Keywords
Computer Science and Digital Science
- A5.1.1. Engineering of interactive systems
- A5.1.2. Evaluation of interactive systems
- A5.1.4. Brain-computer interfaces, physiological computing
- A5.1.5. Body-based interfaces
- A5.1.6. Tangible interfaces
- A5.1.7. Multimodal interfaces
- A5.3.3. Pattern recognition
- A5.4.1. Object recognition
- A5.4.2. Activity recognition
- A5.7.3. Speech
- A5.8. Natural language processing
- A5.10.5. Robot interaction (with the environment, humans, other robots)
- A5.10.7. Learning
- A5.10.8. Cognitive robotics and systems
- A5.11.1. Human activity analysis and recognition
- A6.3.1. Inverse problems
- A9. Artificial intelligence
- A9.2. Machine learning
- A9.5. Robotics
- A9.7. AI algorithmics
Other Research Topics and Application Domains
- B1.2.1. Understanding and simulation of the brain and the nervous system
- B1.2.2. Cognitive science
- B5.6. Robotic systems
- B5.7. 3D printing
- B5.8. Learning and training
- B9. Society and Knowledge
- B9.1. Education
- B9.1.1. E-learning, MOOC
- B9.2. Art
- B9.2.1. Music, sound
- B9.2.4. Theater
- B9.6. Humanities
- B9.6.1. Psychology
- B9.6.8. Linguistics
- B9.7. Knowledge dissemination
1 Team members, visitors, external collaborators
Research Scientists
- Pierre-Yves Oudeyer [Team leader, Inria, Senior Researcher, HDR]
- Clément Moulin-Frier [INRIA, Researcher]
- Eleni Nisioti [INRIA, Starting Research Position]
- Hélène Sauzéon [INRIA, Senior Researcher, from Sep 2022, HDR]
Faculty Members
- David Filliat [ENSTA, Professor, HDR]
- Cécile Mazon [UNIV BORDEAUX, Associate Professor]
- Mai Nguyen [ENSTA Paris Tech, Associate Professor]
Post-Doctoral Fellows
- Cedric Colas [INRIA]
- Eric Meyer [INRIA]
- Remy Portelas [INRIA, until Jan 2022]
PhD Students
- Rania Abdelghani [EVIDENCEB]
- Maxime Adolphe [ONEPOINT]
- Louis Annabi [UMA-ENSTA, from Jun 2022]
- Thomas Carta [UNIV BORDEAUX]
- Mayalen Etcheverry [POIETIS]
- Gautier Hamon [INRIA]
- Tristan Karch [INRIA]
- Grgur Kovac [Inria, until Jan 2022]
- Remy Portelas [INRIA, from Mar 2022]
- Matisse Poupard [CATIE, CIFRE, from Apr 2022]
- Thomas Rojat [GROUPE RENAULT]
- Clément Romac [HUGGING FACE SAS, CIFRE]
- Isabeau Saint-Supery [UNIV BORDEAUX]
- Maria Teodorescu [INRIA]
Technical Staff
- Jesse Lin [INRIA, Engineer, from Oct 2022]
Interns and Apprentices
- Kamélia Belassel [Inria, Intern, until Jun 2022]
- Lena Coulon [Inria, Intern, from Jun 2022 until Jul 2022]
- Yoann Lemesle [Inria, from Apr 2022 until Sep 2022]
- Pauline Lucas [INRIA, until Jun 2022]
- Elias Masquil [Inria, Intern, until Sep 2022]
- Anna Moustaïd [Inria, Intern, until Jun 2022]
- Mathieu Perie [INRIA, Apprentice]
- Erwan Plantec [Inria, Intern, until Sep 2022]
- Agathe Vianey-Liaud [Inria, Intern, from Jun 2022 until Jul 2022]
Administrative Assistant
- Nathalie Robin [INRIA]
Visiting Scientists
- Yadurshana Sivashankar [University of Waterloo, until Apr 2022]
- Yen-Hsiang Wang [National Chung Hsing University]
External Collaborator
- Didier Roy [INRIA]
2 Overall objectives
Abstract: The Flowers project-team studies models of open-ended development and learning. These models are used as tools to help us understand better how children learn, as well as to build machines that learn like children, i.e. developmental artificial intelligence, with applications in educational technologies, assisted scientific discovery, video games, robotics and human-computer interaction.
Context: Great advances have been made recently in artificial intelligence concerning the topic of how autonomous agents can learn to act in uncertain and complex environments, thanks to the development of advanced Deep Reinforcement Learning techniques. These advances have for example led to impressive results with AlphaGo 169 or algorithms that learn to play video games from scratch 149, 128. However, these techniques are still far away from solving the ambitious goal of lifelong autonomous machine learning of repertoires of skills in real-world, large and open environments. They are also very far from the capabilities of human learning and cognition. Indeed, developmental processes allow humans, and especially infants, to continuously acquire novel skills and adapt to their environment over their entire lifetime. They do so autonomously, i.e. through a combination of self-exploration and linguistic/social interaction with their social peers, sampling their own goals while benefiting from the natural language guidance of their peers, and without the need for an “engineer” to open and retune the brain and the environment specifically for each new task (e.g. for providing a task-specific external reward channel). Furthermore, humans are extremely efficient at learning fast (few interactions with their environment) skills that are very high-dimensional both in perception and action, while being embedded in open changing environments with limited resources of time, energy and computation.
Thus, a major scientific challenge in artificial intelligence and cognitive sciences is to understand how humans and machines can efficiently acquire world models, as well as open and cumulative repertoires of skills over an extended time span. Processes of sensorimotor, cognitive and social development are organized along ordered phases of increasing complexity, and result from the complex interaction between the brain/body with its physical and social environment. Making progress towards these fundamental scientific challenges is also crucial for many downstream applications. Indeed, autonomous lifelong learning capabilities similar to those shown by humans are key requirements for developing virtual or physical agents that need to continuously explore and adapt skills for interacting with new or changing tasks, environments, or people. This is crucial for applications like assistive technologies with non-engineer users, such as robots or virtual agents that need to explore and adapt autonomously to new environments, adapt robustly to potential damages of their body, or help humans to learn or discover new knowledge in education settings, and need to communicate through natural language with human users, grounding the meaning of sentences into their sensorimotor representations.
The Developmental AI approach: Human and biological sciences have identified various families of developmental mechanisms that are key to explain how infants can acquire so robustly a wide diversity of skills 130, 148, in spite of the complexity and high-dimensionality of the body 87 and the open-endedness of its potential interactions with the physical and social environment. To advance the fundamental understanding of these mechanisms of development as well as their transposition in machines, the FLOWERS team has been developing an approach called Developmental artificial intelligence, leveraging and integrating ideas and techniques from developmental robotics (184, 140, 92, 154, Deep (Reinforcement) Learning and developmental psychology. This approach consists in developing computational models that leverage advanced machine learning techniques such as intrinsically motivated Deep Reinforcement Learning, in strong collaboration with developmental psychology and neuroscience. In particular, the team focuses on models of intrinsically motivated learning and exploration (also called curiosity-driven learning), with mechanisms enabling agents to learn to represent and generate their own goals, self-organizing a learning curriculum for efficient learning of world models and skill repertoire under limited resources of time, energy and compute. The team also studies how autonomous learning mechanisms can enable humans and machines to acquire and develop grounded and culturally shared language skills, using neuro-symbolic architectures for learning structured representations and handling systematic compositionality and generalization.
Our fundamental research is organized along three strands:
-
Strand 1: Lifelong autonomous learning in machines.
Understanding how developmental mechanisms can be functionally formalized/transposed in machines and explore how they can allow these machines to acquire efficiently open-ended repertoires of skills through self-exploration and social interaction.
-
Strand 2: Computational models as tools to understand human development in cognitive sciences.
The computational modelling of lifelong learning and development mechanisms achieved in the team centrally targets to contribute to our understanding of the processes of sensorimotor, cognitive and social development in humans. In particular, it provides a methodological basis to analyze the dynamics of interactions across learning and inference processes, embodiment and the social environment, allowing to formalize precise hypotheses and later on test them in experimental paradigms with animals and humans. A paradigmatic example of this activity is the Neurocuriosity project achieved in collaboration with the cognitive neuroscience lab of Jacqueline Gottlieb, where theoretical models of the mechanisms of information seeking, active learning and spontaneous exploration have been developed in coordination with experimental evidence and investigation 19, 32.
-
Strand 3: Applications.
Beyond leading to new theories and new experimental paradigms to understand human development in cognitive science, as well as new fundamental approaches to developmental machine learning, the team explores how such models can find applications in robotics, human-computer interaction, multi-agent systems, automated discovery and educational technologies. In robotics, the team studies how artificial curiosity combined with imitation learning can provide essential building blocks allowing robots to acquire multiple tasks through natural interaction with naive human users, for example in the context of assistive robotics. The team also studies how models of curiosity-driven learning can be transposed in algorithms for intelligent tutoring systems, allowing educational software to incrementally and dynamically adapt to the particularities of each human learner, and proposing personalized sequences of teaching activities.
3 Research program
Research in artificial intelligence, machine learning and pattern recognition has produced a tremendous amount of results and concepts in the last decades. A blooming number of learning paradigms - supervised, unsupervised, reinforcement, active, associative, symbolic, connectionist, situated, hybrid, distributed learning... - nourished the elaboration of highly sophisticated algorithms for tasks such as visual object recognition, speech recognition, robot walking, grasping or navigation, the prediction of stock prices, the evaluation of risk for insurances, adaptive data routing on the internet, etc... Yet, we are still very far from being able to build machines capable of adapting to the physical and social environment with the flexibility, robustness, and versatility of a one-year-old human child.
Indeed, one striking characteristic of human children is the nearly open-ended diversity of the skills they learn. They not only can improve existing skills, but also continuously learn new ones. If evolution certainly provided them with specific pre-wiring for certain activities such as feeding or visual object tracking, evidence shows that there are also numerous skills that they learn smoothly but could not be “anticipated” by biological evolution, for example learning to drive a tricycle, using an electronic piano toy or using a video game joystick. On the contrary, existing learning machines, and robots in particular, are typically only able to learn a single pre-specified task or a single kind of skill. Once this task is learnt, for example walking with two legs, learning is over. If one wants the robot to learn a second task, for example grasping objects in its visual field, then an engineer needs to re-program manually its learning structures: traditional approaches to task-specific machine/robot learning typically include engineer choices of the relevant sensorimotor channels, specific design of the reward function, choices about when learning begins and ends, and what learning algorithms and associated parameters shall be optimized.
As can be seen, this requires a lot of important choices from the engineer, and one could hardly use the term “autonomous” learning. On the contrary, human children do not learn following anything looking like that process, at least during their very first years. Babies develop and explore the world by themselves, focusing their interest on various activities driven both by internal motives and social guidance from adults who only have a folk understanding of their brains. Adults provide learning opportunities and scaffolding, but eventually young babies always decide for themselves what activity to practice or not. Specific tasks are rarely imposed to them. Yet, they steadily discover and learn how to use their body as well as its relationships with the physical and social environment. Also, the spectrum of skills that they learn continuously expands in an organized manner: they undergo a developmental trajectory in which simple skills are learnt first, and skills of progressively increasing complexity are subsequently learnt.
A link can be made to educational systems where research in several domains have tried to study how to provide a good learning or training experience to learners. This includes the experiences that allow better learning, and in which sequence they must be experienced. This problem is complementary to that of the learner who tries to progress efficiently, and the teacher here has to use as efficiently the limited time and motivational resources of the learner. Several results from psychology 86 and neuroscience 118 have argued that the human brain feels intrinsic pleasure in practicing activities of optimal difficulty or challenge. A teacher must exploit such activities to create positive psychological states of flow 107 for fostering the indivual engagement in learning activities. A such view is also relevant for reeducation issues where inter-individual variability, and thus intervention personalization are challenges of the same magnitude as those for education of children.
A grand challenge is thus to be able to build machines that possess this capability to discover, adapt and develop continuously new know-how and new knowledge in unknown and changing environments, like human children. In 1950, Turing wrote that the child's brain would show us the way to intelligence: “Instead of trying to produce a program to simulate the adult mind, why not rather try to produce one which simulates the child's” 177. Maybe, in opposition to work in the field of Artificial Intelligence who has focused on mechanisms trying to match the capabilities of “intelligent” human adults such as chess playing or natural language dialogue 123, it is time to take the advice of Turing seriously. This is what a new field, called developmental (or epigenetic) robotics, is trying to achieve 140184. The approach of developmental robotics consists in importing and implementing concepts and mechanisms from developmental psychology 147, cognitive linguistics 106, and developmental cognitive neuroscience 129 where there has been a considerable amount of research and theories to understand and explain how children learn and develop. A number of general principles are underlying this research agenda: embodiment 89158, grounding 121, situatedness 170, self-organization 173153, enaction 179, and incremental learning 96.
Among the many issues and challenges of developmental robotics, two of them are of paramount importance: exploration mechanisms and mechanisms for abstracting and making sense of initially unknown sensorimotor channels. Indeed, the typical space of sensorimotor skills that can be encountered and learnt by a developmental robot, as those encountered by human infants, is immensely vast and inhomogeneous. With a sufficiently rich environment and multimodal set of sensors and effectors, the space of possible sensorimotor activities is simply too large to be explored exhaustively in any robot's life time: it is impossible to learn all possible skills and represent all conceivable sensory percepts. Moreover, some skills are very basic to learn, some other very complicated, and many of them require the mastery of others in order to be learnt. For example, learning to manipulate a piano toy requires first to know how to move one's hand to reach the piano and how to touch specific parts of the toy with the fingers. And knowing how to move the hand might require to know how to track it visually.
Exploring such a space of skills randomly is bound to fail or result at best on very inefficient learning 155. Thus, exploration needs to be organized and guided. The approach of epigenetic robotics is to take inspiration from the mechanisms that allow human infants to be progressively guided, i.e. to develop. There are two broad classes of guiding mechanisms which control exploration:
- internal guiding mechanisms, and in particular intrinsic motivation, responsible of spontaneous exploration and curiosity in humans, which is one of the central mechanisms investigated in FLOWERS, and technically amounts to achieve online active self-regulation of the growth of complexity in learning situations;
- social learning and guidance, a learning mechanisms that exploits the knowledge of other agents in the environment and/or that is guided by those same agents. These mechanisms exist in many different forms like emotional reinforcement, stimulus enhancement, social motivation, guidance, feedback or imitation, some of which being also investigated in FLOWERS.
Internal guiding mechanisms
In infant development, one observes a progressive increase of the complexity of activities with an associated progressive increase of capabilities 147, children do not learn everything at one time: for example, they first learn to roll over, then to crawl and sit, and only when these skills are operational, they begin to learn how to stand. The perceptual system also gradually develops, increasing children perceptual capabilities other time while they engage in activities like throwing or manipulating objects. This make it possible to learn to identify objects in more and more complex situations and to learn more and more of their physical characteristics.
Development is therefore progressive and incremental, and this might be a crucial feature explaining the efficiency with which children explore and learn so fast. Taking inspiration from these observations, some roboticists and researchers in machine learning have argued that learning a given task could be made much easier for a robot if it followed a developmental sequence and “started simple” 84113. However, in these experiments, the developmental sequence was crafted by hand: roboticists manually build simpler versions of a complex task and put the robot successively in versions of the task of increasing complexity. And when they wanted the robot to learn a new task, they had to design a novel reward function.
Thus, there is a need for mechanisms that allow the autonomous control and generation of the developmental trajectory. Psychologists have proposed that intrinsic motivations play a crucial role. Intrinsic motivations are mechanisms that push humans to explore activities or situations that have intermediate/optimal levels of novelty, cognitive dissonance, or challenge 86107109. Futher, the exploration of critical role of intrinsic motivation as lever of cognitive developement for all and for all ages is today expanded to several fields of research, closest to its original study, special education or cognitive aging, and farther away, neuropsychological clinical research. The role and structure of intrinsic motivation in humans have been made more precise thanks to recent discoveries in neuroscience showing the implication of dopaminergic circuits and in exploration behaviours and curiosity 108125167. Based on this, a number of researchers have began in the past few years to build computational implementation of intrinsic motivation 15515616585126143166. While initial models were developed for simple simulated worlds, a current challenge is to manage to build intrinsic motivation systems that can efficiently drive exploratory behaviour in high-dimensional unprepared real world robotic sensorimotor spaces 156, 155, 157, 164. Specific and complex problems are posed by real sensorimotor spaces, in particular due to the fact that they are both high-dimensional as well as (usually) deeply inhomogeneous. As an example for the latter issue, some regions of real sensorimotor spaces are often unlearnable due to inherent stochasticity or difficulty, in which case heuristics based on the incentive to explore zones of maximal unpredictability or uncertainty, which are often used in the field of active learning 100122 typically lead to catastrophic results. The issue of high dimensionality does not only concern motor spaces, but also sensory spaces, leading to the problem of correctly identifying, among typically thousands of quantities, those latent variables that have links to behavioral choices. In FLOWERS, we aim at developing intrinsically motivated exploration mechanisms that scale in those spaces, by studying suitable abstraction processes in conjunction with exploration strategies.
Socially Guided and Interactive Learning
Social guidance is as important as intrinsic motivation in the cognitive development of human babies 147. There is a vast literature on learning by demonstration in robots where the actions of humans in the environment are recognized and transferred to robots 83. Most such approaches are completely passive: the human executes actions and the robot learns from the acquired data. Recently, the notion of interactive learning has been introduced in 174, 88, motivated by the various mechanisms that allow humans to socially guide a robot 162. In an interactive context the steps of self-exploration and social guidance are not separated and a robot learns by self exploration and by receiving extra feedback from the social context 174, 134, 144.
Social guidance is also particularly important for learning to segment and categorize the perceptual space. Indeed, parents interact a lot with infants, for example teaching them to recognize and name objects or characteristics of these objects. Their role is particularly important in directing the infant attention towards objects of interest that will make it possible to simplify at first the perceptual space by pointing out a segment of the environment that can be isolated, named and acted upon. These interactions will then be complemented by the children own experiments on the objects chosen according to intrinsic motivation in order to improve the knowledge of the object, its physical properties and the actions that could be performed with it.
In FLOWERS, we are aiming at including intrinsic motivation system in the self-exploration part thus combining efficient self-learning with social guidance 151, 152. We also work on developing perceptual capabilities by gradually segmenting the perceptual space and identifying objects and their characteristics through interaction with the user 142 and robots experiments 127. Another challenge is to allow for more flexible interaction protocols with the user in terms of what type of feedback is provided and how it is provided 139.
Exploration mechanisms are combined with research in the following directions:
Cumulative learning, reinforcement learning and optimization of autonomous skill learning
FLOWERS develops machine learning algorithms that can allow embodied machines to acquire cumulatively sensorimotor skills. In particular, we develop optimization and reinforcement learning systems which allow robots to discover and learn dictionaries of motor primitives, and then combine them to form higher-level sensorimotor skills.
Autonomous perceptual and representation learning
In order to harness the complexity of perceptual and motor spaces, as well as to pave the way to higher-level cognitive skills, developmental learning requires abstraction mechanisms that can infer structural information out of sets of sensorimotor channels whose semantics is unknown, discovering for example the topology of the body or the sensorimotor contingencies (proprioceptive, visual and acoustic). This process is meant to be open- ended, progressing in continuous operation from initially simple representations towards abstract concepts and categories similar to those used by humans. Our work focuses on the study of various techniques for:
- autonomous multimodal dimensionality reduction and concept discovery;
- incremental discovery and learning of objects using vision and active exploration, as well as of auditory speech invariants;
- learning of dictionaries of motion primitives with combinatorial structures, in combination with linguistic description;
- active learning of visual descriptors useful for action (e.g. grasping).
Embodiment and maturational constraints
FLOWERS studies how adequate morphologies and materials (i.e. morphological computation), associated to relevant dynamical motor primitives, can importantly simplify the acquisition of apparently very complex skills such as full-body dynamic walking in biped. FLOWERS also studies maturational constraints, which are mechanisms that allow for the progressive and controlled release of new degrees of freedoms in the sensorimotor space of robots.
Discovering and abstracting the structure of sets of uninterpreted sensors and motors
FLOWERS studies mechanisms that allow a robot to infer structural information out of sets of sensorimotor channels whose semantics is unknown, for example the topology of the body and the sensorimotor contingencies (proprioceptive, visual and acoustic). This process is meant to be open-ended, progressing in continuous operation from initially simple representations to abstract concepts and categories similar to those used by humans.
Emergence of social behavior in multi-agent populations
FLOWERS studies how populations of interacting learning agents can collectively acquire cooperative or competitive strategies in challenging simulated environments. This differs from "Social learning and guidance" presented above: instead of studying how a learning agent can benefit from the interaction with a skilled agent, we rather consider here how social behavior can spontaneously emerge from a population of interacting learning agents. We focus on studying and modeling the emergence of cooperation, communication and cultural innovation based on theories in behavioral ecology and language evolution, using recent advances in multi-agent reinforcement learning.
Cognitive variability across Lifelong development and (re)educational Technologies
Over the past decade, the progress in the field of curiosity-driven learning generates a lot of hope, especially with regard to a major challenge, namely the inter-individual variability of developmental trajectories of learning, which is particularly critical during childhood and aging or in conditions of cognitive disorders. With the societal purpose of tackling of social inegalities, FLOWERS deals to move forward this new research avenue by exploring the changes of states of curiosity across lifespan and across neurodevelopemental conditions (neurotypical vs. learning disabilities) while designing new educational or rehabilitative technologies for curiosity-driven learning. The information gaps or learning progress, and their awareness are the core mechanisms of this part of research program due to high value as brain fuel by which the individual's internal intrinsic state of motivation is maintained and leads him/her to pursue his/her cognitive efforts for acquisitions /rehabilitations. Accordingly, a main challenge is to understand these mechanisms in order to draw up supports for the curiosity-driven learning, and then to embed them into (re)educational technologies. To this end, two-ways of investigations are carried out in real-life setting (school, home, work place etc): 1) the design of curiosity-driven interactive systems for learning and their effectiveness study ; and 2) the automated personnalization of learning programs through new algorithms maximizing learning progress in ITS.
4 Application domains
Neuroscience, Developmental Psychology and Cognitive Sciences The computational modelling of life-long learning and development mechanisms achieved in the team centrally targets to contribute to our understanding of the processes of sensorimotor, cognitive and social development in humans. In particular, it provides a methodological basis to analyze the dynamics of the interaction across learning and inference processes, embodiment and the social environment, allowing to formalize precise hypotheses and later on test them in experimental paradigms with animals and humans. A paradigmatic example of this activity is the Neurocuriosity project achieved in collaboration with the cognitive neuroscience lab of Jacqueline Gottlieb, where theoretical models of the mechanisms of information seeking, active learning and spontaneous exploration have been developed in coordination with experimental evidence and investigation, see. Another example is the study of the role of curiosity in learning in the elderly, with a view to assessing its positive value against the cognitive aging as a protective ingredient (i.e, Industrial project with Onepoint and joint project with M. Fernendes from the Cognitive neursocience Lab of the University of Waterloo).
Personal and lifelong learning assistive agents Many indicators show that the arrival of personal assistive agents in everyday life, ranging from digital assistants to robots, will be a major fact of the 21st century. These agents will range from purely entertainment or educative applications to social companions that many argue will be of crucial help in our society. Yet, to realize this vision, important obstacles need to be overcome: these agents will have to evolve in unpredictable environments and learn new skills in a lifelong manner while interacting with non-engineer humans, which is out of reach of current technology. In this context, the refoundation of intelligent systems that developmental AI is exploring opens potentially novel horizons to solve these problems. In particular, this application domain requires advances in artificial intelligence that go beyond the current state-of-the-art in fields like deep learning. Currently these techniques require tremendous amounts of data in order to function properly, and they are severely limited in terms of incremental and transfer learning. One of our goals is to drastically reduce the amount of data required in order for this very potent field to work when humans are in-the-loop. We try to achieve this by making neural networks aware of their knowledge, i.e. we introduce the concept of uncertainty, and use it as part of intrinsically motivated multitask learning architectures, and combined with techniques of learning by imitation.
Educational technologies that foster curiosity-driven and personalized learning. Optimal teaching and efficient teaching/learning environments can be applied to aid teaching in schools aiming both at increase the achievement levels and the reduce time needed. From a practical perspective, improved models could be saving millions of hours of students' time (and effort) in learning. These models should also predict the achievement levels of students in order to influence teaching practices. The challenges of the school of the 21st century, and in particular to produce conditions for active learning that are personalized to the student's motivations, are challenges shared with other applied fields. Special education for children with special needs, such as learning disabilities, has long recognized the difficulty of personalizing contents and pedagogies due to the great variability between and within medical conditions. More remotely, but not so much, cognitive rehabilitative carers are facing the same challenges where today they propose standardized cognitive training or rehabilitation programs but for which the benefits are modest (some individuals respond to the programs, others respond little or not at all), as they are highly subject to inter- and intra-individual variability. The curiosity-driven technologies for learning and STIs could be a promising avenue to address these issues that are common to (mainstream and specialized)education and cognitive rehabilitation.
Automated discovery in science. Machine learning algorithms integrating intrinsically-motivated goal exploration processes (IMGEPs) with flexible modular representation learning are very promising directions to help human scientists discover novel structures in complex dynamical systems, in fields ranging from biology to physics. The automated discovery project lead by the FLOWERS team aims to boost the efficiency of these algorithms for enabling scientist to better understand the space of dynamics of bio-physical systems, that could include systems related to the design of new materials or new drugs with applications ranging from regenerative medicine to unraveling the chemical origins of life. As an example, Grizou et al. 119 recently showed how IMGEPs can be used to automate chemistry experiments addressing fundamental questions related to the origins of life (how oil droplets may self-organize into protocellular structures), leading to new insights about oil droplet chemistry. Such methods can be applied to a large range of complex systems in order to map the possible self-organized structures. The automated discovery project is intended to be interdisciplinary and to involve potentially non-expert end-users from a variety of domains. In this regard, we are currently collaborating with Poietis (a bio-printing company) and Bert Chan (an independant researcher in artificial life) to deploy our algorithms. To encourage the adoption of our algorithms by a wider community, we are also working on an interactive software which aims to provide tools to easily use the automated exploration algorithms (e.g. curiosity-driven) in various systems.
Human-Robot Collaboration. Robots play a vital role for industry and ensure the efficient and competitive production of a wide range of goods. They replace humans in many tasks which otherwise would be too difficult, too dangerous, or too expensive to perform. However, the new needs and desires of the society call for manufacturing system centered around personalized products and small series productions. Human-robot collaboration could widen the use of robot in this new situations if robots become cheaper, easier to program and safe to interact with. The most relevant systems for such applications would follow an expert worker and works with (some) autonomy, but being always under supervision of the human and acts based on its task models.
Environment perception in intelligent vehicles. When working in simulated traffic environments, elements of FLOWERS research can be applied to the autonomous acquisition of increasingly abstract representations of both traffic objects and traffic scenes. In particular, the object classes of vehicles and pedestrians are if interest when considering detection tasks in safety systems, as well as scene categories (”scene context”) that have a strong impact on the occurrence of these object classes. As already indicated by several investigations in the field, results from present-day simulation technology can be transferred to the real world with little impact on performance. Therefore, applications of FLOWERS research that is suitably verified by real-world benchmarks has direct applicability in safety-system products for intelligent vehicles.
5 Social and environmental responsibility
5.1 Footprint of research activities
AI is a field of research that currently requires a lot of computational resources, which is a challenge as these resources have an environmental cost. In the team we try to address this challenge in two ways:
- by working on developmental machine learning approaches that model how humans manage to learn open-ended and diverse repertoires of skills under severe limits of time, energy and compute: for example, curiosity-driven learning algorithms can be used to guide agent's exploration of their environment so that they learn a world model in a sample efficient manner, i.e. by minimizing the number of runs and computations they need to perform in the environment;
- by monitoring the number of CPU and GPU hours required to carry out our experiments. For instance, our work 11 used a total of 2.5 cpu years. More globally, our work uses large scale computational resources, such as the Jean Zay supercomputer platform, in which we use several hundred thousands hours of GPU and CPU each year.
5.2 Impact of research results
Our research activities are organized along two fundamental research axis (models of human learning and algorithms for developmental machine learning) and one application research axis (involving multiple domains of application, see the Application Domains section). This entails different dimensions of potential societal impact:
- Towards autonomous agents that can be shaped to human preferences and be explainable We work on reinforcement learning architectures where autonomous agents interact with a social partner to explore a large set of possible interactions and learn to master them, using language as a key communication medium. As a result, our work contributes to facilitating human intervention in the learning process of agents (e.g. digital assistants, video games characters, robots), which we believe is a key step towards more explainable and safer autonomous agents.
- Reproducibility of research: By releasing the codes of our research papers, we believe that we help efforts in reproducible science and allow the wider community to build upon and extend our work in the future. In that spirit, we also provide clear explanations on the statistical testing methods when reporting the results.
- AI and personalized educational technologies that support inclusivity and diversity and reduce inequalities The Flowers team develops AI technologies aiming to personalize sequences of educationa activities in digital educational apps: this entails the central challenge of designing systems which can have equitable impact over a diversity of students and reduce inequalitie. Using models of curiosity-driven learning to design AI algorithms for such personalization, we have been working to enable them to be positively and equitably impactful across several dimensions of diversity: for young learners or for aging populations; for learners with low initial levels as well as for learners with high initial levels; for "normally" developping children and for children with developmental disorders; and for learners of different socio-cultural backgrounds (e.g. we could show in the KidLearn project that the system is equally impactful along these various kinds of diversities).
- Health: Bio-printing The Flowers team is studying the use of curiosity-driven exploraiton algorithm in the domain of automated discovery, enabling scientists in physics/chemistry/biology to efficiently explore and build maps of the possible structures of various complex systems. One particular domain of application we are studying is bio-printing, where a challenge consists in exploring and understanding the space of morphogenetic structures self-organized by bio-printed cell populations. This could facilitate the design and bio-printing of personalized skins or organoids for people that need transplants, and thus could have major impact on the health of people needing such transplants.
- Tools for human creativity and the arts Curiosity-driven exploration algorithms could also in principle be used as tools to help human users in creative activities ranging from writing stories to painting or musical creation, which are domains we aim to consider in the future, and thus this constitutes another societal and cultural domain where our research could have impact.
- Education to AI As artificial intelligence takes a greater role in human society, it is of foremost importance to empower individuals with understanding of these technologies. For this purpose, the Flowers lab has been actively involved in educational and popularization activities, in particular by designing educational robotics kits that form a motivating and tangible context to understand basic concepts in AI: these include the Inirobot kit (used by >30k primary school students in France (see) and the Poppy Education kit (see) now supported by the Poppy Station educational consortium (see)
- Health: optimization of intervention strategies during pandemic events Modelling the dynamics of epidemics helps proposing control strategies based on pharmaceutical and non-pharmaceutical interventions (contact limitation, lock down, vaccination, etc). Hand-designing such strategies is not trivial because of the number of possible interventions and the difficulty to predict long-term effects. This task can be cast as an optimization problem where state-of-the-art machine learning algorithms such as deep reinforcement learning, might bring significant value. However, the specificity of each domain – epidemic modelling or solving optimization problem – requires strong collaborations between researchers from different fields of expertise. Due to its fundamental multi-objective nature, the problem of optimizing intervention strategies can benefit from the goal-conditioned reinforcement learning algorithms we develop at Flowers. In this context, we have developped EpidemiOptim, a Python toolbox that facilitates collaborations between researchers in epidemiology and optimization (see).
6 Highlights of the year
- Autotelic AI: We designed a new research program towards human-like AI centered on the use of language and culture as cognitive tools in autotelic agents, i.e. agents that learn by generating and exploring their own goals. This program was described in a Nature Machine Intelligence paper 102, building upon our review of research on autotelic agents published in Journal of Artificial Intelligence Research 103. As steps in this direction, we published several papers about how language can be used to shape exploration (Neurips 47), to ask questions (ICML, 54) or how it shapes representations (ICLR, 48). We also began projects in interactive textual environments 172. We also completed work to scale up autotelic learning algorithm in robots (in JMLR, 116) and visual environments (in IEEE TCDS, 39).
- Educational technologies: The team designed innovative educational technologies to stimulate curiosity and meta-cognition in children by training their curious question asking skills, in collaboration with the evidenceB company. Experiments were made in primary schools, leading to positive results published in International Journal of Human Computer Studies 34, and described in a blog post. We further extended this work by using large language models (GPT-3) to automate key aspects of conversational agents (involving a collaboration with Microsoft Research Montreal). In another project, we conducted pilot experiments to test how the ZPDES algorithm 98 can personalize attention training exercises (collaboration with the OnePoint company). To measure impact, we designed and shared an open-source cognitive battery test 35. We also published a paper studying the use of this algorithm in an intelligent tutoring system used with children with ASD (in the maths domain) 41. Finally, the ToGather project started, aiming to study the impact of a web application fostering collaboration among people accompanying children with neurodevevelopmental disorders in schools (see).
- Assisted scientific discovery: We studied the use of exploration algorithms in the domain of continuous cellular automata (Lenia), leading us to discover self-organized structures with basic forms of agency and sensorimotor behaviour (see). We also implemented mass conservation in the Flow Lenia cellular automaton, facilitating self-organization of diverse dynamic structures 68. This involved a collaboration with B. Chan (Google Brain). We released the first version of an integrated software aiming to allow the scientific community to explore complex dynamical systems with curiosity-driven algorirthms (AutoDisc).
- Learning and self-organization of cultural conventions: We introduced an approach enabling agents to learn a communication system without being able to share rewards in the architect-builder problem 53 (see also). Other works studied how sensorimotor agents can self-organize a shared graphical language 137, coordinate self-generation of goals 66, or learn socio-cognitive skills in interaction with social peers 135.
- Ecological AI: We developed an ecological research perspective on AI, highlighting the interactions between environmental, adaptive, multi-agent and cultural dynamics in sculpting intelligence. This led to the proposition of a detailled conceptual framework for studying these interactions 60, as well as computational experiments simulating the evolution of plasticity in variable environments (GECCO, 55) and the role of the topology of social structures in guiding innovation (67, under review at ICLR). This new research perspective is leading to several international and national collaborations: with Ida Momennejad from Microsoft Research (USA), with Marti Sanchez-Fibla and Ricard Solé from the University Pompeu Fabra (Spain) and with Francesco d'Errico from the University of Bordeaux (France).
- Scientific events co-organization: SMILES ICDL workshop (C. Moulin-Frier), Inria workshop on archaelogy and AI (C. Moulin-Frier and PY. Oudeyer), Language and Reinforcement Learning workshop at the NeurIPS conference in New Orleans, 2022 (L. Teodorescu, T. Karch and C. Colas), Dagstuhl developmental machine learning seminar (PY Oudeyer).
- Interaction with society: H. Sauzeon intervened at OPCST to inform french parliamentarians about the urgent need to develop research on technologies for people with disabilities that meet more to their real needs, such as those related to school & learning in children, or those related to work and vocational training (see). C. Moulin-Frier was interviewed for the Podcast "Désassemblons le numérique" (see). P. Germon, C. Romac and R. Portelas co-designed an interactive web demo presenting deep reinforcement learning algorithms (see). D. Roy and PY Oudeyer published the second edition of the population science book "Robotique et Intelligence Artificielle" (see).
- International research visits: PY. Oudeyer was a research visitor at Microsoft Montreal (until june 2022), and R. Portelas and L. Teodorescu visited Microsoft Research Montreal for several months, leading to new projects and collaboration on language-guided Deep RL and automatic curriculum learning. M. Etcheverry visited M. Levin's lab at University of Tufts for several months to develop a new projects studying automatic exploration algorithms in biological systems.
- C. Colas obtained a postdoctoral Marie Curie fellowship enabling him to start a 2 year research visit to J. Tenenbaum's lab at MIT, to further develop autotelic learning research.
.
6.1 Awards
Cedric Colas was awarded the prize for the best PhD thesis in AI in France (AFIA, see, as well as was ranked 2nd for the ERCIM Cor-Baayen PhD prize (see).
6.2 Defenses
- Rémy Portelas defended hi PhD thesis entitled "Automatic Curriculum Learning for Developmental Machine Learners", 61 (see also video).
- Alexander Ten defended his PhD thesis entitled "The Role of Progress-Based Intrinsic Motivation in Learning : Evidence from Human Behavior and Future Directions", 62 (see also video).
- Clément Moulin-Frier defended his HDR (Habilitation à Diriger des Recherche) on December 7, 2022. The thesis document is on HAL 60.
7 New software and platforms
7.1 New software
7.1.1 grimgep
-
Name:
GRIMGEP: Learning Progress for Robust Goal Sampling in Visual Deep Reinforcement Learning
-
Keywords:
Machine learning, Reinforcement learning, Artificial intelligence, Exploration, Intrinsic motivations, Git, Deep learning
-
Functional Description:
Source code for the GRIMGEP paper (https://arxiv.org/abs/2008.04388) Contains: - Implementation of the GRIMGEP framework on top of three different underlying imgeps (Skew-fit, CountBased, OnlineRIG). - image-based 2D environment (PlaygroundRGB)
- URL:
-
Contact:
Grgur Kovac
7.1.2 SocialAI
-
Name:
SocialAI: Benchmarking Socio-Cognitive Abilities in Deep Reinforcement Learning Agents
-
Keywords:
Artificial intelligence, Deep learning, Reinforcement learning
-
Functional Description:
Source code for the paper https://arxiv.org/abs/2107.00956.
A suite of environments for testing socio-cognitive abilities of RL agents. Simple RL baselines.
- URL:
-
Contact:
Grgur Kovac
7.1.3 AutoDisc
-
Keyword:
Complex Systems
-
Functional Description:
AutoDisc is a software built for automated scientific discoveries in complex systems (e.g. self-organizing systems). It can be used as a tool to experiment automated discovery of various systems using exploration algorithms (e.g. curiosity-driven). Our software is fully Open Source and allows user to add their own systems, exploration algorithms or visualization methods.
- URL:
-
Contact:
Clément Romac
7.1.4 Kids Ask
-
Keywords:
Human Computer Interaction, Cognitive sciences
-
Functional Description:
Kids Ask is a web-based educational platform that involves an interaction between a child and a conversational agent. The platform is designed to teach children how to generate curiosity-based questions and use them in their learning in order to gain new knowledge in an autonomous way.
-
News of the Year:
The kids Ask platform was used during two experiments with two different French primary schools, with a total of 53 participants that used the different functions of it.
- URL:
-
Contact:
Rania Abdelghani
7.1.5 ToGather
-
Keywords:
Education, Handicap, Environment perception
-
Scientific Description:
With participatory design methods, we have designed an interactive website application for educational purposes. This application aims to provide interactive services with continuously updated content for the stakeholders of school inclusion of children with specific educational needs.
-
Functional Description:
Website gathering information on middle school students with neurodevelopmental disorders. Authentication is required to access the site's content. Each user can only access the student file(s) of the young person(s) they are accompanying. A student file contains 6 tabs, in which each type of user can add, edit or delete information: 1. Profile: to quickly get to know the student 2. Skills: evaluation at a given moment and evolution over time 3. Compendium of tips: includes psycho-educational tips 4. Meetings: manager and reports 5. News: share information over time 6. Contacts: contact information for stakeholders The student only has the right to view information about him/her.
- Publication:
-
Contact:
Cécile Mazon
-
Participants:
Isabeau Saint-Supery, Cécile Mazon, Eric Meyer, Hélène Sauzéon
7.1.6 mc_training
-
Name:
Platform for metacognitive training
-
Keywords:
Human Computer Interaction, Education
-
Functional Description:
This is a web platform for children between 9 and 11 years old, designed to help children practice 4 metacognitive skills that are thought to be involved in curiosity-driven learning: - the ability to identify uncertainties - the ability to generate informed hypotheses - the ability to ask questions - the ability to evaluate the value of a preconceived inference.
Children work on a reading-comprehension tasks and, for each of these skills, the platform offers help through a "conversation" with conversational agents that give instructions to perform the task, with respect to every skill, and can give suggestions if the child asks for it.
-
Contact:
Rania Abdelghani
7.1.7 Evolution of adaptation mechanisms in complex environments
-
Name:
Plasticity and evolvability under environmental variability: the joint role of fitness-based selection and niche-limited competition
-
Keywords:
Evolution, Ecology, Dynamic adaptation
-
Functional Description:
This is the code accompannying our paper Plasticity and evolvability under environmental variability: the joint role of fitness-based selection and niche-limited competition" which is to be presented at the Gecco 2022 conference.
In this work we have studied the evolution of a population of agents in a world where the fitness landscape changes with generations based on climate function and a latitudinal model that divides the world in different niches. We have implemented different selection mechanisms (fitness-based selection and niche-limited competition).
The world is divided into niches that correspond to different latitudes and whose state evolves based on a common climate function.
We model the plasticity of an individual using tolerance curves originally developed in ecology. Plasticity curves have the form of a Gaussian the capture the benefits and costs of plasticity when comparing a specialist (left) with a generalist (right) agent.
The repo contains the following main elements :
folder source contains the main functionality for running a simulation scripts/run/reproduce_gecco.py can be used to rerun all simulations in the paper scripts/evaluate contains scripts for reproducing figures. reproduce_figures.py will produce all figures (provided you have already run scripts/run/reproduce_gecco.py to generate the data) folder projects contains data generated from running a simulation How to run To install all package dependencies you can create a conda environment as:
conda env create -f environment.yml
All script executions need to be run from folder source. Once there, you can use simulate.py, the main interface of the codebase to run a simulation, For example:
python simulate.py –project test_stable –env_type stable –num_gens 300 –capacity 1000 –num_niches 10 –trials 10 –selection_type NF –climate_mean_init 2
will run a simulation with an environment with a climate function whose state is constantly 2 consisting of 100 niches for 300 generations and 10 independent trials. The maximum population size will be 1000*2 and selection will be fitness-based (higher fitness means higher chances of reproduction) and niche limited (individuals reproduce independently in each niche and compete only within a niche),
You can also take a look at scripts/run/reproduce_gecco.py to see which flags were used for the simulations presented in the paper.
Running all simulations requires some days. You can instead download the data produced by running scripts/run/reproduce_gecco.py from this google folder and unzip them under the projects directory.
- URL:
-
Contact:
Eleni Nisioti
7.1.8 SAPIENS
-
Name:
SAPIENS: Structuring multi-Agent toPology for Innovation through ExperieNce Sharing
-
Keywords:
Reinforcement learning, Multi-agent
-
Functional Description:
SAPIENS is a reinforcement learning algorithm where multiple off-policy agents solve the same task in parallel and exchange experiences on the go. The group is characterized by its topology, a graph that determines who communicates with whom.
All agents are DQNs and exchange experiences have the form of transitions from their replay buffers.
Using SAPIENS we can define groups of agents that are connected with others based on a a) fully-connected topology b) small-world topology c) ring topology or d) dynamic topology.
Install required packages You can install all required python packages by creating a new conda environment containing the packages in environment.yml:
conda env create -f environment.yml
And then activating the environment:
conda activate sapiens
Example usages Under notebooks there is a Jupyter notebook that will guide you through setting up simulations with a fully-connected and a dynamic social network structure for solving Wordcraft tasks. It also explains how you can access visualizations of the metrics produced during th$
Reproducing the paper results Scripts under the scripts directory are useful for reproducing results and figures appearing in the paper.
With scripts/reproduce_runs.py you can run all simulations presented in the paper from scratch.
This file is useful for looking at how the experiments were configured but better avoid running it: simulations will run locally and sequentially and will take months to complete.
Instead, you can access the data files output by simulations on this online repo.
Download this zip file and uncompress it under the projects directory. This should create a projects/paper_done sub-directory.
You can now reproduce all visualization presented in the paper. Run:
python scripts/reproduce_visuals.py
This will save some general plots under visuals, while project-specific plots are saved under the corresponding project in projects/paper_done
- URL:
-
Contact:
Eleni Nisioti
7.1.9 architect-builder-abig
-
Name:
Architect-Builder Iterated Guiding
-
Keyword:
Artificial intelligence
-
Functional Description:
Codebase for the paper Learning to guide and to be guided in the Architect-Builder Problem
ABIG stands for Architect-Builder Iterated Guiding and is an algorithmic solution to the Architect-Builder Problem. The algorithm leverages a learned model of the builder to guide it while the builder uses self-imitation learning to reinforce its guided behavior.
- URL:
-
Contact:
Tristan Karch
7.1.10 EAGER
-
Name:
Exploit question-Answering Grounding for effective Exploration in language-conditioned Reinforcement learning
-
Keywords:
Reinforcement learning, Language, Question Generation Question Answering, Reward shaping
-
Functional Description:
A novel QG/QA framework for RL called EAGER In EAGER, an agent reuses the initial language goal sentence to generate a set of questions (QG): each of these self-generated questions defines an auxiliary objective. Here, generating a question consists in masking a word of the initial language goal. Then the agent tries to answer these questions (guess the missing word) only by observing its trajectory so far. When it manages to answer a question correctly (QA) it obtains an intrinsic reward proportional to its confidence in the answer. The QA module is trained using a set of successful example trajectories. If the agent follows a path too different from correct ones at some point in its trajectory, the QA module will not answer the question correctly, resulting in zero intrinsic reward. The sum of all the intrinsic rewards measures the quality of a trajectory in relation to the given goal. In other words, maximizing this intrinsic reward incentivizes the agent to produce behaviour that unambiguously explains various aspects of the given goal.
- URL:
-
Contact:
Thomas Carta
7.1.11 IMGC-MARL
-
Name:
Intrinsically Motivated Goal-Conditioned Reinforcement Learning in Multi-Agent Environments
-
Keywords:
Reinforcement learning, Multi-agent, Curiosity
-
Functional Description:
This repo contains the code base of the paper Intrinsically Motivated Goal-Conditioned Reinforcement Learning in Multi-Agent Environments.
In this work, we have studied the importance of the alignment of goals in the training of instrinsically motivated agents in the multi agent goal conditioned RL case. We also proposed a simple algorithm called goal coordination game which allows such agent to learn, in a completely decentralized/selfish way, to communicate in order to align their goal.
The repository contains the code to reproduce the results of the paper. Which includes a custom RL environment ( using SimplePlayground "game engine"), model used (architecture + hyperparameters) and custom training (mostly based on RLlib ) to train both the model and the communication. We also provide the scripts for the training of every condition we test and notebook to study the results.
- URL:
-
Contact:
Gautier Hamon
7.1.12 Flow-Lenia
-
Name:
Flow Lenia: Mass conservation for the study of virtual creatures in continuous cellular automata
-
Keywords:
Cellular automaton, Self-organization
-
Functional Description:
This repo contains the code to run the Flow Lenia system which is a continuous parametrized cellular automaton with mass conservation. This work extends the classic Lenia system with mass conservation and allows to implement new feature like local parameter, environment components etc
Several declination of the system (1 or several channels etc ) are available
Please refer to the associated paper for the details of the system
Implemented in JAX
- URL:
-
Contact:
Gautier Hamon
7.1.13 Kidlearn: money game application
-
Functional Description:
The games is instantiated in a browser environment where students are proposed exercises in the form of money/token games (see Figure 1). For an exercise type, one object is presented with a given tagged price and the learner has to choose which combination of bank notes, coins or abstract tokens need to be taken from the wallet to buy the object, with various constraints depending on exercises parameters. The games have been developed using web technologies, HTML5, javascript and Django.
Figure 1: Four principal regions are defined in the graphical interface. The first is the wallet location where users can pick and drag the money items and drop them on the repository location to compose the correct price. The object and the price are present in the object location. Four different types of exercises exist: M : customer/one object, R : merchant/one object, MM : customer/two objects, RM : merchant/two objects. - URL:
-
Contact:
Benjamin Clement
7.1.14 cognitive-testbattery
-
Name:
Cognitive test battery of human attention and memory
-
Keywords:
Open Access, Cognitive sciences
-
Scientific Description:
Cognitive test batteries are widely used in diverse research fields, such as cognitive training, cognitive disorder assessment, or brain mechanism understanding. Although they need flexibility according to the objectives of their usage, most of the test batteries are not be available as open-source software and not be tuned by researchers in detail. The present study introduces an open-source cognitive test battery to assess attention and memory, using a javascript library, p5.js. Because of the ubiquitous nature of dynamic attention in our daily lives, it is crucial to have tools for its assessment or training. For that purpose, our test battery includes seven cognitive tasks (multiple-objects tracking, enumeration, go/no-go, load-induced blindness, task-switching, working memory, and memorability), common in cognitive science literature. By using the test battery, we conducted an online experiment to collect the benchmark data. Results conducted on two separate days showed the high cross-day reliability. Specifically, the task performance did not largely change with the different days. Besides, our test battery captures diverse individual differences and can evaluate them based on the cognitive factors extracted from latent factor analysis. Since we share our source code as open-source software, users can expand and manipulate experimental conditions flexibly. Our test battery is also flexible in terms of the experimental environment, i.e., it is possible to experiment either online or in a laboratory environment.
-
Functional Description:
The evaluation battery consists of 6 cognitive activities (serious games: multi-object tracking, enumeration, go/no-go, Corsi, load-induced blindness, taskswitching, memorability). Easily deployable as a web application, it can be re-used and modified for new experiments. The tool is documented in order to facilitate the deployment and the analysis of results.
- URL:
- Publication:
-
Contact:
Maxime Adolphe
-
Participants:
Pierre-yves Oudeyer, Hélène Sauzéon, Masataka Sawayama, Maxime Adolphe
7.2 New platforms
7.2.1 ToGather application
Participants: Cécile Mazon, Hélène Sauzéon, Eric Meyer, Isabeau Saint-Supery.
-
Name:
Application for Specialized education
-
Keywords:
Parent-professional relationships; user-centered design; school inclusion; autism spectrum disorder; ecosystemic approach
-
Participants:
Isabeau Saint-supery, Cécile Mazon, Hélène Sauzéon, Agilonaute
-
Scientific Description:
With participatory design methods, we have designed an interactive website application for educational purposes. This application aims to provide interactive services with continuously updated content for the stakeholders of school inclusion of children with specific educational needs. Especially, the services provide: 1) the student's profile with strengths and weaknesses; 2) an evaluation and monitoring over time of the student's repertoire of acquired, emerging or targeted skills; 3) a shared notebook of effective psycho-educational solutions for the student ; 4) a shared messaging system for exchanging "news" about the student and his/her family and, 5) a meeting manager allowing updates of evaluations (student progress). This application is currently assessed with a field study. Then, it will be transferred to the Academy of Nouvelle-Aquitaine-Bordeaux of the National Education Ministery.
-
URL:
The website is not online yet.
- Publication:
8 New results
We here present new results of the Flowers team, within the domain of developmental artificial intelligence which includes the following research dimensions:
- Designing and studying computational models of development of sensorimotor, cognitive and cultural structures, both at the level of individuals and at the level of populations;
- Designing and studying machines that learn like children (autonomous, autotelic, open-ended), and can self-organize in groups to achieve forms of cultural evolution;
- Designing and studying machines that help humans learn, explore and develop, across all age ranges, e.g. targeting educational technologies or assisted discovery in the sciences.
8.1 Models of Curiosity-Driven Learning in Humans
8.1.1 Testing the Learning Progress Hypothesis in Curiosity-Driven exploration in Human Adults
Participants: Pierre-Yves Oudeyer [correspondant], Alexandr Ten.
Alexandr Ten defended his PhD thesis 62, on this topic, which main results were published in 32.
Summary of the PhD thesis:
Intrinsic motivation – the desire to do things for their inherent joy and pleasure – has received its first share of scientific attention over 70 years ago, ever since we saw monkeys solving puzzles for free. Since then, research on intrinsic motivation has been steadily gaining momentum. We have come to understand, in the context of learning and discovery, that intrinsic motivation (namely, intrinsically motivated information-seeking) is foundational for the biological and technological success of our species. But where does intrinsic motivation to learn and seek information come from? Today, with the thriving synergy be- tween perpetually advancing fields of psychology, neuroscience, and computer science, we are well positioned to investigate this question.
The Learning Progress Hypothesis (LPH) proposes that humans are motivated by feelings of and/or beliefs about progress in knowledge (including progress in competence). In artificial learners, progress-based intrinsic motivation enables autonomous exploration of the environment (including the agent’s own body), resulting in better performance, more efficient learning, and richer skill sets. Due to similar computational challenges facing artificial and biological learners, researchers have proposed that progress-based intrinsic motivation might have evolved in humans to help us transition from babies with few skills and little knowledge to knowledgeable grownups capable of performing many sophisticated tasks. The Learning Progress Hypothesis (LPH) is attractive, not only because it is consistent with several studies of human curiosity, but also because it resonates with existing theories on metacognitive self-regulation in learning. However, the LPH has not been extensively studied using behavioral experimentation.
This thesis provides an empirical examination of the LPH. It introduces a novel experimental paradigm where participants explore multiple learning activities, some easy, others difficult. The activities involve guessing the binary category of randomly presented stimuli. To let their intrinsic motivation shine, no material incentives encouraging specific behaviors or strategies were provided – we simply observed which activities people engaged in and how their knowledge about these activities unfolded over time. The thesis presents statistical analyses and a computational model that support the LPH. This thesis also suggests ideas for future investigations into progress-based motivation. These ideas are inspired by a pilot study in which we asked participants to practice a naturalistic sensorimotor skill (a video game) over the course of 3 sessions spanning 5 days. At the end of each session, participants reported their subjective judgments of past and future progress, as well as their evolving beliefs about their perceived competence, self-efficacy beliefs, and intrinsic motivation. In support of the LPH, participants’ subjective judgments correlated with the objective improvement. However, contrary to the LPH’s prediction, objective and subjective progress measures did not show reliable relationships with verbal and behavioral measures of intrinsic motivation. Instead, progress measures were in strong relationships with beliefs about task learnability, which in turn predicted intrinsic motivation. Based on these findings, we suggest a novel mechanism in which learning progress interacts with intrinsic motivation via subjective beliefs.
The thesis concludes with an extended discussion of findings, and of some limitations of experiments. It also proposes promising future steps. In summary, the behavioral paradigms introduced in this thesis afford be reused to not only replicate the results, but also to advance the scientific research of intrinsically motivated information-seeking.
8.2 Open-ended Autotelic Agents, Intrinsic Motivation and Language
Participants: Pierre-Yves Oudeyer [correspondant], Olivier Sigaud, Cédric Colas, Adrien Laversanne-Finot, Rémy Portelas, Tristan Karch, Grgur Kovac, Laetitia Teodorescu.
8.2.1 A new research perspective: Autotelic agents with language and culture internalization
Participants: Cédric Colas [correspondant], Tristan Karch, Clément Moulin-frier, Pierre-Yves Oudeyer.
One of the fundamental goals of our team is to build autonomous agents able to grow open-ended repertoires of skills across their lives. As mentioned in previous section, a promising developmental approach recommends the design of intrinsically motivated agents that learn new skills by generating and pursuing their own goals—autotelic agents. But despite recent progress, existing algorithms still show serious limitations in terms of goal diversity, exploration, generalization, or skill composition. In a recent perspective paper 102 published in Nature Machine Intelligence, we call for the immersion of autotelic agents into rich socio-cultural worlds, an immensely important attribute of our environment that shapes human cognition but is mostly omitted in modern AI. Inspired by the seminal work of Vygotsky, we propose Vygotskian autotelic agents—agents able to internalize their interactions with others and turn them into cognitive tools (as illustrated in figure 2. We focus on language and show how its structure and informational content may support the development of new cognitive functions in artificial agents as it does in humans. We justify the approach by uncovering several examples of new artificial cognitive functions emerging from interactions between language and embodiment in recent works at the intersection of deep reinforcement learning and natural language processing. Looking forward, we highlight future opportunities and challenges for Vygotskian autotelic AI research, including the use of language models as cultural models supporting artificial cognitive development.
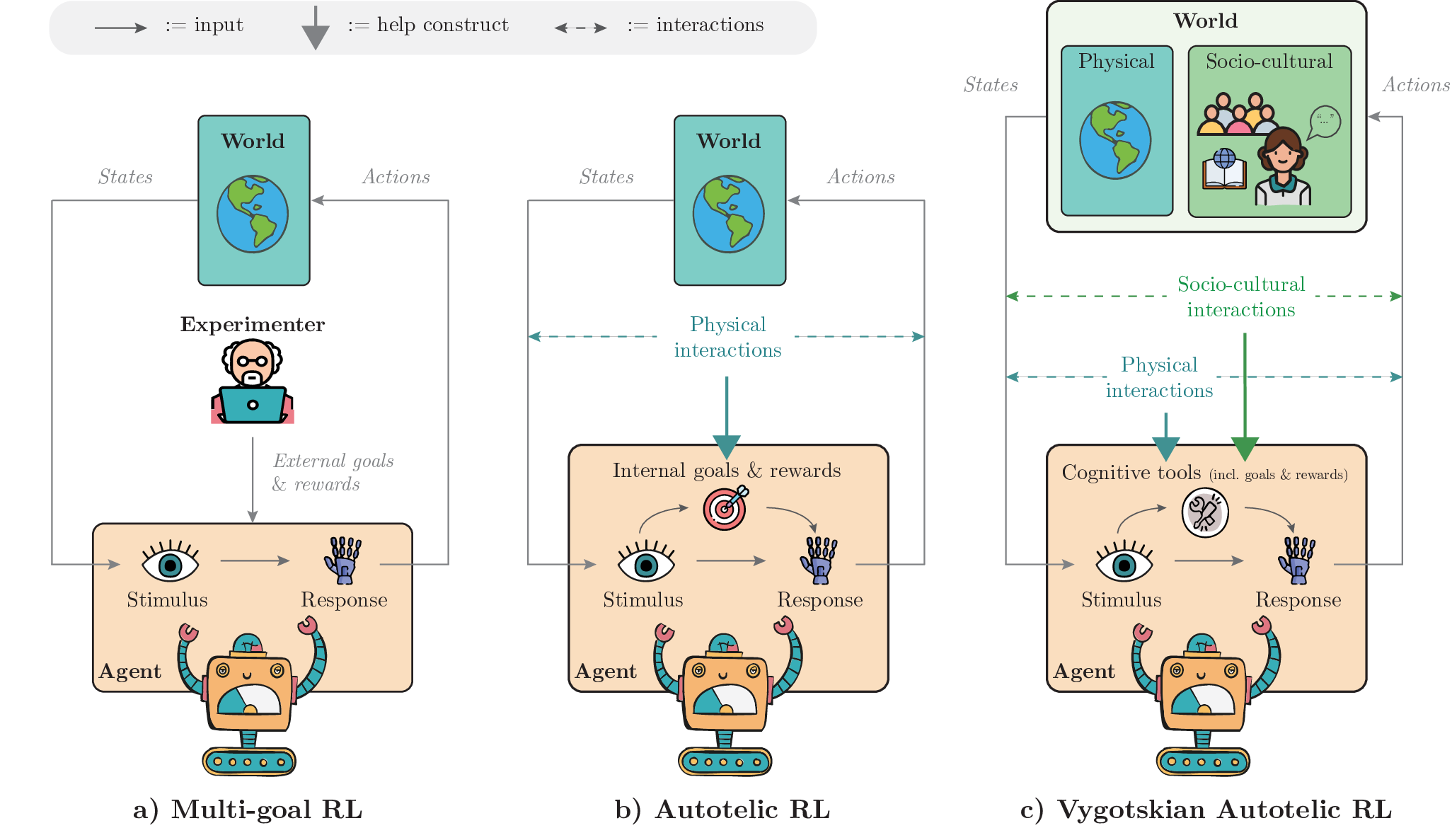
8.2.2 Autotelic agents in complex visuo-linguistic environments (ALFRED)
Participants: Laetitia Teodorescu [correspondant], Eric Yuan, Marc-Alexandre Côté, Pierre-Yves Oudeyer.
In the following subsections we describe projects and new results on language-augmented autotelic agents. Following long-standing work in the team on the concept of Intrinsically Motivated Goal Exploration Processes 117, the concept of the autotelic agent has been developed. Much like a child imagines play scenarios and creative goals to achieve, for the simple pleasure of doing novel and interesting things (build a castle, climb a tree), an autotelic agent is a goal-conditioned agent that imagines its own goals and tires to achieve them. By doing so it collects experience that allows it to master an open-ended repertoire of skills. This section describes work on language-conditioned autotelic agents (see 101 and also Section 8.2.1 for a definition of Vygotskian autotelic agents).
The aim of this project was to scale the autotelic agent framework to a richer setting consisting of visual observations of a simulated rich indoor environment, to showcase the abilities of an agent to learn to explore while interacting with a social partner in natural language. For this purpose we placed ourselves inside the ALFRED 168 indoor simulator. ALFRED is a framework for instruction-following agents (closely tied to research in robotics). It consists in a virtual environment containing several rooms with medium visual complexity (many objects, randomized textures and lighting) containing different interactable objects (ingredients, furniture, appliances, etc). The environment also features a simplified action space (discrete movement and symbolic interactions such as open fridge, turn on oven, etc). ALFRED comes with a set of predefined tasks that form the associated benchmark, as well as with a dataset of planner-generated trajectories for each of the predefined tasks and annotations in a symbolic as well as natural language (the latter being generated by a set of Amazon Mechanical Turk workers).
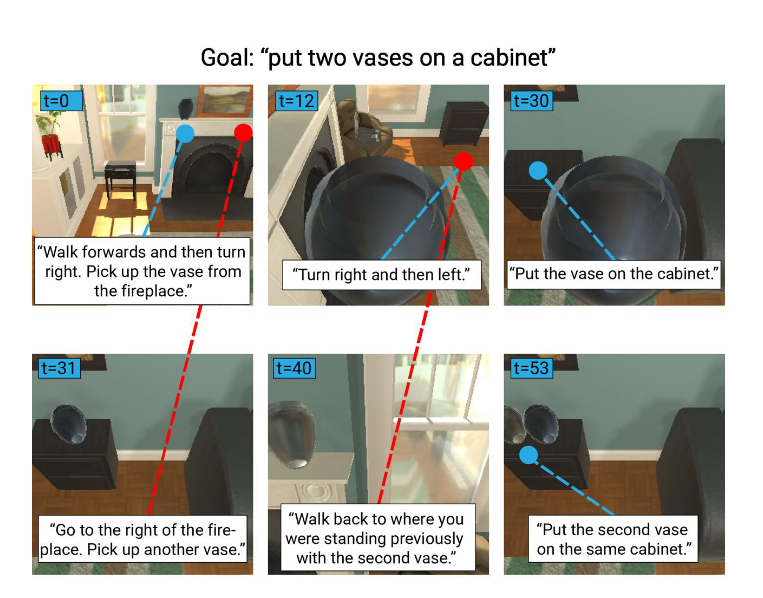
One of the difficulties in training language-based autotelic agents is language grounding 131; the ability of the agent to relate its linguistic instruction to its current observation. Such language grounding is actually needed in 2 modules: first to train a reward function that is able to take as input one or several visual observations as well as the language goal and predict a scalar reward quantifying how much the observations fit the goal (a multimodal similarity function reminiscent of CLIP 161). This (instruction-conditioned) reward function is then used to train the agent within the goal-conditioned reinforcement learning (RL) paradigm. The second difficulty concerns the multitask nature of autotelic agents. The most efficient way to train goal-conditioned agents is to use hindsight experience replay (HER) 81, allowing to leverage previous experience of the agent setting itself a goal that it did not manage to achieve, re-labelling it with the achieved goal instead. For HER to work, we need a so-called relabeller, a function that takes the trajectory as input and outputs one or several sentences describing the goals the agent actually managed to achieve.
In this project, the aim was to sidestep the reward function grounding by leveraging the dataset provided by the ALFRED framework to pre-train the agent with behavioral cloning (BC), thus sidestepping the problem of learning a reward function. We also leverage the dataset to pre-train a video-based captioner that we can use as our relabeler function for hindsight relabelling. We use as a base the code of the Episodic Transformer 159 paper, using a Transformer-based BC agent trained on the ALFRED dataset. For the captioner we used a transformer-based seq2seq model similar to the ones used in Natural Language Translation, taking as input the sequence of frames in an episode and outputting the corresponding language that describes what happened in the episode. The natural-language (NL) based captioner, trained on a total of 8k trajectories with an average of 3 NL annotations, was prone to hallucinations and repetitions (a common pitfall for language models trained on a small amount of data). The most common failure case was the captioner inventing navigation descriptions that did not exist; the captioner based on symbolic language was better behaved. However, these captioners only ever described goals that were part of the ALFRED training data. The Episodic Transformer agent was similarly trained on the same data, and thus was never able to use direct interaction with the environment to discover novel skills, thus getting stuck at the same success rate of about 30 percent on the benchmark tasks. Furthermore, we identified that navigation was the most important bottleneck in this environment, with a total success rate of about 60%; and since navigation is necessary to get to any object that the agent needs to navigate to this greatly impacts the ability of the agent to complete especially long tasks.
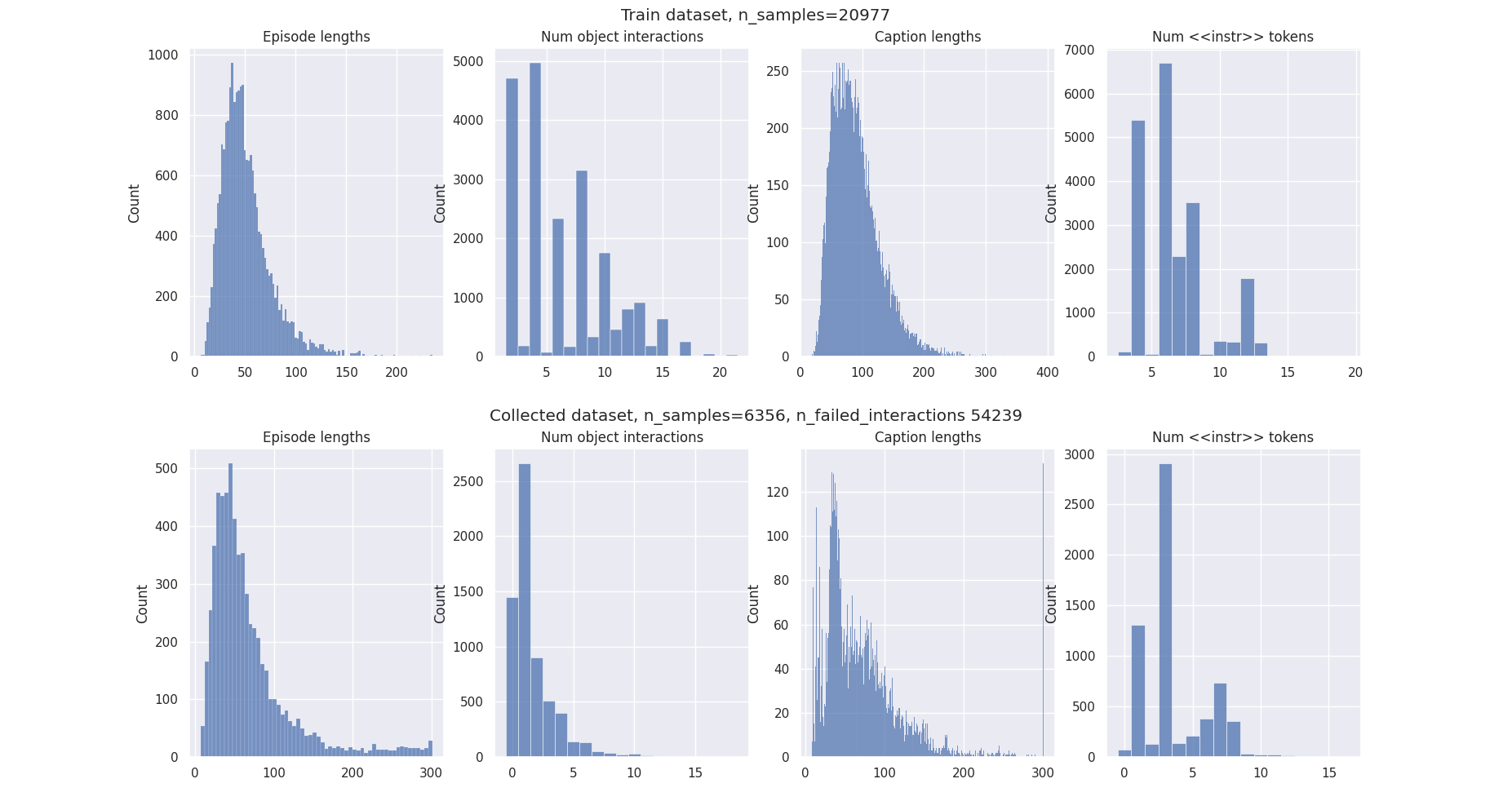
From these experiments we concluded that for language-based autotelic agents to learn more skills than they currently master, they need to leverage a source of linguistic knowledge that is broader than their prior knowledge, allowing them to correctly identify, label and integrate novel things they observe in their environment (see section 8.2.4 for further developments on leveraging external sources of linguistic knowledge).
8.2.3 Autotelic agents in complex textual environments (ScienceWorld)
Participants: Laetitia Teodorescu [correspondant], Eric Yuan, Marc-Alexandre Côté, Pierre-Yves Oudeyer.
To sidestep the harder part of language grounding and focus more specifically on exploration dynamics, we have performed series of experiments in text environments for language-based autotelic agents. Text worlds 105 are RL environments that feature textual observation and action spaces, and have first been proposed as a challenging framework for RL agents because of the large, combinatorial action space and necessity for language understanding. Additionally, they are an excellent framework to study language-instructed agents because of the ease of defining a reward function and relabelling function, thus sidestepping the problem of learning a multimodal reward function or a captioner.
In this project we are interested in studying different exploration drivers in the context of an autotelic agent's learning algorithm. We define 3 ways in which an autotelic agent can discover novel environment interactions it can use as goals:
- Failed goal achievement leading to relabelling of novel goals;
- Further random exploration after a goal has been achieved;
- Goal composition, in particular chaining different goals.
The latter two exploration drivers are the ones we study in particular in this project, and are inspired by the Go-Explore 112 framework. Please see Figure 5 for an outline of the architecture of the agent.
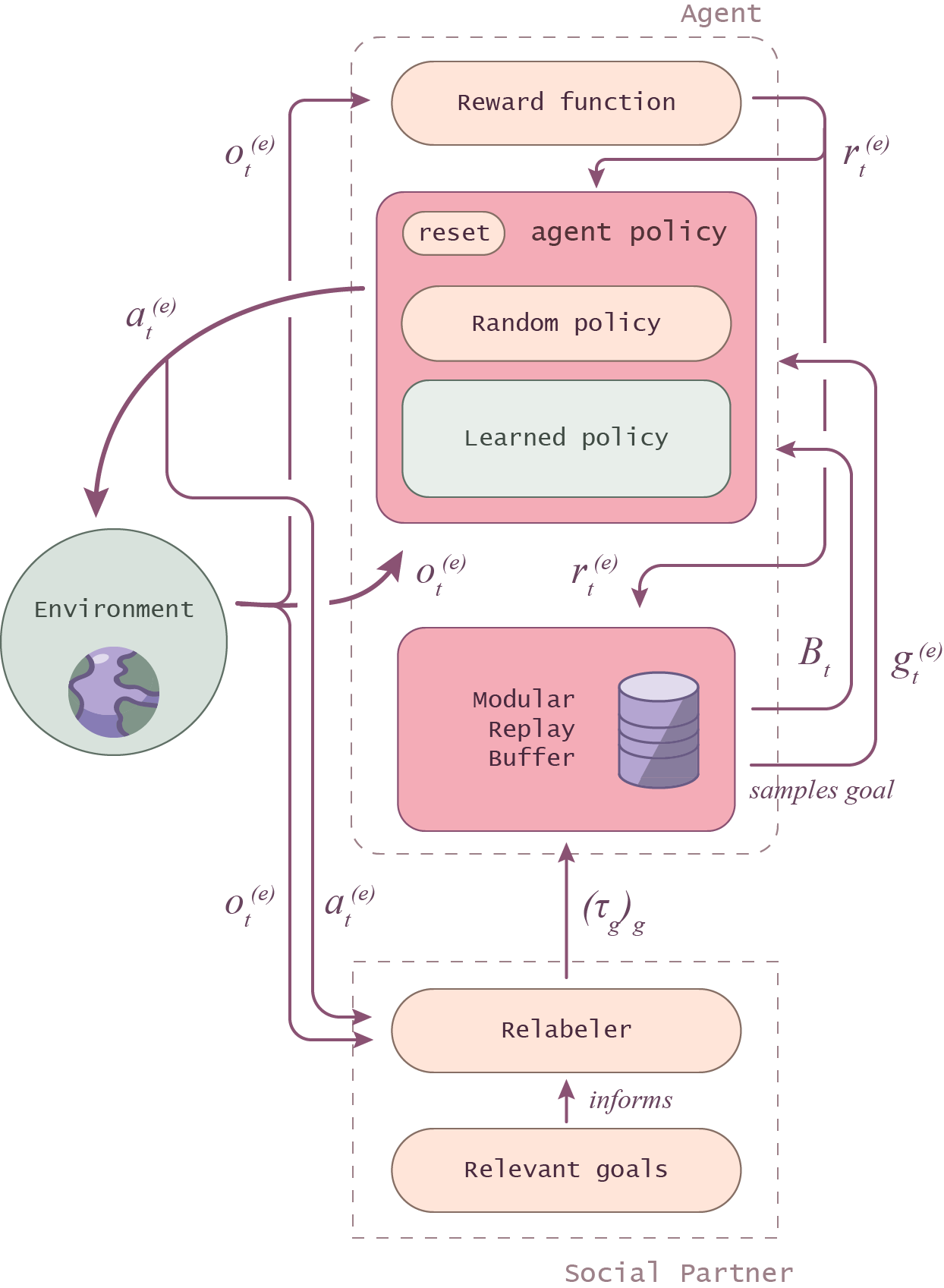
Overview of the language-based autotelic agent architecture used for the ScienceWorld experiments. At each time step the agent observes a goal g(e)g^{(e)} and an observation ot(e)o_t^{(e)} and produces an action at(e)a_t^{(e)}. The reward is given by a goal-conditioned reward function and is used to decide whether to terminate the current episode. If the episode is terminated, if the agent is under the seq_compose condition, with probability 0.5 the agent samples another goal. If the agent is under the do_then_explore condition then 5 additional steps of exploration are produced with a random policy. Once the trajectory is over, it is relabelled by the relabeller based on the chosen relevant goals and added to the modular memory buffer. The buffer also receives the reward signal to update the online estimation of competence for the agent. A new episode then starts, and the memory buffer is used to produce a new goal for the agent. While the agent is interacting with the environment, the replay buffer samples minibatches of transitions and uses those to train the policy by minimizing the TD-error.
We place ourselves in the ScienceWorld 182 textual environment, a complex textual environment featuring a series of rooms in a house with many different objects. ScienceWorld implements simplified versions of thermodynamics, biology, newtonian mechanics, chemistry and electronics. The environment was designed with the aim of testing scientific knowledge of text agents in an interactive context. We leverage this environment with complex interactions to define a goal tree for a language-conditioned RL agent (see Figure) with several layers of depth, featuring nested goals. For the agent we build upon the DRRN 124 agent of the original ScienceWorld paper. This agent is a TD-error based agent with a Huber loss. In this work, goals are defined as a part of the textual observation space, and are achieved if the text describing them is present in the environment description (string matching). Only the goals in this goal tree are allowed to be relabeled. We study the impact of the following exploration drivers on final performance over all goals:
- do_then_explore: After the provided goal has been achieved or the maximum allowed number of steps have been reached, we perform an additional 5 steps of random exploration.
- seq_compose: After the provided goal has been achieved or the maximum allowed number of steps have been reached, with probability 0.5 we choose another goal and pursue this one.
We demonstrate that an agent combining do_then_explore with seq_compose and that uses the current estimated performance to bias sampling of the first goal towards the easy ones and sampling of the hard goal towards the hard ones achieved the best final performance and the lowest variance off all considered variants (See Table). This underlines the importance of correctly following the goal curriculum in this setting, targeting the easier goals allowing the agent to first find and then master easier goals first and building upon these goals to achieve the hardest ones.
This is ongoing work, and is done in collaboration with Marc-Alexandre Côté and Eric Yuan from Microsoft Research Montreal.
8.2.4 Autotelic Agents Augmented with Large Language Models
Participants: Cédric Colas [correspondant], Laetitia Teodorescu, Eric Yuan, Marc-Alexandre Côté, Pierre-Yves Oudeyer.
In this project, we take a step towards truly open-ended language-based autotelic agents by leveraging GPT3 90, a Large Language Model (LLM) demonstrating impressive language understanding capabilities. For an autotelic agent to be truly open-ended, it needs to be able to:
- Generate its own goals creatively (Goal generator)
- For an arbitrary goal, decide whether a given trajectory achieved the goal or not (Reward function)
- For a given trajectory, give a list of relevant achieved goals (Relabeller or Social Partner)
In this project, we also place ourselves in a textual environment. CookingWorld has been proposed as part of the First TextWorld Problems competition on text agents, and features a house with ingredients that can be cooked to achieve a recipe. We leverage the textual nature of the trajectories of agents in this environment by using GPT3, with different prompts, as our goal generator, reward function, and relabeller. More precisely:
- Goal generator: we present GPT3 with a prompt composed of a context explanation ("We are in this video game environment"), a list of previously seen rooms, a list of previously seen objects, a list of previously achieved goals, possibly a past trajectory example, and ask it to generate new goals;
- Reward function: given an arbitrary language goal and a trajectory including actions and observations, labelled with the steps index at which those actions and observations were issued, we ask GPT3 to decide whether a given goal is achieved or not in the trajectory, and if it is, at which time step. In this way, we only need to call the GPT3 based reward function once per episode (compared with once per step in a traditional RL setup), significantly speeding up our experiments.
- Relabeller: Once a trajectory is finished, we show GPT-3 the completed trajectory and ask it to provide a list of goals achieved in this trajectory. We extract these goals and we add the relabelled trajectories to the replay buffer.
As in the ScienceWorld project (8.2.3), we use a variant of the DRRN architecture for text worlds (cite). We tried several variants of the architecture with transformers instead of a recurrent architecture, and using a sentencebert (cite) pooling operation. We find nontrivial performance of this truly open-ended textual agent on a set of human-defined evaluation goals, as well as on the set of imagined and relabelled goals. Imagined and relabelled goals have also have a nontrivial stem distribution (see Figure). We also demonstrate the alignment of the GPT3 reward function with a priori human-defined reward functions: (true positive rate of 0.81, false positive fate of 0., the latter being excellent news for use in a reward function).
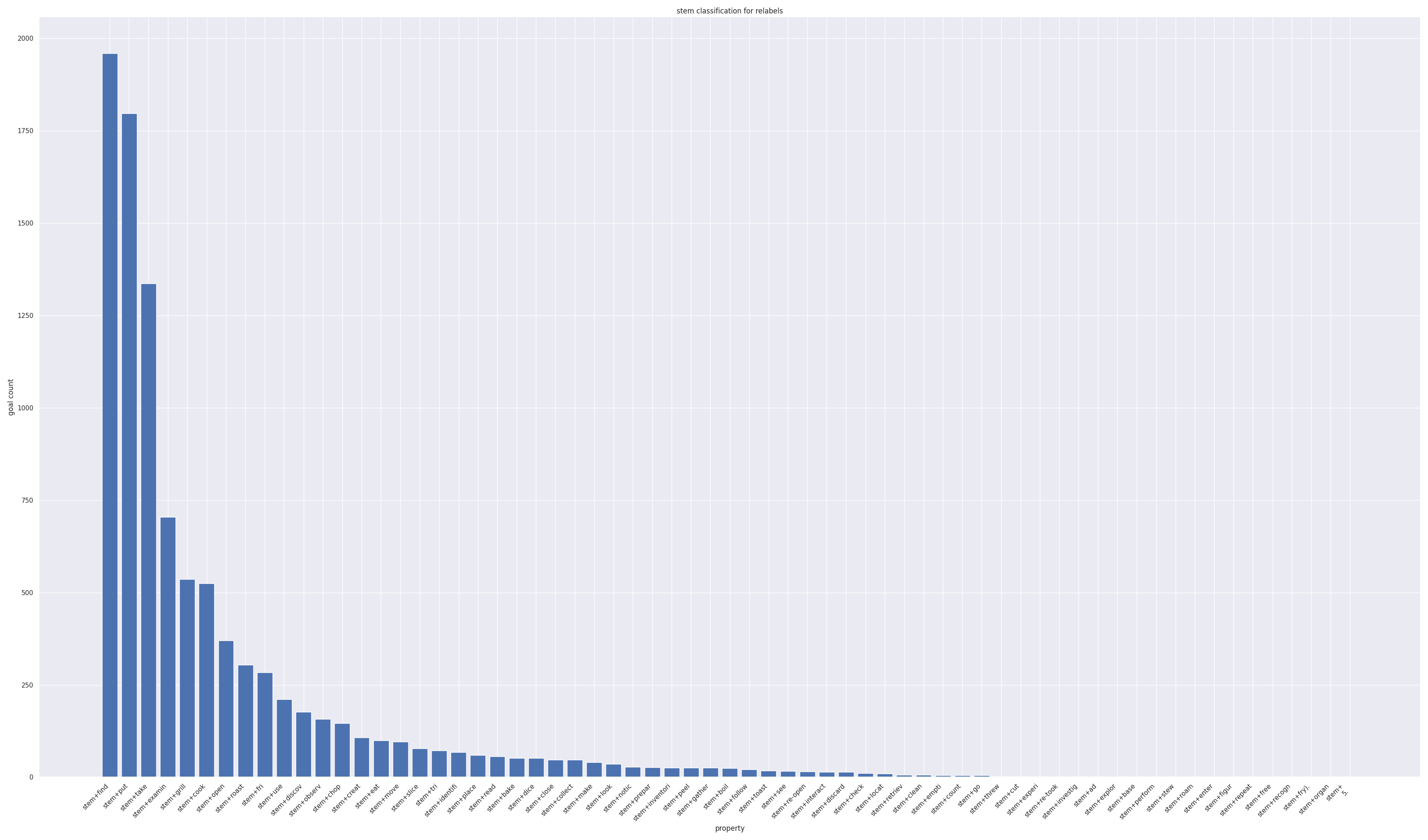
This is ongoing work, and is done in collaboration with Marc-Alexandre Côté and Eric Yuan from Microsoft Research Montreal.
8.2.5 EAGER: Asking and Answering Questions for Automatic Reward Shaping in Language-guided RL
Participants: Thomas Carta, Pierre-Yves Oudeyer, Olivier Sigaud [ISIR Sorbonne Université, Paris, France], Sylvain Lamprier [Univ Angers, LERIA].
Reinforcement learning (RL) in long horizon and sparse reward tasks is notoriously difficult and requires a lot of training steps. A standard solution to speed up the process is to leverage additional reward signals, shaping it to better guide the learning process. In the context of language-conditioned RL, the abstraction and generalisation properties of the language input provide opportunities for more efficient ways of shaping the reward. In this paper, we leverage this idea and propose an automated reward shaping method where the agent extracts auxiliary objectives from the general language goal. These auxiliary objectives use a question generation (QG) and question answering (QA) system: they consist of questions leading the agent to try to reconstruct partial information about the global goal using its own trajectory. When it succeeds, it receives an intrinsic reward proportional to its confidence in its answer (as illustrated in figure 7). This incentivizes the agent to generate trajectories which unambiguously explain various aspects of the general language goal. Our experimental study shows that this approach, which does not require engineer intervention to design the auxiliary objectives, improves sample efficiency by effectively directing exploration.
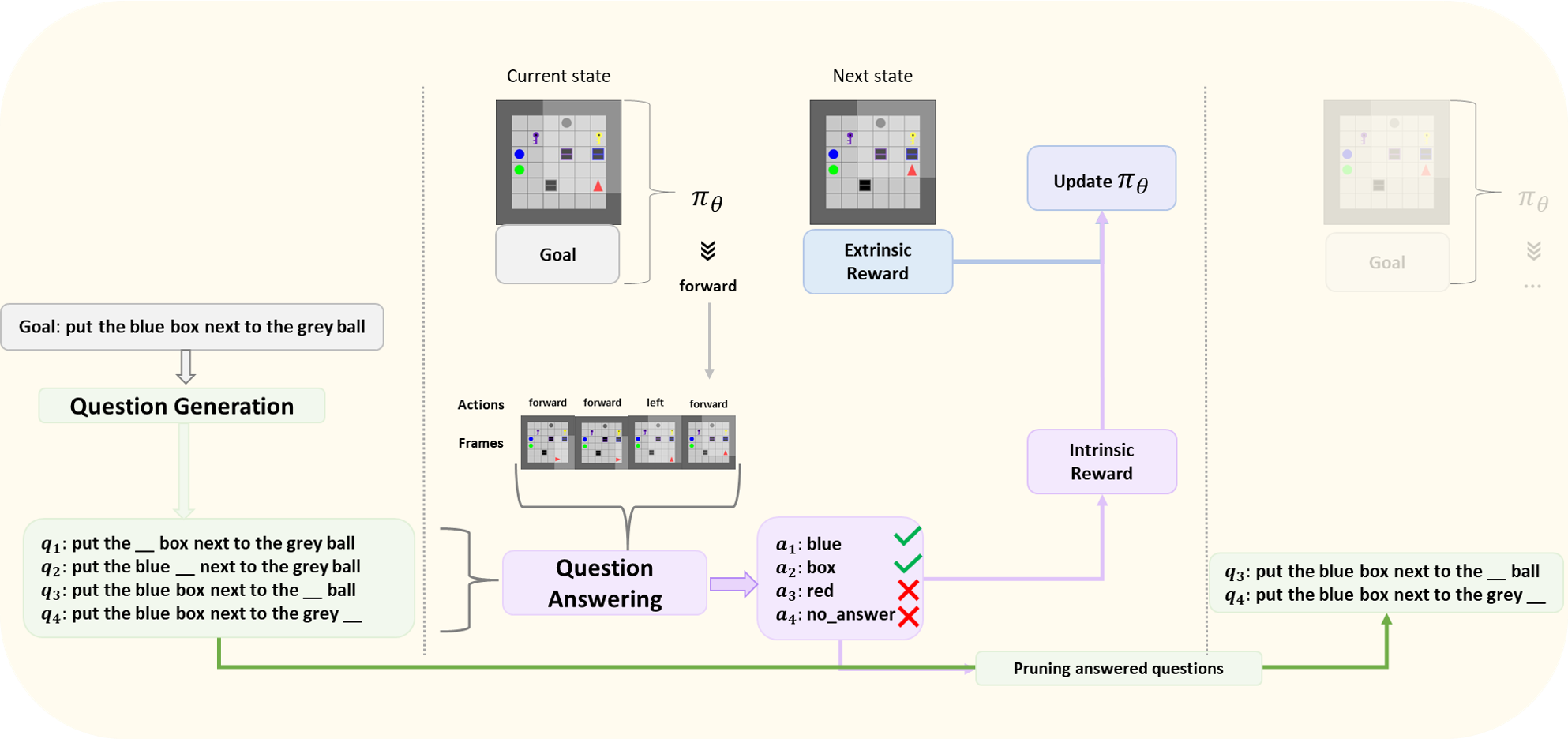
8.2.6 Open-ended recipe crafting through meta-learning
Participants: Gautier Hamon [correspondant], Eleni Nisioti, Clément Moulin-Frier.
As a first step towards studying the evolution of open-ended skill acquisition in artificial agents, we studied the environmental conditions favoring the systematic exploration of combinatorial recipes involving various objects. In this work, the training of an agent uses meta-learning where an outer loop, equivalent to an evolutionary mechanism, meta-learns the parameters of an inner loop which can be seen as a developmental mechanism (where the agent acquire skills during its lifetime by interacting with the environment). In the current setup we use as our meta-learning algorithm 111, 183 which has already been used for acquiring behaviors efficiently balancing exploration and exploitation in a simple navigation task. Other work studied how different conditions in a bandit favor the evolution of innate vs learned behaviors 136.
Our experiments with recipe crafting are inspired by the little alchemy game. The difference with previous works in similar environments (e.g. 8.5.3) is that at every episode the structure of the recipe is randomly chosen. The agent therefore cannot predict what recipes will be rewarding and have to explore different combinations of objects in order to find the rewarding ones. The agent should also memorize the successful and unsuccessful combinations in order to explore and exploit efficiently.
Our preliminary results use both in a vectorized version of the game (where the agents action are only to choose the 2 objects to combine) and an embodied gridworld version (where the agent has to move, grab objects and put them on top of others in order to craft new ones). In both of these cases, the training efficiently meta learns an exploration/exploitation strategy which is to try new recipes (most of the time it does not try non working recipes more than once) until it finds the working ones and then simply exploits them by making them over and over.
Further work will study how we can change the environment/training in order to evolve open-ended exploration strategies where an agent will continuously explore new recipes even if it has already found rewarding ones, as a way to be better prepared for future changes in the recipe structure. We hypothesize that this could be done by introducing drastic changes of recipes which the agent has to anticipate in order to survive.
This work uses JAX python library for both the model/machine learning part and the environment simulation. JAX allows easy parallelization and fast GPU computation and so learning it through this project will be useful for later projects.
8.2.7 Robust Autotelic Learning using Learning Progress in Visual Deep Reinforcement Learning: the GRIMGEP architecture
Participants: Grgur Kovač [correspondant], Adrien Laversanne-Finot, Pierre-Yves Oudeyer.
Autonomous agents, using novelty based goal exploration, are often efficient in environments that require exploration. However, they get attracted to various forms of distracting unlearnable regions. To address this problem, Absolute Learning Progress (ALP) has been used in reinforcement learning agents with predefined goal features and access to expert knowledge. This work extends those concepts to unsupervised image-based goal exploration.
We present the GRIMGEP framework: it provides a learned robust goal sampling prior that can be used on top of current state-of-the-art novelty seeking goal exploration approaches, enabling them to ignore noisy distracting regions while searching for novelty in the learnable regions. It clusters the goal space and estimates ALP for each cluster. These ALP estimates can then be used to detect the distracting regions, and build a prior that enables further goal sampling mechanisms to ignore them.
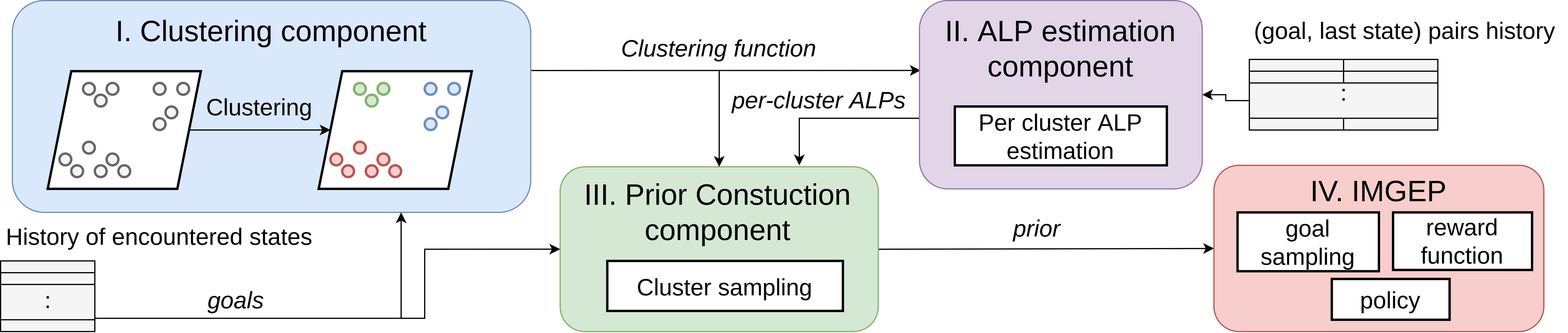
We construct an image based environment with distractors, on which we show that wrapping current state-of-the-art goal exploration algorithms with our framework allows them to concentrate on interesting regions of the environment and drastically improve performances.
In our experiments shown on figure 9, we compare the performance of two novelty-based exploration approaches: Countbased, and Skewfit (with two different values of its hyperparameter ). We can see that wrapping all baselines with the GRIMGEP framework drastically improves their performance.
![]() |
![]() |
![]() |
(a) | (b) | (c) |
Most of this project was conducted in the year 2021. In this year we added an ablation study in which we analysed the impact of choosing the cluster by ALP compared to uniformly. We observed that ALP is essential for the CountBased approach, while for Skewfit the clustering part of GRIMGEP is sufficient to escape the noisy distractor. We finalized the paper and published it in 39. The source code is available at.
8.3 Automatic Curriculum Learning
8.3.1 Towards Automatic Curriculum Learning in Hierarchical Task Spaces
Participants: Rémy Portelas [correspondant], Harm van Seijen, Pierre-Yves Oudeyer.
This work follows a previous team contribution: the TeachMyAgent benchmark 31, which allows researchers to conduct thorough experiments with state-of-the-art Automatic Curriculum Learning methods in various deep reinforcement learning scenarios. Our – still ongoing – objective is to extend the benchmark to feature a new set of ACL challenges: Procgen's 99 challenging pixel-based environments. In addition, considering controllable Procgen environments allows to focus on an understudied ACL problem: how to deal with hierarchical task spaces?, i.e. how to control level generation when encoded as a parametric tree. As a first step towards hierarchical ACL, we propose to adapt ALP-GMM 28 into Hierarchical-ALP-GMM (HALP-GMM). HALP-GMM decomposes the parameter sampling process by using one ALP-GMM teacher per group of parameters, creating a tree of ALP-GMM teachers. After validating our approach in a simulated task space, we are performing experiments on a controllable (hierarchical) Coinrun environment.
8.3.2 Automatic Curriculum Learning for Language Modeling
Participants: Clément Romac [correspondant], Rémy Portelas, Pierre-Yves Oudeyer.
We showed in recent works how Automatic Curriculum Learning (ACL) could help Deep Reinforcement Learning methods by tayloring a curriculum adapted to learner's capabilities 31, 28. Using ACL can lead to sample efficiency, asymptotic performance boost and help in solving hard tasks.
Parallel to this, recent works in Language Modeling using Transformers (e.g. GPT-2) have starting to get more interested in better understanding convergence and learning dynamics of these models. Trained in a supervised setup, these models are fed with hundred of millions of natural language sequences crawled from the web. The current standard way of training these models (i.e. constructing batches of randomly selected sequences) makes the assumption that all sequences have same interest for the model. However, recent works showed that this does not seem to be the case and that datasets can contain outliers harming training. Additionally, some works also showed that hand-designing a curriculum over sequences (e.g. ordered by their length) could speed up and stabilize training.
Building on this, we propose to experiment how ACL could help taylor such a curriculum in an automated way relying on Learning Progress. Our study has several contributions:
- Propose a standardized and more in-depth comparison of current curriculum learning methods used to train language models
- Introduce the first study of ACL in such a training
- Use ACL to propose deeper insights about training dynamics of Transformer models when doing Language Modeling by analysing generated curricula and Learning Progress estimations
We chose to train GPT-2 on the standard OSCAR dataset and use teacher algorithms to select samples that are shown to the model (see fig. 10).
Using ACL, we perform an in-depth analysis of prior methods changing the size of tokens' sequences observed during training following a hand-designed curriculum. Our experiments showed that a Random baseline outperforms these methods. We also provide, thanks to ACL methods based on Learning-Progress Multi-Armed Bandits, hints that while short sequences should not be used as training advances (as Large Language Models quickly learn them), there is no clear evidence that short sequences should be prioritized (and thus long sequences avoided) at the beginning of training.
Additionally, we performed several experiments using more advanced ACL methods on different task spaces and show that these lead to overfitting and underperform in comparison the no-curriculum strategy usually applied in Language Modeling. We hypothesize that, given how large models used in Language Modeling are, it is better to give a huge amount of very diverse and different samples (even though outliers or harmful samples exist) without any curriculum than using a curriculum that restrains the diversity of samples and introduces duplicates (leading to overfitting).
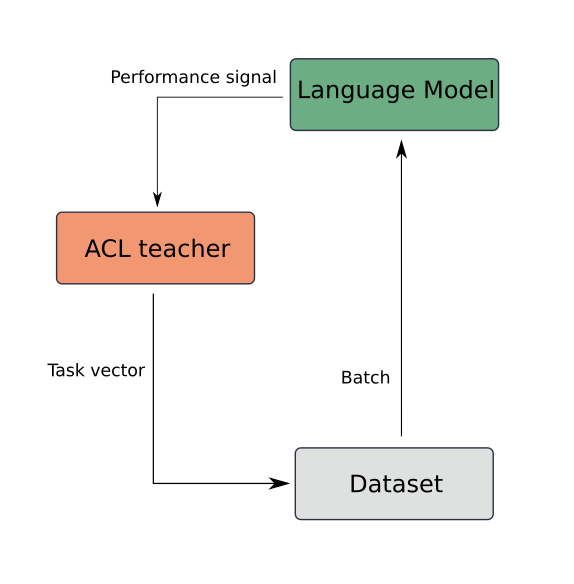
8.4 Learning and Self-organization of Cultural Conventions Between Artificial Agents
Participants: Tristan Karch [correspondant], Clément Moulin-frier, Pierre-Yves Oudeyer.
As introduced in the previous section, Vygotskian artificial agents internalize cultural conventions in order to transform linguistic production into cognitive tools that help them acquire new skills. A fundamental question is therefore to investigate how such cultural conventions can emerge between agents situated in social contexts. The next two new results investigate this question.
8.4.1 Self-organizing cultural conventions in a new interactive AI paradigm: the Architect-Builder Problem
In this experiment, we are interested in interactive agents that learn to coordinate, namely, a builder – which performs actions but ignores the goal of the task, i.e. has no access to rewards – and an architect which guides the builder towards the goal of the task. We define and explore a formal setting where artificial agents are equipped with mechanisms that allow them to simultaneously learn a task while at the same time evolving a shared communication protocol. Ideally, such learning should only rely on high-level communication priors and be able to handle a large variety of tasks and meanings while deriving communication protocols that can be reused across tasks. We present the Architect-Builder Problem (ABP): an asymmetrical setting in which an architect must learn to guide a builder towards constructing a specific structure. The architect knows the target structure but cannot act in the environment and can only send arbitrary messages to the builder. The builder on the other hand can act in the environment, but receives no rewards nor has any knowledge about the task, and must learn to solve it relying only on the messages sent by the architect. Crucially, the meaning of messages is initially not defined nor shared between the agents but must be negotiated throughout learning. The Architect-Builder problem was initially introduced by Vollmer et. al 180 in an experiment named the CoCo game studying the formation of communication protocol between humans in such a context. Diagrams of interactions in the CoCo game and in our numerical adaptation are given in figure 11.
![]() |
![]() |
(a) | (b) |
Under these constraints, we propose Architect-Builder Iterated Guiding (ABIG), a solution to ABP where the architect leverages a learned model of the builder to guide it while the builder uses self-imitation learning to reinforce its guided behavior. We analyze the key learning mechanisms of ABIG and test it in 2D tasks involving grasping cubes, placing them at a given location, or building various shapes. ABIG results in a low-level, high-frequency, guiding communication protocol that not only enables an architect-builder pair to solve the task at hand, but that can also generalize to unseen tasks as illustrated in figure 12. These results were published at the International Conference on Representation Learning (ICLR 2022) 53.
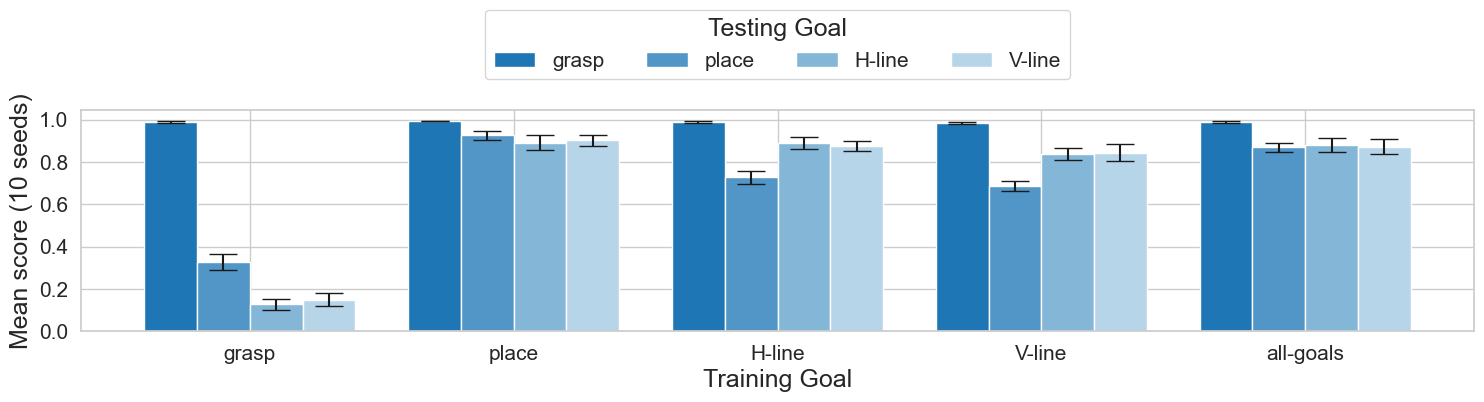
8.4.2 Emergence of a Shared Graphical Language from Visual Inputs
Participants: Tristan Karch [correspondant], Yoann Lemesle, Clément Moulin-frier, Pierre-Yves Oudeyer.
For this project, we place ourselves in the framework of Language Games which are vastly used to study the emergence of languages in populations of agents. Recent contributions relying on deep learning methods focused on agents communicating via an idealized communication channel, where utterances produced by a speaker are directly perceived by a listener. This comes in contrast with human communication, which instead relies on a sensory-motor channel, where motor commands produced by the speaker (e.g. vocal or gestural articulators) result in sensory effects perceived by the listener (e.g. audio or visual). Here, we investigate if agents can evolve a shared language when they are equipped with a continuous sensory-motor system to produce and perceive signs, e.g. drawings. To this end, we introduce the Graphical Referential Game (GREG) where a speaker must produce a graphical utterance to name a visual referent object consisting of combinations of MNIST digits while a listener has to select the corresponding object among distractor referents, given the produced message. The utterances are drawing images produced using dynamical motor primitives combined with a sketching library. A schematic of the GREG is provided in figure 13
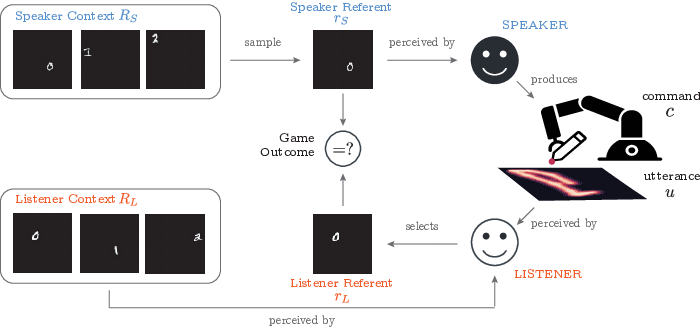
The Graphical Referential Game: During the game, the speaker's goal is to produce a motor command cc that will yield an utterance uu in order to denote a referent rSr_S sampled from a context R˜S\tilde{R}_S. Following this step, the listener needs to interpret the utterance in order to guess the referent it denotes among a context R˜L\tilde{R}_L. The game is a success if the listener and the speaker agree on the referent (rL≡rSr_L\equiv r_S).
To tackle GREG we present CURVES: a multimodal contrastive deep learning mechanism that represents the energy (alignment) between named referents and utterances generated through gradient ascent on the learned energy landscape. We, then, present a set of experiments showing that our method allows the emergence of a shared, graphical language that generalizes to feature compositions never seen during training. We also propose a topographic metric to investigate the compositionality of emergent graphical symbols. Finally, we conduct an ablation study illustrating that sensory-motor constraints are required to yield interpretable lexicons.
![]() |
![]() |
(a) | (b) |
8.4.3 Intrinsically motivated agents in multi-agent environments
Participants: Eleni Nisioti [correspondant], Elias Masquil, Gautier Hamon, Clément Moulin-Frier.
The intrinsically-motivated goal-conditioned learning paradigm is well-established in single-agent settings: by setting its own goals and pursuing them in an environment without external supervision, such an agent is able to acquire a wide diversity of skills 103. Such agents are called autotelic, from the Greek words auto (self) and telos (end). What happens when you transfer the autotelic paradigm to multi-agent environments, where some skills may require the cooperation of multiple agents (lifting a heavy box for example)? This is the question we aimed at addressing in this project. We believe that multi-agent applications will benefit from agents that can autonomously discover and learn cooperative skills, but entail additional challenges to the ones found in single-agent settings: agents that independently set their own goals will have a very low probability of simultaneously sampling the same cooperative goal, which will make solving these goals difficult.
To explore this question, we implemented what we call cooperative navigation tasks in the Simple Playgrounds environments. This 2-D environment, illustrated on the left of figure 15, consists of a room with 6 landmarks on its walls and two agents that receive continuous-valued observations about the distance and angle to all landmarks and other agents and perform discrete-valued actions that control their angular velocity and longitudinal force. A navigation task is a description of the landmarks that need to be reached: some tasks are individual (for example "at least one agent reaches the red landmark") and some are cooperative (for example "at least one agent reaches the red landmark and at least one agent reaches the blue landmark"). Each autotelic agent is learning using the RL algorithm PPO and, at each training episode, chooses which goal to pursue by random sampling within the goal distribution. In addition to a policy conditioned on its goals, the agent also needs a reward function that indicates whether a goal is achieved (see the schematic on the right of figure 15 for an illustration of the main algorithmic components of autotelic agents). In this project, we assume that the two agents already know this reward function and focus on examining the process of decentralized goal selection.
![]() |
![]() |
(a) | (b) |
Our empirical analysis of this set-up showed that goal alignment is important for solving the cooperative tasks we considered: agents that were independently sampling the goals failed to solve all tasks (see orange curve in figure 16) while agents whose goals were determined by a centralized process (blue curve) that guaranteed that the two agents are always pursuing the same goal performed optimally. We then wondered: can we achieve the same performance without requiring centralization? To achieve this we designed a communication-based algorithm that enables a group to align its goals while remaining decentralized: at the beginning of an episode and before determining their goals the two agents exchange messages and then use these messages to condition their goal-selection (see dashed arrows in the schematic on the right of figure 15). This communication is asymmetric: one randomly chosen agent, the leader, uses its goal generator to choose which goal to pursue and then decides what message to transmit to the follower, which conditions its goal selection on the received message. We observed that the agents learn a communication protocol that leads to the alignment of cooperative goals, even though they were not directly incentivised to do so. They were both independently learning a protocol that maximised their individual rewards but, as we show in our experiments corresponding to figure 16, goal alignment was able to emerge from such decentralized learning. We called this algorithm the Goal-coordination game, as it was inspired from another emergent communication algorithm called the Naming game 171.
To get a better understanding of how alignment helps solve this task we measured specialization, which is the tendency of agents to always go the same landmark when there are two options. For example, if for the goal "at least one agent reaches the red landmark and at least one agent reaches the blue landmark" the first agent always go to red and the second goes to blue, then specialization is maximum. We empirically observed that specialization correlates with alignment and is an optimal strategy in our tasks (see right plot of figure 16).
This work was submitted at the AAMAS 2023 conference and a preprint is available on HAL 66. The source code for reproducing the experiments is available at.
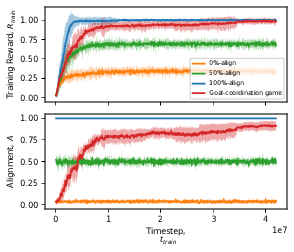
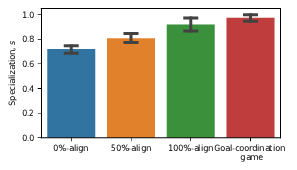
8.4.4 The SocialAI School: Insights from Developmental Psychology Towards Artificial Socio-Cultural Agents
Participants: Grgur Kovač [correspondant], Remy Portelas, Peter Ford Dominey, Pierre-Yves Oudeyer.
Developmental psychologists have long-established socio-cognitive abilities as a core aspect of human intelligence and development 18191. Those abilities enable us to enter, participate in and benefit from human culture. Humans are then able to push our culture forward by improving on already existing cultural artifacts. This is referred to as the cumulative cultural evolution, and it has been argued that the most impressive of human achievements are the product of it 176. It seems clear that to construct artificial agents capable of interacting with and learning from humans one must equip them with socio-cognitive abilities enabling them to enter the human culture. This would enable artificial agents to benefit from our culture, and also push it forwards, i.e. to participate in the cumulative cultural evolution.
Current AI research mostly studies asocial settings or, in the case of Multi-Agent Reinforcement Learning, the emergence of culture (how culture emerges in the first place, rather than how one enters an already existing culture). Furthermore, this research is often done without a strong grounding in developmental psychology.
In this project, we follow the work of Michael Tomasello and Jerome Bruner who outlined a set of core socio-cognitive skills, e.g. social cognition (joint attention, theory of mind), referential and conventionalized communication, imitation, role-reversal, scaffolding, and many more 175, 91. Importantly, they also showed the importance the (cultural) environment for cognitive development.
To introduce some of those concepts to the AI community, we constructed a procedurally generated suite of environments - The SocialAI school. With The SocialAI school, experiments studying those socio-cognitive abilities can be easily conducted and cognitive-science experiments reconstructed. An example of a SocialAI school environment is shown in figure 17. In it the peer is pointing towards the red box. The agent (the red triangle) has to infer this to mean that the apple is hidden inside the red box.
We conducted many experiments, here we outline a few more important ones. In the following experiments we experimented with multimodal RL agents. We tested generalization of inferring the meaning of referential communication (ex. the pointing gesture) to new scenarios/objects. We found that such a generalization is very hard for standard reinforcement learning agents. We show how a scaffolded environment helps with learning complex interaction sequences (formats). To show how cognitve science experiments can be recreated - we reconstruct a study of role reversal from 115. Furthermore, we conducted experiments regarding other aspects of social-cognition: joint attention, imitation, perspective taking, etc. The SocialAI school is not limited to RL agents. For example, these environments can easily be transformed into pure text. We created one case study to demonstrate how this feature can be used to study large-language models as interactive agents.
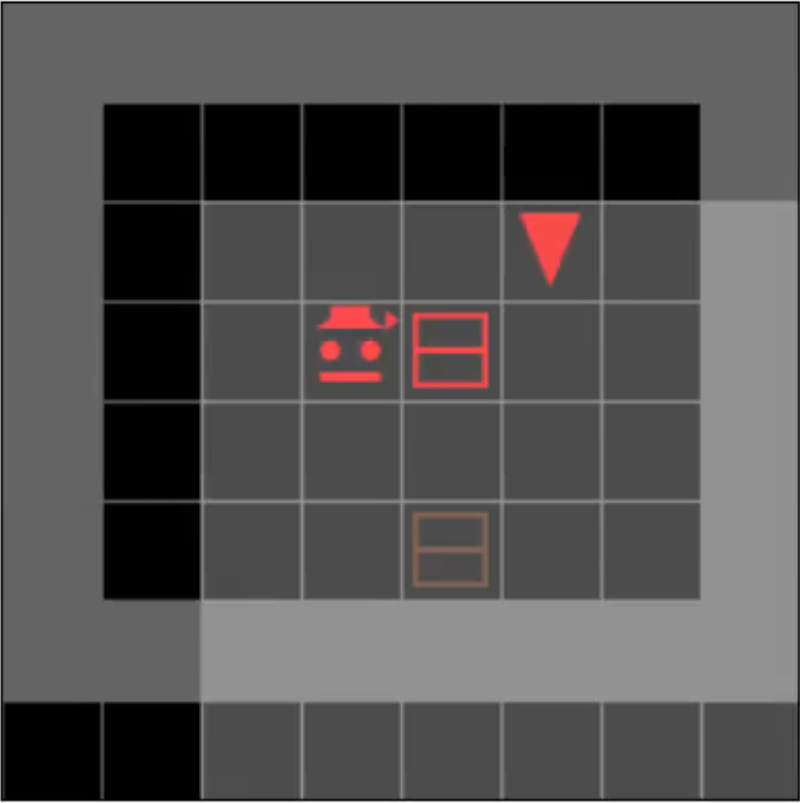
We stared working on this project in the year 2021, in which we created only a few small experiments 135. In this year, we significantly extended the project. We grounded it more explicitly in cognitive-science (especially in the work of Tomasello and Bruner). We created a procedural generation engine using which we constructed many additional environments and conducted many additional experiments (discussed above).
8.5 Ecological Artificial Intelligence
8.5.1 Research perspective: The Ecology of Open-Ended skill Acquisition
Participants: Clément Moulin-Frier [correspondant], Eleni Nisioti, Pierre-Yves Oudeyer.
An intriguing feature of the human species is our ability to continuously invent new problems and to proactively acquiring new skills in order to solve them: what is called Open-Ended Skill Acquisition (OESA). Understanding the mechanisms underlying OESA is an important scientific challenge in both cognitive science (e.g. by studying infant cognitive development, see section 8.1) and in artificial intelligence (aiming at computational architectures capable of open-ended learning, see section 8.2). Both fields, however, mostly focus on cognitive and social mechanisms at the scale of an individual’s life. It is rarely acknowledged that OESA, an ability that is fundamentally related to the characteristics of human intelligence, has been necessarily shaped by ecological, evolutionary and cultural mechanisms interacting at multiple spatiotemporal scales.
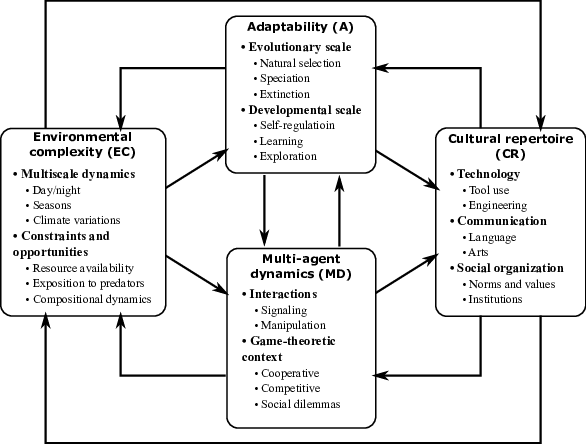
We have recently initiated a new research direction aiming at understanding, modeling and simulating the dynamics of OESA in artificial systems, grounded in theories studying its eco-evolutionary bases in the human species. For this aim, we have proposed a conceptual framework, called ORIGINS (illustrated Fig. 18 and developed in 60), expressing the complex interactions between environmental, adaptive, multi-agent and cultural dynamics. This framework raises three main research questions:
- What are the ecological conditions favoring the evolution of autotelic agents?
- How to bootstrap the formation of a cultural repertoire in populations of adaptive agents?
- What is the role of cultural feedback effects in the open-ended dynamics of human skill acquisition?
The contributions described below are addressing some aspects of these research questions.
8.5.2 Evolution of plasticity and evolvability in variable environments
Participants: Eleni Nisioti [correspondant], Clément Moulin-Frier.
The diversity and quality of natural systems have been a puzzle and inspiration for communities studying artificial life. It is now widely admitted that the adaptation mechanisms enabling these properties are largely influenced by the environments they inhabit. Organisms facing environmental variability have two alternative adaptation mechanisms operating at different timescales: plasticity, the ability of a phenotype to survive in diverse environments and evolvability, the ability to adapt through mutations. Although vital under environmental variability, both mechanisms are associated with fitness costs hypothesized to render them unnecessary in stable environments. In this work, we aimed at studying the interplay between environmental dynamics and adaptation in a minimal model of the evolution of plasticity and evolvability.
To achieve this we designed a simulation environment that attempts to capture the spatial and temporal heterogeneity of real-world environments while keeping the computational complexity low: the user can choose the number of niches, which are arranged in a simple longitudinal model, and a climate function that captures the temporal variation of environmental conditions, which are arranged based on a simple longitudinal model (see left of figure 19 for an illustration of the environment). We defined the evolvability of an agent as its mutation rate and capture plasticity using tolerance curves, a tool developed in ecology 120. Tolerance curves (which we visualize on the right of Figure 19) have the form of a Gaussian whose mean shows the preferred environmental state of an individual and the variance its plasticity, i.e., its ability to survive under different environmental conditions. This figure also illustrates the cost and benefit of plasticity. If both individuals are at their preferred niche, which coincides with the environmental state, then the plastic individual has lower fitness that the non-plastic (cost of plasticity). If the actual environmental state differs significantly from the preferred one, the plastic individual has higher fitness (benefit of plasticity).
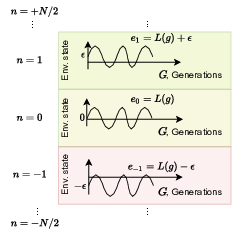
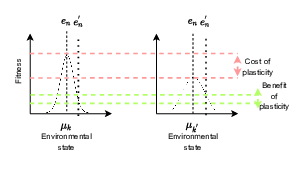
We conducted an extensive empirical study in this environment that aimed at disentangling the effects of different mechanisms: we studied three types of climate functions (stable, sinusoid, noisy) and two types of evolutionary selection pressures (survival of the fittest and niche-limited competition) and environments were the number of niches varies from 1 to 100. Through these simulations we showed that environmental dynamics affect plasticity and evolvability differently and that the selection mechanism matters: a) in stable environments with a large number of niches when both selection-based fitness and niche-limited competition (we call this method NF-selection) are activated, plasticity remains high despite its cost (see left plot in Figure 20) ; b) in a noisy environment introducing niche-limited competition (N-selection and NF-selection) makes populations capable of resisting larger amounts of noise (see right plot in Figure 20). We presented our work at GECCO 2022 55 and open-sourced the software for reproducing our simulations at.
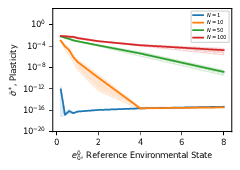
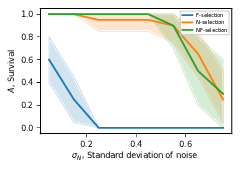
8.5.3 The effect of social network structure on collective innovation
Participants: Eleni Nisioti [correspondant], Mateo Mahaut, Pierre-Yves Oudeyer, Ida Momennejad, Clément Moulin-Frier.
Innovations are a central component of open-ended skill acquisition: they denote the emergence of new solutions by the recombination of existing ones and their presence is necessary to ensure a continuous complexification of an agent's cultural repertoire. While we often tend to attribute discoveries to certain innovative individuals, if we shed a broad perspective at the history of our species we see that human innovation is primarily a collective process. Fields such as psychology and anthropology have been studying the ability of human groups to innovate for some time, with studies indicating that the social network structure has a significant impact: fully-connected structures are better suited for quick convergence in easy problems with clear global optima, while partially-connected structures perform best in difficult tasks where local optima may lure agents away from the globally optimal solution 110. At the same time a parallel story is unfolding in reinforcement learning (RL): distributed RL is a sub-field where multiple agents solve a task collectively 150. Compared to the single-agent paradigm, distributed RL algorithms converge quicker and often achieve superior performance. However, these algorithms have only considered full connectivity. In this inter-disciplinary project, we presented a novel learning framework that augments distributed RL with the notion of a social network structure and employed it to study the hypothesis from human studies that partial connectivity performs best in innovation tasks.
We implemented such innovation tasks using Wordcraft, a recently introduced RL playground inspired from the Little Alchemy 2 game (see left of figure 21 for an illustration of how this task works). We considered a wide diversity of social network structures: static structures that remain constant throughout learning (fully-connected, ring, small-world) and a dynamic structure where the group oscillates between phases of low and high connectivity (we illustrate this dynamic structure on the right of figure 21). Each agent in our implementation employs the DQN learning algorithm and exchanges experiences that have the form of sequences of state-action combinations with its neighbors.
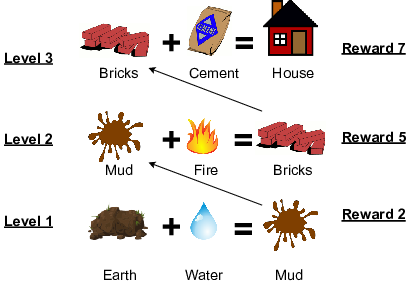
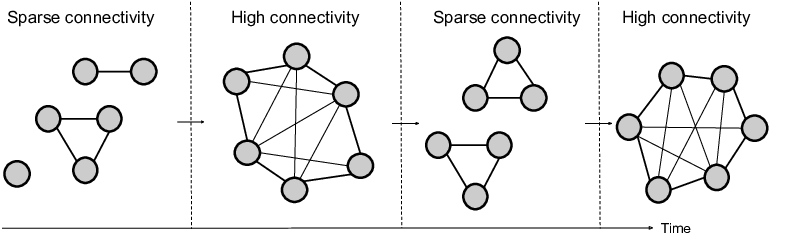
A central conclusion of our empirical analysis was that the dynamic social network structure performs best. In addition to the performance groups achieve we measured behavioral and mnemonic metrics such as behavioral conformity and mnemonic diversity. Such metrics were inspired from human studies and helped us further analyze the behavior of groups. For example, one empirical observation was that sharing experiences did not help the group learn quicker in a very simple innovation task; instead the fully-connected group was the slowest. By looking at the diversity in the memories of the agents we observed that the fully-connected structure had the highest individual diversity (left of figure 22 ) and the lowest group diversity (right of figure 22): sharing experiences with others diversifies an individual's experiences but also homogenizes the group, which is bad for its performance.
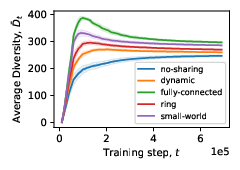
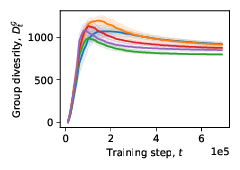
We see the contribution of this project as two-fold. From the perspective of fields studying human intelligence, we have shown that using RL algorithms as computational tool is a promising direction towards increasing the verisimilitude of simulations and analyzing both behavior and memory. From the perspective of RL, we have shown that distributed RL algorithm should move beyond the fully-connected architecture and explore groups with dynamic topologies. This work is currently a preprint 67 and under review at ICLR 2023 and we open-source the code at.
8.5.4 Socially Supervised Representation Learning: the Role of Subjectivity in Learning Efficient Representations
Participants: Julius Taylor, Eleni Nisioti [correspondant], Clément Moulin-Frier.
Introduction
In this work 50 (published at the AAMAS 2022 conference), we propose that aligning internal subjective representations, which naturally arise in a multi-agent setup where agents receive partial observations of the same underlying environmental state, can lead to more data-efficient representations. We propose that multi-agent environments, where agents do not have access to the observations of others but can communicate within a limited range, guarantees a common context that can be leveraged in individual representation learning. The reason is that subjective observations necessarily refer to the same subset of the underlying environmental states and that communication about these states can freely offer a supervised signal. To highlight the importance of communication, we refer to our setting as socially supervised representation learning. We present a minimal architecture comprised of a population of autoencoders, where we define loss functions, capturing different aspects of effective communication, and examine their effect on the learned representations.
Contributions.
We summarise our contributions as follows:
- We highlight an interesting link between data-augmentation traditionally used in single-agent self-supervised setting and a group of agents interacting in a shared environment.
- We introduce Socially Supervised Representation Learning, a new learning paradigm for unsupervised learning of efficient representations in a multi-agent setup.
- We present a detailed analysis of the conditions ensuring both the learning of efficient individual representations and the alignment of those representations across the agent population.
Methods
We consider a population of agents and environment states , hidden to the agents. Each agent receives a private observation of the state , where is an observation space. Agents are essentially convolutional autoencoders, though other self-supervised learning technique could be used (for example variational autoencoders 132). We define encoder and decoder functions and , respectively, where is a latent representation space (also called a message space, see below). Given an input observation, an agent encodes it into a latent representation and attempts at reconstructing the observation through , dropping the dependence on for brevity. Agents will use these latent vectors to communicate to other agents about their perceptual inputs (hence the term message space for ). When agent receives a message from agent they decode the message using their own decoder, i.e. .
In order to incentivise communication in our system, we define four loss functions which encourage agents to converge on a common protocol in their latent spaces. First, we define the message-to-message loss as
This loss directly incentivises that two messages (i.e. encodings) are similar. Since messages are always received in a shared context, this loss encourages agents to find a common representation for the observed state, abstracting away particularities induced by the specific viewpoint of an agent. Next, we propose the decoding-to-input loss, given by
This loss brings the decoding of agent from agent 's message closer to agent 's input observation, indirectly incentivising an alignment of representations because both agents can reconstruct from the other agents message more easily, when they agree on a common latent code i.e. they have similar representations for a given . Then, we propose the decoding-to-decoding loss:
which is computed using the reconstructed input of agent and the reconstruction of incurred from the message sent by . Lastly, the standard autoencoding loss is given by
The message of agent is defined as , with , where is a hyperparameter in our system. The total loss we optimise is thus
with , , , and being tunable hyperparameters.
Results
![]() |
![]() |
![]() |
![]() |
We show that our proposed architecture allows the emergence of aligned representations. This means that different agents find similar encodings for the same sensory inputs. The subjectivity introduced by presenting agents with distinct perspectives of the environment state contributes to learning abstract representations that outperform those learned by a single autoencoder and a population of autoencoders, presented with identical perspectives of the environment state, which is shown in the left column of Fig. 23. Furthermore, in Fig. 23 (right) we show that the learned representations are data-efficient, i.e. they enjoy the most benefit when evaluated on small testing splits. This is important, because good representations should allow agents to adapt to downstream tasks quickly and with few samples. Altogether, our results demonstrate how communication from subjective perspectives can lead to the acquisition of more abstract representations in multi-agent systems, opening promising perspectives for future research at the intersection of representation learning and emergent communication.
8.6 Applications in Educational Technologies
8.6.1 Machine Learning for Adaptive Personalization in Intelligent Tutoring Systems
Participants: Pierre-Yves Oudeyer [correspondant], Benjamin Clément, Didier Roy, Hélène Sauzeon.
The Kidlearn project
is a research project studying how machine learning can be applied to intelligent tutoring systems. It aims at developing methodologies and software which adaptively personalize sequences of learning activities to the particularities of each individual student. Our systems aim at proposing to the student the right activity at the right time, maximizing concurrently his learning progress and his motivation. In addition to contributing to the efficiency of learning and motivation, the approach is also made to reduce the time needed to design ITS systems.
We continued to develop an approach to Intelligent Tutoring Systems which adaptively personalizes sequences of learning activities to maximize skills acquired by students, taking into account the limited time and motivational resources. At a given point in time, the system proposes to the students the activity which makes them progress faster. We introduced two algorithms that rely on the empirical estimation of the learning progress, RiARiT that uses information about the difficulty of each exercise and ZPDES that uses much less knowledge about the problem.
The system is based on the combination of three approaches. First, it leverages recent models of intrinsically motivated learning by transposing them to active teaching, relying on empirical estimation of learning progress provided by specific activities to particular students. Second, it uses state-of-the-art Multi-Arm Bandit (MAB) techniques to efficiently manage the exploration/exploitation challenge of this optimization process. Third, it leverages expert knowledge to constrain and bootstrap initial exploration of the MAB, while requiring only coarse guidance information of the expert and allowing the system to deal with didactic gaps in its knowledge. The system was evaluated in several large-scale experiments relying on a scenario where 7-8 year old schoolchildren learn how to decompose numbers while manipulating money 98. Systematic experiments were also presented with simulated students.
Kidlearn Experiments 2018-2019: Evaluating the impact of ZPDES and choice on learning efficiency and motivation
An experiment was held between March 2018 and July 2019 in order to test the Kidlearn framework in classrooms in Bordeaux Metropole. 600 students from Bordeaux Metropole participated in the experiment. This study had several goals. The first goal was to evaluate the impact of the Kidlearn framework on motivation and learning compared to an Expert Sequence without machine learning. The second goal was to observe the impact of using learning progress to select exercise types within the ZPDES algorithm compared to a random policy. The third goal was to observe the impact of combining ZPDES with the ability to let children make different kinds of choices during the use of the ITS. The last goal was to use the psychological and contextual data measures to see if correlation can be observed between the students psychological state evolution, their profile, their motivation and their learning. The different observations showed that generally, algorithms based on ZPDES provided a better learning experience than an expert sequence. In particular, they provide a more motivating and enriching experience to self-determined students. Details of these new results, as well as the overall results of this project, are presented in Benjamin Clément PhD thesis 97 and are currently being processed to be published.
Kidlearn and Adaptiv'Math
The algorithms developed during the Kidlearn project and Benjamin Clement thesis 97 are being used in an innovation partnership for the development of a pedagogical assistant based on artificial intelligence intended for teachers and students of cycle 2. The algorithms are being written in typescript for the need of the project. The expertise of the team in creating the pedagogical graph and defining the graph parameters used for the algorithms is also a crucial part of the role of the team for the project. One of the main goal of the team here is to transfer technologies developed in the team in a project with the perspective of industrial scaling and see the impact and the feasibility of such scaling.
Kidlearn for numeracy skills with individuals with autism spectrum disorders
Few digital interventions targeting numeracy skills have been evaluated with individuals with autism spectrum disorder (ASD) 57146. Yet, some children and adolescents with ASD have learning difficulties and/or a significant academic delay in mathematics. While ITS are successfully developed for typically developed students to personalize learning curriculum and then to foster the motivation-learning coupling, they are not or fewly proposed today to student with specific needs. The objective of this pilot study is to test the feasibility of a digital intervention using an STI with high school students with ASD and/or intellectual disability. This application (KidLearn) provides calculation training through currency exchange activities, with a dynamic exercise sequence selection algorithm (ZPDES). 24 students with ASD and/or DI enrolled in specialized classrooms were recruited and divided into two groups: 14 students used the KidLearn application, and 10 students received a control application. Pre-post evaluations show that students using KidLearn improved their calculation performance, and had a higher level of motivation at the end of the intervention than the control group. These results encourage the use of an STI with students with specific needs to teach numeracy skills, but need to be replicated on a larger scale. Suggestions for adjusting the interface and teaching method are suggested to improve the impact of the application on students with autism. 41.
8.6.2 Machine learning for adaptive cognitive training
Participants: Pierre-Yves Oudeyer, Hélène Sauzéon [correspondant], Masataka Sawayama, Benjamin Clément, Maxime Adolphe.
Because of its cross-cutting nature to all cognitive activities such as learning tasks, attention is a hallmark of good cognitive health throughout life and more particularly in the current context of societal crisis of attention. Recent works have shown the great potential of computerized attention training for an example of attention training, with efficient training transfers to other cognitive activities, and this, over a wide spectrum of individuals (children, elderly, individuals with cognitive pathology such as Attention Deficit and Hyperactivity Disorders). Despite this promising result, a major hurdle is challenging: the high inter-individual variability in responding to such interventions. Some individuals are good responders (significant improvement) to the intervention, others respond variably, and finally some respond poorly, not at all, or occasionally. A central limitation of computerized attention training systems is that the training sequences operate in a linear, non-personalized manner: difficulty increases in the same way and along the same dimensions for all subjects. However, different subjects require in principle a progression at a different, personalized pace according to the different dimensions that characterize attentional training exercises.
To tackle the issue of inter-individual variability, the present project proposes to apply some principles from intelligent tutoring systems (ITS) to the field of attention training. In this context, we have already developed automatic curriculum learning algorithms such as those developed in the KidLearn project, which allow to customize the learner's path according to his/her progress and thus optimize his/her learning trajectory while stimulating his/her motivation by the progress made. ITS are widely identified in intervention research as a successful way to address the challenge of personalization, but no studies to date have actually been conducted for attention training. Thus, whether ITS, and in particular personalization algorithms, can optimize the number of respondents to an attention training program remains an open question.
To investigate this question, an ongoing work on systematically reviewing the literature of the use of ITS in the field of cognitive training has been started. In parallel to this, a web platform has been designed for planning and implementing remote behavioural studies. This tool provides means for registering recruited participants remotely and executing complete experimental protocols: from presenting instructions and obtaining informed consents, to administering behavioural tasks and questionnaires, potentially throughout multiple sessions spanning days or weeks. In addition to this platform, a cognitive test battery composed of seven classical behavioural tasks has been developed. This battery aims to evaluate the evolution of the cognitive performance of participants before and after training. Fully open-source, it mainly targets attention and memory. A preliminary study on a large sample of 50 healthy participants showed that the developed tasks reproduced the results of previous studies, that there were large differences between individuals (no ceiling effect) and that the results were significantly reliable between two measurements taken on two days separated by one night 35.
Utilizing these tools, a pilot study campaign was conducted to evaluate the impact of our AI-based personalized cognitive training program. The first experiment involved n=27 participants and aimed to compare the effectiveness of a cognitive training program using a linear difficulty management procedure (staircase procedure) to a program using an ITS for difficulty manipulation. The online training lasted for 10 hours over a period of 2 weeks. The results indicated that the ITS-based intervention produced diverse learning trajectories compared to the linear procedure 24, leading to broader improvements in pre-post cognitive assessment. However, no significant differences were observed in subjective measures of motivation and engagement between the two groups.
Subsequent to this initial experiment, two pilot studies (n=11 and n=10, respectively) were conducted with the goal of enhancing motivation and engagement in the game. The first study implemented gamified components such as scores and feedback, while the second study examined hyperparameter updates to the ITS. The analysis of learning trajectories, learning outcomes, and subjective measures yielded promising results in favor of the AI-based personalized procedure. However, the optimal hyperparameter configuration for the ITS algorithm remains uncertain due to the multiple mechanisms involved. To address this issue, a toy environment is being developed with the objective of identifying an a priori optimal configuration of the ITS. The next step will be to test this optimal configuration in a new study with human participants.
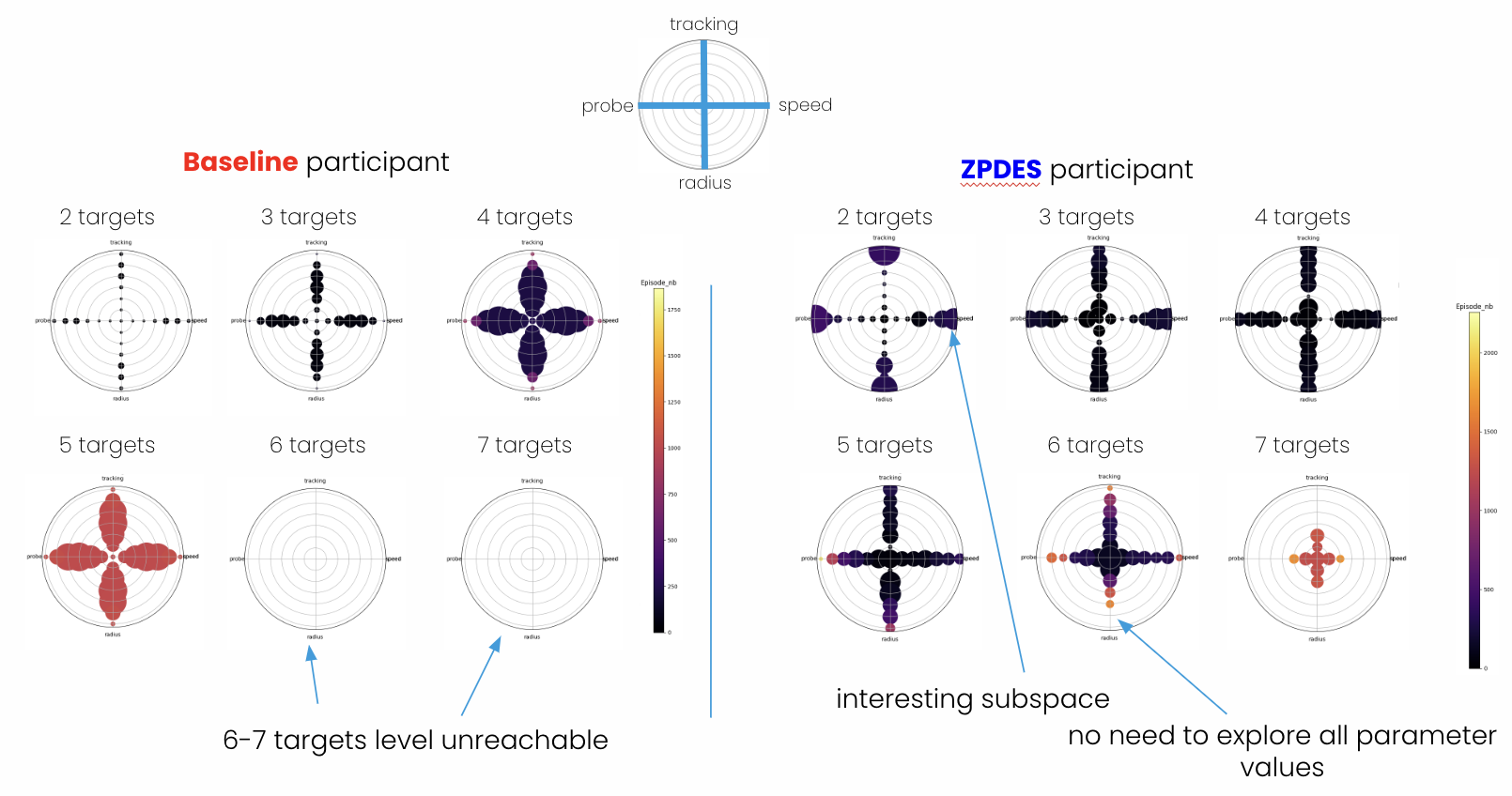
Different learning trajectories for a selected participant in the staircase group (left) and the ITS group (right). The color of a dot indicates the initial presentation of the parameter value, while the size of the dot represents the frequency of the parameter value.
8.6.3 Fostering curiosity and meta-cognition in children using conversational agents
Participants: Pierre-Yves Oudeyer, Hélène Sauzéon [correspondant], Mehdi Alami, Rania Abdelghani, Didier Roy, Edith Law, Pauline Lucas.
Since 2019 via the renewal of the Idex cooperation fund (between the University of Bordeaux and the University of Waterloo, Canada) led by the Flowers team and also involving F. Lotte from the Potioc team, we continue our work on the development of new curiosity-driven interaction systems. Although experiments have been slowed down by sanitary conditions, progress has been made in this area of application of FLOWERS works. In particular, three studies have been completed.
The first study regards a new interactive educational application to foster curiosity-driven question-asking in children. This study has been performed during the Master 2 internship of Mehdi Alaimi co-supervised by H. Sauzéon, E. Law and PY Oudeyer. It addresses a key challenge for 21st-century schools, i.e., teaching diverse students with varied abilities and motivations for learning, such as curiosity within educational settings. Among variables eliciting curiosity state, one is known as « knowledge gap », which is a motor for curiosity-driven exploration and learning. It leads to question-asking which is an important factor in the curiosity process and the construction of academic knowledge. However, children questions in classroom are not really frequent and don’t really necessitate deep reasoning. Determined to improve children’s curiosity, we developed a digital application aiming to foster curiosity-related question-asking from texts and their perception of curiosity. To assess its efficiency, we conducted a study with 95 fifth grade students of Bordeaux elementary schools. Two types of interventions were designed, one trying to focus children on the construction of low-level question (i.e. convergent) and one focusing them on high-level questions (i.e. divergent) with the help of prompts or questions starters models. We observed that both interventions increased the number of divergent questions, the question fluency performance, while they did not significantly improve the curiosity perception despite high intrinsic motivation scores they have elicited in children. The curiosity-trait score positively impacted the divergent question score under divergent condition, but not under convergent condition. The overall results supported the efficiency and usefulness of digital applications for fostering children’s curiosity that we need to explore further. The overall results are published in CHI'20 80. In parallel to these first experimental works, we wrote this year a review of the existing works on the subject 93.
The second study investigates the neurophysiological underpinnings of curiosity and the opportunities of their use for Brain-computer interactions 82. Understanding the neurophysiological mechanisms underlying curiosity and therefore being able to identify the curiosity level of a person, would provide useful information for researchers and designers in numerous fields such as neuroscience, psychology, and computer science. A first step to uncovering the neural correlates of curiosity is to collect neurophysiological signals during states of curiosity, in order to develop signal processing and machine learning (ML) tools to recognize the curious states from the non-curious ones. Thus, we ran an experiment in which we used electroencephalography (EEG) to measure the brain activity of participants as they were induced into states of curiosity, using trivia question and answer chains. We used two ML algorithms, i.e. Filter Bank Common Spatial Pattern (FBCSP) coupled with a Linear Discriminant Algorithm (LDA), as well as a Filter Bank Tangent Space Classifier (FBTSC), to classify the curious EEG signals from the non-curious ones. Global results indicate that both algorithms obtained better performances in the 3-to-5s time windows, suggesting an optimal time window length of 4 seconds to go towards curiosity states estimation based on EEG signals. These results have been published 82
Finally, the third study investigates the role of intrinsic motivation in spatial learning in children (paper in progress). In this study, the state curiosity is manipulated as a preference for a level of uncertainty during the exploration of new environments. To this end, a series of virtual environments have been created and is presented to children. During encoding, participants explore routes in environments according the three levels of uncertainty (low, medium, and high), thanks to a virtual reality headset and controllers and, are later asked to retrace their travelled routes. The exploration area and the wayfinding. ie the route overlap between encoding and retrieval phase, (an indicator of spatial memory accuracy) are measured. Neuropsychological tests are also performed. Preliminary results showed that there are better performances under the medium uncertainty condition in terms of exploration area and wayfinding score. These first results supports the idea that curiosity states are a learning booster (paper in progress).
At the end of 2020, we started an industrial collaboration project with EvidenceB on this topic (CIFRE contract of Rania Abdelghani validated by the ANRT). The overall objective of the thesis is to propose new educational technologies driven by epistemic curiosity, and allowing children to express themselves more and learn better. To this end, a central question of the work will be to specify the impact of self-questioning aroused by states of curiosity about student performance. Another objective will be to create and study the pedagogical impact of new educational technologies in real situations (schools) promoting an active education of students based on their curiosity. To this end, a web platform called 'Kids Ask' has been designed, developed and tested in three primary schools. The tool offers an interaction with a conversational agent that trains children's abilities to generate curiosity-driven questions and use these questions to explore a learning environment and acquire new knowledge. The results suggest that the configuration helped enhance children's questioning and exploratory behaviors; they also show that learning progress differences in children can be explained by the differences in their curiosity-driven behaviors 34.
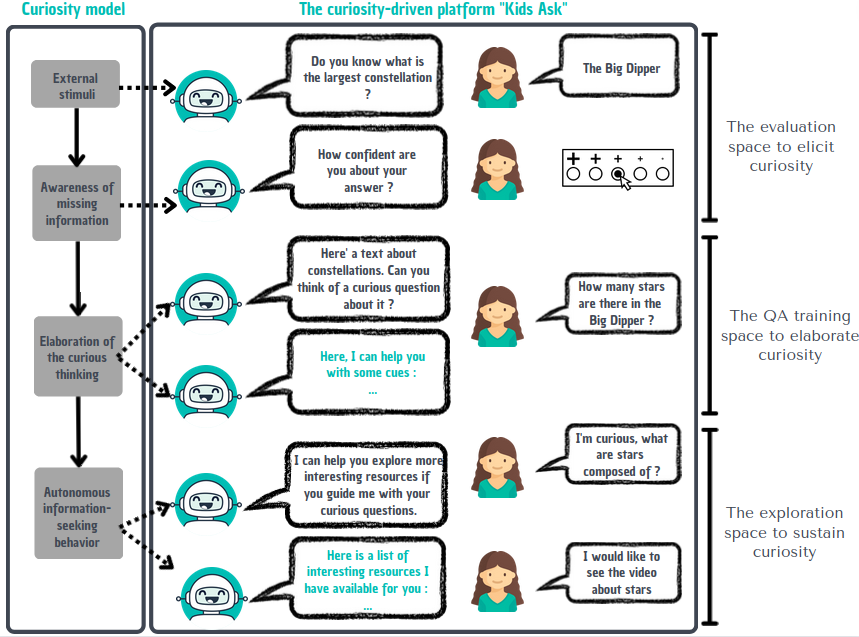
Illustration of a conversational agent's strategies in the different work spaces of the "Kids Ask" platform
Despite showing pedagogical efficiency, the method used in the first study of this PhD is still very limited since it relies on generating curiosity-prompting cues by hand for each educational resource in order to feed the "discussion" with the agent, which can be a very long and costly process. For this reason, a logical follow-up to scale-up and generalize this study was to explore ways to automate the said conversational agents' behaviors in order to facilitate their implementation on a larger scale and for different learning tasks. More particularly, we move towards the natural language processing (NLP) field and the large language models (LLMs) that showed an impressive ability in generating text that resembles the way people write.
In this context, we study using the recent LLM GPT-3 to implement conversational agents that can prompt children's curiosity about a given text-based educational content, by proposing some specific cues. We investigate the validity of this automation method by comparing its impact on children's divergent question-asking skills with respect to the hand-crafted condition we had in our previous work. In a second step, we explore using GPT-3 to propose a new curiosity-prompting behavior for our agent that aims to better support the children's needs of competence, autonomy and relatedness during the question-asking training.
The study was conducted in two primary schools with 75 children aged between 9 and 11. Our first results suggest the validity of using GPT-3 to facilitate the implementation of curiosity-stimulating learning technologies. Indeed, children's performance was similar between the conditions where they had hand-generated or GPT-3-generated cues. In a second step, we also found that GPT-3 can be efficient in proposing the relevant cues that leave children with more autonomy to express their curiosity 63 (publication in process).
![]() |
![]() |
Finally, as a follow-up direction to this line of work, we design new digital interventions that focus on eliciting the metacognitive mechanisms involved in the stimulation and continuity of curiosity, and not just giving the tools to pursue it as done in the previous studies. For this, we use findings from the theories explaining curiosity in order to break this latter up into a set of relevant metacognitive skills. We then take an operational approach to propose adequate digital metacognitive exercises for each one of the said skills (i.e. exercises to identify uncertainty, generate hypotheses etc). We aim to implement this set of metacognitive exercises and investigate its impact on children's abilities to initiate and maintain curious behaviors. We would also be interested in investigating the impact of such training on the learning progress children can achieve. A pilot study has been conducted to evaluate the accessibility of this new training and we are working to recruit more participants to investigate the desired effects in the upcoming months.
8.6.4 ToGather : Interactive website to foster collaboration among stakeholders of school inclusion for pupils with neurodevelopmental disorders
Participants: Hélène Sauzéon [correspondant], Cécile Mazon, Eric Meyer, Isabeau Saint-Supery, Christelle Maillart [Uni. Liège, Belgium], Kamélia Belassel, Mathieu Périé.
Sustain and support the follow-up of the school inclusion of children with neurodevelopmental disorders (e.g., autism, attention disorders, intellectual deficiencies) has become an emergency : the higher is the school level, the lower is the amount of schooled pupils with cognitive disabilities.Technology-based interventions to improve school inclusion of children with neurodevelopmental disorders have mostly been individual centered, focusing on their socio-adaptive and cognitive impairments and implying they have to adapt themselves in order to fit in our society's expectations. Although this approach centered on the normalization of the person has some advantages (reduction of clinical symptoms), it carries social stereotypes and misconceptions of cognitive disability that are not respectful of the cognitive diversity and intrinsic motivations of the person, and in particular of the student's wishes in terms of school curriculum to achieve his or her future life project.
The "ToGather" project aims at enlightening the field of educational technologies for special education by proposing an approach centered on the educational needs of the students and by bringing a concerted and informed answer between all the stakeholders including the student and all their support spheres (family, school, medico-social care). To this end, ToGather project that emanates from participatory design methods, primarily consists of having developed a pragmatic tool (interactive website) to help students with cognitive disability and their caregivers to formalize and to visualize the repertoire of academic skills of the student and to make it evolve according to his or her proximal zone of development (in the sense of Vygotsky) on the one hand, and to the intrinsic motivations of the student (his or her own educational and life project) on the other 145.
Usability validation in France
To validate its usability (interaction data, user experience, motivations of different users, etc. ), user testing were performed on the mock-up of the website with 7 parents, 7 teachers and 7 social services professionals (n=21 in total).
![]() |
![]() |
Participants were given a scenario with tasks to perform on the mock-up, then they were asked to answer some questionnaires to collect subjective measures, followed by an interview to debrief on the experiment. It was conducted online so the participants' screen were recorded and a video analysis was performed according to a grid to collect objective measures (effectiveness and efficiency). Using the mock-up elicited low cognitive load and showed low average perceived difficulty of usability with an excellent user experience, as asked per the users who participated in the design process. The high level of elicited self-determination feeling underlines the need for a collaborative tool to gather information on the pupil, empower each stakeholder by inviting them to participate in every collective decision and giving them the means to coordinate actions led towards the pupil.
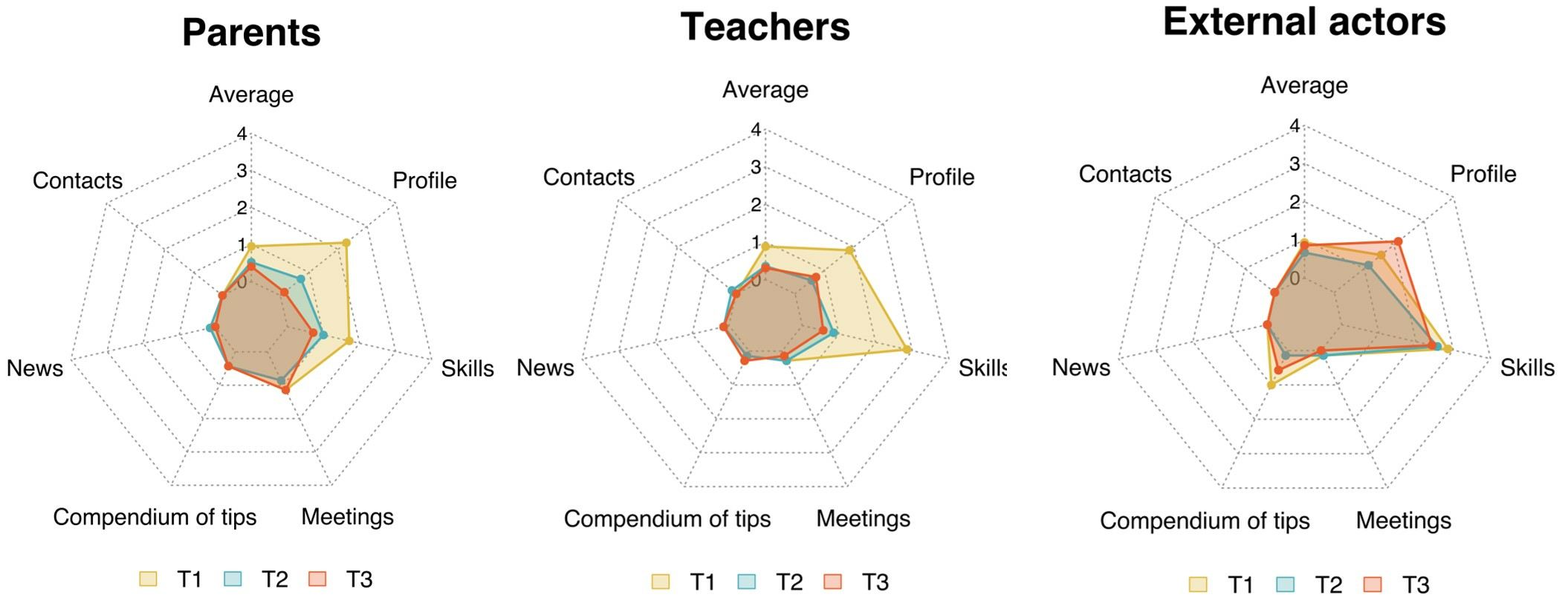
Perceived difficulty answers’ distribution depending on the role, the assessed tab and the test instance on a scale from 0 (very easy) to 10 (very difficult)
From those results, user testing for the new release of ToGather appears conclusive 69 (under review).
Transferability of the tool to non-French socio-educational context
With the support of the Clinical Speech Therapy Unit of Liège, we are currently replicating the user study in Belgium to explore the applicability of ToGather tool. The study is currently being finalized. The first results, obtained from 16 participants, are very similar to those obtained in France: 1) Usability is good, 2) Cognitive load is low, 3) Self-determination is high. In terms of user experience, measured with the User Experience Questionnaire, the pragmatic qualities and attractiveness are high, which allows us to conclude that the user experience is good in the eyes of the Belgian audience. The applicability study in Belgium is expected to be completed in early 2023, with additional participants. The interaction data of the participants with the mock-up still needs to be analyzed to extract data related to objective usability (effectiveness and efficiency). Nevertheless, preliminary results on subjective usability data (satisfaction) are very encouraging for the transferability of the ToGather tool to the Belgian context.
The next part of the project has two goals: to validate its added value through a controlled and randomized field study evaluating the impact on the student (user experience, academic success, school-related well-being and motivation) and their caregivers (self-efficacy, perception of school inclusion, perceived health, communication quality etc.) A pre-study has already been conducted during the last trimester of the 2021-2022 school year with 12 groups of persons supporting a pupil. Half of them were using ToGather app on a weekly basis. The results show some differences between T1 and T2, particularly for perceived burden and well-being. Although some results are encouraging, the pre-study does not allow us to conclude on the evolution of all the variables. It is therefore necessary, after some adjustments, to continue the experimentation with a larger population. For this reason, a study is being conducted with a larger sample of groups, until June 2023.
To better understand the current collaboration practices, semi-structured interview were conducted with participants from the control group. Interviews were recorded and we are currently developing a solution to automatically transcribe those recordings. This software is based on open source neural networks (whisper, pyannote) able to transform speech into text and detect the speaker changes. The user has access to a web interface to easily use the models on its record files. The texts thus obtained can be viewed and corrected through the software. Interface have been created for the tool to be easily adoptable by other researchers.
This project is in partnership with the School Academy of Bordeaux of the French Education Minestery, the ARI association, the Centre of Autism of Aquitaine. It is funded by the FIRAH (foundation) and the Nouvelle-Aquitaine Region.
8.6.5 Curious and therefore not overloaded : Study of the links between curiosity and cognitive load in learning mediated by immersive technologies
Participants: Hélène Sauzéon [correspondant], Matisse Poupard, André Tricot [Cosupervisor - Univ. Montpellier], Florian Larrue [Industrialist - Le Catie].
With the ever-increasing interest in digital technologies in education, many questions about their use and effectiveness are emerging. In this context, this thesis will focus on the relationships between three key dimensions of technology-mediated learning: the learner's internal learning processes, the instructional design, and the educational technology used. In partnership with CATIE (industrial partner) and the EPSYLON laboratory of the University of Montpellier (PR. André Tricot), two main objectives are targeted in this research program started in April 2022:
- To relate the theory of cognitive load to models of curiosity -driven learning;
- To experiment the impact of the choice of educational technology on the links between pedagogical choice (guided instruction vs. exploration) and learner expertise, by studying the reversal effect due to expertise.
To this end, the program includes 3 main phases of study:
State of the art
A systematic review on the learning benefits related to an improvement of the cognitive load (CL) and the curiosity states (CS) elicited by immersive technologies such as virtual reality and augmented reality. The planned systematic review is currently submitted to Computer & Education journal 160. Its main results are as follows: From 2802 studies, we selected only 31 studies with a reliable study-design for investigating the impact of Virtual Reality (VR) and/or Augmented Reality (AR) on learning performance with respect to measurements of cognitive load and/or curiosity state. To this end, we built an analytical grid for probing positive, negative, null, or uninterpretable relationships between the learning performance and CL or CS measures. The 24 studies focusing on CL show that the immersive Technology (imT) benefit for learning depends on technology with CL advantage for AR and with CL disadvantage for VR. For the 15 studies with a focus on CS, the results are inconclusive and inconsistent due to large methodological differences in measuring this facet of the learning experience. Of the 8 studies investigating both CL and CS, very few studies documented causal links between these two learning-related constructs, and the reported results were contradictory. We examined the role of variables such as the type of knowledge taught, the type of learning, or the level of prior knowledge of the learners, but they did not yield significant insight into the relationships between the imT-learning performance and the learner experience in terms of CL and/or CS. Hence further works have to be conductef for an understanding of links between CL and CS into learning activities performed with educational technologies.
Experimentation
Three experiments will be conducted in which we will vary the level of learner expertise, the instructional design and the educational technology used (Video, Virtual Reality and Augmented Reality).
Transfer
We hope to extend the results obtained to the industrial context in which CATIE's activities are carried out. CATIE's mission is to accelerate technology transfer between the worlds of research and industry. The Human Centered Systems team, in which this research project is part of, supports companies in improving the design of existing or new digital systems, by proposing a human-centered approach. The different questions raised by this project are intended to help CATIE to answer these issues, to improve its know-how in terms of learning and digital systems, and then to transfer this knowledge to EdTech companies.
8.7 Applications to Automated Scientific Discovery in Self-Organizing Systems
8.7.1 Design of an Interactive Software for Automated Discovery in Complex Systems
Participants: Clément Romac [correspondant], Jesse Lin, Mathieu Périé, Mayalen Etcheverry, Clément Moulin-Frier, Pierre-Yves Oudeyer.
We further developed our Automated Discovery software and started experimenting with it. First, we worked on improving our standalone Python library allowing users to save their experiments and reload them. We also now provide tools allowing users to log information during experiments and easily retrieve them.Secondly, this year was mostly focused on moving our software towards large-scale experiments and fostering human-in-the-loop interactions with automated discovery algorithms. For the former, we now allow users to run experiments on remote servers through the use of simple configuration files. Our software then seamlessly communicates with the distant server, sends the code necessary for the experiment, launches the experiment and retrieves results while the experiment is running allowing users to still monitor in quasi-real-time. Our method supports any server, including SLURM clusters commonly used for large-scale experiments.
We also focused our efforts on implementing tools and interfaces for users to give feedback or instructions to the automated discovery algorithm that explores the complex system. As identified by 16, empowering experiments to collaborate with automated discovery methods can be key to obtain interesting discoveries. Integrating such a collaborative process in our tool came with several engineering challenges and we are currently experimenting with our solution and working on making it user-friendly for non-experts end users.
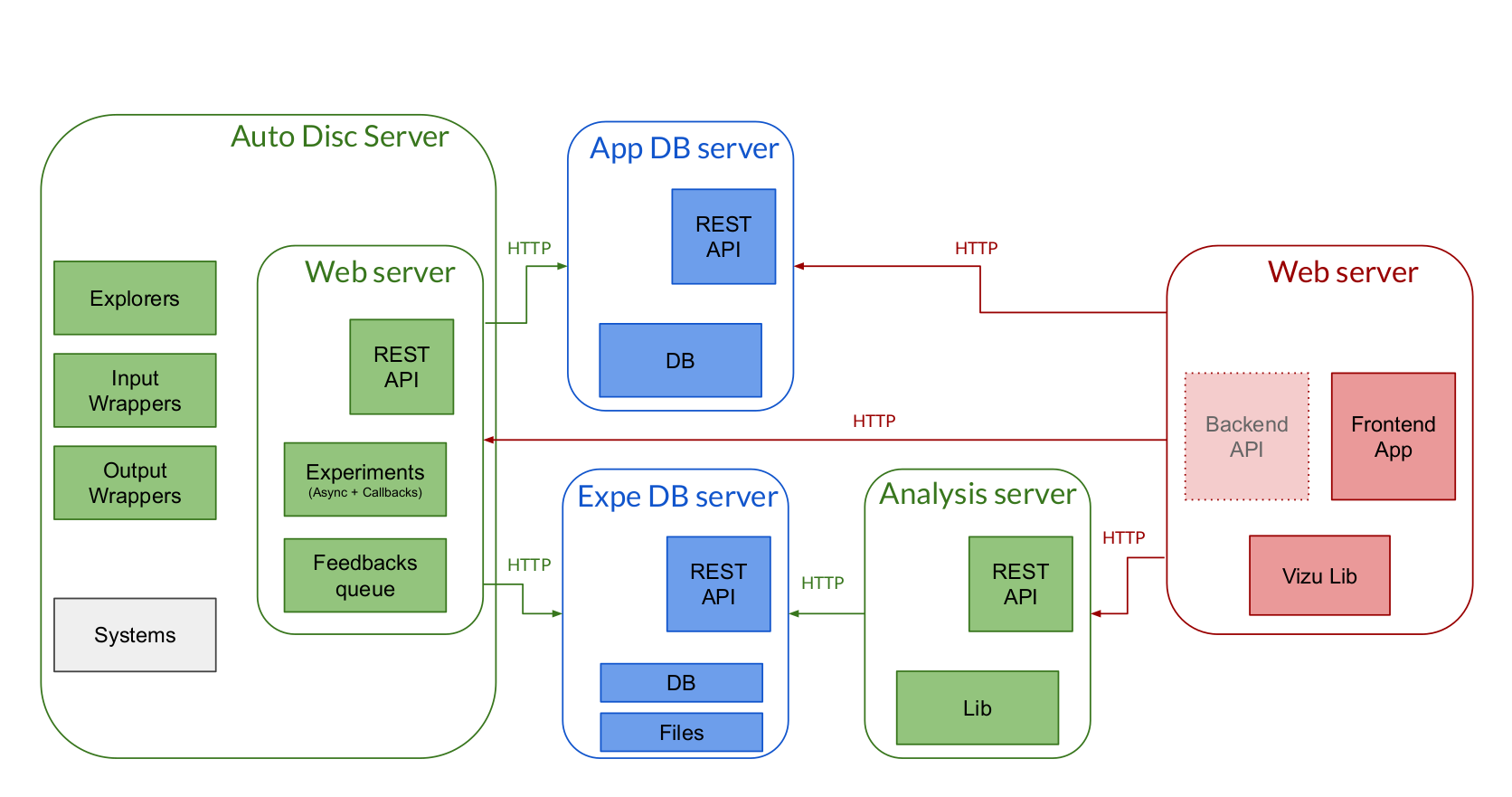
8.7.2 Learning Sensorimotor Agency in Cellular Automata
Participants: Gautier Hamon [correspondant], Mayalen Etcheverry, Bert Chan, Clément Moulin-Frier, Pierre-Yves Oudeyer.
As a continuation of the previous projects in 8.7, we have been working on expanding the set of discoveries of possible structures in continuous CAs such as Lenia 95, 94, and in particular we have been interested to search for emerging agents with sensorimotor capabilities. Understanding what has led to the emergence of life and sensorimotor agency as we observe in living organisms is a fundamental question. In our work, we initially only assume environments made of low-level elements of matter (called atoms, molecules or cells) locally interacting via physics-like rules. There is no predefined notion of agent embodiment and yet we aim to answer the following scientific question: is it possible to find environments in which there exists/emerge a subpart that could be called a sensorimotor agent?We use Lenia continuous cellular automaton as our artificial "world" 94. We introduce a novel method based on gradient descent and curriculum learning combined within an intrinsically-motivated goal exploration process (IMGEP) to automatically search parameters of the CA rule that can self-organize spatially localized 1 and moving patterns 2 within Lenia. The IMGEP defines an outer exploratory loop (generation of training goal/loss) and an inner optimization loop (goal-conditioned). We use a population-based version of IMGEP 17, 104 but introduce two novel elements compared to previous papers in the IMGEP literature. First, whereas previous work in 30 and 16 used a very basic nearest-neighbor goal-achievement strategy, our work relies on gradient descent for the local optimization of the (sensitive) parameters of the complex system, which has shown to be very powerful. To do so we made a differentiable version of the Lenia framework, which is also a contribution of this work. Secondly, we propose to control subparts of the environmental dynamics with functional constraints (through predefined channels and kernels in Lenia) to build a curriculum of tasks; and to integrate this stochasticity in the inner optimization loop. This has shown central to train the system to emerge sensorimotor agents that are robust to stochastic perturbations in the environment. In particular, we focus on modeling obstacles in the environment physics and propose to probe the agent sensorimotor capability as its performance to move forward under a variety of obstacle configurations. We also provide in this work tests and metrics to measure the robustness of the obtained agents.
![]() |
![]() |
![]() |
![]() |
![]() |
![]() |
![]() |
While many complex behaviors have already been observed in Lenia, among which some could qualify as sensorimotor behaviors, they have so far been discovered "by chance" as the result of time-consuming manual search or with simple evolutionary algorithms. Our method provides a more systematic way to automatically learn the CA rules leading to the emergence of basic sensorimotor structures, as shown in Figure 32. Moreover, we investigated and provided ways to measure the (zero-shot) generalization of the discovered sensorimotor agents to several out-of-distribution perturbations that were not encountered during training. Impressively, even though the agents still fail to preserve their integrity in certain configurations, they show very strong robustness to most of the tested variations. The agents are able to navigate in unseen and harder environmental configurations while self-maintaining their individuality (Figure 30). Not only the agents are able to recover their individuality when subjected to external perturbations but also when subjected to internal perturbations: they resist variations of the morphogenetic processes such that less frequent cell updates, quite drastic changes of scales as well as changes of initialization (Figure 31). Furthermore, when tested in a multi-entity initialization and despite having been trained alone, not only the agents are able to preserve their individuality but they show forms of coordinated interactions (attractiveness and reproduction). Our results suggest that, contrary to the (still predominant) mechanistic view on embodiment, biologically-inspired embodiment could pave the way toward agents with strong coherence and generalization to out-of-distribution changes, mimicking the remarkable robustness of living systems to maintain specific functions despite environmental and body perturbations 133. Searching for rules at the cell-level in order to give rise to higher-level cognitive processes at the level of the organism and at the level of the group of organisms opens many exciting opportunities to the development of embodied approaches in AI in general.
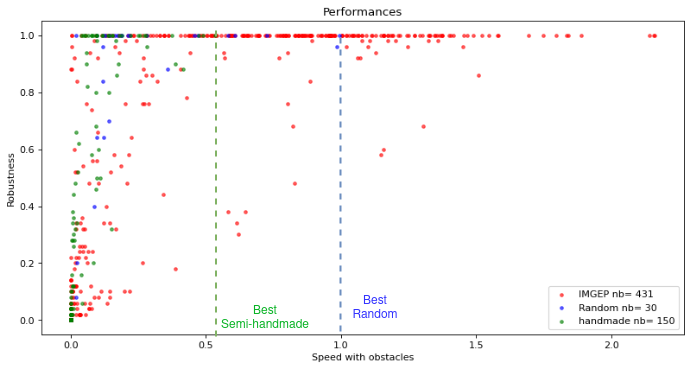
Scatter plot of the agents as their measured performances of robustness to obstacles (y axis) and speed in obstacles (x axis) obtained by IMGEP (red), random search with the same compute resources as IMGEP(blue) and the one from the original lenia paper (green)
The work has been released as a distill-like article which is currently hosted at. This article contains an interactive demo in webGL and javascript, as well as many videos and animations of the results. A colab notebook with the source code of the work is publicly available at. We are currently working on a journal publication.
8.7.3 Flow lenia: Mass conservation for the study of virtual creatures in continuous cellular automata
Participants: Erwan Plantec, Gautier Hamon [correspondant], Mayalen Etcheverry, Pierre-Yves Oudeyer, Clément Moulin-Frier, Bert Chan.
Following our work on trying to find sensorimotor cabapabilities in cellular automata such as Lenia 95, 94, we kept exploring the search for low level cognition in continuous cellular automata. This led to preliminary search on trying to emerge memory in self-organizing agents as well as work on trying to implement other environmental constraints in the CA in order to emerge interesting behavior. To implement more easily those environmental constraints as well as to ease the emergence of spatially localized patterns (and thus have the optimization/search to focus more on the cognitive ability, removing the need to optimize to prevent uncontrollable growth/explosion of the pattern), we worked on adding mass conservation to the Lenia system.
We propose in this work a mass-conservative (i.e the sum of the CA’s activations remains constant over time) extension to Lenia called Flow Lenia 68. We hypothesize that such conservation laws will help in the search for artificial life-forms by constraining emerging patterns to spatially localized ones. It also allows to implement more easily environmental constraints on the self-organizing agents such as a need for food to grow etc.
Furthermore, we show that this new model allows for the integration of the update rule parameters within the CA dynamics enabling the emergence of creatures with different parameters and so different properties in the same environment/grid. This leads to multi-species simulation where the grid is filled with agents with different behaviors and properties 33. Such a feature opens up research perspectives towards the achievement of open-ended intrinsic evolution inside continuous CAs, which means that all the evolutionary part would be a result of the dynamic of the CA (without any external loop/system). We hypothesize that this open-ended instrinsic evolution could, through the competition/cooperation, lead to the emergence of interesting low level cognition in those system.
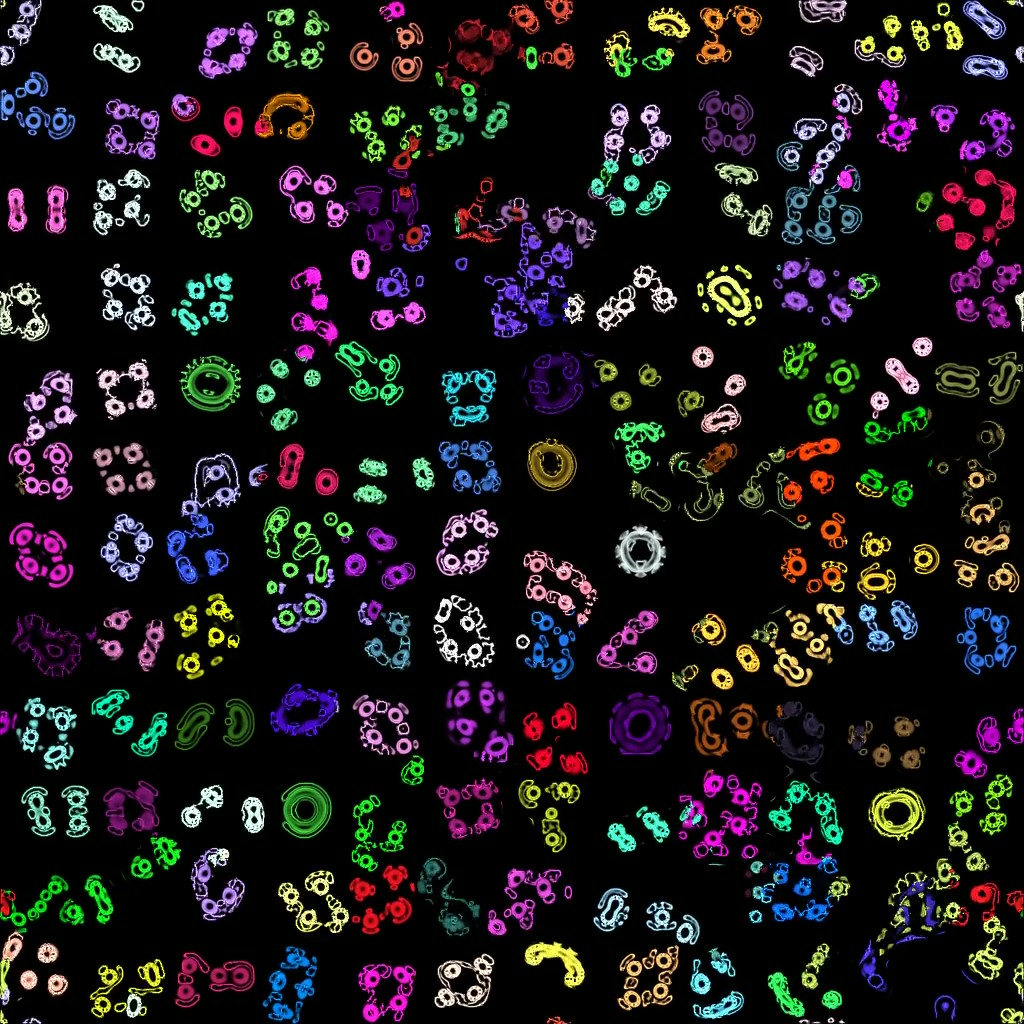
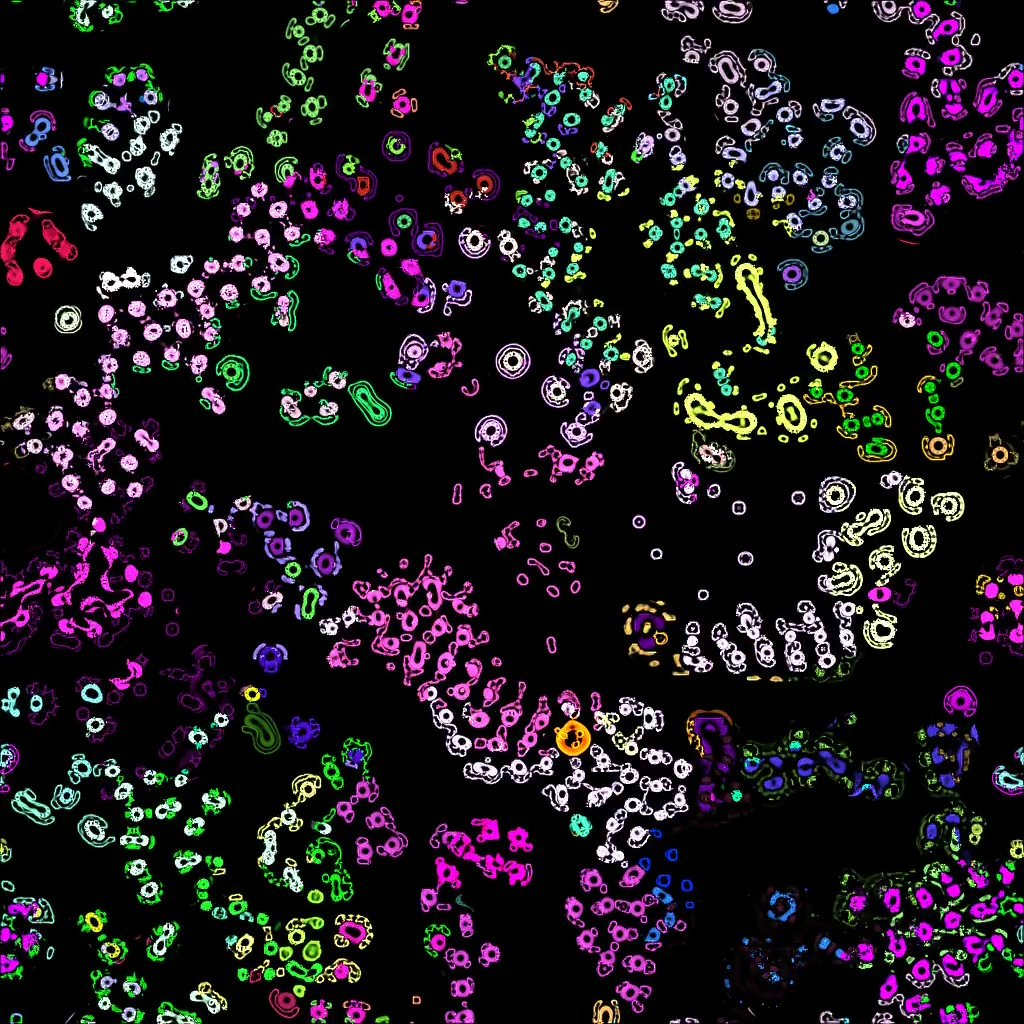
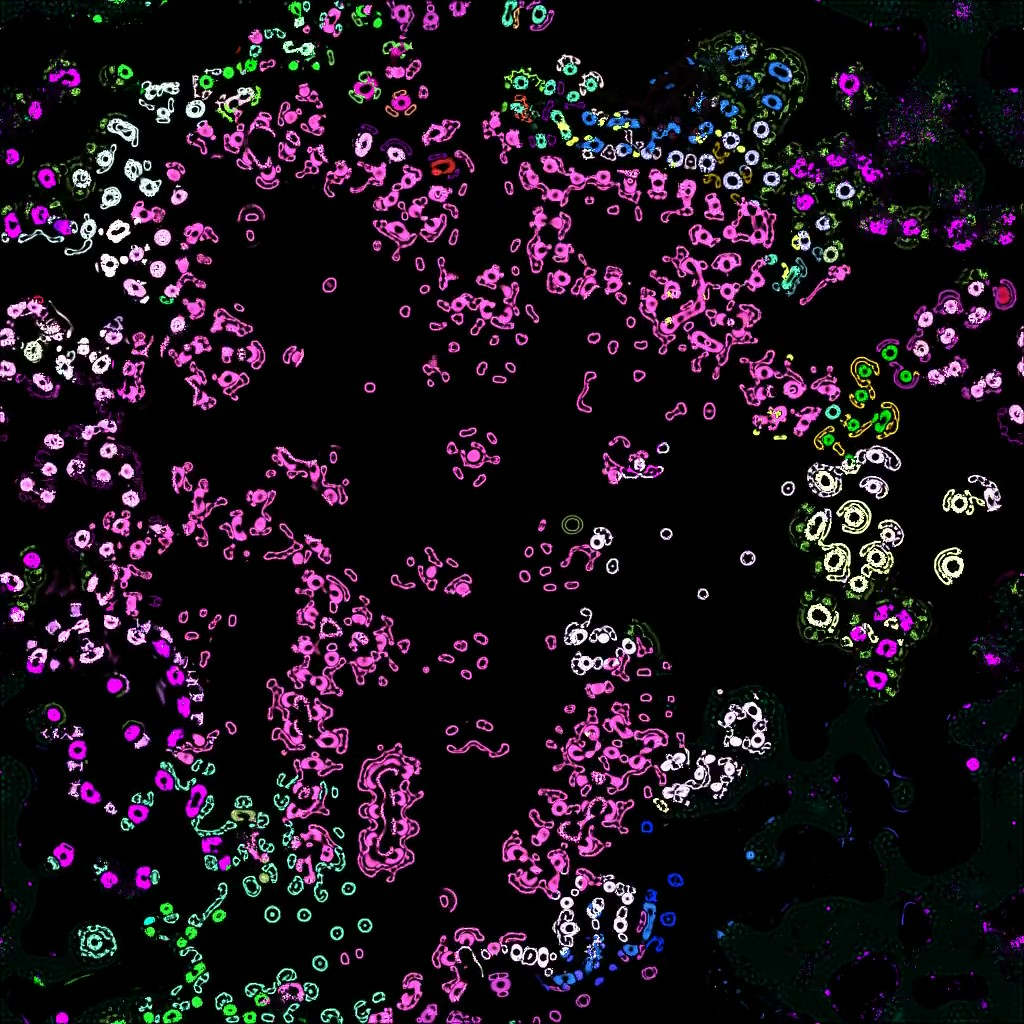
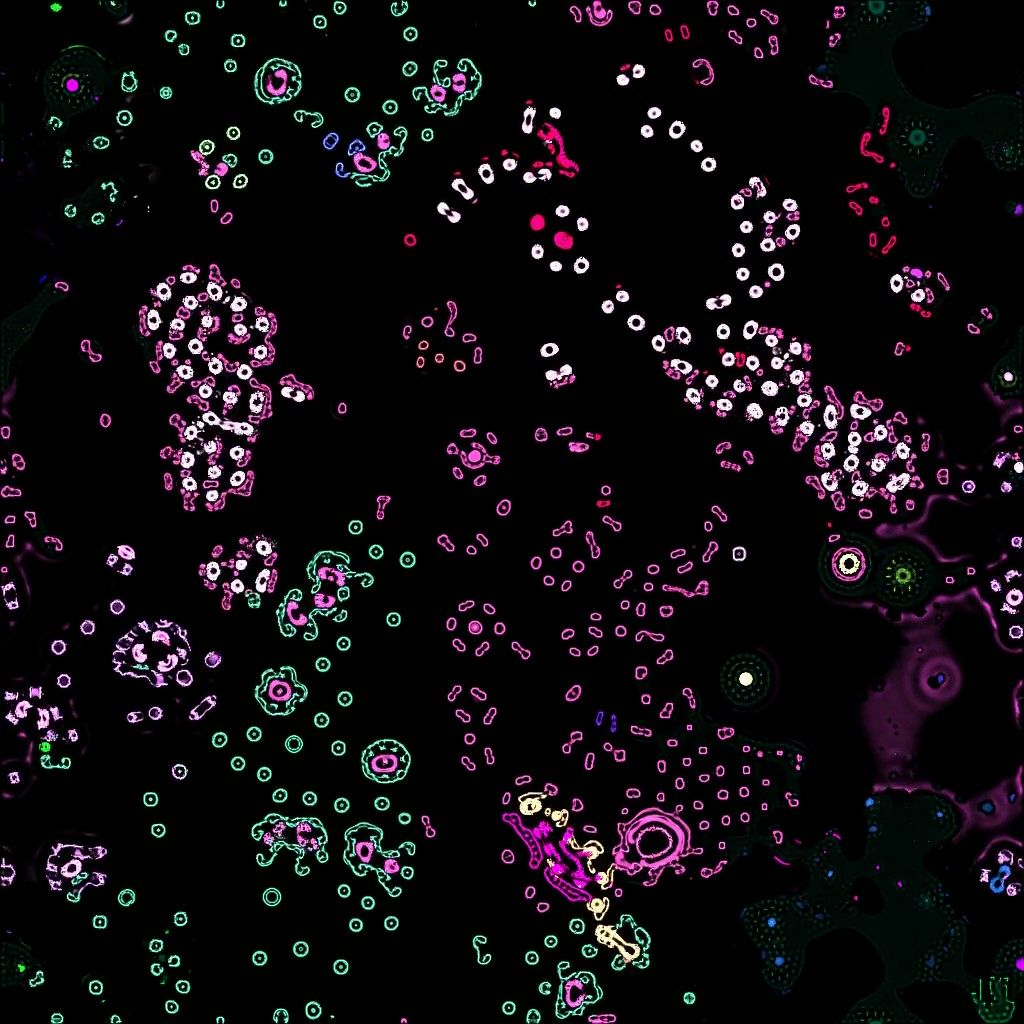
Multi-species simulation in Flow Lenia where each colour represents different values of parameters. Left to right shows the evolution of the system over time with some species stealing the mass of others.: Multi-species simulation in Flow Lenia where each colour represents different parameters. Left to right shows the evolution of the system over time with some species stealing the mass of others.
Multi-species simulation in Flow Lenia where each colour represents different values of parameters. Left to right shows the evolution of the system over time with some species stealing the mass of others.: Multi-species simulation in Flow Lenia where each colour represents different parameters. Left to right shows the evolution of the system over time with some species stealing the mass of others.
Multi-species simulation in Flow Lenia where each colour represents different values of parameters. Left to right shows the evolution of the system over time with some species stealing the mass of others.: Multi-species simulation in Flow Lenia where each colour represents different parameters. Left to right shows the evolution of the system over time with some species stealing the mass of others.
Multi-species simulation in Flow Lenia where each colour represents different values of parameters. Left to right shows the evolution of the system over time with some species stealing the mass of others.: Multi-species simulation in Flow Lenia where each colour represents different parameters. Left to right shows the evolution of the system over time with some species stealing the mass of others.
Simple evolutionary strategy (with an evolutionary loop outside the system) was also used to optimized for pattern with directional and rotational movement.
You can find some examples of the system and pattern in this companion website, including the ones trained for movement, random parameters, food in flow Lenia, and multi species simulations: see. Notebook with the system can be found : here.
This work led to an oral presentation to the WIVACE 2022, 15th International Workshop on Artificial Life and Evolutionary Computation.
Collaboration with Bert Chan
In the context of the project 8.7, we have an ongoing collaboration with Bert Chan, a previously independant researcher on Artificial Life and author of the Lenia system 95, 94 and who is now working as a research engineer at Google Brain. During this collaboration, Bert Chan help us design versions of IMGEP usable by scientists (non ML-experts) end-users, which is the aim of project 8.7.1. Having himself created the Lenia system, he is highly-interested to use our algorithms to automatically explore the space of possible emerging structures and provides us valuable insights into end-user habits and concerns. Bert Chan also co-supervised with Mayalen Etcheverry the master internship of Gautier Hamon which led to the work described in section 8.7.2. He also co-supervised with Gautier Hamon and Mayalen Etcheverry the master internship of Erwan plantec which led to the work described in section 8.7.3.
8.7.4 Use of Automated Discovery Tools for the study of Goal-Directedness and Navigation Competency in Models of Gene Regulatory Networks
Participants: Mayalen Etcheverry [correspondant], Clément Moulin-Frier, Pierre-Yves Oudeyer, Michael Levin.
Previous work around project 8.7 has shown that modern tools that leverage computational models of curiosity developed in the Flowers team can be transposed to form efficient AI-driven “discovery assistants” that assist scientists in mapping and navigating the space of possible outcomes in complex systems 30, 16, 78. In particular, recent work from project 8.7.2 has shown how these tools can be very useful to study the origins of sensorimotor agency in continuous cellular automata models. This work is part of the emerging effort to study the fundamental mechanisms that enable the emergence of agency and cognition across all (and unconventional) domains of biological and synthetic life, which is known as the field of basal cognition 141. In particular, the lab of Dr. Michael Levin at Tufts University is pioneer in the field of basal cognition and has shown that it holds great promises to open pioneering opportunities for top down control in synthetic and regenerative biology 138. Discussions with Michael Levin has highlighted the great opportunity to leverage curiosity-driven exploration algorithms as tools to empower scientific exploration and analysis of basal cognition in numerical models of biological systems. Hence we have started a collaboration with his Lab, under the form of a 5 month academic exchange of Mayalen Etcheverry in their lab in Boston.
The objective of this collaboration is to develop a new machine-learning-based toolkit to study goal-directedness and navigation competency in self-organizing systems, more particularly focusing the research program on the analysis of navigation competency 114 in numerical models of gene regulatory networks (GRN).
GRN are molecular network that describe how genes in a cell influence the activation/inhibition of others by means of their end product. Those network of interactions govern protein synthesis and hence cellular functions. Understanding the dynamics of these pathways is a central challenge for the design of effective strategies for bio-medicine and bio-engineering.
In this project, we aim to study the behavioral competencies of these molecular networks in navigating the transcriptional space, i.e. the space of gene expression. In particular we are interesting in evaluating the goal-directedness of these molecular networks and their robustness to a distribution of perturbations (dynamical noise, pushes, energetical barrier, structural changes in the network, etc). To do so, we propose to use intrinsically motivated goal exploration processes (IMGEPs) to automatically organize the exploration of initial GRN states that will lead to diverse final states (goals) in the transcriptional space.
To that end, we have worked on the design of two software packages: AutoDiscJax and SBMLtoODEJax. AutoDiscJax is a python software built upon jax, that allows to perform automated discovery and exploration of complex systems and that we use to organize the exploration of computational models of biological GRNs. SBMLtoODEJax is a python package which allows to convert Systems Biology Markup Language (SBML) models into python classes that can then easily be simulated and manipulated in python projects. SBMLtoODEJax makes use of jax mains features which allows: just-in-time compilation and automatic vectorization, hence parallel computing and speed-ups, as well as automatic differentiation and hence gradient-descent based optimization. Those codebases are at the moment private but will be publicly released in 2023.
After having defined an experimental framework to quantitatively study the navigation competency and goal-directedness in gene regulatory networks, we have preliminary results on the ability of certain networks to reach goals in the transcriptional space under a variety of perturbations. Future work will consist in running experimental campaign on a larger database of GRN models and will hopefully lead to a scientific publication.
8.8 Other
8.8.1 Language-biased image classification: evaluation based on semantic representations
Participants: Yoann Lemesle, Masataka Sawayama, Guillermo Valle-Perez, Maxime Adolphe, Hélène Sauzéon, Pierre-Yves Oudeyer.
Humans show language-biased image recognition for a word-embedded image, known as picture-word interference. Such interference depends on hierarchical semantic categories and reflects that human language processing highly interacts with visual processing. Similar to humans, recent artificial models jointly trained on texts and images, e.g., OpenAI CLIP, show language-biased image classification. Exploring whether the bias leads to interference similar to those observed in humans can contribute to understanding how much the model acquires hierarchical semantic representations from joint learning of language and vision 34.
In this work 48, 75, we introduced methodological tools from the cognitive science literature to assess the biases of artificial models. Specifically, we introduce a benchmark task to test whether words superimposed on images can distort the image classification across different category levels and, if it can, whether the perturbation is due to the shared semantic representation between language and vision. Our dataset is a set of word-embedded images and consists of a mixture of natural image datasets and hierarchical word labels with superordinate/basic category levels. Using this benchmark test, we evaluate the CLIP model. We show that presenting words distorts the image classification by the model across different category levels, but the effect does not depend on the semantic relationship between images and embedded words. This suggests that the semantic word representation in the CLIP visual processing is not shared with the image representation, although the word representation strongly dominates for word-embedded images.
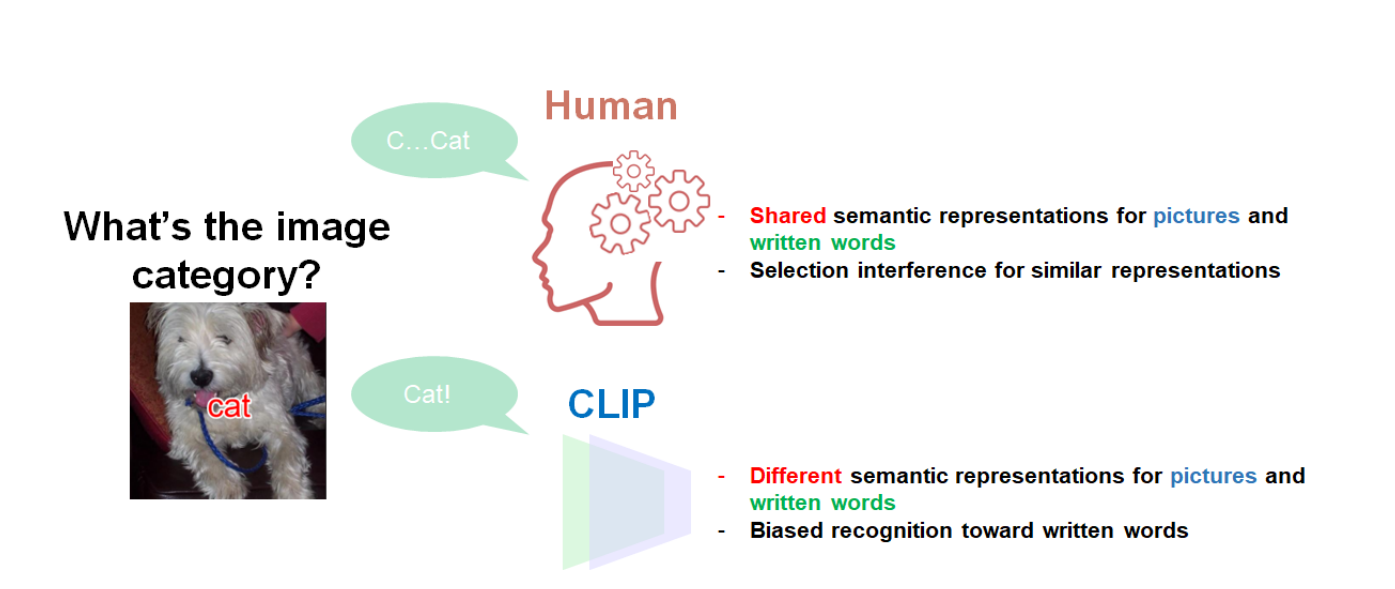
8.8.2 Towards locally adaptive model based deep reinforcement learning
Participants: Rémy Portelas [correspondant], Harm van Seijen, Ali-Rahimi Kalahroudi, Pierre-Yves Oudeyer.
In this project in collaboration with Harm van Seijen and Ali-Rahimi Kalahroudi from Microsoft Research Montréal, we focused on “local adaptivity”, which is a measure defining how quickly an RL method adapts to a local change in the environment. This metric, presented by Harm van Seijen and colleagues in 178 enables to identify model-based behavior and provides insight in how close a method's behavior is from optimal model-based behavior. To enable the study of their proposed Deep RL method showed to be adaptive in simple settings in more complex environments, we created LoCa Coinrun, a modified coinrun environment well-suited for local adaptivity tests. We then performed baseline experiments with PPO, a model-free agent, and EfficientZero, a model-based agent without adaptivity mechanisms, and showed that they both failed to learn in LoCa Coinrun. Next experiments will aim to scale their adaptive approach to LoCa Coinrun.
9 Bilateral contracts and grants with industry
9.1 Bilateral contracts with industry
Research on lifelong Deep Reinforcement Learning of multiple tasks (Microsoft
Participants: Pierre-Yves Oudeyer [correspondant], Laetitia Teodorescu.
Financing of the PhD grant of Laetitia Teodorescu.
Automated Discovery of Self-Organized Structures (Poïetis)
Participants: Pierre-Yves Oudeyer [correspondant], Mayalen Etcheverry.
Financing of the CIFRE PhD grant of Mayalen Etcheverry by Poietis.
Machine learning for adaptive cognitive training (OnePoint)
Participants: Hélène Sauzéon [correspondant], Pierre-Yves Oudeyer, Maxime Adolph.
Financing of the CIFRE PhD grant of Maxime Adolphe by Onepoint.
Curiosity-driven interaction system for learning (evidenceB)
Participants: Hélène Sauzéon [correspondant], Pierre-Yves Oudeyer, Rania Abdelghani.
Financing of the CIFRE PhD grant of Rania Abdelghani by EvidenceB.
Curious and therefore not overloaded : Study of the links between curiosity and cognitive load in learning mediated by immersive technologies (CATIE)
Participants: Hélène Sauzéon [correspondant], Matisse Poupard, André Tricot [Cosupervisor - Univ. Montpellier], Florian Larrue [Industrialist - Le Catie].
Financing of a PhD grant of Matisse Poupard with CATIE and EPSYLON Lab (Univ. Montpellier).
Augmenting curiosity-driven exploration with very large language models in deep reinforcement learning agents (Hugging Face)
Participants: Pierre-Yves Oudeyer [correspondant], Clément Romac, André Tricot [Cosupervisor - Univ. Montpellier], Florian Larrue [Industrialist - Le Catie].
Financing of the PhD grant of Clément Romac by Hugging Face.
Autonomous Driving Commuter Car (Renault)
Participants: David Filliat [correspondant], Emmanuel Battesti.
We developed planning algorithms for a autonomous electric car for Renault SAS in the continuation of the previous ADCC project. We improved our planning algorithm in order to go toward navigation on open roads, in particular with the ability to reach higher speed than previously possible, deal with more road intersection case (roundabouts), and with multiple lane roads (overtake, insertion...).
9.2 Bilateral contracts with industry
We received a 30keuros grant from Google Brain, as well as 30keuros Google cloud credit, for developing projects on automated exploration of continuous cellular automata.
9.3 Bilateral Grants with Fundation
School+ /ToGather project (FIRAH and Region Nouvelle-Aquitaine)
Participants: Hélène Sauzéon [correspondant], Cécile Mazon, Isabeau Saint-supery, Eric Meyer.
Financing of one year-postdoctoral position and the app. development by the International Foundation for Applied Research on Disability (FIRAH). The School+ project consists of a set of educational technologies to promote inclusion for children with Autism Spectrum Disorder (ASD). School+ primary aims at encouraging the acquisition of socio-adaptive behaviours at school while promoting self-determination (intrinsic motivation), and has been created according to the methods of the User-Centered Design (UCD). Requested by the stakeholders (child, parent, teachers, and clinicians) of school inclusion, Flowers team works to the adding of an interactive tool for a collaborative and shared monitoring of school inclusion of each child with ASD. This new app will be assessed in terms of user experience (usability and elicited intrinsic motivation), self-efficacy of each stakeholder and educational benefit for child. This project includes the Academie de Bordeaux –Nouvelle Aquitaine, the CRA (Health Center for ASD in Aquitania), and the ARI association.
10 Partnerships and cooperations
10.1 International initiatives
10.1.1 International Programs
Idex mobility program- Univ. Of Bordeaux
Participants: Pierre-Yves Oudeyer, Hélène Sauzéon, Edith Law, Myra Fernandes.
-
Title:
Curiosity-driven learning and personalized (re-)education technologies across the lifespan
-
Partner Institution(s):
- University of Bordeaux, France
- University of Waterloo, Canada
-
Date/Duration:
2019-2021 -Prolonged in 2022 (20 000€)
-
Additionnal info/keywords
: Interactive systems, education, curiosity
MITACS mobility program
Participants: Hélène Sauzéon, Myra Fernandes, Yadurshana Sivashankar.
-
Title:
Curiosity-driven spatial learning across the lifespan
-
Partner Institution(s):
- Inria, France
- University of Waterloo, Canada
-
Date/Duration:
2021-2022 (6000$)
-
Additionnal info/ keywords
: Intrinsic motivation, spatial learning, children, older adults.
10.1.2 Other
The FLOWERS has initiated or is continuing international collaborations, including:
- Collaboration with Bert Chan (Google Brain Tokyo, Japan) on curiosity-driven algorithms applied to continuous cellular automata (sections 8.7.2, 8.7 and 8.7.3).
- Collaboration with Michael Levin (Tufts and Harvard University, USA) on curiosity-driven algorithms applied to the control of gene regulatory networks (section 8.7.4)
- Collaboration with Ida Momennejad (Microsoft Research NYC, USA) on the role of social network structures in collective innovation (section 8.5.3)
- Clément Moulin-Frier is collaborating with Marti Sanchez-Fibla and Ricard Solé (University Pompeu Fabra, Barcelona, Spain) on the cooperative control of environmental extremes by artificial intelligent agents. A preprint is available on Arxiv 163 (soon on HAL) and the paper will be soon submitted to a journal.
10.1.3 Visits to international teams
Research stays abroad
Pierre-Yves Oudeyer
-
Visited institution:
Microsoft Research Montréal
-
Country:
Canada
-
Dates:
Sept 21 - June 22
-
Context of the visit:
The objective was to develop new projects and collaborations at large with both MSR and the larger AI ecosystem in Montreal, in particular on topics at the crossroads of developmental AI and large language models. This was implemented through the "detachement" scheme.
Rémy Portelas
-
Visited institution:
Microsoft Research Montréal
-
Country:
Canada
-
Dates:
March 15 - September 7
-
Context of the visit:
The objective of this mission was to deepen a research theme in the continuity of Rémy Portelas' thesis, and to develop new relationships between the team and the AI research ecosystem in Montreal. This postdoctoral mission was in synergy with Pierre-Yves Oudeyer's stay in Montreal and aimed to set up new collaborations and partnerships. More specifically, the mission focused on the analysis and improvement of the generalization capacities of autonomous agents learning by deep reinforcement learning, themes which were studied with Harm Von Seijen (Microsoft / MILA / Univ. McGill) and Maxime Gasse (MILA / Polytech. Montreal). An ongoing contribution is the adaptation of a recent test platform, named ProcGen. The objective is to make the procedural generation of ProcGen environments controllable, which will make it possible to study the contribution of adaptive curriculum in learning. Contributions also included the participation in an ongoing project revolving around the study of adaptivity in model-based deep reinforcement learning.
-
Mobility program/type of mobility:
Research stay
Laetitia Teodorescu
-
Visited institution:
Microsoft Research (MSR) Montreal
-
Country:
Canada
-
Dates:
April 21 - July 13th
-
Context of the visit:
The objective of this research visit (in synergy with Pierre-Yves Oudeyer's visit at MSR Montreal) was to open up a collaboration between a sub-team of MSR Montreal and Inria on the subject of autotelic text agents. In particular, a collaboration with Marc-Alexandre Côtánd Eric Yuan and Laetitia Teodorescu was begun, which is still onging; and another project involving the same participants and additionally Cédric Colas, former member of the Flowers team, now at MIT. The research stay focused on studying different exploration drivers (inspired by the go-explore framework and previous work in the team) on text agents. Text agents are RL agents evolving in textual environments and are a prime way of studying language-conditioned agents while sidestepping the problem of language grounding.
-
Mobility program/type of mobility:
Research stay
Mayalen Etcheverry
-
Visited institution:
the Levin Lab, Allen Discovery Center, Tufts University, Boston
-
Country:
USA
-
Dates:
August 15 - December 31
-
Context of the visit:
The objective of this research visit was to open up a collaboration between the Levin Lab and Inria on the subject of automated discovery in numerical and/or in-vitro models of basal cognition. In particular, a collaboration with Michael Levin, Clément Moulin Frier, Pierre-Yves Oudeyer and Mayalen Etcheverry was begun, which is still onging. The research stay focused on studying how curiosity-driven exploration algorithms can serve as discovery assistants to better understand the mechanisms behind the origin and self-regulation of goals in biological systems, as well as how those mechanisms influence the overall information processing of the system in space and time. In a broader longer-term perspective, the project aim to contribute to develop computational tools to assist next-generation regenerative medicine approaches in health sciences and technology.
-
Mobility program/type of mobility:
Research stay
10.2 European initiatives
10.2.1 Horizon Europe
INTERACT
INTERACT project on cordis.europa.eu
-
Title:
Help Me Grow: Artificial Cognitive Development via Human-Agent Interactions Supported by New Interactive, Intrinsically Motivated Program Synthesis Methods.
-
Duration:
From October 1, 2022 to September 30, 2025
-
Partners:
- INSTITUT NATIONAL DE RECHERCHE EN INFORMATIQUE ET AUTOMATIQUE (INRIA), France
- MASSACHUSETTS INSTITUTE OF TECHNOLOGY (MIT), United States
-
Inria contact:
Cédric Colas
- Coordinator:
-
Summary:
Building machines that interact with their world, discover interesting interactions and learn open-ended repertoires of skills is a long-standing goal in AI. This project aims at tackling the limits of current AI systems by building on three families of methods: Bayesian program induction, intrinsically motivated learning and human-machine linguistic interactions. It targets three objectives: 1) building autonomous agents that learn to generate programs to solve problems with occasional human guidance; 2) studying linguistic interactions between humans and machines via web-based experiments (e.g. properties of human guidance, its impact on learning, human subjective evaluations); and 3) scaling the approach to the generation of constructions in Minecraft, guided by real players. The researcher will collaborate with scientific pioneers and experts in the key fields and methods supporting the project. This includes supervisors Joshua Tenenbaum (program synthesis, MIT) and Pierre-Yves Oudeyer (autonomous learning, Inria); diverse collaborators, and an advisory board composed of an entrepreneur and leading scientists in developmental psychology and human-robot interactions. The 3rd objective will be pursued via a secondment with Thomas Wolf (CSO) at HuggingFace, a world-leading company in the open source development of natural language processing methods and their transfer to the industry. By enabling users to participate in the training of artificial agents, the project aims to open research avenues for more interpretable, performant and adaptive AI systems. This will result in scientific (e.g. interactive program synthesis approaches), societal (e.g. democratized AI training) and economic impacts (e.g. adaptive AI assistants). The dissemination, communication and exploitation plans support these objectives by targeting scientific (AI, cognitive science), industrial (video games, smart homes) and larger communities (gamers, software engineers, large public).
10.3 National initiatives
ANR Chaire Individuelle Deep Curiosity
- PY Oudeyer continued to work on the research program of this Chaire, funding 2 PhDs and 3 postdocs for five years (until 2025).
ANR JCJC ECOCURL
- C. Moulin-Frier obtained an ANR JCJC grant. The project is entitled "ECOCURL: Emergent communication through curiosity-driven multi-agent reinforcement learning". The project starts in Feb 2021 for a duration of 48 months. It will fund a PhD student (36 months) and a Research Engineer (18 months) as well as 4 Master internships (one per year).
Inria Exploratory Action ORIGINS
- Clément Moulin-Frier obtained an Exploratory Action from Inria. The project is entitled "ORIGINS: Grounding artificial intelligence in the origins of human behavior". The project starts in October 2020 for a duration of 24 months. It funds a post-doc position (24 months). Eleni Nisioti has been recruited on this grant.
Inria Exploratory Action AIDE
- Didier Roy is collaborator of the Inria Exploratory Action AIDE "Artificial Intelligence Devoted to Education", ported by Frédéric Alexandre (Inria Mnemosyne Project-Team), Margarida Romero (LINE Lab) and Thierry Viéville (Inria Mnemosyne Project-Team, LINE Lab). The aim of this Exploratory Action consists to explore to what extent approaches or methods from cognitive neuroscience, linked to machine learning and knowledge representation, could help to better formalize human learning as studied in educational sciences. AIDE is a four year project started middle 2020 until 2024 see.
10.3.1 Adaptiv'Math
- Adaptiv'Math
- Program: PIA
- Duration: 2019 - 2020
- Coordinator: EvidenceB
- Partners:
- EvidenceB
- Nathan
- APMEP
- LIP6
- INRIA
- ISOGRAD
- Daesign
- Schoolab
- BlueFrog
The solution Adaptiv'Math comes from an innovation partnership for the development of a pedagogical assistant based on artificial intelligence. This partnership is realized in the context of a call for projects from the Ministry of Education to develop a pedagogical plateform to propose and manage mathematical activities intended for teachers and students of cycle 2. The role of Flowers team is to work on the AI of the proposed solution to personalize the pedagogical content to each student. This contribution is based on the work done during the Kidlearn Project and the thesis of Benjamin Clement 97, in which algorithms have been developed to manage and personalize sequence of pedagogical activities. One of the main goal of the team here is to transfer technologies developed in the team in a project with the perspective of industrial scaling.
10.4 Regional initiatives
SNPEA (RNA and Inria)
- Associated researcher : Hélène Sauzéon
- Duration: 2020 - 2025
- Amount: 150 000€
- Participants: Pierre-Yves Oudeyer, Masataka Sawayama, Maxime Adolphe
- Description: the project's objective is twofold: 1) to adapt and develop new algorithms in machine learning to the field of attention training and 2) to evaluate with the help of experimental methods from psychology whether automated personalization according to progress generates more responders in elderly and young people with attentional disorders.
Evaluation TousEnsemble ; ToGatherAssessment project (RNA)
- Associated researcher : Hélène Sauzéon
- Duration: 2021 - 2026
- Amount: 57 000€
- Participants: Cécile Mazon; Eric Meyer; Isabeau Saint-Supery; Christelle Maillart (Univ. Liège); Bordeaux Academy of National French education; Centre Ressources Autisme d'Aquitaine; ARI Association.
- Description: this project is the continuation of the Togather app. project. To validate the effectiveness of this new tool, a controlled study (control group vs. equipped group) is planned over 2 to 3 quarters with 60 students (ASD and/or ID). In addition, a study of applicability to the Walloon context will be conducted.
11 Dissemination
11.1 Promoting scientific activities
11.1.1 Scientific events: organisation
Member of the organizing committees
Clément Moulin-Frier co-organized the 3rd SMILES workshop in September 2022. Satellite event of the International Conference on Development and Learning, ICDL (London).
Clément Moulin-Frier and Pierre-Yves Oudeyer organized the 1st workshop on Artificial Intelligence and Archeology at Inria Bordeaux in November 2022.
Clément Moulin-Frier organized a workshop on "The ecology of open-ended skill acquisition" in December 2022, as a satellite event of his HDR defense.
Laetitia Teodorescu, Tristan Karch and Cédric Colas organized the second Language and Reinforcement Learning workshop at the NeurIPS conference in New Orleans, 2022, along with an international team of high-profile students and researchers (MIT, UCL, Mila, DeepMind, MSR Montreal)
PY Oudeyer co-organized the Life, Structure and Cognition symposium 2022, and the Dagstuhl seminar on Developmental Learning.
11.1.2 Scientific events: selection
Reviewer
PY Oudeyer was a reviewer for the RLDM conference
11.1.3 Journal
Member of the editorial boards
PY Oudeyer was member of the editorial board of: IEEE Transactions on Cognitive and Developmental Systems and Frontiers in Neurorobotics.
Reviewer - reviewing activities
- Hélène Sauzéon reviewed one journal paper for Nature Scientific Report
- Cécile Mazon reviewed one journal paper for Education and Information Technology and another one for IEEE Transactions on Affective Computing.
11.1.4 Invited talks
Clément Moulin-Frier gave two invited talks:
- November 2022. “Language, Curiosity, Social Networks And Cultural Innovation”. Workshop Reservoir-SMILES at Inria Bordeaux Sud-Ouest, France.
- October 2022. “Grounding Artificial Intelligence In The Origins Of Human Behavior: The Origins Project”. Annual joint workshop Inria-DFKI at Inria Bordeaux Sud-Ouest, France.
Hélène Sauzéon gave four invited talks:
- HomeAssist technology for aging in place: From technology-design to clinical assessment amongst frail older individuals. INNOVCARE 2nd Annual Forum “Care-led innovation: The case of elderly care in France and Japan”, Fondation France Japon de l'EHESS, December, 7th, Paris, France.
- IA and Education. Annual joint workshop Inria-DFKI at Inria Bordeaux Sud-Ouest, 18- October, 17-19 th, Talence, France.
- Participation in the round table "General state of digital health at the University of Bordeaux", 6th of July, Bordeaux.
- Presentation of scientific advances in the field technologies for mental disability, Audition « Les progrès récents des technologies au service de la prise en charge du handicap à l’Office Parlementaire d’Evaluation des Choix Scientifique et Technologique, vidéos, 27 janvier 2022.
PY Oudeyer gave 2 invited talks:
- Developmental artificial intelligence, at the Life, Structure and Cognition symposium 2022,
- Language and culture internalization in developmental AI, Dagstuhl seminar on Developmental Learning.
Rémy Portelas gave one invited talk at Microsoft Research Montréal on Automatic Curriculum Learning and Deep Reinforcement Learning, on April 24th.
Tristan Karch gave an invited talk at the RL Sofa at Mila (Montreal) in January to present the paper Learning to Guide and to be Guided in the Architect-Builder Problem.
Cédric Colas gave an invited talk at Facebook AI Research Paris, on Vygotskian Autotelic AI in July.
Mayalen Etcheverry gave an invited talk at the ICLR 2022 From Cells to Societies workshop, as well as a panel discussion about self-organization in natural and artificial systems with Alex Mordvintsev & Richard A. Watson.
Cécile Mazon gave an invited talk at the Brain conference NeuroDev (Bordeaux) in May, on digital technologies for the schooling of children with ASD.
11.1.5 Leadership within the scientific community
Flowers’ team members have been highly active withing the scientific community, including the organisation of events, editing journals, reviewing or giving invited talks.
11.1.6 Scientific expertise
- Hélène Sauzéon was a reviewer for the ANRT (2 PhD projects)
- Hélène Sauzéon participated to several Selection committees for permanent positions (e.g., Chaire de professeur junior en Santé & Médecine digitale - solutions digitales pour la sante mentale at the Univ. of Bordeaux).
- PY Oudeyer was a member of the jury selecting grants for PhDs in AI in the context of Plan IA at University of Bordeaux (April 21).
- PY Oudeyer was a member of the advisory board of the BigScience project
- Pierre-Yves Oudeyer and Hélène Sauzéon participated to several Selection committees for permanent positions as researcher (e.g., inria) or assistant professor at the university (2 committee organization for Assistant Professors positions at the Univ. of Bordeaux) , and for young and senior non permanent researchers position at inria (SRP and ARP).
- PY Oudeyer was a member of the hiring committee for the Moex Inria team.
11.1.7 Research administration
- Hélène Sauzéon was the head of Flowers team during the scientific stay of Pierre-Yves Oudeyer, and thus was member of the Project-teams’s committee of the center of Inria of the university of Bordeaux.
- Hélène Sauzéon and Cécile Mazon are members of committee of LILLAB which is a living and learning lab funded by the “délégation interministérielle à la stratégie nationale à l’autisme et troubles neurodéveloppementaux” and aiming the dissemination of knowledge in connection with the 3 centers of excellence for autism and Neurodevelopmental syndromes; since 2020.
- Hélène Sauzéon is member of directory committee of IFHR which is a national institute on disability funded by Inserm aiming the researcher networking and dissemination of knowledge on multidisciplinary research on disability; since 2018.
- Hélène Sauzéon is the head of the Innovations and Transfer Committee of the BIND Center of Excellence in Bordeaux and member of the BIND directory Committee since 2018
- Hélène Sauzéon is a member of extended office of Project-team committee of the centre of Inria of university of Bordeaux, since 2020.
- PY Oudeyer has been head of the team in the september-december period
- PY Oudeyer was member of piloting committees of consortium projects Adaptiv'Maths on educational technologies.
- Cécile Mazon is co-responsible of the workpackage "Digital technologies" of the PIA AspieFriendly
- H. Sauzéon is responsible of Education topic into the centre Inria of the university of Bordeaux
11.2 Teaching - Supervision - Juries
11.2.1 Teaching
Teaching Responsibilities:
- Hélène Sauzéon was director of the curriculum in Technology, Ergonomics, Cognition and handicap (First and Second years of master degree in cognitive Science - University of Bordeaux) until sept. 2022.
- Hélène Sauzéon was in charge of the "Autonomy & Digital" axis of the Bordeaux instanciation of the PIA3 project (2018-22) Aspie-Friendly (P. Monthubert, Univ. Toulouse) aiming to develop digital tools to prepare, support the university inclusion of students with ASD.
- David Filliat is in charge since 2012 of the "Robotics and autonomous systems" third year speciality at ENSTA Paris.
- Sao Mai Nguyen is in charge of the "Robot Learning" third year course at ENSTA Paris.
- Clément Moulin-Frier is responsible professor of the "System Design, Integration and Control" course at the University Pompeu Fabra in Barcelona, Spain.
- Cécile Mazon is responsible of the second year of the curriculum in Technology, Ergonomics, Cognition and Handicap (Cognitive Sciences - University of Bordeaux) since sept. 2021.
- Cécile Mazon is responsible of the curriculum in Technology, Ergonomics, Cognition and Handicap (Cognitive Sciences - University of Bordeaux) since sept. 2022.
Teaching Involvement in Computer / Engineer science or in cognitive science:
- Université de Bordeaux - MIASHS Bachelor: Introductory course on assistive technology, disability and its management in the workplace, 7.5h (Isabeau Saint-Supery)
- ENSC/ENSEIRB Presentation of developmental artificial intelligence and the Flowers Lab, 2h, Option Robot (Laetitia Teodorescu)
- ENSC Introduction to bayesian analysis, 8h, Option AI (Adolphe Maxime)
- BS & Master: Cognitive Science, Univ. of Bordeaux- , 96h, Hélène Sauzéon
- BS & Master: Cognitive Science, Univ. of Bordeaux- , 192h, Cécile Mazon
- Master: Navigation for Robotics, 21 h, M2, ENSTA Paris, David Filliat
- Master: Navigation for Robotics, 24 h, M2 DataAI, IP Paris - Paris, David Filliat
- 2nd year : Deep Learning, 12h, IMT Atlantique (Sao Mai Nguyen).
- Université de Bordeaux - TCEH Master: IT Project Management, 18h (Isabeau Saint-Supery)
- Master UPF-Barcelona: Robotics and AI, 10h (Clément Moulin-Frier)
- Master : PY Oudeyer taught a course (3h) on Developmental Machine Learning at CogMaster, University Paris-Sorbonne (Jan 21)
- Industry : PY Oudeyer gave a course (1h) on Developmental AI at the AI4Industry event, Bordeaux (Jan 21)
- Academic : PY Oudeyer gave an invited tutorial (1h) at the CoRL robot learning conference (Nov 21)
- Master: Cognitive Sience, 24h (Eric Meyer)
- Teacher training at the Rectorat of Bordeaux : digital technologies for students with special educational needs , 6h (Eric Meyer)
- 2nd year Master in cognitive science : Assistive technologies (20h), Rania Abdelghani.
- PY Oudeyer gave a course on developmental reinforcement learning at ENSEIRB (2h), dec. 2022.
- PY Oudeyer gave a course on interaction grounded learning at the Microsoft Research machine learning school (1h30), april. 2022.
- PY Oudeyer gave a course on developmental AI at the Seminaire Numerics, for the EDMI graduate school, University of Bordeaux (1h30), oct. 2022.
11.2.2 Supervision
- PhD in progress: Maxime Adolphe, "Adaptive personalization in attention training systems", beg. in sept. 2020 (supervisors: H. Sauzéon and PY. Oudeyer)
- PhD in progress: Rania Abdelgani, "Fostering curiosity and meta-cognitive skills in educational technologies", beg. in dec. 2020 (supervisors: H. Sauzéon and PY. Oudeyer).
- PhD in progress: Isabeau Saint-Supery, "Designing and Assessing a new interactive tool fostering stakeholders' cooperation for school inclusion", (supervisors: Sauzéon and C. Mazon.)
- PhD in progress : Matisse Poupard "Optimize learning in a digital environment according to learners' level of expertise, epistemic curiosity and mode of instruction", (supervisors : H. Sauzéon and A. Tricot, Univ. Montpellier).
- PhD in progress : Gautier Hamon, "Environmental, adaptive, multi-agent and cultural dynamics of open-ended skill acquisition" '(supervisor: C. Moulin-Frier)
- PhD in progress: Mayalen Etcheverry, "Automated discovery with intrinsically motivated goal exploration processes", beg. in sept. 2020 (supervisors: PY. Oudeyer and C. Moulin-Frier)
- PhD in progress: Laetitia Teodorescu, "Graph Neural Networks in Curiosity-driven Exploring Agents", beg. in sept. 2020 (supervisors: PY. Oudeyer and K. Hoffman)
- PhD in progress: Tristan Karch, "Language acquisition in curiosity-driven Deep RL", beg. in sept. 2019 (supervisors: PY. Oudeyer and C. Moulin-Frier)
- PhD defended : Rémy Portelas, "Teacher algorithms for curriculum learning in Deep RL", beg. in sept. 2018 (supervisors: PY. Oudeyer and K. Hoffmann)
- PhD defended: Alexander Ten, "Models of human curiosity-driven learning and exploration", beg. in sept. 2018 (supervisors: PY. Oudeyer and J. Gottlieb)
- Master Thesis Defended : Elias Masquil "Intrinsically Motivated Goal-Conditioned Reinforcement Learning in Multi-Agent Environments", co-supervised by Eleni Nisioti, Gautier Hamon and Clément Moulin-Frier
- Master Thesis Defended : Yoann Lemesle "Self-organization of shared graphical languages in groups of agents using multimodal contrastive deep learning mechanisms", co-supervised by Tristan Karch, Pierre-Yves Oudeyer, Clément Moulin-Frier and Romain Laroche
- Master Thesis Defended : Erwan Plantec, Mass conservation for the study of virtual creatures in continuous cellular automata", co-supervised by Gautier Hamon, Mayalen Etcheverry, Pierre-Yves Oudeyer, Clément Moulin-Frier and Bert Chan
- Master Thesis Defended: Pauline Lucas " Designing of a conversational agent for fostering the curiosity states in children : Assessment of the use of GPT3- LLM ", co-supervised by R. Abdelghani and H. Sauzéon
11.2.3 Juries
- H. Sauzéon has been president and mentor of HDR degree of E. Altena
- H. Sauzéon was a member of PhD jury of Alexander Ten (Title : "The Role of Progress-Based Intrinsic Motivation in Learning : Evidence from Human Behavior and Future Directions") and was in the PhD "comité de suivi" of Charlotte Bettencourt (Univ. of Paris/ Sorbone University), Hugo Fournier (Univ. of Bordeaux), and Marion Pèch (Univ. of Bordeaux).
- H. Sauzéon and C. Mazon are permanent members of jury of Master degree in cognitive science at the university of Bordeaux.
- C. Colas has been a member of the jury of the undergrad thesis defence of Natan de Almeida Junges from Universidade Tecnológica Federal do Paraná. Title: Learning Pragmatic Frames in a Computational Architecture.
- PY Oudeyer was a member of the admissibility jury of the CR2 competition at Inria Bordeaux Sud-Ouest
- PY Oudeyer was a reviewer in the PhD juries of Leonard Hussenot (Title: "Apprenticeship learning: transferring human motivation to artificial agents", University of Lille), and an examiner in the juries of Ahmed Akakzia (Title: "Teaching Predicate-based Autotelic Agents", University Paris-Sorbonne) and Valentin Guillet (Title: "Distillation de réseaux de neurones pour la généralisation et le transfert en apprentissage par renforcement", University of Toulouse).
- PY Oudeyer was in the PhD "comité de suivi" of Alexandre Chenu (Univ. Paris VI), Marc Welter (Univ. Bordeaux), Jean-Baptiste Gaya (Université Paris-Sorbonne),
11.3 Popularization
11.3.1 Internal or external Inria responsibilities
NA
11.3.2 Articles and contents
- Meyer, E., Saint-Supery, I., Mazon, C., Sauzeon, H. (à paraître) L’inclusion scolaire des enfants ayant un TSA en favorisant la collaboration entre la famille, l’école et les professionnels médicosociaux : l’intérêt des outils numériques. Revue de littérature, Ressources documentaires de la FIRAH.
- R. Abdelghani led writing a blog post on training curiosity in children with conversational agents,
- M. Sawayama led writing a blog post on studying AI systems through the lens of cognitive science methods,
- T. Karch and P. Barde led writing a blog post on self-organization of communication protocols in groups of agents,
- D. Roy and PY. Oudeyer published the second edition of their popular science book for children on AI and robotics, Nathan,
11.3.3 Education
- Sauzéon, H. (2022) L'IA au service d'un apprentissage personnalisé basé sur la curiosité. Colloque InFine - Sens et finalités du numérique pour l’éducation, 13 octobre, Futuroscope Poitiers.
- H. Sauzéon, P.Y. Oudeyer, C. Moulin-Frier, C. Mazon, I. Saint-Supery and M. Etcheverry have received Eleonore, for a one week internship (stage de 3eme) in the team as part of the actions at Inria to promote the inclusion of people with disabilities.
- H. Sauzéon, M. Perie, C. Romac, T. Carta, G. Hamon have received 3 interns, for a day as part of their internship at inria (stage de 3eme). They were introduced to the research topic of the team and did some exploratory play with machine learning tools.
11.3.4 Interventions
- Members of the Flowers team participated to many interviews and documentaries for the press, the radio and television.
- March 2022. Conférence UniThé ou Café - Hélène Sauzéon & Clément Moulin-Frier - Des machines curieuses pour des humains curieux.
- June 2022. Webinaire IMIND - Cécile Mazon presented the study about the pilot study of an ITS-based intervention in specialized classrooms with second degree students with ASD.
- July 2022. Clément Moulin-Frier was interviewed for the Inria Podcast "Désassemblons le numérique".
- September 2022. Webinaire organized by Lorraine University and INSPE Nancy - Cécile Mazon gave a talk about digital technology for children with ASD and the interest of a systemic approach of their support
- November, 2022. G. Hamon and PY. Oudeyer presented the team and the internships available in the team to students of the MVA master (mathématiques vision apprentissage) at their "forum des stages", ENS Paris-Saclay.
- December, 2022. I. Saint-Supery and PY. Oudeyer welcomed and presented the Flowers team to L3 students of Ecole Normale Supérieure de Lyon.
- PY. Oudeyer was interviewed by magazine Polytechnic Insights on modeling curiosity-driven learning in AI and humans
12 Scientific production
12.1 Major publications
- 1 articleConversational agents for fostering curiosity-driven learning in children.International Journal of Human-Computer Studies167November 2022, 102887
- 2 articleAn Open-Source Cognitive Test Battery to Assess Human Attention and Memory.Frontiers in Psychology13June 2022
- 3 inproceedingsGrounding Language to Autonomously-Acquired Skills via Goal Generation.ICLR 2021 - Ninth International Conference on Learning RepresentationVienna / Virtual, AustriaMay 2021
- 4 inproceedingsPedagogical Agents for Fostering Question-Asking Skills in Children.CHI '20 - CHI Conference on Human Factors in Computing SystemsHonolulu / Virtual, United StatesApril 2020
- 5 articleActive Learning of Inverse Models with Intrinsically Motivated Goal Exploration in Robots.Robotics and Autonomous Systems611January 2013, 69-73
- 6 inproceedingsS-TRIGGER: Continual State Representation Learning via Self-Triggered Generative Replay.IJCNN 2021 - International Joint Conference on Neural NetworksShenzhen / Virtual, ChinaIEEEJuly 2021, 1-7
- 7 inproceedingsSymmetry-Based Disentangled Representation Learning requires Interaction with Environments.NeurIPS 2019Vancouver, CanadaDecember 2019
- 8 articleTowards Truly Accessible MOOCs for Persons with Cognitive Impairments: a Field Study.Human-Computer Interaction2021
- 9 inproceedingsCURIOUS: Intrinsically Motivated Modular Multi-Goal Reinforcement Learning.International Conference on Machine LearningLong Beach, FranceJune 2019
- 10 articleEpidemiOptim: a Toolbox for the Optimization of Control Policies in Epidemiological Models.Journal of Artificial Intelligence ResearchJuly 2021
- 11 inproceedingsLanguage as a Cognitive Tool to Imagine Goals in Curiosity-Driven Exploration.NeurIPS 2020 - 34th Conference on Neural Information Processing SystemsContains main article and supplementariesVancouver / Virtual, CanadaDecember 2020
- 12 inproceedingsGEP-PG: Decoupling Exploration and Exploitation in Deep Reinforcement Learning Algorithms.International Conference on Machine Learning (ICML)Stockholm, SwedenJuly 2018
- 13 articleExploring to learn visual saliency: The RL-IAC approach.Robotics and Autonomous Systems112February 2019, 244-259
- 14 articleIntrinsically Motivated Open-Ended Multi-Task Learning Using Transfer Learning to Discover Task Hierarchy.Applied Sciences113February 2021, 975
- 15 articleIntelligent Behavior Depends on the Ecological Niche.KI - Künstliche IntelligenzJanuary 2021
- 16 inproceedingsHierarchically Organized Latent Modules for Exploratory Search in Morphogenetic Systems.NeurIPS 2020 - 34th Conference on Neural Information Processing SystemsVancouver / Virtual, CanadaDecember 2020
- 17 unpublishedIntrinsically Motivated Goal Exploration Processes with Automatic Curriculum Learning.November 2017, working paper or preprint
- 18 inproceedingsA Unified Model of Speech and Tool Use Early Development.39th Annual Conference of the Cognitive Science Society (CogSci 2017)Proceedings of the 39th Annual Conference of the Cognitive Science SocietyLondon, United KingdomJuly 2017
- 19 articleTowards a neuroscience of active sampling and curiosity.Nature Reviews Neuroscience1912December 2018, 758-770
- 20 inproceedingsGrounding Spatio-Temporal Language with Transformers.NeurIPS 2021 - 35th Conference on Neural Information Processing SystemsVirtuel, FranceDecember 2021
- 21 inproceedingsCuriosity Driven Exploration of Learned Disentangled Goal Spaces.CoRL 2018 - Conference on Robot LearningZürich, SwitzerlandOctober 2018
- 22 articleState Representation Learning for Control: An Overview.Neural Networks108December 2018, 379-392
- 23 articlePilot study of an intervention based on an intelligent tutoring system (ITS) for instructing mathematical skills of students with ASD and/or ID.Education and Information Technologies2022
- 24 articleActive Navigation in Virtual Environments Benefits Spatial Memory in Older Adults.Brain Sciences92019
- 25 articleEmergent Jaw Predominance in Vocal Development through Stochastic Optimization.IEEE Transactions on Cognitive and Developmental Systems992017, 1-12
- 26 inproceedingsGrounding an Ecological Theory of Artificial Intelligence in Human Evolution.NeurIPS 2021 - Conference on Neural Information Processing Systems / Workshop: Ecological Theory of Reinforcement Learningvirtual event, FranceDecember 2021
- 27 inproceedingsUnsupervised Learning of Goal Spaces for Intrinsically Motivated Goal Exploration.ICLR2018 - 6th International Conference on Learning RepresentationsVancouver, CanadaApril 2018
- 28 inproceedingsTeacher algorithms for curriculum learning of Deep RL in continuously parameterized environments.CoRL 2019 - Conference on Robot Learninghttps://arxiv.org/abs/1910.07224Osaka, JapanOctober 2019
- 29 inproceedingsAutomatic Curriculum Learning For Deep RL: A Short Survey.IJCAI 2020 - International Joint Conference on Artificial IntelligenceKyoto / Virtuelle, JapanJanuary 2021
- 30 inproceedingsIntrinsically Motivated Discovery of Diverse Patterns in Self-Organizing Systems.International Conference on Learning Representations (ICLR)Source code and videos athttps://automated-discovery.github.io/Addis Ababa, EthiopiaApril 2020
- 31 inproceedingsTeachMyAgent: a Benchmark for Automatic Curriculum Learning in Deep RL.Proceedings of the 38th International Conference on MachineLearning, PMLR 139, 2021.ICML 2021 - Thirty-eighth International Conference on Machine Learning139Proceedings of the 38th International Conference on Machine LearningVienna / Virtual, AustriaJuly 2021, 9052--9063
- 32 articleHumans monitor learning progress in curiosity-driven exploration.Nature Communications121December 2021
- 33 inproceedingsTransflower: probabilistic autoregressive dance generation with multimodal attention.SIGGRAPH Asia 2021 - 14th ACM SIGGRAPH Conference and Exhibition on Computer Graphics and Interactive TechniquesTokyo, JapanDecember 2021
12.2 Publications of the year
International journals
International peer-reviewed conferences
National peer-reviewed Conferences
Conferences without proceedings
Scientific book chapters
Doctoral dissertations and habilitation theses
Reports & preprints
Other scientific publications
12.3 Other
Scientific popularization
12.4 Cited publications
- 80 inproceedingsPedagogical Agents for Fostering Question-Asking Skills in Children.CHI '20 - CHI Conference on Human Factors in Computing SystemsHonolulu / Virtual, United StatesApril 2020
- 81 articleHindsight experience replay.Advances in neural information processing systems302017
- 82 inproceedingsTowards measuring states of epistemic curiosity through electroencephalographic signals.IEEE SMC 2020 - IEEE International conference on Systems, Man and CyberneticsToronto / Virtual, CanadaOctober 2020
- 83 articleA Survey of Robot Learning from Demonstration.Robotics and Autonomous Systems5752009, 469--483
- 84 articlePurposive Behavior Acquisition On A Real Robot By Vision-Based Reinforcement Learning.Machine Learning231996, 279-303
- 85 inproceedingsIntrinsically Motivated Learning of Hierarchical Collections of Skills.Proceedings of the 3rd International Conference on Development and Learning (ICDL 2004)Salk Institute, San Diego2004
- 86 bookConflict, Arousal and Curiosity.McGraw-Hill1960
- 87 bookThe Coordination and Regulation of Movements.Preliminary but descriptive evidence that in some tasks the activity of the number of degrees of freedom is initially reduced and subsequently increasedPergamon1967
- 88 bookDesigning sociable robots.The MIT Press2004
- 89 inproceedingsAlternative essences of intelligence.Proceedings of 15th National Conference on Artificial Intelligence (AAAI-98)AAAI Press1998, 961--968
- 90 articleLanguage models are few-shot learners.arXiv preprint arXiv:2005.141652020
- 91 articleChild's Talk: Learning to Use Language.Child Language Teaching and Therapy111985, 111-114URL: https://doi.org/10.1177/026565908500100113
- 92 bookDevelopmental robotics: From babies to robots.MIT press2015
- 93 articleIdentifying Functions and Behaviours of Social Robots for In-Class Learning Activities: Teachers' Perspective.International Journal of Social RoboticsSeptember 2021
- 94 proceedingsLenia and Expanded Universe.ALIFE 2020: The 2020 Conference on Artificial LifeALIFE 2021: The 2021 Conference on Artificial Life07 2020, 221-229URL: https://doi.org/10.1162/isal_a_00297
- 95 articleLenia-biology of artificial life.Complex Systems2832019, 251-286
- 96 bookMindware: An Introduction to the Philosophy of Cognitive Science.Oxford University Press2001
- 97 phdthesisAdaptive Personalization of Pedagogical Sequences using Machine Learning.Université de BordeauxDecember 2018
- 98 articleMulti-Armed Bandits for Intelligent Tutoring Systems.Journal of Educational Data Mining (JEDM)72June 2015, 20--48
- 99 inproceedingsLeveraging procedural generation to benchmark reinforcement learning.International conference on machine learningPMLR2020, 2048--2056
- 100 articleActive learning with statistical models.Journal of artificial intelligence research41996, 129--145
- 101 inproceedingsLanguage as a Cognitive Tool to Imagine Goals in Curiosity-Driven Exploration.NeurIPS 2020 - 34th Conference on Neural Information Processing Systemshttps://arxiv.org/abs/2002.09253 - Contains main article and supplementariesVancouver / Virtual, CanadaDecember 2020
- 102 articleLanguage and culture internalization for human-like autotelic AI.412December 2022, 1068--1076URL: https://doi.org/10.1038/s42256-022-00591-4
- 103 articleAutotelic Agents with Intrinsically Motivated Goal-Conditioned Reinforcement Learning: A Short Survey.Journal of Artificial Intelligence Research74July 2022, 1159--1199URL: https://www.jair.org/index.php/jair/article/view/13554
- 104 unpublishedIntrinsically Motivated Goal-Conditioned Reinforcement Learning: a Short Survey.January 2021, working paper or preprint
- 105 inproceedingsTextworld: A learning environment for text-based games.Workshop on Computer GamesSpringer2018, 41--75
- 106 bookCognitive Linguistics.Cambridge Textbooks in LinguisticsCambridge University Press2004
- 107 bookFlow-the psychology of optimal experience.Harper Perennial1991
- 108 articleReward, motivation and reinforcement learning.Neuron362002, 285--298
- 109 bookIntrinsic Motivation and Self-Determination in Human Behavior.Plenum Press1985
- 110 articlePartial connectivity increases cultural accumulation within groups.Proceedings of the National Academy of Sciences11311March 2016, 2982--2987URL: http://www.pnas.org/lookup/doi/10.1073/pnas.1518798113
- 111 articleRL$̂2$: Fast Reinforcement Learning via Slow Reinforcement Learning.arXiv:1611.02779 [cs, stat]2016
- 112 articleFirst return, then explore.Nature59078472021, 580--586
- 113 articleLearning and development in neural networks: The importance of starting small.Cognition481993, 71--99
- 114 articleCompetency in Navigating Arbitrary Spaces: Intelligence as an Invariant for Analyzing Cognition in Diverse Embodiments.2022
- 115 articleDifferences in cognitive processes underlying the collaborative activities of children and chimpanzees.Cognitive Development2722012, 136-153URL: https://www.sciencedirect.com/science/article/pii/S0885201412000093
- 116 inproceedingsA Unified Model of Speech and Tool Use Early Development.39th Annual Conference of the Cognitive Science Society (CogSci 2017)Proceedings of the 39th Annual Conference of the Cognitive Science SocietyLondon, United KingdomJuly 2017
- 117 articleIntrinsically motivated goal exploration processes with automatic curriculum learning.arXiv preprint arXiv:1708.021902017
- 118 articleInformation-seeking, curiosity, and attention: computational and neural mechanisms.Trends in Cognitive Sciences1711November 2013, 585-93
- 119 articleA curious formulation robot enables the discovery of a novel protocell behavior.Science advances652020, eaay4237
- 120 articleEvolution and dispersal under climatic instability: a simple evolutionary algorithm.Adaptive Behavior224August 2014, 235--254URL: http://journals.sagepub.com/doi/10.1177/1059712314533573
- 121 articleThe symbol grounding problem.Physica D401990, 335--346
- 122 bookActive learning in neural networks.Heidelberg, Germany, GermanyPhysica-Verlag GmbH2002, 137--169
- 123 bookArtificial Intelligence: the very idea.Cambridge, MA, USAThe MIT Press1985
- 124 articleDeep reinforcement learning with a natural language action space.arXiv preprint arXiv:1511.046362015
- 125 articleMesolimbocortical and nigrostriatal dopamine responses to salient non-reward events.Neuroscience9642000, 651-656
- 126 inproceedingsNovelty and reinforcement learning in the value system of developmental robots.Proceedings of the 2nd international workshop on Epigenetic Robotics : Modeling cognitive development in robotic systemsLund University Cognitive Studies 942002, 47--55
- 127 inproceedingsPerception and human interaction for developmental learning of objects and affordances.Proc. of the 12th IEEE-RAS International Conference on Humanoid Robots - HUMANOIDSforthcomingJapan2012, URL: http://hal.inria.fr/hal-00755297
- 128 articleHuman-level performance in 3D multiplayer games with population-based reinforcement learning.Science3646443Publisher: American Association for the Advancement of Science2019, 859--865
- 129 bookDevelopmental Cognitive Neuroscience.Blackwell publishing2005
- 130 bookDevelopmental cognitive neuroscience.Wiley-Blackwell2011
- 131 articleGrounding Spatio-Temporal Language with Transformers.Advances in Neural Information Processing Systems342021, 5236--5249
- 132 articleAuto-encoding variational bayes.arXiv preprint arXiv:1312.61142013
- 133 articleBiological robustness.Nature Reviews Genetics5112004, 826--837
- 134 inproceedingsCombining manual feedback with subsequent MDP reward signals for reinforcement learning.Proceedings of the 9th International Conference on Autonomous Agents and Multiagent Systems (AAMAS'10)Toronto, Canada2010, 5--12
- 135 unpublishedSocialAI: Benchmarking Socio-Cognitive Abilities in Deep Reinforcement Learning Agents.October 2021, working paper or preprint
- 136 miscLearning not to learn: Nature versus nurture in silico.2020
- 137 articleEmergence of Shared Sensory-motor Graphical Language from Visual Input.arXiv preprint arXiv:2210.064682022
- 138 articleTechnological approach to mind everywhere: an experimentally-grounded framework for understanding diverse bodies and minds.Frontiers in Systems Neuroscience2022, 17
- 139 inproceedingsSimultaneous Acquisition of Task and Feedback Models.Development and Learning (ICDL), 2011 IEEE International Conference onGermany2011, 1 - 7URL: http://hal.inria.fr/hal-00636166/en
- 140 articleDevelopmental Robotics: A Survey.Connection Science1542003, 151-190
- 141 miscReframing cognition: getting down to biological basics.2021, 20190750
- 142 inproceedingsDevelopmental Approach for Interactive Object Discovery.Neural Networks (IJCNN), The 2012 International Joint Conference onAustraliaJune 2012, 1-7
- 143 inproceedingsAn Emergent Framework for Self-Motivation in Developmental Robotics.Proceedings of the 3rd International Conference on Development and Learning (ICDL 2004)Salk Institute, San Diego2004
- 144 inproceedingsRobot Self-Initiative and Personalization by Learning through Repeated Interactions.6th ACM/IEEE International Conference on Human-RobotSwitzerland2011, URL: http://hal.inria.fr/hal-00636164/en
- 145 articleFostering parents-professional collaboration for facilitating the school inclusion of students with ASD: Design of the ''ToGather'' web-based prototype.Educational Technology Research and DevelopmentDecember 2021
- 146 articleEffectiveness and usability of technology-based interventions for children and adolescents with ASD: A systematic review of reliability, consistency, generalization and durability related to the effects of intervention.Computers in Human Behavior93April 2019
- 147 bookTheories of developmental psychology.New York: Worth2001
- 148 bookTheories of developmental psychology.Worth2004
- 149 articleHuman-level control through deep reinforcement learning.Nature5187540February 2015, 529--533URL: http://www.nature.com/articles/nature14236
- 150 techreportMassively Parallel Methods for Deep Reinforcement Learning.arXiv:1507.04296arXiv:1507.04296 [cs]arXivJuly 2015, URL: http://arxiv.org/abs/1507.04296
- 151 inproceedingsBootstrapping Intrinsically Motivated Learning with Human Demonstrations.IEEE International Conference on Development and LearningFrankfurt, Germany2011, URL: http://hal.inria.fr/hal-00645986/en
- 152 inproceedingsConstraining the Size Growth of the Task Space with Socially Guided Intrinsic Motivation using Demonstrations..IJCAI Workshop on Agents Learning Interactively from Human Teachers (ALIHT)Barcelona, Spain2011, URL: http://hal.inria.fr/hal-00645995/en
- 153 incollection L'auto-organisation dans l'évolution de la parole.Parole et Musique: Aux origines du dialogue humain, Colloque annuel du Collège de FranceOdile Jacob2009, 83-112URL: http://hal.inria.fr/inria-00446908/en/
- 154 incollectionDevelopmental Robotics.Encyclopedia of the Sciences of LearningSpringer Reference SeriesSpringer2011, URL: http://hal.inria.fr/hal-00652123/en
- 155 articleIntrinsic Motivation Systems for Autonomous Mental Development.IEEE Transactions on Evolutionary Computation1122007, 265--286
- 156 inproceedingsIntelligent adaptive curiosity: a source of self-development.Proceedings of the 4th International Workshop on Epigenetic Robotics117Lund University Cognitive Studies2004, 127--130
- 157 articleWhat is intrinsic motivation? A typology of computational approaches.Frontiers in Neurorobotics112007
- 158 incollectionSur les interactions entre la robotique et les sciences de l'esprit et du comportement.Informatique et Sciences Cognitives : influences ou confluences ?Presses Universitaires de France2009, URL: http://hal.inria.fr/inria-00420309/en/
- 159 inproceedingsEpisodic transformer for vision-and-language navigation.Proceedings of the IEEE/CVF International Conference on Computer Vision2021, 15942--15952
- 160 unpublishedA systematic review of immersive technologies for education: effects of cognitive load and curiosity state on learning performance.December 2022, working paper or preprint
- 161 inproceedingsLearning transferable visual models from natural language supervision.International Conference on Machine LearningPMLR2021, 8748--8763
- 162 inbookImitation and Social Learning in Robots, Humans and Animals: Behavioural, Social and Communicative Dimensions.K.Kerstin DautenhahnC.C. NehanivCambridge University Press2004, How to build an imitator?
- 163 articleCooperative control of environmental extremes by artificial intelligent agents.arXiv preprint arXiv: Arxiv-2212.023952022
- 164 inproceedingsLearning motor dependent Crutchfield's information distance to anticipate changes in the topology of sensory body maps.IEEE International Conference on Learning and DevelopmentChine Shangai2009, URL: http://hal.inria.fr/inria-00420186/en/
- 165 articleEvolving internal reinforcers for an intrinsically motivated reinforcement-learning robot. IEEE 6th International Conference on Development and Learning, 2007. ICDL 2007.July 2007, 282-287URL: http://dx.doi.org/10.1109/DEVLRN.2007.4354052
- 166 inproceedingsCurious Model-Building Control Systems.Proceedings of the International Joint Conference on Neural Networks, Singapore2IEEE press1991, 1458--1463
- 167 articleA neural substrate of prediction and reward.Science2751997, 1593-1599
- 168 inproceedingsAlfred: A benchmark for interpreting grounded instructions for everyday tasks.Proceedings of the IEEE/CVF conference on computer vision and pattern recognition2020, 10740--10749
- 169 articleMastering the game of Go with deep neural networks and tree search.nature52975872016, 484--489
- 170 bookL.Luc SteelsR.Rodney BrooksThe Artificial Life Route to Artificial Intelligence: Building Embodied, Situated Agents.Hillsdale, NJ, USAL. Erlbaum Associates Inc.1995
- 171 bookThe Talking Heads experiment.Computational Models of Language Evolution1BerlinLanguage Science Press2015
- 172 articleAutomatic Exploration of Textual Environments with Language-Conditioned Autotelic Agents.arXiv preprint arXiv:2207.041182022
- 173 bookA dynamic systems approach to the development of cognition and action.Cambridge, MAMIT Press1994
- 174 articleTeachable robots: Understanding human teaching behavior to build more effective robot learners.Artificial Intelligence Journal1722008, 716-737
- 175 incollectionBecoming human.Becoming HumanHarvard University Press2019
- 176 bookThe Cultural Origins of Human Cognition.Harvard University Press1999, URL: http://www.jstor.org/stable/j.ctvjsf4jc
- 177 articleComputing machinery and intelligence.Mind591950, 433-460
- 178 articleThe LoCA regret: a consistent metric to evaluate model-based behavior in reinforcement learning.Advances in Neural Information Processing Systems332020, 6562--6572
- 179 bookThe embodied mind : Cognitive science and human experience.Cambridge, MAMIT Press1991
- 180 inproceedingsStudying the co-construction of interaction protocols in collaborative tasks with humans.4th International Conference on Development and Learning and on Epigenetic RoboticsIEEE2014, 208--215
- 181 bookMind in society: Development of higher psychological processes.Harvard university press1978
- 182 article ScienceWorld: Is your Agent Smarter than a 5th Grader? arXiv preprint arXiv:2203.07540 2022
- 183 articleLearning to Reinforcement Learn.arXiv:1611.05763 [cs, stat]2017
- 184 articleAutonomous mental development by robots and animals.Science2912001, 599-600