-based strategy. This also ensures a reliable solution even in the case of a change in the requests’ sizes or a node’s failure, showing promising perspectives for solutions combining control-theory and machine learning.
As DRL algorithms present different performances according to the employed approach, and the problem to be treated, we propose, in 12 the adoption of the best algorithm, from a selection of learning strategies, in terms of reward and sample efficiency at each time step. The proposed strategy acts as a meta-algorithm that brings more robustness to the network by dynamically selecting the best solution for a specific scenario. Our solution proved its efficiency and managed to dynamically select the best algorithm in terms of the best acceptance ratio of the deployed services and outperform all the stand-alone algorithms.
ML-based Caching systems optimisation.
HPC systems are composed of multiple tiers of storage, from the top high performance tier (high speed SSDs) to the bottom capacitive one (tapes). File placement in such architecture is managed through prefetchers (bottom-up) and eviction policies (top-down). Most state-of-the-art work focus on the former while using algorithm flavors of LRU, LFU and FIFO for the latter. LRU was for long considered the best choice. However, recent studies has shown that the simplicity of FIFO could make it more scalable than LRU because of metadata management, and thus more adequate in several cases. In 8, we propose a new eviction policy based on predicted files lifetimes. It is comparable to FIFO in terms of metadata overhead and simplicity (thus scalability), while giving a hit ratio comparable to LRU (or even 10% better for some tested traces). We also propose a naive multi-tier heterogeneous storage simulator implementation to evaluate such policies.
8 Bilateral contracts and grants with industry
8.1 Bilateral contracts with industry
8.1.1 Common Lab INRIA - Nokia
Participants: Yassine Hadjadh-Aoul, Gerardo Rubino.
Gerardo Rubino was the coordinator of the research action “Analytics and machine learning”, between Nokia Bell Labs and INRIA. The objective was to carry out common research on an integrated framework for 5G, programmable networks, IoT and clouds that aims at statically and dynamically managing and optimizing the 5G infrastructure using, in particular, Machine Learning techniques. The project involves several teams in INRIA and the work of several PhD students. It ended its mlife-time in 2022. At Ermine, Yassine Hadjadh-Aoul is an active member of the action.8.1.2 ANR Intelligentsia
Participants: Soraya Ait Chellouche, Ghina Dandachi, Yassine Hadjadj-Aoul, Patrick Maillé, Gerardo Rubino.
-
Title:
INTelligent Edge using Learning Loops & Information GEneration for NeTwork State Inferencebased Automation.
-
Partner Institution(s):
- Orange Labs
- Inria Rennes
- CNAM
- Acklio
- Aguila
-
Date/Duration:
Nov. 2020-Nov. 2024 (4 years)
-
Summary:
The Intelligentsia project aims at (1) specifying novel media access protocols and resources sharing policies to be able to support network slicing for IoT access networks in general, and LoRa networks in particular; (2) designing of a network automation framework that incorporates novel learning algorithms to configure the IoT access network.
8.1.3 BPI Beyond 5G project
Participants: Yann Busnel.
-
Title:
Sovereign and resilient solutions for 5G and Beyond
-
Partner Institution(s):
- Thales, France
- Ericson, France
- Institut Mines Telecom
-
Date/Duration:
2021-2024 (3 years)
-
Additionnal info/keywords:
Sovereignty in Telecommunications Networks to Accelerate 5G Applications in Vertical Markets. The project aims to design technical solutions for the development of sovereign and secure next-generation 5G networks, while developing innovative uses for the industry of the future.
8.1.4 BPI 5G JO
Participants: Yassine Hadjadj-Aoul, Gerardo Rubino.
-
Title:
5G JO
-
Partner Institution(s):
- Orange
- Inria Rennes
- CEA Saclay
- Ericsson
-
Date/Duration:
Nov. 2020-Nov. 2023 (3 years)
-
Summary:
The objective of the project is to develop a platform for the placement of constrained services on the fly, with environmental consideration.
8.1.5 Common lab IMT Atlantique - b<>com with AI@IMT project
Participants: Yann Busnel, Patrick Maillé.
Yann Busnel is the coordinator of the research action “AI&Cyber”, between IRT b<>com and IMT Atlantique, cofunded by ANR within the project AI@IMT. The objective is to carry out common research on the usage of AI in Cybersecurity for Networking context. The project involves several researchers in IMT Atlantique (Patrick Maillé and Romaric Ludinard at least) and involves the work of several PhD students.
8.1.6 Cyber CNI chair at IMT Atlantique
Participants: Yann Busnel.
Yann Busnel is the financial chair of the industrial chair Cybersecurity for Critical Networked Infrastructures (Cyber CNI: cybercni). This is an industrial research chair, founded in 2016, with the following current industry partners in phase 2 (2019-2021) : Airbus, Amossys, BNP Paribas, EDF, Nokia Bell Labs, and SNCF.
8.2 Bilateral Grants with Industry
8.2.1 Cifre on Resiliency as a Service for 5G, with Nokia
Participants: Sofiene Jelassi, Gerardo Rubino.
This is a Cifre contract 2020–2023 including a PhD thesis supervision (PhD of Soumaya Kaada), done with Nokia (Paris-Saclay), about Resiliency as a Service for 5G networks using Machine Learning. It concerns providing on demand and evolving resiliency schemes over 5G network using advanced Machine Learning algorithms. It relies on a highly flexible network infrastructure supporting both wired and wireless programmable data planes through a highly-efficient distributed network operating system.
8.2.2 Cifre on availability-aware NFVs placements, with Exfo
Participants: Yassine Hadjadh-Aoul, Gerardo Rubino.
This is a Cifre contract 2020–2023 including a PhD thesis supervision (PhD of Amine Rguez), done with Exfo (Rennes), about availability-aware NFVs placements of network services, using Deep Reinforcement Learning. The objective of the thesis is to exploit the potential of Deep Learning, and more particularly, of Deep Reinforcement Learning, in order to guarantee the high availability of placed network services.
8.2.3 CIFRE on Automation beyond 5G, with Nokia Bell Lab
Participants: César Viho.
This is a Cifre contract 2020–2023 including a PhD thesis supervision (PhD of Sid Ali Hamideche), done with Nokia Bell Labs (Nozay) and IRISA-Université Rennes 1. We are searching for solutions to improve the decision process in SONs (Self Organized Networks), in a 5G environment and beyond, by introducing external knowledge through personalization. The objective is to build a system for profiling mobile network user behavior using artificial intelligence methods. In other words, it is to provide mobile networks with the ability to understand the habits of these users. This knowledge is then used to configure and optimize the mobile network according to the needs of each user while better managing resources.8.2.4 CIFRE on Multi-task learning to discover the context of the Home, with Orange
Participants: César Viho, Yassine Hadjadh-Aoul.
This is a Cifre contract 2020–2023 including a PhD thesis supervision (PhD of Ahcen Boumhand), done with Orange(Rennes) and IRISA-Université Rennes 1. The objective is to design a multi-task learning model capable of recognising the different elements involved in users' digital activities (i.e. digital context) from network traces. We also aim to designing a solution capable of recognising (detecting) the appearance of a Media multitasking situation and describing it in a detailed manner (identifying the activities, their temporal framework, their semantic relations) from the network traces.8.2.5 CIFRE on learning mechanisms in IoT, with Orange
Participants: Patrick Maillé.
This is a Cifre contract 2019–2022 including a PhD thesis supervision (PhD of Hiba Dakdouk), done with Orange Labs (Lannion and Grenoble), about bandit algorithms to be implemented by IoT nodes to select the channels to use to send data. In particular, some collaborative learning versions of bandit algorithms have been designed and analyzed. The thesis has been successfully defended in May 2022.
8.2.6 Cifre on network slicing, with Nokia
Participants: Yassine Hadjadh-Aoul, Gerardo Rubino.
This is a Cifre contract 2021–2024 including a PhD thesis supervision (PhD of Abdelmounaim Bouroudi), done with Nokia Bell Labs (Nozay) and INRIA, on multi-agent reinforcement learning for resource allocation and scheduling in post-5G networks. The objective of the thesis is to propose a distributed resource placement and control system allowing automating network operations in their whole life-cycle from the creation of an end-to-end network slice to its deletion, while continuously optimally self-tuning the resource allocation to match the targeted performances.
8.2.7 Cifre on Data science in support of cybersecurity operations, with Airbus Cybersecurity
Participants: Yann Busnel.
This is a Cifre contract 2020–2023 including a PhD thesis supervision (PhD of Alexandre Day), done with Airbus Cybersecurity (Rennes), about Data science in support of cybersecurity operations. This thesis describes methods to allow security analysts, with no expertise in data science, to create and adapt security analytics that leverage machine learning models to automate more of their investigation procedures. This thesis focuses on anomaly detection to highlight unusual behaviours and clustering to regroup similar alerts and avoid repeating the same alerts again and again. We specifically explore the application of these methods to novel attack detection. To assess our approach, we also describe a method to automatically generate user activity to create realistic datasets.
9 Partnerships and cooperations
9.1 Informal International Partners
- G. Rubino works with Alan Krinik from Cal Poly, Pomona, US, on transient analysis of Markovian models.
- G. Rubino collaborates with Sebastián Basterrech from the VSB-Technical University of Ostrava, Czech Republic, on Machine Learning problems, these days on time series prediction using Reservoir Computing techniques.
- G. Rubino collaborates with Claudio Risso at the Engineering Faculty of the University of the Republic (UDELAR) in Uruguay, in the preparation of an Uruguayan national project concerning the optimization of the energy production and distribution in the country.
- B. Sericola works with Marie-Ange Remiche from the university of Namur, Belgium, and with Fabrice Guillemin from Orange Labs in Lannion, France, on the analysis of fluid queues.
- Y. Busnel has taken part in several events to develop Indo-French collaborations, notably within the framework of Campus France. In particular, he led the partnership with IIT Indore, ISI Kolkata and co-organized the Indo-French Knowledge Summit (chair of the Artificial Intelligence session) in Pune in November 2021. He has been nominated as member of the CEFIPRA Scientific Council for 2023–2025.
- Y. Busnel works with Professor Kapil Ahuja from IIT Indore, India, on Machine Learning and Sampling for Large Datasets.
- Y. Busnel works with Professor Sanghamitra Bandyopadhyay from ISI Kolkata, India, on Machine Learning and Sampling for e-Health and pharmacovigilence.
- C. Viho works on Machine Learning for Cognitive Autonomic Networking in 5G and beyond with Kamal Singh from Laboratoire Hubert Currien, Université de Saint-Etienne France and with Marie Line Alberi-Morel from Nokia Bell Lab (Nozay) France. They supervised the PhD Thesis of Illyyne Saffar defended in November 2020 and the ongoing PhD thesis of Sid Ali Hamideche. Kamal is also a co-supervisor of the ongoing PhD Thesis of Ahcene Boumhand on Multi-task learning to discover the context of the Home.
- C. Viho cooperated and organized several events on interoperability testing applied to IPv6 and Iot with Professor Xiaohong Huang from BUPT Beijin China, and ETSI (European Telecommunication Standardization Institute).
- M. Le Treust works with Matthieu Bloch, Georgia Tech Atlanta, on coordination and channel state masking.
- M. Le Treust works with L. Briceño Arias, Universidad Técnica Federico Santa María, Santiago de Chile, on convex optimization algorithms.
- M. Le Treust works with T. Oechtering from KTH, Stockholm, on coding for decentralized control problems.
- B. Tuffin works with M.K. Nakayama (NJIT, USA) and P.W. Glynn (Stanford University, USA) on rare event simulation.
- B. Tuffin works with P. L'Ecuyer (Université de Montréal, Canada), M.K. Nakayama (NJIT, USA) and A. Owen (Stanford University, USA) on randomized quasi-Monte Carlo methods.
- Y. Hadjadj works with M. Bagaa (Aalto University, Finland) on Slicing IoT networks, and in particular LoRaWan.
- Y. Hadjadj works with S. Djahel (University of Huddersfield, UK) on resources management in wireless networks.
9.2 International initiatives
9.2.1 STIC/MATH/CLIMAT AmSud projects
Climate AmSud program: project “ClimateDL”
Participants: Yassine Hadjadh-Aoul, Gerardo Rubino.
- Title: Using deep learning spatial-temporal graph models for seasonal forecasting of extreme temperature events (acronym: ClimateDL)
- International Partners (Institution - Laboratory - Researcher):
- Universidad de la República (Uruguay) / Faculty of Engineering: Pablo Rodríguez-Bocca (coord.), Diego Kiedanski, Guillermo Pereira / Faculty of Sciences: Madeleine Renom, Yilian Montesino
- Universidad de Buenos Aires, Argentina: Matilde Rusticucci (coord.), Mariana Barrucand, Soledad Collazo, Solange Suli
- Universidad Adolfo Ibañez (Chile) / Faculty of Engineering and Sciences: Rodrigo Carrasco (coord.), Javiera Barrera, Eduardo Moreno
- INRIA Rennes: Yassine Hadjadh-Aoul (coord.), Gerardo Rubino, Sebastián Basterrech (collab. ext.)
- Coordinator of the project: Pablo Rodríguez-Bocca
- Duration: 2 years
- Start year: 2022
- See also: ClimateDL
- The ClimateDL project brings together experts in climatology, data science and probability and statistical techniques, with as its main objective, the exploration of the different available methods for the forecasting of extreme climate events. Our focus will be on the case of extreme temperatures on the seasonal scale, and we will mainly address the case of the southern South America geographical area, which corresponds to the geographical position of the South American partners. As a completely related goal, we will work on finding new forecasting technologies, centering our research around machine learning tools, but taking into account classic and more recent statistical procedures.
9.2.2 Participation in other International Programs
CEFIPRA CSRP project
Participants: Yann Busnel.
-
Title:
Exploring Graph Neural Networks (GNNs) for Data-driven Modeling of Poly-pharmacy Adverse Drug Events from drug-drug interactions
-
Partner Institution(s):
- ISI Kolkata, India: Prof. Sanghamitra Bandyopadhyay
- Jadavpur University, India: Prof. Ujjwal Maulik
- CHU Rennes, France: Prof. Marc Cuggia, Prof. Pascal Le Corre, Guillaume Bouzillé
- IMT Atlantique, France: Prof. Yann Busnel
-
Date/Duration:
2022-2025 (3 years)
-
Additionnal info/keywords:
Graph neural network, Adverse drug events, Drug-drug interactions, Machine Learning, Poly-pharmacy, Healthcare
9.3 International research visitors
9.3.1 Visits of international scientists
Other international visits to the team
Pablo Rodríguez-Bocca
-
Status
researcher (professor)
-
Institution of origin:
University of the Republic
-
Country:
Uruguay
-
Dates:
July 3 to July 13, 2022
-
Context of the visit:
research activities for the project “ClimateDL” of the Climate AmSud type
-
Mobility program/type of mobility:
research stay
Jorge Graneri
-
Status
researcher (assistant professor)
-
Institution of origin:
University of the Republic
-
Country:
Uruguay
-
Dates:
July 5 to August 31, 2022
-
Context of the visit:
end of project ECOS “MASC”
-
Mobility program/type of mobility:
research stay
Soledad Collazo
-
Status
researcher (assistant professor)
-
Institution of origin:
Université de Buenos Aires
-
Country:
Argentine
-
Dates:
Septembre 19 to 30, 2022
-
Context of the visit:
research activities for the project “ClimateDL” of the Climate AmSud type
-
Mobility program/type of mobility:
research stay
Ujjwal Maulik
-
Status
researcher (professor)
-
Institution of origin:
Jadavpur University, Kolkata
-
Country:
India
-
Dates:
November 9 to 17, 2022
-
Context of the visit:
research activities for the project CEFIPRA CSRP
-
Mobility program/type of mobility:
research stay
9.3.2 Visits to international teams
Gerardo Rubino
-
Visited institution:
CalPoly, Pomona, California
-
Country:
USA
-
Dates:
June 20 to July 2, 2022
-
Context of the visit:
long term collaboration with Prof. Alan Krinik on transient analysis of Markovian models
-
Mobility program/type of mobility:
research stay
Yann Busnel
-
Visited institution:
VJTI, Mumbai
-
Country:
India
-
Dates:
March 29 to April 2, 2022
-
Context of the visit:
long term collaboration with Prof. Dhiren Patel on cybersecurity and blockchain
-
Mobility program/type of mobility:
research stay
Yann Busnel
-
Visited institution:
Indian Statistical Institute, Kolkata
-
Country:
India
-
Dates:
April 2 to April 7, 2022
-
Context of the visit:
long term collaboration with Prof. Sanghamitra Bandyopadhyay on machine learning for e-health
-
Mobility program/type of mobility:
research stay
Yann Busnel
-
Visited institution:
Indian Institute of Technology, Indore
-
Country:
India
-
Dates:
August 23 to August 31, 2022
-
Context of the visit:
long term collaboration with Prof. Kapil Ahuja on machine learning and sampling for big data
-
Mobility program/type of mobility:
invited professorship
9.4 European initiatives
9.4.1 Other european programs/initiatives
EDIH Bretagne
Participants: Yann Busnel.
-
Title:
European Digital Innovation Hub in Cybersecurity
-
Partner Institution(s):
- Pole Images&réseaux
- IMT Atlantique
- Inria
- CNAM Bretagne
- IRT b<>com
- 7 Technopoles Bretagne
- Pole d'Excellence Cyber
- Biotech Santé Bretagne
- Bretagne Développement Innovation
-
Date/Duration:
2022-2025 (3 years)
-
Additionnal info/keywords:
EDIH Bretagne aims to be a major player in the digitization actions of mainly SMEs, but also mid-caps (MC) and non-profit public sector organizations (PSO) having innovation at the heart of its value proposition, and working in the key sectors of S3 (Smart Specialisation Strategy) of the Brittany Region.
9.5 National initiatives
9.5.1 Inria Exploratory Action SNIDE
Participants: Patrick Maillé, Bruno Tuffin.
We are leading the Inria Exploratory Action SNIDE (Search Non neutratlIty DEtection) 2019-2023, involving Ermine and MIMR (Grenoble). The involved researchers are Patrick Maillé and Bruno Tuffin.
Search engines play a key role to access content and are accused to bias their results to favor their own services among others. This has led to the sensitive search neutrality debate, similar to the network neutrality debate currently discussed on the role of ISPs. Our goal in this project is to develop and apply a methodology aiming at highlighting a bias and quantifying its impact.
The current version of our meta-engine (which will be further develop by incorporating outlier detection tests) can be found at this web site.
9.6 Regional initiatives
9.6.1 Rennes Metropole Chair of Research
Participants: Yann Busnel.
-
Title:
Operations Models and Artificial Intelligence to Manage Disasters
-
Partner Institution(s):
- Rennes School of Business
- IMT Atlantique
-
Date/Duration:
2021-2024 (3 years)
-
Additionnal info/keywords:
The objective of this Chair is to provide decision-makers (public organisations, governments, cities, etc.) with decision support tools (mathematical models, decision support systems, software) to deal with natural (including pandemics/ epidemics) and man-made disasters (including technological disasters). We will propose solutions based on distributed algorithms and real-time data processing regarding natural and technological disasters. In particular, we utilize artificial intelligence to ensure near-optimal solutions to the problems raised (e.g., victim location and rescue, aid distribution etc.).
9.6.2 AAP PME NaviDEC
Participants: Soraya Ait Chellouche, Yassine Hadjadj-Aoul.
-
Title:
Network for Automated Virtual services placement in Deep Edge Computing
-
Partner Institution(s):
- Sodira-Connect
- University of Rennes 1
- Inpixal
-
Date/Duration:
2021-2024 (30 months)
-
Additionnal info/keywords:
The NaviDEC project aims to develop an IoT and Image Processing platform in the context of Edge Computing. The idea is to leverage the computing and networking resources at the edge of the network, close to end users and data production and consumption, to allow low latency and better bandwidth utilization. The platform thus developed will be deployed and tested in the maritime sector thanks to the local organizations: Celadon and Thalos.
10 Dissemination
10.1 Promoting scientific activities
Participants: Yann Busnel, Yassine Hadjadj-Aoul, Sofiene Jelassi, Maël Le Treust, Patrick Maillé, Gerardo Rubino, Bruno Tuffin, César Viho.
10.1.1 Scientific events: organisation
Member of the organizing committees
- Bruno Tuffin has been the Chair of the Steering Committee of the International Conference on Monte Carlo Methods and Applications (MCM) series since August 2021.
- Gerardo Rubino and Bruno Tuffin are members of the Steering Committee of the International Workshop on Rare Event Simulation (RESIM).
- Yassine Hadjadj-Aoul is chairing the steering committee of the International Conference on Information and Communication Technologies for Disaster Management (ICT-DM)
10.1.2 Scientific events: selection
Chair of conference program committees
- Yassine Hadjadj-Aoul served as a TPC track chair of the IEEE International Smart Cities Conference (IEEE ISC2), Paphos, Cyprus (September 2022)
Member of the conference program committees
- Bruno Tuffin served as a TPC member for MCQMC'22, ITC 34, ECOFEC 2022, IEEE Globecom 2022.
- Gerardo Rubino served as TPC member of MCQMC'22, QEST'22, MASCOTS'22
- César Viho served as TPC member of CARI'22, ICN'22
- Bruno Sericola served as TPC member for NCA'22
- Yann Busnel served as TPC member for NCA'22
- Patrick Maillé served as TPC member of IEEE Globecom 2022, GECON 2022
- Yassine Hadjadj-Aoul served TPC member of several conferences, including IEEE Globecom'22, IEEE ICC'22, IEEE ISC2'22, IEEE MASCOTS'22
10.1.3 Journal
Member of the editorial boards
- Bruno Tuffin is Area Editor for INFORMS Journal on Computing and Associate Editor for ACM Transactions on Modeling and Computer Simulation and Queueing Systems.
- Gerardo Rubino is Associate Editor of the Journal of Dynamics & Games.
- Patrick Maillé is an Associate Editor for Electronic Commerce Research and Applications and for the IEEE Open Journal of the Communications Society
- Bruno Sericola serves as associate editor for Performance Evaluation. He is also Editor in Chief of the books series “StochasticModels in Computer Science and Telecommunications Networks”, ISTE/WILEY.
- Maël Le Treust serves as associate editor for IEEE Transactions on Information Theory
10.1.4 Invited talks
- Bruno Tuffin gave a talk at the 1st SCI-Rennes seminar in September 2022: “Les moteurs de recherche sont-ils biaisés ? Pourquoi et comment étudier cette problématique ?”
- Yassine Hadjadj-Aoul and Gerardo Rubino gave a talk about the evaluation of the Quality of Experience of video-based and audio-based applications and services on the Internet, and about our PSQA technology, at the DGA/CELAR Center, on July 7, 2022.
- Yassine Hadjadj-Aoul gave a talk, entitled “Robust Deep Reinforcement learning-based Network Slicing”, at a Webinar organized by Nokia Bell Labs.
- Yassine Hadjadj-Aoul gave a talk, entitled “Resource Management in Next-Generation Networks”, at ESIEE Paris, Gustave Eiffel University.
- Gerardo Rubino gave a talk about time series forecasting, co-authored with Sebastián Basterrech, at an open workshop of the project ClimateDL that took place associated with the conference LA-CCI'22.
- Yann Busnel gave an invited talk about Involvement of AI in Cyber-Attack Detection at the 3rd Japanese-German-French AI Symposium, Tokyo, Japan, October 2022.
- Yann Busnel gave an invited talk about The value of learning for securing large-scale networks at 7th Franco-Japanese Cybersecurity Workshop, Tokyo, Japan, October 2022
- Yann Busnel gave an invited talk about Drone Fleet Coordination: How self-organisation solves all your problems at IIT Indore, India, August 2022
- Yann Busnel gave an invited talk about How self-organisation and decentralisation should make a smarter world? at UPL Sustainable University, India, March 2022
- Yann Busnel gave an invited talk about L'auto-organisation et la décentralisation pour un monde plus intelligent ! at CITI, Lyon, February 2022
- Yann Busnel gave an invited tutorial about Drone Fleet Coordination: How self-organisation solves all your problems at GDR RSD ResCom Summer School, Yennes, June 2022
- Sofiene Jelassi gave an invited talk about Multimedia Streaming Services Virtualization for an Industrial-Grade QoE-based Control Loop at the First International Colloquium on Intelligent Systems and Industry 4.0, Durazno, Uruguay. April 21, 2022
10.1.5 Leadership within the scientific community
- Gerardo Rubino is the coordinator of the research action “Analytics and Machine Learning” between INRIA and Nokia Bell Labs.
10.1.6 Research administration
- Bruno Tuffin was member of the Inria Rennes CRCN selection committee in 2022.
- Gerardo Rubino served at the CSV (the technical committee) of the Images and Networks Cluster of Brittany, France, since its foundation, until March 2022.
- Maël Le Treust is secretary of the scientific council of CNRS INS2I.
- Yann Busnel is head of D2 – Network, Telecommunication and Services department – at UMR IRISA
- Yann Busnel is head of Network Systems, Cybersecurity and Digital Law department at IMT Atlantique
- Patrick Maillé serves as an elected member for the Research Committee of IMT Atlantique
10.2 Teaching - Supervision - Juries
Participants:
10.2.1 Teaching
- Master: Bruno Tuffin, MEPS (simulation and performance evaluation), 22 hours, M1 University of Rennes 1, France
- Master: Bruno Tuffin, MEPS (simulation), 20 hours, ENS Rennes, France
- Master: Patrick Maillé & Bruno Tuffin, GTA (Game Theory and Applications), 20 hours, M2, ENS Rennes, France
- Master: Patrick Maillé (Game Theory and Agent-Based Modeling), 20 hours, IMT Atlantique
- Bachelor L3: Patrick Maillé (Network performance modeling basics), 20 hours, IMT Atlantique
- Master: Patrick Maillé (Economics of Digital Platforms), 15 hours, IMT Atlantique
- Master, 1st year: Patrick Maillé (Probability and Statistics), 20 hours, IMT Atlantique
- Master, 2nd year: Gerardo Rubino, Scalable Network Infrastructure (SNI), 10 hours, The Research in Computer Science (SIF) master and EIT Digital Master/University of Rennes 1, France
- Supelec Rennes 3rd year: Gerardo Rubino, Dependability Analysis, 15 hours.
- Master 2nd year: Gerardo Rubino, Quality of Experience, twice 4 hours (two different groups of students), Esir/University of Rennes 1, France
- Master Pro 2nd year: Gerardo Rubino, Quality of Experience, 4 hours Esir/University of Rennes 1, France
- Master: Bruno Sericola, Logistic and performance, 12h, M2, Faculté de sciences économiques, Univ of Rennes 1, France
- Master: Bruno Sericola, MEPS (performance evaluation), 35h, M1, Univ Rennes 1 and ENS Rennes, France
- MOOC on “Ose les métiers de l'industrie du futur”, available on Fun: Yann Busnel (in charge of one week of class)
- IMT Atlantique 3rd year: Yann Busnel, Blockchain: Synchronisation and Token Economy, 3 hours.
- Master M2-Cyber: César Viho, Dynamic Routing Algorithms, 6 hours, ISTIC/University of Rennes 1, France
- Master M1-Cloud and Networks: César Viho, Networks : from Services to protocols, 36 hours, ISTIC/University of Rennes 1, France
- Bachelor L3: César Viho, Algorithms on graphs, 40 hours, Istic/University of Rennes 1, France
- Bachelor L2: César Viho, Network basics and main protocols, 32 hours, ISTIC/University of Rennes 1, France
- Bachelor L3: Sofiene Jelassi, Oriented Object Programming (JAVA), 44 hours, Istic/University of Rennes 1, France
- Bachelor L3: Sofiene Jelassi, Computer Networks, 20 hours, Istic/University of Rennes 1, France
- Bachelor L3: Sofiene Jelassi, Computer Networks, 4 hours, Istic/University of Rennes 1, France
- BUT 2 : Soraya Ait Chellouche, Core Networks, 35 hours, IUT de Lannion - Univ Rennes 1, France
- BUT 2 : Soraya Ait Chellouche, Network security, 12 hours, IUT de Lannion - Univ Rennes 1, France
- BUT 1 : Soraya Ait Chellouche, Internet architecture, 50 hours, IUT de Lannion - Univ Rennes 1, France
- BUT 1 : Soraya Ait Chellouche, Network services, 26 hours, IUT de Lannion - Univ Rennes 1, France
- BUT 1 : Soraya Ait Chellouche, Introduction to networking, 20 hours, IUT de Lannion - Univ Rennes 1, France
- LP ASUR : Soraya Ait Chellouche, TIC, 50 hours, IUT de Saint Malo - Univ Rennes 1, France
- Engineering school 3rd year & Master 2, Soraya Ait Chellouche, Voice Over IP, 15 hours, ESIR & ISTIC - univ Rennes 1, France
- Master, 2nd year: Yassine Hadjadj-Aoul, Scalable Network Infrastructure (SNI), 18 hours, The Research in Computer Science (SIF) master and EIT DigitalMaster/University of Rennes 1, France
- Master, pro 2nd year: Yassine Hadjadj-Aoul, Multimedia streaming over IP (MMR), 48 hours, Esir/University of Rennes 1, France
- Master, pro 2nd year: Yassine Hadjadj-Aoul,Multimedia services in IP networks (RSM), 29 hours, Esir/University of Rennes 1, France
- Master, pro 2nd year: Yassine Hadjadj-Aoul, Software Defined Networks, 10 hours, Istic/University of Rennes 1, France
- Master, 2nd year: Yassine Hadjadj-Aoul, Video streaming over IP, 8 hours, Istic/University of Rennes 1, France
- Master: YassineHadjadj-Aoul, Introduction to networking (RES), 26 hours, Esir/University of Rennes 1, France
- Master: Yassine Hadjadj-Aoul,Mobile and wireless networking (RESA), 20 hours, Esir/University of Rennes 1, France
- Master 2nd year: Yassine Hadjadj-Aoul, Overview of IoT technologies: focus on LPWAN, 2 hours, INSA, France
- C. Viho was a member of the juries for the recruitment of young Associate Professors and senior Professors at ISTIC-Université Rennes 1.
- Yann Busnel was a member of the juries for the recruitment of young Associate Professors and senior Professors at ESIR-Université Rennes 1 and IMT Atlantique.
- Soraya Ait Chellouche was member of validation and certification of studies juries for military personal on network security.
- Soraya Ait Chellouche supervised 15 students on dual study program, IUT de Lannion/IUT de Saint Malo - univ-Rennes 1 & engineering school ESAIP.
10.2.2 Supervision
-
Thesis defended in 2022:
- PhD of Hiba Dakdouk (formerly member of Dionysos team), “Massive Multi-Player ”; Advisors: Patrick Maillé, Raphaël Féraud, Nadège Varsier; IMT Atlantique. Defense on May 30, 2022.
- PhD of Imane Taibi (formerly member of Dionysos team), “Big data analysis for network monitoring and troubleshooting”; Advisors: Gerardo Rubino, Chadi Barakat and Yassine Hadjadj-Aoul; Univ. of Rennes 1. Defense on September 19, 2022.
- PhD of Rony Bou Rouphael (external thesis but co-supervision by a team member), “Strategic Compression for Multi-User Bayesian Persuasion”; Advisors: Maël Le Treust and Inbar Fijalkow; CY Cergy Paris University. Defense on December 1st, 2022.
- PhD of Alexandre Dey (external thesis but co-supervision by a team member), “Data science in support of cybersecurity operations”; Advisors: Yann Busnel and Eric Totel; IMT Atlantique. Defense on December 2nd, 2022.
-
Thesis in progress:
- PhD in progress: Soumeya Kaada, “Resiliency as a Service for 5G networks using Machine Learning”, CIFRE Thesis with Nokia Bell Labs/INRIA, started in January 2020, supervised by Sofiene Jelassi and Gerardo Rubino.
- PhD in progress: Amine Rguez, “An availability-aware NFVs placement using Deep Reinforcement Learning”, CIFRE Thesis with EXFO/INRIA, started in November 2020, supervised by Yassine Hadjadj-Aoul and Gerardo Rubino.
- PhD in progress: Abdelmounaim Bouroudi, “Multi-Agent Deep Reinforcement Learning for Resources Allocation and Scheduling in 5G/6G”, CIFRE Thesis with Nokia Bell Labs, started in November 2021, supervised by Yassine Hadjadj-Aoul and Gerardo Rubino.
- PhD in progress: Sid Ali Hamideche, “Automation beyond 5G: Machine learning applied to user behaviour profiling of mobile services”, CIFRE Thesis with Nokia Bell Labs/IRISA-Université Rennes 1, started in 2020, supervised by César Viho.
- PhD in progress: Ahcene Boumhand, “Multi-task learning to discover the context of the Home”, CIFRE Thesis with Orange/IRISA-Université Rennes 1, started in 2021, supervised by César Viho and Yassine Hadjadj-Aoul.
- PhD in progress: Khalil El Houssni, “Analysis of the interactions between cybersecurity IA systems using game theory”, Thesis with IMT Atlantique and B<>Com, Started in December 2021, supervised by Yann Busnel and Patrick Maillé.
- PhD in progress: Antoine Rebstock, “Extraction of probable scenarios by correlation of alerts”, started in October 2021; supervisors: Yann Busnel, Romaric Ludinard, IMT Atlantique, and Stéphane Paquelet, IRT b<>com.
-
External thesis co-supervised by team members:
- PhD in progress (PhD at St Joseph University of Beyrouth): Ali Hodroj, Enhancing content delivery tomulti-homed users in broadband mobile networks, started in November 2015; supervisors: Bruno Sericola, Marc Ibrahim and Yassine Hadjadj-Aoul, St Joseph University of Beyrouth.
- PhD in progress (PhD within Sirocco team): Nicolas Charpenay, “Combinatorial and Strategic Aspect in Information Theory”; started in October 2020; Advisors: Maël Le Treust and Aline Roumy; University of Rennes 1.
- PhD in progress (PhD at IMT Atlantique): Léo Lavaur, “Threat intelligence tools for IoT threat indexing and cooperative sharin`”, started in October 2020; supervisors: Yann Busnel, Marc-Oliver Pahl, Fabien Autrel, IMT Atlantique.
- PhD in progress (PhD at IMT Atlantique): Pierre-Marie Lechevalier, “Trust and security in the composition of distributed network services and functions”, started in October 2021; supervisors: Yann Busnel, Géraldine Texier, Hélène Le Bouder, Romaric Ludinard, IMT Atlantique.
- PhD in progress (PhD within Adopnet IRISA team): Zahra Al Attar, “Network Function Virtualization and Slice Monitoring”; started in November 2021; Advisors: Yassine hadjadj-aoul and Géraldine Texier, IMT Atlantique.
10.2.3 Juries
- Bruno Tuffin was an examiner for the HDR defense of Maël Le Treust (ENSEA) on 29/11/2022.
- Bruno Tuffin was reviewer for the PhD defenses of Maxim El Masri (Université de Toulouse) in March 2022 and of Mandar Datar (Université d'Avignon) in May 2022.
- Bruno Tuffin was an examiner for the PhD of Thomas Tournaire (Institut Polytechnique de Paris) in May 2022.
- Bruno Sericola was president of the jury for the PhD defense of Mohamed Aimen Djari (Université de Rennes) in December 2022
- Yann Busnel was reviewer for the PhD defense of Swati Kumari (Thapar Institute of Engineering & Technology, India), in October 2022.
10.3 Popularization
Participants: Yann Busnel, Yassine Hadjadj-Aoul, Patrick Maillé, Bruno Tuffin, César Viho.
10.3.1 Internal or external Inria responsibilities
- César Viho was director of the MathSTIC doctoral school (Mathematics, Electronics and Computer Sciences) in charge of managing the recruitment of around 1100 PhD students and their activities during their doctorate, in all the concerned areas of the UBL (Université Bretagne Loire).
- Yann Busnel is IMT Atlantique representative on the Scientific and Pedagogical Committee of the EUR CyberSchool
- Yann Busnel is co-head of the MOOC Editorial Board at Institut Mines-Télécom
10.3.2 Articles and contents
Four our activities on network economics, we aim at publishing white papers and popularization articles. In 2022, we published:
- Interview in Alternatives Économiques : Faut-il faire payer la bande passante aux Gafam? 30 mai 2022.
- A. Blandin, P. Maillé, B. Tuffin. Neutralité du Net : l'UE est-elle en train d'opérer un revirement ? The Conversation, 30 août 2022 (also published in le Journal du Dimanche and several other newspapers) 48.
- G. Andrade-Barroso, P. Maillé and B. Tuffin. Un biais dans le moteur ? Pour la Science, 2022 49.
- Yassine Hadjadj-Aoul, Patrick Maillé, Bruno Tuffin. La neutralité du Net face à l’évolution des réseaux, Interstices, 2022 50.
10.3.3 Education
- G. Rubino makes regular presentations to high school students about the research work in general, and specific technical topics in particular. Current talks:
- Randomness as a tool
- Internet as a research problem
- Greatest challenges in maths: the Riemann Hypothesis
- Greatest challenges in math/computer science: the “P versus NP” problem
11 Scientific production
11.1 Publications of the year
International journals
International peer-reviewed conferences
Conferences without proceedings
Scientific books
Scientific book chapters
Doctoral dissertations and habilitation theses
Reports & preprints
Other scientific publications
11.2 Other
Scientific popularization
Educational activities