2023Activity reportProject-TeamLIFEWARE
RNSR: 201421205T- Research center Inria Saclay Centre
- Team name: Computational systems biology and optimization
- Domain:Digital Health, Biology and Earth
- Theme:Computational Biology
Keywords
Computer Science and Digital Science
- A2.1.1. Semantics of programming languages
- A2.1.5. Constraint programming
- A2.1.10. Domain-specific languages
- A2.2.1. Static analysis
- A2.3.2. Cyber-physical systems
- A2.4. Formal method for verification, reliability, certification
- A2.4.1. Analysis
- A2.4.2. Model-checking
- A2.4.3. Proofs
- A3.4.2. Unsupervised learning
- A3.4.4. Optimization and learning
- A6.1.1. Continuous Modeling (PDE, ODE)
- A6.1.2. Stochastic Modeling
- A6.1.3. Discrete Modeling (multi-agent, people centered)
- A6.1.4. Multiscale modeling
- A6.2.4. Statistical methods
- A6.2.6. Optimization
- A6.3.1. Inverse problems
- A6.3.4. Model reduction
- A7.2. Logic in Computer Science
- A8.1. Discrete mathematics, combinatorics
- A8.2. Optimization
- A8.7. Graph theory
- A9.7. AI algorithmics
Other Research Topics and Application Domains
- B1. Life sciences
- B1.1.2. Molecular and cellular biology
- B1.1.7. Bioinformatics
- B1.1.8. Mathematical biology
- B1.1.10. Systems and synthetic biology
- B2.2.3. Cancer
- B2.2.6. Neurodegenerative diseases
- B2.4.1. Pharmaco kinetics and dynamics
- B9. Society and Knowledge
1 Team members, visitors, external collaborators
Research Scientists
- François Fages [Team leader, INRIA, Senior Researcher, HDR]
- Jakob Ruess [INRIA, Researcher]
- Sylvain Soliman [INRIA, Researcher, HDR]
Faculty Members
- Anna Niarakis [UNIV TOULOUSE III, Professor, from Sep 2023, HDR]
- Anna Niarakis [Univ EVRY, Associate Professor, until Aug 2023, Univ EVRY Val d'Essonne Paris-Saclay, HDR]
Post-Doctoral Fellows
- Guillaume Ballif [INRIA, Post-Doctoral Fellow, from Jul 2023]
- Henri Mermoz Kouye [INRIA, Post-Doctoral Fellow, from Mar 2023]
PhD Students
- Sahar Aghakhani [UNIV EVRY, until Sep 2023]
- Marine Collery [IBM, until Sep 2023]
- Alexandre Tan-Lhernould [SERVIER, from Oct 2023]
Technical Staff
- Mathieu Hemery [INRIA, Engineer, until Aug 2023]
Interns and Apprentices
- Hugo Buscemi [ENS PARIS-SACLAY, Intern, from Mar 2023 until Jul 2023]
Administrative Assistant
- Melanie Da Silva [INRIA, from May 2023]
External Collaborators
- Guillaume Ballif [INSTITUT PASTEUR, until Jun 2023]
- Julien Martinelli [UNIV AALTO]
- Denis Thieffry [ENS PARIS, HDR]
2 Overall objectives
This project aims at developing formal methods for understanding the cell machinery and establishing computational paradigms in cell biology. It is based on the vision of
cells as machines,
chemical reaction networks as programs,
and on the use of concepts from computer science to master the complexity of cell biochemical processes 25.
We contribute to the development of a computational theory of chemical reaction networks (CRNs), by addressing fundamental research issues in computer science on the concepts of analog computation and analog computational complexity in biochemistry, and on the interplay between structure and dynamics in CRNs.
Since 2002, we develop a software platform, called the Biochemical Abstract Machine (BIOCHAM), for modeling, analyzing and now synthesizing CRNs, with some unique algorithmic contributions. The reaction rule-based language of BIOCHAM allows us to reason about CRNs at different levels of abstraction in the hierarchy of their stochastic, differential, Boolean and hybrid semantics. Various static analysis methods, most of them based on constraint solving or graph theory, provide useful information before going to simulations and dynamical analyses, for which quantitative temporal logic is used to formalize cell behaviors with imprecise data, and to constrain model building.
A tight integration between dry lab and wet lab efforts is also essential for the success of the project. This is achieved through collaborations with biologists and experimentalists, including partners from the pharmaceutical industry, on concrete biological and biomedical questions.
Because of the importance of constraint solving and optimization techniques in our approach, and the need of rapid prototyping for our software developments, we keep some research and teaching activity in relational programming (i.e. logic programming, constraint programming, interval arithmetic) as a general paradigm for computing with partial information systems, and solving practical instances of NP-hard problems.
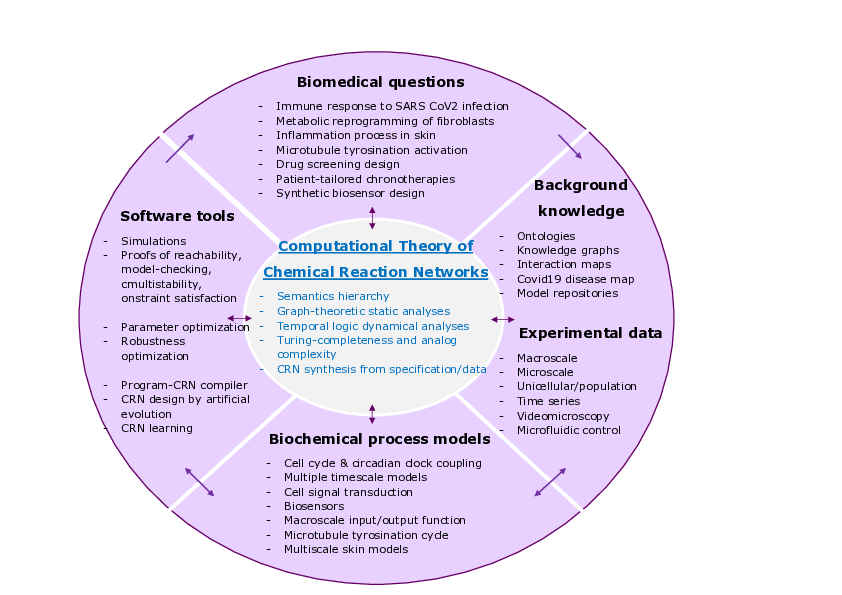
Wheel of biomedical questions, background knowledge and experimental data, biological process models and decision making tools, with the computational theory of chemical reaction networks at the center.
3 Research program
3.1 Chemical Reaction Network (CRN) Theory
Originally, Feinberg's mathematical theory of Chemical Reaction Networks (CRN) and Thomas's influence networks, were created to provide sufficient and/or necessary structural conditions for the existence of multiple steady states and oscillations in complex gene networks. Those conditions can be verified by static analyzers without knowing kinetic parameter values, nor making any simulation. In this first approach, most of our work consists in considering the hypergraph structure of a CRN (Petri net invariants, species-reaction labelled hypergraph, influence graph, reductions by subgraph epimorphisms) and analysing their interplay with the dynamics of CRNs in their different interpretations (Boolean, CTMC, ODE, time scale separations) which can be related in the framework of abstract interpretation1. For example, our study of the influence graphs of reaction systems 7 lead recently to sufficient graphical conditions ensuring rate-independence of CRN 4, or some time ago to the non-trivial generalization to reaction systems of Thomas' conditions of multi-stationarity and Soulé's proof given for influence systems, with much greater efficiency by several orders of magnitude for testing them when compared to current symbolic computation methods 2.
However, we aim at development a computational theory of CRNs and biochemical programming. Our original method to infer CRNs from ODEs 5 showed the generality of CRNs, and lead us to prove the Turing-completeness of continuous CRNs over a finite set of molecular species, showing that any computable function over the real numbers (i.e. computable in arbitrary precision by a Turing machine) can be computed by a CRN over a finite set of molecular species 6. (Best paper award CMSB 2017, Prix La Recherche 2019). This result closed the last open problem on the computation power of CRNs in their different semantics and has opened a whole research avenue on CRN design by compilation of mathematical functions. This is illustrated by a series of publications since that date 10 (best paper award CMSB 2022), again this year 23 , and innovative applications in synthetic biology 3.
3.2 Logical Paradigm for Systems Biology
Our group was among the first ones in 2002 to apply model-checking methods to systems biology in order to reason on large molecular interaction networks, such as Kohn's map of the mammalian cell cycle (800 reactions over 500 molecules) 2. The logical paradigm for systems biology that we have subsequently developed for quantitative models can be summarized by the following identifications :
biological model = transition system , initial state
dynamical behavior specification = temporal logic formula
model validation = model-checking
model reduction = sub-model-checking, s.t.
model prediction = formula enumeration, s.t.
static experiment design = symbolic model-checking,
model synthesis = constraint solving
model inference = constraint solving
In particular, the definition of a continuous satisfaction degree for first-order temporal logic formulae with constraints over the reals, was the key to generalize this approach to quantitative models, opening up the field of model-checking to model optimization 34
This line of research continues with the development of temporal logic constraint patterns with efficient solvers, and their use for model building, in partnership with biologists to answer concrete questions in the biomedical domain5616, 15 and the pharmaceutical industry 9, 20, 14, 20.
3.3 Constraint Solving and Optimization
Constraint solving and optimization algorithms are important in our research. On the one hand, static analyses of CRNs often involve solving hard combinatorial optimization problems, for which we have shown that constraint logic programming techniques, including Answer Set Programming (ASP), are particularly successful, often beating dedicated algorithms on real-size instances from model repositories by orders of magnitude 12, 217.
On the other hand, parameter search problems involve solving hard continuous optimization problems, for which evolutionary algorithms, and especially the covariance matrix evolution strategy (CMA-ES) (EPI RANDOPT) have shown to provide best results in our context. Constraint-based models and efficient constraint solvers are thus instrumental in our approach for building quantitative models, gaining model-based insights, revisiting biological hypotheses, and contributing to biological knowledge.
4 Application domains
4.1 Preamble
Our collaborative work on biological applications is expected to serve as a basis for groundbreaking advances in cell functioning understanding, cell monitoring and control, and novel therapy design and optimization. Our collaborations with biologists are focused on concrete biological questions, and on the building of mechanistic models of biological systems to answer them. Furthermore, one important application of our research is the development and distribution of a modeling software for computational systems biology and synthetic biology.
4.2 Modeling software for systems and synthetic biology at the cellular level
Since 2002, we develop an open-source software environment for modeling and analyzing biochemical reaction systems. This software, called the Biochemical Abstract Machine (BIOCHAM), is compatible with SBML for importing and exporting models from repositories such as BioModels. It can perform a variety of static analyses, specify behaviors in Boolean or quantitative temporal logics, search parameter values satisfying temporal constraints, and make various simulations. While the primary reason of this development effort is to be able to implement our ideas and experiment them quickly on a large scale, using rapid prototyping techniques based on constraint logic programming, BIOCHAM is distributed and used by other groups worldwide, for building CRN models, for comparing CRN analysis/synthesis techniques, and for teaching computational systems biology. A Jupyter BIOCHAM kernel has been developed to use BIOCHAM on our web server without any installation which is heavily used for teaching. We plan to continue developing BIOCHAM for these different purposes with the recruitment of a research engineer to improve the software quality and animation of the community of users.
Since 2018, the CaSQ software complements this effort by providing an interface to import large interaction maps written in SBML using the CellDesigner tool and translate them into Boolean influence models with various tools compatible with the SBML-qual standard.
Since 2020, we participate in the CoLoMoTo notebook platform, which provides an integrated collection of software tools for the analysis of qualitative models, including CaSQ. This platform encourages the reproducibility of analysis by combining Docker images (reproducible software environment) and Jupyter notebooks (reproducible and shareable workflows).
These two last efforts play a central role in the global Covid-19 Disease Maps project.
4.3 Biomedical applications
We plan to continue to tackle challenging concrete biomedical questions with academic and industrial partners in an opportunist way, according to both the scope of the question on the international scene, and the relevance of our theoretical approaches to the question.
As mentioned above, our long-standing collaboration with F. Molina's CNRS-ALCEN lab on the design, optimization and industrialization of biochemical diagnosis vesicles 3 continues on a design methodology of robust CRNs including analog functions and derivative estimation 23.
Our successful collaboration with Servier on knowledge graphs and CRN model parameterizations using quantitative temporal logic for drug screening 9 continues with a new CIFRE thesis with Servier started this fall on CRN model learning from experimental data.
Our similarly successful collaboration with Johnson& Johnson Santé Beauté France on multi-scale modeling of the epidermis and multifactorial aspects of atopic dermatitis 14, 20 has revealed complex metastability behaviors in our population dynamics models and further emerging properties when combined with a multi-agent model at the tissue level. This research continued with O. Radulescu in Montpellier on the tropical algebraic analysis of quasi-stability in our model has led to a generalization of this phenomenon in population dynamicas models 20.
We also keep ready to continue our long-standing collaborations on chronotherapies as specialists of coupled models of the cell cycle and the circadian clock 11, 16 and their systemic regulators8.
Last but not least, our group became very active in cooperative efforts at the European scale to design large molecular interaction maps for diseases such as Covid-19 13 or rheumatoid arthritis 19 and derive from them Boolean dynamical models using the CaSQ software as well as developing hybrid models 17.
5 Social and environmental responsibility
5.1 Footprint of research activities
In synthetic biology, our approach based on analog computation target enzymatic reactions with proteins in artificial DNA-free RNA-free vesicles. This is an original approach to solving major safety issue for applications in medicine and the environment.
5.2 Impact of research results
Our multidisciplinary research rooted in fundamental computer science aims at contributing to biology and medicine by going quite far in the applications with partners from academia and industry, in particular with the pharma industry.
6 Highlights of the year
We consider as major results for our project the following results published this year and described in Section 8
6.1 Trap spaces of Boolean networks are conflict-free siphons of their Petri net encoding
Participants: Sylvain Soliman.
Boolean network modeling of gene regulation but also of post-transcriptomic systems has proven over the years that it can bring powerful analyses and corresponding insight to the many cases where precise biological data is not sufficiently available to build a detailed quantitative model. Besides simulation, the analysis of such models is mostly based on attractor computation, since those correspond roughly to observable biological phenotypes. The recent use of trap spaces made a real breakthrough in that field allowing to consider medium-sized models that used to be out of reach. However, with the continuing increase in model size and complexity of Boolean update functions, the state-of-the-art computation of minimal trap spaces based on prime implicants shows its limits due to the difficulty of the prime-implicant computation.
In 21, we explore and prove for the first time a connection between trap spaces of a general Boolean network and siphons of its Petri net encoding. Besides important theoretical applications in studying properties of trap spaces, the connection enables us to propose an alternative approach to compute minimal trap spaces, and hence complex attractors, of a general Boolean network. It replaces the need for prime implicants by a completely different technique, namely the enumeration of maximal siphons in the Petri net encoding of the original model. We then demonstrate its efficiency and compare it to the state-of-the-art methods on a large collection of real-world and randomly generated models.
6.2 Stochastic chemical kinetics of cell fate decision systems: from single cells to populations and back
Participants: Guillaume Ballif, Jakob Ruess.
Stochastic chemical kinetics is a widely used formalism for studying stochasticity of chemical reactions inside single cells. Experimental studies of reaction networks are generally performed with cells that are part of a growing population, yet the population context is rarely taken into account when models are developed. Models that neglect the population context lose their validity whenever the studied system influences traits of cells that can be selected in the population, a property that naturally arises in the complex interplay between single-cell and population dynamics of cell fate decision systems.
In 18, we represent such systems as absorbing continuous-time Markov chains. We show that conditioning on non-absorption allows one to derive a modified master equation that tracks the time evolution of the expected population composition within a growing population. This allows us to derive consistent population dynamics models from a specification of the single-cell process. We use this approach to classify cell fate decision systems into two types that lead to different characteristic phases in emerging population dynamics. Subsequently, we deploy the gained insights to experimentally study a recurrent problem in biology: how to link plasmid copy number fluctuations and plasmid loss events inside single cells to growth of cell populations in dynamically changing environments.
6.3 A Skin Microbiome Model with AMP interactions and Analysis of Quasi-Stability vs Stability in Population Dynamics
Participants: François Fages, Elea Thibault-Greugny.
The skin microbiome plays an important role in the maintenance of a healthy skin. It is an ecosystem, composed of several species, competing for resources and interacting with the skin cells. Imbalance in the cutaneous microbiome, also called dysbiosis, has been correlated with several skin conditions, including acne and atopic dermatitis. Generally, dysbiosis is linked to colonization of the skin by a population of opportunistic pathogenic bacteria. Treatments consisting in non-specific elimination of cutaneous microflora have shown conflicting results.
In 20, we introduce a mathematical model based on ordinary differential equations, with 2 types of bacteria populations (skin commensals and opportunistic pathogens) and including the production of antimicrobial peptides to study the mechanisms driving the dominance of one population over the other. By using published experimental data, assumed to correspond to the observation of stable states in our model, we reduce the number of parameters of the model from 13 to 5. We then use a formal specification in quantitative temporal logic to calibrate our model by global parameter optimization and perform sensitivity analyses. On the time scale of 2 days of the experiments, the model predicts that certain changes of the environment, like the elevation of skin surface pH, create favorable conditions for the emergence and colonization of the skin by the opportunistic pathogen population, while the production of human AMPs has non-linear effect on the balance between pathogens and commensals. Surprisingly, simulations on longer time scales reveal that the equilibrium reached around 2 days can in fact be a quasi-stable state followed by the reaching of a reversed stable state after 12 days or more. We analyse the conditions of quasi-stability observed in this model using tropical algebraic methods, and show their non-generic character in contrast to slow-fast systems. These conditions are then generalized to a large class of population dynamics models over any number of species.
7 New software, platforms, open data
7.1 New software
7.1.1 BIOCHAM
-
Name:
The Biochemical Abstract Machine
-
Keywords:
Bioinformatics, Systems Biology, Computational biology
-
Functional Description:
The Biochemical Abstract Machine (BIOCHAM) is a software environment for modeling, analyzing and synthesizing biochemical reaction networks (CRNs) with respect to a formal specification of the observed or desired behavior of a biochemical system. BIOCHAM is compatible with the Systems Biology Markup Language (SBML) and contains some unique features about formal specifications in quantitative temporal logic, sensitivity and robustness analyses and parameter search in high dimension w.r.t. behavioral specifications, static analyses, and synthesis of CRNs.
-
Release Contributions:
– compilation pipeline of elementary mathematical functions in at most bi-molecular reaction networks over a finite set of abstract molecular species – improved heuristic quadratization algorithm to generate at most bi-molecular reactions – notebooks of Master classes in Bioinfomratics of molecular interaction networks – multiple improvements of the commands and documentation
- URL:
-
Contact:
François Fages
-
Participants:
François Fages, Mathieu Hemery, Sylvain Soliman
7.1.2 CaSQ
-
Name:
CellDesigner as SBML-Qual
-
Keywords:
SBML, Logical Framework, Knowledge representation
-
Functional Description:
CaSQ is a tool that can convert a molecular interaction map built with CellDesigner, or any similar SBML-capable tool, to an executable Boolean model. CaSQ is developed in Python (download and install instructions can be found on the Python package index) and uses as source the xml file of CellDesigner, in order to infer preliminary Boolean rules based solely on network topology and semantic annotations (e.g., certain arcs are noted as catalysis, inhibition, etc.). The aim is to convert a Process Description representation, i.e., a reaction model, into a full logical model. The resulting structure is closer to an Activity Flow diagram, though not in a strict SBGN-PD to SBGN-AF notion. Moreover logical rules that make the model executable are also obtained. CaSQ was used on maps of the Rheumatoïd Arthritis, of the MAP-Kinase cascade, etc. and is now being used by the Covid-19 DiseaseMaps consortium to automatically obtain logical models from maps [2].
CaSQ has recently been added to the CoLoMoTo Docker image and can be used in such a notebook.
- URL:
- Publications:
-
Contact:
Sylvain Soliman
-
Participants:
Sylvain Soliman, Anna Niarakis, Aurélien Naldi
-
Partner:
Université d'Evry-Val d'Essonne
7.1.3 CoLoMoTo Notebook
-
Keywords:
Systems Biology, Computational biology
-
Functional Description:
Dynamical models are widely used to summarize our understanding of biological networks, to challenge its consistency, and to guide the generation of realistic hypothesis before experimental validation. Qualitative models in particular enable the study of the dynamical properties that do not depend on kinetic parameters, which can be difficult to obtain. This platform integrates a wide collection of complementary software tools, along with interoperability features and a consistent Programming interface based on the popular Python language.
The platform is distributed as a Docker image, ensuring the reproducibility of the software environment. The use of Jupyter Notebooks provides a good balance between ease of use and flexibility. These notebooks can be further used to share precise analysis workflows, ensuring the dissemination of fully reproducible research work using this platform.
- URL:
- Publication:
-
Contact:
Aurélien Naldi
-
Participants:
Sylvain Soliman, Aurélien Naldi, Denis Thieffry
-
Partners:
LaBRI, Institut Curie, ENS Paris, LRI - Laboratoire de Recherche en Informatique
8 New results
8.1 Stochastic chemical kinetics of cell fate decision systems: from single cells to populations and back
Participants: Guillaume Ballif, Henri Mermoz Kouyé, Jakob Ruess.
Stochastic chemical kinetics is a widely used formalism for studying stochasticity of chemical reactions inside single cells. Experimental studies of reaction networks are generally performed with cells that are part of a growing population, yet the population context is rarely taken into account when models are developed. Models that neglect the population context lose their validity whenever the studied system influences traits of cells that can be selected in the population, a property that naturally arises in the complex interplay between single-cell and population dynamics of cell fate decision systems.
In 18, we represent such systems as absorbing continuous-time Markov chains. We show that conditioning on non-absorption allows one to derive a modified master equation that tracks the time evolution of the expected population composition within a growing population. This allows us to derive consistent population dynamics models from a specification of the single-cell process. We use this approach to classify cell fate decision systems into two types that lead to different characteristic phases in emerging population dynamics. Subsequently, we deploy the gained insights to experimentally study a recurrent problem in biology: how to link plasmid copy number fluctuations and plasmid loss events inside single cells to growth of cell populations in dynamically changing environments.
8.2 A Skin Microbiome Model with AMP interactions and Analysis of Quasi-Stability vs Stability in Population Dynamics
Participants: François Fages.
The skin microbiome plays an important role in the maintenance of a healthy skin. It is an ecosystem, composed of several species, competing for resources and interacting with the skin cells. Imbalance in the cutaneous microbiome, also called dysbiosis, has been correlated with several skin conditions, including acne and atopic dermatitis. Generally, dysbiosis is linked to colonization of the skin by a population of opportunistic pathogenic bacteria. Treatments consisting in non-specific elimination of cutaneous microflora have shown conflicting results.
In 20, we introduce a mathematical model based on ordinary differential equations, with 2 types of bacteria populations (skin commensals and opportunistic pathogens) and including the production of antimicrobial peptides to study the mechanisms driving the dominance of one population over the other. By using published experimental data, assumed to correspond to the observation of stable states in our model, we reduce the number of parameters of the model from 13 to 5. We then use a formal specification in quantitative temporal logic to calibrate our model by global parameter optimization and perform sensitivity analyses. On the time scale of 2 days of the experiments, the model predicts that certain changes of the environment, like the elevation of skin surface pH, create favorable conditions for the emergence and colonization of the skin by the opportunistic pathogen population, while the production of human AMPs has non-linear effect on the balance between pathogens and commensals. Surprisingly, simulations on longer time scales reveal that the equilibrium reached around 2 days can in fact be a quasi-stable state followed by the reaching of a reversed stable state after 12 days or more. We analyse the conditions of quasi-stability observed in this model using tropical algebraic methods, and show their non-generic character in contrast to slow-fast systems. These conditions are then generalized to a large class of population dynamics models over any number of species.
In 8, this is combined with a multi-agent model of the skin for further analyses of the multifactorial factors leading to atopic dermatitis.
8.3 On Estimating Derivatives of Input Signals in Biochemistry
Participants: François Fages, Mathieu Hemery.
The online estimation of the derivative of an input signal is widespread in control theory and engineering. In the realm of chemical reaction networks (CRN), this raises however a number of specific issues on the different ways to achieve it. A CRN pattern for implementing a derivative block has already been proposed for the PID control of biochemical processes, and proved correct using Tikhonov's limit theorem.
In 23, we give a detailed mathematical analysis of that CRN, thus clarifying the computed quantity and quantifying the error done as a function of the reaction kinetic parameters. In a synthetic biology perspective, we show how this can be used to design error correcting terms to compute online functions involving derivatives with CRNs. In the systems biology perspective, we give the list of models in BioModels containing (in the sense of subgraph epimorphisms) the core derivative CRN, most of which being models of oscillators and control systems in the cell, and discuss in detail two such examples: one model of the circadian clock and one model of a bistable switch.
This provides one more brick to our vision of the cell as an analog chemical computer, calculating with proteins, and changing of programs according to gene expression 25.
8.4 Trap spaces of Boolean networks are conflict-free siphons of their Petri net encoding
Participants: Sylvain Soliman.
Boolean network modeling of gene regulation but also of post-transcriptomic systems has proven over the years that it can bring powerful analyses and corresponding insight to the many cases where precise biological data is not sufficiently available to build a detailed quantitative model. Besides simulation, the analysis of such models is mostly based on attractor computation, since those correspond roughly to observable biological phenotypes. The recent use of trap spaces made a real breakthrough in that field allowing to consider medium-sized models that used to be out of reach. However, with the continuing increase in model size and complexity of Boolean update functions, the state-of-the-art computation of minimal trap spaces based on prime implicants shows its limits due to the difficulty of the prime-implicant computation.
In 21, we explore and prove for the first time a connection between trap spaces of a general Boolean network and siphons of its Petri net encoding. Besides important theoretical applications in studying properties of trap spaces, the connection enables us to propose an alternative approach to compute minimal trap spaces, and hence complex attractors, of a general Boolean network. It replaces the need for prime implicants by a completely different technique, namely the enumeration of maximal siphons in the Petri net encoding of the original model. We then demonstrate its efficiency and compare it to the state-of-the-art methods on a large collection of real-world and randomly generated models.
8.5 Efficient Enumeration of Fixed Points in Complex Boolean Networks Using Answer Set Programming
Participants: Sylvain Soliman.
Boolean Networks (BNs) are an efficient modeling formalism with applications in various research fields such as mathematics, computer science, and more recently systems biology. One crucial problem in the BN research is to enumerate all fixed points, which has been proven crucial in the analysis and control of biological systems. Indeed, in that field, BNs originated from the pioneering work of R. Thomas on gene regulation and from the start were characterized by their asymptotic behavior: complex attractors and fixed points. The former being notably more difficult to compute exactly, and specific to certain biological systems, the computation of stable states (fixed points) has been the standard way to analyze those BNs for years. However, with the increase in model size and complexity of Boolean update functions, the existing methods for this problem show their limitations. To our knowledge, the most efficient state-of-the-art methods for the fixed point enumeration problem rely on Answer Set Programming (ASP).
In 24, we propose two new efficient ASP-based methods to solve this problem. We evaluate them on both real-world and pseudo-random models, showing that they vastly outperform four state-of-the-art methods as well as can handle very large and complex models.
8.6 Hybrid computational modeling highlights reverse warburg effect in breast cancer-associated fibroblasts
Participants: Sahar Aghakhani, Anna Niarakis, Sylvain Soliman.
Cancer-associated fibroblasts (CAFs) are amongst the key players of the tumor microenvironment (TME) and are involved in cancer initiation, progression, and resistance to therapy. They exhibit aggressive phenotypes affecting extracellular matrix remodeling, angiogenesis, immune system modulation, tumor growth, and proliferation. CAFs phenotypic changes appear to be associated with metabolic alterations, notably a reverse Warburg effect that may drive fibroblasts transformation. However, its precise molecular mechanisms and regulatory drivers are still under investigation. Deciphering the reverse Warburg effect in breast CAFs may contribute to a better understanding of the interplay between TME and tumor cells, leading to new treatment strategies. In this regard, dynamic modeling approaches able to span multiple biological layers are essential to capture the emergent properties of various biological entities when complex and intertwined pathways are involved.
In 17, we present the first hybrid large-scale computational model for breast CAFs covering major cellular signaling, gene regulation, and metabolic processes. It was generated by combining a cell-and diseasespecific asynchronous Boolean model with a generic core metabolic network leveraging both data-driven and manual curation approaches. This model reproduces the experimentally observed reverse Warburg effect in breast CAFs and further identifies Hypoxia-Inducible Factor 1 (HIF-1) as its key molecular driver. Targeting HIF-1 as part of a TME-centered therapeutic strategy may prove beneficial in the treatment of breast cancer by addressing the reverse Warburg effect. Such findings in CAFs, in light of our previously published results in rheumatoid arthritis synovial fibroblasts, point to a common HIF-1-driven metabolic reprogramming of fibroblasts in breast cancer and rheumatoid arthritis.
In 27, this is generalized to computational modeling of metabolic reprogramming in both rheumatoid arthritis synovial fibroblasts and cancer associated fibroblasts.
8.7 A large-scale Boolean model of the rheumatoid arthritis fibroblast-like synoviocytes predicts drug synergies in the arthritic joint
Participants: Anna Niarakis, Sylvain Soliman.
Rheumatoid arthritis (RA) is a complex autoimmune disease with an unknown aetiology. However, rheumatoid arthritis fibroblast-like synoviocytes (RA-FLS) play a significant role in initiating and perpetuating destructive joint inflammation by expressing immuno-modulating cytokines, adhesion molecules, and matrix remodelling enzymes. In addition, RA-FLS are primary drivers of inflammation, displaying high proliferative rates and an apoptosis-resistant phenotype. Thus, RA-FLS-directed therapies could become a complementary approach to immune-directed therapies by predicting the optimal conditions that would favour RA-FLS apoptosis, limit inflammation, slow the proliferation rate and minimise bone erosion and cartilage destruction.
In 19, we present a large-scale Boolean model for RA-FLS that consists of five submodels focusing on apoptosis, cell proliferation, matrix degradation, bone erosion and inflammation. The five-phenotype-specific submodels can be simulated independently or as a global model. In silico simulations and perturbations reproduced the expected biological behaviour of the system under defined initial conditions and input values. The model was then used to mimic the effect of mono or combined therapeutic treatments and predict novel targets and drug candidates through drug repurposing analysis.
8.8 Reactmine: a statistical search algorithm for inferring chemical reactions from time series data
Participants: François Fages, Julien Martinelli, Sylvain Soliman.
Inferring chemical reaction networks (CRN) from concentration time series is a challenge encouraged by the growing availability of quantitative temporal data at the cellular level. This motivates the design of algorithms to infer the preponderant reactions between the molecular species observed in a given biochemical process, and build CRN structure and kinetics models. Existing ODE-based inference methods such as SINDy resort to least square regression combined with sparsity-enforcing penalization, such as Lasso. However, we observe that these methods fail to learn sparse models when the input time series are only available in wild type conditions, i.e. without the possibility to play with combinations of zeroes in the initial conditions.
In 31, we present a CRN inference algorithm which enforces sparsity by inferring reactions in a sequential fashion within a search tree of bounded depth, ranking the inferred reaction candidates according to the variance of their kinetics on their supporting transitions, and re-optimizing the kinetic parameters of the CRN candidates on the whole trace in a final pass. We show that Reactmine succeeds both on simulation data by retrieving hidden CRNs where SINDy fails, and on two real datasets, one of fluorescence videomicroscopy of cell cycle and circadian clock markers, the other one of biomedical measurements of systemic circadian biomarkers possibly acting on clock gene expression in peripheral organs, by inferring preponderant regulations in agreement with previous model-based analyses. The code is available at together with introductory notebooks. , a statistical constraint-based search algorithm is introduced to learn chemical reaction networks from single time series data, and compared to state-of-the-art SINDy algorithm to learn differential equations from time series data.
8.9 Neural-based classification rule learning for sequential data
Participants: Marine Collery, François Fages.
Discovering interpretable patterns for classification of sequential data is of key importance for a variety of fields, ranging from genomics to fraud detection or more generally interpretable decision-making.
In 22, we propose a novel differentiable fully interpretable method to discover both local and global patterns (i.e. catching a relative or absolute temporal dependency) for rule-based binary classification. It consists of a convolutional binary neural network with an interpretable neural filter and a training strategy based on dynamically-enforced sparsity. We demonstrate the validity and usefulness of the approach on synthetic datasets and on an open-source peptides dataset. Key to this end-to-end differentiable method is that the expressive patterns used in the rules are learned alongside the rules themselves.
In 28, this approach is compared to other approaches to rule learning from sequential data.
9 Bilateral contracts and grants with industry
9.1 Bilateral contracts with industry
9.1.1 Institut de Recherches Servier
Participants: François Fages, Alexandre Than-Lhernould, Mathieu Hemery, Julien Martinelli, Sylvain Soliman.
Cifre PhD thesis of Alexandre Than-Lhernould on "Inference of biological models from temporal data for drug screening".
9.1.2 Johnson&Johnson Santé Beauté France
Participants: François Fages, Elea Greugny, Mathieu Hemery, Sylvain Soliman.
Cifre PhD thesis of Eléa Thibault-Greugny on "Computational modeling approaches to multifactorial aspects of atopic dermatitis" defended in 2022.
9.1.3 IBM research, France
Participants: Marine Collery, François Fages, Sylvain Soliman.
PhD thesis of Marine Collery at IBM France on "Expressive classification rule learning with an emphasis on learning from sequential data."28.
10 Partnerships and cooperations
10.1 International research visitors
10.1.1 Visits of international scientists
We had the visit of Corentin Briat, ETH Zurich, Switzerland.
10.2 European initiatives
10.2.1 ERC Starting Grant BridgingScales
Participants: Guillaume Ballif, Henri Mermoz Kouyé, Jakob Ruess.
ERC Starting Grant BridgingScales - From single cells to microbial consortia: bridging the gaps between synthetic circuit design and emerging dynamics of heterogeneous populations (2023-2028) coord. Jakob Ruess.
10.3 National initiatives
10.3.1 ANR Project: Opt-MC
Participants: Guillaume Ballif, Henri Mermoz Kouyé, Jakob Ruess.
ANR Opt-MC - Optogenetic control of microbial communities (2023-2026) coord. Jakob Ruess, with Frédéric Bonnans, Laurent Pfeiffer (DISCO).
10.3.2 ANR Project: Difference
Participants: François Fages, Mathieu Hemery, Sylvain Soliman.
ANR ifference on "Complexity theory with discrete ODEs", coordinated by Olivier Bournez, Ecole Polytechnique, with F. Fages, F. Chyzak (MATEXP), A. Durand Univ. Paris-Diderot, F. Madelaine P. Valarché Univ. Créteil, Jerôme Durand-Lose, Orléans -Moulay Barkatou Thomas Cluzeau, Limoges - Mathieu Sablik, Toulouse.
11 Dissemination
Participants: François Fages, Mathieu Hemery, Henri Mermoz Kouyé, Anna Niarakis, Jakob Ruess, Sylvain Soliman.
11.1 Promoting scientific activities
11.1.1 Scientific events: organisation
Jakob Ruess was organizer of the department seminar series of the Department of Computational Biology at Institut Pasteur.
General chair, scientific chair
- François Fages is chairman of the Steering Committee of CMSB international conference.
- Anna Niaraki was co-chair of the Digital Twin Symposium at Institut Pascal, Orsay.
Member of the organizing committees
François Fages was member of the scientific committee of the summer school Modélisation formelle de réseaux de régulation biologique, Ile de Porquerolles, 4-9 June 2023.
Member of the conference program committees
- François Fages and Jakob Ruess were members of the program committee of CMSB 2023.
- Sylvain Soliman was member of the program committee of BIOINFORMATICS (BIOSTEC) 2023.
Reviewer
11.1.2 Journal
Member of the editorial boards
François Fages is member of the Editorial board of Royal Society Open Science
Reviewer - reviewing activities
- François Fages made reviews for JACM, RSOS.
- Mathieu Hemery made reviews for Natural Computing, RSOS, Acta Biotheoretica, PR Research.
- Jakob Ruess was reviewer for Automatica.
- Sylvain Soliman was a reviewer for JOMB, Bioinformatics, PLoS Computational Biology.
11.1.3 Invited talks
- Guillaume Ballif gave an invited presentation at the Mathematical Seminar of the Inria team MUSCA.
- François Fages gave invited talks at the Thematic workshop on networks and biological models inference, CIRM, Marseille, at the Digital Twin Symposium, Institut Pascal, Orsay, at the Scryer Prolog meeting in Düsseldorf, Germany, and at the chemical reation network visioconference meeting in Bangalore, India.
- Mathieu Hemery gave an invited talk at the Thematic workshop on networks and biological models inference, CIRM, Marseille.
- Henri Mermoz Kouyé gave an invited presentation at the INRAE team MAIAGE.
- Jakob Ruess gave invited presentations at the 10th International Workshop on Applied Probability (Thessaloniki, Greece), at the Second SOS Workshop on Self-organization in space and time (Darmstadt, Germany), and in the Seminar on Biological Control Systems (online).
- Sylvain Soliman gave an invited talk at the Digital Twin Symposium, Institut Pascal, Orsay.
11.1.4 Scientific expertise
François Fages evaluated one CIFRE PhD thesis proposal, and was member of the jury for the recruitment of one Maître de conférence at the University of Nice Alpes Provence côte d'azur.
11.2 Teaching - Supervision - Juries
11.2.1 Teaching
- Summer school: François Fages gave two lectures at the summer school Modélisation formelle de réseaux de régulation biologique, Ile de Porquerolles, 4-9 June 2023.
- Master 2: François Fages (coordinator 24h and teacher 12h), Jérôme Feret (12h) C2-19 - Biochemical Programming, Master Parisien de Recherche en Informatique (MPRI), Paris.
- Cycle ingénieur Ecole Polytechnique, Master 1: François Fages (coordinator, 18h lectures, 14h TD), Mathieu Hemery (6h TD) INF555 - Constraint-based Modeling and Algorithms for Decision Making ProblemsMaster Artificial Intelligence, Master Science and Technology, Ecole Polytechnique.
- Bachelor 3: François Fages (co-coordinator, 12h lectures), Mathieu Hemery (12h TD), CSE 307 - Relational Programming, Ecole Polytechnique.
- Anna Niarakis is the pedagogical responsible for the Double Bachelor in Life Sciences and Informatics at Paris Saclay University.
- François Fages supervised the Master 2 internship of Hugo Buscemi.
11.2.2 Juries
- François Fages was reviewer of the PhD theses of Albin Salazar (ENS Paris), Athénaïs Vaginay (Nancy), and examiner of the PhD thesis of Maxime Mahout (UPSaclay).
- François Fages was member of the TAC committee of Mitsuhiro Odaka (Univ. Tokyo, Japan, and Nantes).
- Jakob Ruess is member of the TAC committee of Emrys Reginato (Inria Grenoble).
- Jakob Ruess was examiner of the PhD thesis and defense of Kaan Ocal (University of Edinburgh).
- Sylvain Soliman was reviewer of the PhD thesis of Mitsuhiro Okada (SOKENDAI, Japan, and École Centrale Nantes, France), member of the CRCN-TH 2023 (handicapped researcher) jury for Inria.
11.3 Popularization
11.3.1 Internal or external Inria responsibilities
Mathieu Hemery took the co-direction of the scientific mediation of the Centre de Saclay along with Irene Vignon-Clementel (EPI SIMBIOTX).
11.3.2 Articles and contents
François Fages published a large audience chapter on the foundations of biochemical programming which makes of programming a natural science... 25.
11.3.3 Education
François Fages received five 12yo scool students to investigate the question of whether one can program any function on the integer numbers ? And showing that the answer is negative by proving Cantor's theorem using diagonal argument, an easy proof of a hard theorem they can redo to their Professor of Mathematics.
11.3.4 Interventions
Mathieu Hemery participated to the "Fête de la Science" on the IPP campus.
12 Scientific production
12.1 Major publications
- 1 articleMetabolic Reprogramming in Rheumatoid Arthritis Synovial Fibroblasts: a Hybrid Modeling Approach.PLoS Computational Biology1812December 2022, e1010408HALDOI
- 2 articleGraphical Requirements for Multistationarity in Reaction Networks and their Verification in BioModels.Journal of Theoretical Biology459December 2018, 79--89HALDOIback to text
- 3 articleComputer‐aided biochemical programming of synthetic microreactors as diagnostic devices.Molecular Systems Biology144April 2018HALDOIback to textback to text
- 4 inproceedingsGraphical Conditions for Rate Independence in Chemical Reaction Networks.Proceedings CMSB 2020: The 18th International Conference on Computational Methods in Systems BiologyCMSB 2020 - 18th International Conference on Computational Methods in Systems BiologyKonstanz / Online, GermanySeptember 2020HALback to text
- 5 articleInferring reaction systems from ordinary differential equations.Theoretical Computer Science599September 2015, 64--78HALDOIback to text
- 6 inproceedingsStrong Turing Completeness of Continuous Chemical Reaction Networks and Compilation of Mixed Analog-Digital Programs.CMSB 2017 - 15th International Conference on Computational Methods in Systems BiologyLecture Notes in Computer ScienceProceedings of the fiveteen international conference on Computational Methods in Systems Biology, CMSB 201710545Darmstadt, GermanySeptember 2017, 108-127HALback to text
- 7 articleInfluence Networks compared with Reaction Networks: Semantics, Expressivity and Attractors.IEEE/ACM Transactions on Computational Biology and BioinformaticsPP992018, 1-14HALDOIback to text
- 8 thesisComputational modeling approaches to multifactorial aspects of atopic dermatitis.Institut Polytechnique de ParisOctober 2022HALback to text
- 9 articleMathematical modeling of the microtubule detyrosination/tyrosination cycle for cell-based drug screening design.PLoS Computational Biology186June 2022, e1010236HALDOIback to textback to text
- 10 inproceedingsAlgebraic Biochemistry: a Framework for Analog Online Computation in Cells.CMSB 2022 - International Conference on Computational Methods in Systems BiologyBucarest, RomaniaSeptember 2022HALback to text
- 11 thesisOn learning mechanistic models from time series data with applications to personalised chronotherapies.Institut Polytechnique de ParisFebruary 2022HALback to text
- 12 articleOn Enumerating Minimal Siphons in Petri nets using CLP and SAT solvers: Theoretical and Practical Complexity.Constraints212April 2016, 251--276HALDOIback to text
- 13 articleCOVID19 Disease Map, a computational knowledge repository of virus-host interaction mechanisms.Molecular Systems Biology1710October 2021, e10387HALDOIback to text
- 14 articleComputational modeling predicts impaired barrier function and higher sensitivity to skin inflammation following pH elevation.Experimental DermatologyNovember 2022HALDOIback to textback to text
- 15 articleLogical model specification aided by model- checking techniques: application to the mammalian cell cycle regulation.Bioinformatics32172016, i772-i780HALDOIback to text
- 16 articleModel-based investigation of the circadian clock and cell cycle coupling in mouse embryonic fibroblasts: Prediction of RevErb-α up-regulation during mitosis.BioSystems149November 2016, 59--69HALDOIback to textback to text
12.2 Publications of the year
International journals
International peer-reviewed conferences
Scientific book chapters
Edition (books, proceedings, special issue of a journal)
Doctoral dissertations and habilitation theses
Reports & preprints