2024Activity reportProject-TeamSTARS
RNSR: 201221015V- Research center Inria Centre at Université Côte d'Azur
- Team name: Spatio-Temporal Activity Recognition of Social interactions
- Domain:Perception, Cognition and Interaction
- Theme:Vision, perception and multimedia interpretation
Keywords
Computer Science and Digital Science
- A5.3. Image processing and analysis
- A5.3.3. Pattern recognition
- A5.4. Computer vision
- A5.4.2. Activity recognition
- A5.4.4. 3D and spatio-temporal reconstruction
- A5.4.5. Object tracking and motion analysis
- A9. Artificial intelligence
- A9.1. Knowledge
- A9.2. Machine learning
- A9.3. Signal analysis
- A9.8. Reasoning
Other Research Topics and Application Domains
- B1. Life sciences
- B1.2. Neuroscience and cognitive science
- B1.2.2. Cognitive science
- B2. Health
- B2.1. Well being
- B7. Transport and logistics
- B7.1.1. Pedestrian traffic and crowds
- B8. Smart Cities and Territories
- B8.4. Security and personal assistance
1 Team members, visitors, external collaborators
Research Scientists
- François Brémond [Team leader, INRIA, Senior Researcher]
- Michal Balazia [INRIA, ISFP]
- Antitza Dantcheva [INRIA, Senior Researcher, from Oct 2024]
- Antitza Dantcheva [INRIA, Researcher, until Sep 2024]
- Monique Thonnat [INRIA, Emeritus, from Jun 2024]
- Monique Thonnat [INRIA, Senior Researcher, until May 2024]
Post-Doctoral Fellows
- Baptiste Chopin [INRIA, Post-Doctoral Fellow]
- Olivier Huynh [INRIA, Post-Doctoral Fellow]
PhD Students
- Tanay Agrawal [INRIA]
- Abid Ali [UNIV COTE D'AZUR]
- Yuan Gao [INRAE]
- Mohammed Guermal [INRIA, until Jul 2024]
- Snehashis Majhi [INRIA]
- Tomasz Stanczyk [INRIA]
- Valeriya Strizhkova [INRIA]
- Charbel Yahchouchi [Supponor, CIFRE, from May 2024 until Oct 2024]
- Di Yang [INRIA, until Feb 2024]
- Seongro Yoon [INRIA, from Oct 2024]
Technical Staff
- Mahmoud Ali [INRIA, Engineer]
- Ezem Sura Ekmekci [INRIA, Engineer, from Nov 2024]
- Ezem Sura Ekmekci [INRIA, Engineer, until Sep 2024]
- Yoann Torrado [INRIA, Engineer]
Interns and Apprentices
- Maheswar Bora [INRIA, Intern, from Jul 2024]
- Junuk Cha [INRIA, from Sep 2024 until Nov 2024]
- Antonio Cimino [INRIA, Intern, from May 2024 until Aug 2024]
- Anil Egin [INRIA, Intern, from Nov 2024]
- Lucas Ferez [INRIA, Intern, from Apr 2024 until May 2024]
- Rui-Han Lee [INRIA, Intern, until Jan 2024]
- Cyprien Michel-Deletie [ENS DE LYON, Intern, until Mar 2024]
- Dorval De Celestin Mobendza [INRIA, Intern, from May 2024 until Aug 2024]
- David Prigodin [UNIV COTE D'AZUR, Intern, from Mar 2024 until Apr 2024]
- Nabyl Quignon [INRIA, from Dec 2024]
- Dominick Reilly [INRIA, Intern, until May 2024]
- Aglind Reka [UNIV COTE D'AZUR, Intern, from May 2024 until Oct 2024]
- Sanya Sinha [INRIA, Intern, until Jun 2024]
- Sujith Sai Sripadam [INRIA, Intern, from Jul 2024 until Aug 2024]
- Utkarsh Tiwari [INRIA, Intern, from Jul 2024 until Aug 2024]
Administrative Assistant
- Sandrine Boute [INRIA]
Visiting Scientists
- Diana Borza [Babeș Bolyai University Cluj-Napoca, from May 2024 until Jul 2024]
- Giacomo D'Amicantonio [TU Eindhoven, from Aug 2024]
- Salvatore Fiorilla [UNIV BOLOGNA, from Sep 2024]
- Sabine Moisan [Retired, Emeritus]
- Jean-Paul Rigault [Retired]
External Collaborators
- Nibras Abo Alzahab [UNIV COTE D'AZUR, until Jun 2024]
- David Anghelone [BANQUE LOMBARD ODIER, until Jun 2024]
- Laura Ferrari [Scuola Superiore Sant'Anna]
- Rachid Guerchouche [CoBTeK]
- Alexandra Konig [CHU NICE, from Mar 2024]
- Hali Lindsay [KIT - ALLEMAGNE, from Jun 2024]
- Philippe Robert [CoBTeK]
- Yaohui Wang [Shanghai AI Lab]
2 Overall objectives
2.1 Presentation
The STARS (Spatio-Temporal Activity Recognition Systems) team focuses on the design of cognitive vision systems for Activity Recognition. More precisely, we are interested in the real-time semantic interpretation of dynamic scenes observed by video cameras and other sensors. We study long-term spatio-temporal activities performed by agents such as human beings, animals or vehicles in the physical world. The major issue in semantic interpretation of dynamic scenes is to bridge the gap between the subjective interpretation of data and the objective measures provided by sensors. To address this problem Stars develops new techniques in the field of computer vision, machine learning and cognitive systems for physical object detection, activity understanding, activity learning, vision system design and evaluation. We focus on two principal application domains: visual surveillance and healthcare monitoring.
2.2 Research Themes
Stars is focused on the design of cognitive systems for Activity Recognition. We aim at endowing cognitive systems with perceptual capabilities to reason about an observed environment, to provide a variety of services to people living in this environment while preserving their privacy. In today's world, a huge amount of new sensors and new hardware devices are currently available, addressing potentially new needs of the modern society. However, the lack of automated processes (with no human interaction) able to extract a meaningful and accurate information (i.e. a correct understanding of the situation) has often generated frustrations among the society and especially among older people. Therefore, Stars objective is to propose novel autonomous systems for the real-time semantic interpretation of dynamic scenes observed by sensors. We study long-term spatio-temporal activities performed by several interacting agents such as human beings, animals and vehicles in the physical world. Such systems also raise fundamental software engineering problems to specify them as well as to adapt them at run time.
We propose new techniques at the frontier between computer vision, knowledge engineering, machine learning and software engineering. The major challenge in semantic interpretation of dynamic scenes is to bridge the gap between the task dependent interpretation of data and the flood of measures provided by sensors. The problems we address range from physical object detection, activity understanding, activity learning to vision system design and evaluation. The two principal classes of human activities we focus on, are assistance to older adults and video analytics.
Typical examples of complex activity are shown in Figure 1 and Figure 2 for a homecare application (See Toyota Smarthome Dataset here). In this example, the duration of the monitoring of an older person apartment could last several months. The activities involve interactions between the observed person and several pieces of equipment. The application goal is to recognize the everyday activities at home through formal activity models (as shown in Figure 3) and data captured by a network of sensors embedded in the apartment. Here typical services include an objective assessment of the frailty level of the observed person to be able to provide a more personalized care and to monitor the effectiveness of a prescribed therapy. The assessment of the frailty level is performed by an Activity Recognition System which transmits a textual report (containing only meta-data) to the general practitioner who follows the older person. Thanks to the recognized activities, the quality of life of the observed people can thus be improved and their personal information can be preserved.
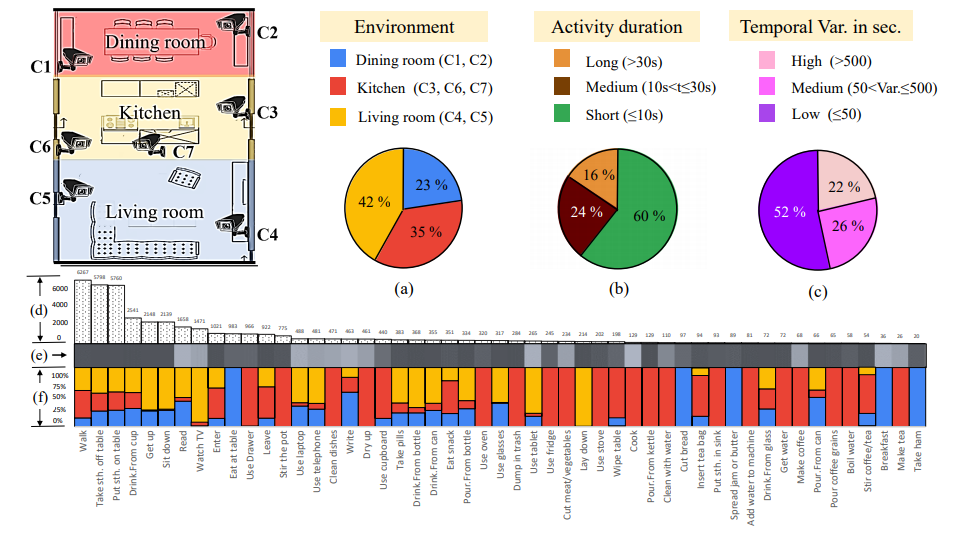
.png)
Activity (PrepareMeal, | |
PhysicalObjects( | (p : Person), (z : Zone), (eq : Equipment)) |
Components( | (s_inside : InsideKitchen(p, z)) |
(s_close : CloseToCountertop(p, eq)) | |
(s_stand : PersonStandingInKitchen(p, z))) | |
Constraints( | (z->Name = Kitchen) |
(eq->Name = Countertop) | |
(s_close->Duration >= 100) | |
(s_stand->Duration >= 100)) | |
Annotation( | AText("prepare meal"))) |
The ultimate goal is for cognitive systems to perceive and understand their environment to be able to provide appropriate services to a potential user. An important step is to propose a computational representation of people activities to adapt these services to them. Up to now, the most effective sensors have been video cameras due to the rich information they can provide on the observed environment. These sensors are currently perceived as intrusive ones. A key issue is to capture the pertinent raw data for adapting the services to the people while preserving their privacy. We plan to study different solutions including of course the local processing of the data without transmission of images and the utilization of new compact sensors developed for interaction (also called RGB-Depth sensors, an example being the Kinect) or networks of small non-visual sensors.
2.3 International and Industrial Cooperation
Our work has been applied in the context of more than 10 European projects such as COFRIEND, ADVISOR, SERKET, CARETAKER, VANAHEIM, SUPPORT, DEM@CARE, VICOMO, EIT Health.
We had or have industrial collaborations in several domains: transportation (CCI Airport Toulouse Blagnac, SNCF, Inrets, Alstom, Ratp, Toyota, GTT (Italy), Turin GTT (Italy)), banking (Crédit Agricole Bank Corporation, Eurotelis and Ciel), security (Thales R&T FR, Thales Security Syst, EADS, Sagem, Bertin, Alcatel, Keeneo), multimedia (Thales Communications), civil engineering (Centre Scientifique et Technique du Bâtiment (CSTB)), computer industry (BULL), software industry (AKKA), hardware industry (ST-Microelectronics) and health industry (Philips, Link Care Services, Vistek).
We have international cooperations with research centers such as Reading University (UK), ENSI Tunis (Tunisia), Idiap (Switzerland), Multitel (Belgium), National Cheng Kung University, National Taiwan University (Taiwan), MICA (Vietnam), IPAL, I2R (Singapore), University of Southern California, University of South Florida (USA), Michigan State University (USA), Chinese Academy of Sciences (China), IIIT Delhi (India), Hochschule Darmstadt (Germany), Fraunhofer Institute for Computer Graphics Research IGD (Germany).
3 Research program
3.1 Introduction
Stars follows three main research directions: perception for activity recognition, action recognition and semantic activity recognition. These three research directions are organized following the workflow of activity recognition systems: First, the perception and the action recognition directions provide new techniques to extract powerful features, whereas the semantic activity recognition research direction provides new paradigms to match these features with concrete video analytics and healthcare applications.
Transversely, we consider a new research axis in machine learning, combining a priori knowledge and learning techniques, to set up the various models of an activity recognition system. A major objective is to automate model building or model enrichment at the perception level and at the understanding level.
3.2 Perception for Activity Recognition
Participants: François Brémond, Antitza Dantcheva, Michal Balazia, Monique Thonnat.
Keywords: Activity Recognition, Scene Understanding, Machine Learning, Computer Vision, Cognitive Vision Systems, Software Engineering.
3.2.1 Introduction
Our main goal in perception is to develop vision algorithms able to address the large variety of conditions characterizing real world scenes in terms of sensor conditions, hardware requirements, lighting conditions, physical objects, and application objectives. We have also several issues related to perception which combine machine learning and perception techniques: learning people appearance, parameters for system control and shape statistics.
3.2.2 Appearance Models and People Tracking
An important issue is to detect in real-time physical objects from perceptual features and predefined 3D models. It requires finding a good balance between efficient methods and precise spatio-temporal models. Many improvements and analysis need to be performed in order to tackle the large range of people detection scenarios.
Appearance models. In particular, we study the temporal variation of the features characterizing the appearance of a human. This task could be achieved by clustering potential candidates depending on their position and their reliability. This task can provide any people tracking algorithms with reliable features allowing for instance to (1) better track people or their body parts during occlusion, or to (2) model people appearance for re-identification purposes in mono and multi-camera networks, which is still an open issue. The underlying challenge of the person re-identification problem arises from significant differences in illumination, pose and camera parameters. The re-identification approaches have two aspects: (1) establishing correspondences between body parts and (2) generating signatures that are invariant to different color responses. As we have already several descriptors which are color invariant, we now focus more on aligning two people detection and on finding their corresponding body parts. Having detected body parts, the approach can handle pose variations. Further, different body parts might have different influence on finding the correct match among a whole gallery dataset. Thus, the re-identification approaches have to search for matching strategies. As the results of the re-identification are always given as the ranking list, re-identification focuses on learning to rank. "Learning to rank" is a type of machine learning problem, in which the goal is to automatically construct a ranking model from a training data.
Therefore, we work on information fusion to handle perceptual features coming from various sensors (several cameras covering a large-scale area or heterogeneous sensors capturing more or less precise and rich information). New 3D RGB-D sensors are also investigated, to help in getting an accurate segmentation for specific scene conditions.
Long-term tracking. For activity recognition we need robust and coherent object tracking over long periods of time (often several hours in video surveillance and several days in healthcare). To guarantee the long-term coherence of tracked objects, spatio-temporal reasoning is required. Modeling and managing the uncertainty of these processes is also an open issue. In Stars we propose to add a reasoning layer to a classical Bayesian framework modeling the uncertainty of the tracked objects. This reasoning layer can take into account the a priori knowledge of the scene for outlier elimination and long-term coherency checking.
Controlling system parameters. Another research direction is to manage a library of video processing programs. We are building a perception library by selecting robust algorithms for feature extraction, by ensuring they work efficiently with real time constraints and by formalizing their conditions of use within a program supervision model. In the case of video cameras, at least two problems are still open: robust image segmentation and meaningful feature extraction. For these issues, we are developing new learning techniques.
3.3 Action Recognition
Participants: François Brémond, Antitza Dantcheva, Michal Balazia, Monique Thonnat.
Keywords: Machine Learning, Computer Vision, Cognitive Vision Systems.
3.3.1 Introduction
Due to the recent development of high processing units, such as GPU, it is now possible to extract meaningful features directly from videos (e.g. video volume) to recognize reliably short actions. Action Recognition benefits also greatly from the huge progress made recently in Machine Learning (e.g. Deep Learning), especially for the study of human behavior. For instance, Action Recognition enables to measure objectively the behavior of humans by extracting powerful features characterizing their everyday activities, their emotion, eating habits and lifestyle, by learning models from a large amount of data from a variety of sensors, to improve and optimize for example, the quality of life of people suffering from behavior disorders. However, Smart Homes and Partner Robots have been well advertized but remain laboratory prototypes, due to the poor capability of automated systems to perceive and reason about their environment. A hard problem is for an automated system to cope 24/7 with the variety and complexity of the real world. Another challenge is to extract people fine gestures and subtle facial expressions to better analyze behavior disorders, such as anxiety or apathy. Taking advantage of what is currently studied for self-driving cars or smart retails, there is a large avenue to design ambitious approaches for the healthcare domain. In particular, the advance made with Deep Learning algorithms has already enabled to recognize complex activities, such as cooking interactions with instruments, and from this analysis to differentiate healthy people from the ones suffering from dementia.
To address these issues, we propose to tackle several challenges which are detailed in the following subsections:
3.3.2 Action recognition in the wild
The current Deep Learning techniques are mostly developed to work on few clipped videos, which have been recorded with students performing a limited set of predefined actions in front of a camera with high resolution. However, real life scenarios include actions performed in a spontaneous manner by older people (including people interactions with their environment or with other people), from different viewpoints, with varying framerate, partially occluded by furniture at different locations within an apartment depicted through long untrimmed videos. Therefore, a new dedicated dataset should be collected in a real-world setting to become a public benchmark video dataset and to design novel algorithms for Activities of Daily Living (ADL) activity recognition. A special attention should be taken to anonymize the videos.
3.3.3 Attention mechanisms for action recognition
ADL and video-surveillance activities are different from internet activities (e.g. Sports, Movies, YouTube), as they may have very similar context (e.g. same background kitchen) with high intra-variation (different people performing the same action in different manners), but in the same time low inter-variation, similar ways to perform two different actions (e.g. eating and drinking a glass of water). Consequently, fine-grained actions are badly recognized. So, we will design novel attention mechanisms for action recognition, for the algorithm being able to focus on a discriminative part of the person conducting the action. For instance, we will study attention algorithms, which could focus on the most appropriate body parts (e.g. full body, right hand). In particular, we plan to design a soft mechanism, learning the attention weights directly on the feature map of a 3DconvNet, a powerful convolutional network, which takes as input a batch of videos.
3.3.4 Action detection for untrimmed videos
Many approaches have been proposed to solve the problem of action recognition in short clipped 2D videos, which achieved impressive results with hand-crafted and deep features. However, these approaches cannot address real life situations, where cameras provide online and continuous video streams in applications such as robotics, video surveillance, and smart-homes. Here comes the importance of action detection to help recognizing and localizing each action happening in long videos. Action detection can be defined as the ability to localize starting and ending of each human action happening in the video, in addition to recognizing each action label. There have been few action detection algorithms designed for untrimmed videos, which are based on either sliding window, temporal pooling or frame-based labeling. However, their performance is too low to address real-word datasets. A first task consists in benchmarking the already published approaches to study their limitations on novel untrimmed video datasets, recorded following real-world settings. A second task could be to propose a new mechanism to improve either 1) the temporal pooling directly from the 3DconvNet architecture using for instance Temporal Convolution Networks (TCNs) or 2) frame-based labeling with a clustering technique (e.g. using Fisher Vectors) to discover the sub-activities of interest.
3.3.5 View invariant action recognition
The performance of current approaches strongly relies on the used camera angle: enforcing that the camera angle used in testing is the same (or extremely close to) as the camera angle used in training, is necessary for the approach to perform well. On the contrary, the performance drops when a different camera view-point is used. Therefore, we aim at improving the performance of action recognition algorithms by relying on 3D human pose information. For the extraction of the 3D pose information, several open-source algorithms can be used, such as openpose or videopose3D (from CMU or Facebook research, click here). Also, other algorithms extracting 3d meshes can be used. To generate extra views, Generative Adversial Network (GAN) can be used together with the 3D human pose information to complete the training dataset from the missing view.
3.3.6 Uncertainty and action recognition
Another challenge is to combine the short-term actions recognized by powerful Deep Learning techniques with long-term activities defined by constraint-based descriptions and linked to user interest. To realize this objective, we have to compute the uncertainty (i.e. likelihood or confidence), with which the short-term actions are inferred. This research direction is linked to the next one, to Semantic Activity Recognition.
3.4 Semantic Activity Recognition
Participants: François Brémond, Monique Thonnat.
Keywords: Activity Recognition, Scene Understanding, Computer Vision.
3.4.1 Introduction
Semantic activity recognition is a complex process where information is abstracted through four levels: signal (e.g. pixel, sound), perceptual features, physical objects and activities. The signal and the feature levels are characterized by strong noise, ambiguous, corrupted and missing data. The whole process of scene understanding consists in analyzing this information to bring forth pertinent insight of the scene and its dynamics while handling the low-level noise. Moreover, to obtain a semantic abstraction, building activity models is a crucial point. A still open issue consists in determining whether these models should be given a priori or learned. Another challenge consists in organizing this knowledge in order to capitalize experience, share it with others and update it along with experimentation. To face this challenge, tools in knowledge engineering such as machine learning or ontology are needed.
Thus, we work along the following research axes: high level understanding (to recognize the activities of physical objects based on high level activity models), learning (how to learn the models needed for activity recognition) and activity recognition and discrete event systems.
3.4.2 High Level Understanding
A challenging research axis is to recognize subjective activities of physical objects (i.e. human beings, animals, vehicles) based on a priori models and objective perceptual measures (e.g. robust and coherent object tracks).
To reach this goal, we have defined original activity recognition algorithms and activity models. Activity recognition algorithms include the computation of spatio-temporal relationships between physical objects. All the possible relationships may correspond to activities of interest and all have to be explored in an efficient way. The variety of these activities, generally called video events, is huge and depends on their spatial and temporal granularity, on the number of physical objects involved in the events, and on the event complexity (number of components constituting the event).
Concerning the modeling of activities, we are working towards two directions: the uncertainty management for representing probability distributions and knowledge acquisition facilities based on ontological engineering techniques. For the first direction, we are investigating classical statistical techniques and logical approaches. For the second direction, we built a language for video event modeling and a visual concept ontology (including color, texture and spatial concepts) to be extended with temporal concepts (motion, trajectories, events ...) and other perceptual concepts (physiological sensor concepts ...).
3.4.3 Learning for Activity Recognition
Given the difficulty of building an activity recognition system with a priori knowledge for a new application, we study how machine learning techniques can automate building or completing models at the perception level and at the understanding level.
At the understanding level, we are learning primitive event detectors. This can be done for example by learning visual concept detectors using SVMs (Support Vector Machines) with perceptual feature samples. An open question is how far can we go in weakly supervised learning for each type of perceptual concept (i.e. leveraging the human annotation task). A second direction is to learn typical composite event models for frequent activities using trajectory clustering or data mining techniques. We name composite event a particular combination of several primitive events.
3.4.4 Activity Recognition and Discrete Event Systems
The previous research axes are unavoidable to cope with the semantic interpretations. However, they tend to let aside the pure event driven aspects of scenario recognition. These aspects have been studied for a long time at a theoretical level and led to methods and tools that may bring extra value to activity recognition, the most important being the possibility of formal analysis, verification and validation.
We have thus started to specify a formal model to define, analyze, simulate, and prove scenarios. This model deals with both absolute time (to be realistic and efficient in the analysis phase) and logical time (to benefit from well-known mathematical models providing re-usability, easy extension, and verification). Our purpose is to offer a generic tool to express and recognize activities associated with a concrete language to specify activities in the form of a set of scenarios with temporal constraints. The theoretical foundations and the tools being shared with Software Engineering aspects.
The results of the research performed in perception and semantic activity recognition (first and second research directions) produce new techniques for scene understanding and contribute to specify the needs for new software architectures (third research direction).
4 Application domains
4.1 Introduction
While in our research the focus is to develop techniques, models and platforms that are generic and reusable, we also make efforts in the development of real applications. The motivation is twofold. The first is to validate the new ideas and approaches we introduce. The second is to demonstrate how to build working systems for real applications of various domains based on the techniques and tools developed. Indeed, Stars focuses on two main domains: video analytic and healthcare monitoring.
Domain: Video Analytics
Our experience in video analytic (also referred to as visual surveillance) is a strong basis which ensures both a precise view of the research topics to develop and a network of industrial partners ranging from end-users, integrators and software editors to provide data, objectives, evaluation and funding.
For instance, the Keeneo start-up was created in July 2005 for the industrialization and exploitation of Orion and Pulsar results in video analytic (VSIP library, which was a previous version of SUP). Keeneo has been bought by Digital Barriers in August 2011 and is now independent from Inria. However, Stars continues to maintain a close cooperation with Keeneo for impact analysis of SUP and for exploitation of new results.
Moreover, new challenges are arising from the visual surveillance community. For instance, people detection and tracking in a crowded environment are still open issues despite the high competition on these topics. Also detecting abnormal activities may require to discover rare events from very large video data bases often characterized by noise or incomplete data.
Domain: Healthcare Monitoring
Since 2011, we have initiated a strategic partnership (called CobTek) with Nice hospital (CHU Nice, Prof P. Robert) to start ambitious research activities dedicated to healthcare monitoring and to assistive technologies. These new studies address the analysis of more complex spatio-temporal activities (e.g. complex interactions, long term activities).
4.1.1 Research
To achieve this objective, several topics need to be tackled. These topics can be summarized within two points: finer activity description and longitudinal experimentation. Finer activity description is needed for instance, to discriminate the activities (e.g. sitting, walking, eating) of Alzheimer patients from the ones of healthy older people. It is essential to be able to pre-diagnose dementia and to provide a better and more specialized care. Longer analysis is required when people monitoring aims at measuring the evolution of patient behavioral disorders. Setting up such long experimentation with dementia people has never been tried before but is necessary to have real-world validation. This is one of the challenges of the European FP7 project Dem@Care where several patient homes should be monitored over several months.
For this domain, a goal for Stars is to allow people with dementia to continue living in a self-sufficient manner in their own homes or residential centers, away from a hospital, as well as to allow clinicians and caregivers remotely provide effective care and management. For all this to become possible, comprehensive monitoring of the daily life of the person with dementia is deemed necessary, since caregivers and clinicians will need a comprehensive view of the person's daily activities, behavioral patterns, lifestyle, as well as changes in them, indicating the progression of their condition.
4.1.2 Ethical and Acceptability Issues
The development and ultimate use of novel assistive technologies by a vulnerable user group such as individuals with dementia, and the assessment methodologies planned by Stars are not free of ethical, or even legal concerns, even if many studies have shown how these Information and Communication Technologies (ICT) can be useful and well accepted by older people with or without impairments. Thus, one goal of Stars team is to design the right technologies that can provide the appropriate information to the medical carers while preserving people privacy. Moreover, Stars will pay particular attention to ethical, acceptability, legal and privacy concerns that may arise, addressing them in a professional way following the corresponding established EU and national laws and regulations, especially when outside France. Now, Stars can benefit from the support of the COERLE (Comité Opérationnel d'Evaluation des Risques Légaux et Ethiques) to help it to respect ethical policies in its applications.
As presented in 2, Stars aims at designing cognitive vision systems with perceptual capabilities to monitor efficiently people activities. As a matter of fact, vision sensors can be seen as intrusive ones, even if no images are acquired or transmitted (only meta-data describing activities need to be collected). Therefore, new communication paradigms and other sensors (e.g. accelerometers, RFID (Radio Frequency Identification), and new sensors to come in the future) are also envisaged to provide the most appropriate services to the observed people, while preserving their privacy. To better understand ethical issues, Stars members are already involved in several ethical organizations. For instance, F. Brémond has been a member of the ODEGAM - “Commission Ethique et Droit” (a local association in Nice area for ethical issues related to older people) from 2010 to 2011 and a member of the French scientific council for the national seminar on “La maladie d'Alzheimer et les nouvelles technologies - Enjeux éthiques et questions de société” in 2011. This council has in particular proposed a chart and guidelines for conducting researches with dementia patients.
For addressing the acceptability issues, focus groups and HMI (Human Machine Interaction) experts, are consulted on the most adequate range of mechanisms to interact and display information to older people.
5 Social and environmental responsibility
5.1 Footprint of research activities
We have limited our travels by reducing our physical participation to conferences and to international collaborations.
5.2 Impact of research results
We have been involved for many years in promoting public transportation by improving safety onboard and in station. Moreover, we have been working on pedestrian detection for self-driving cars, which will help also reducing the number of individual cars.
6 Highlights of the year
- We have created a new research team in November 2024 after the Spatio-Temporal Activity Recognition Systems (STARS) team reached its 12 years duration at the end of 2024.
- The new Spatio-Temporal Activity Recognition of Social Interactions (STARS) research project-team focuses on the design of computer vision methods for real-time understanding of social interactions observed by sensors. Our objective is to propose new algorithms to analyze the behavior of people suffering from behavioral disorders, in order to improve their quality of life. We will study long-term spatio-temporal interactions performed by humans in their natural environment. We will address this challenge by proposing novel deep-learning architectures to model behavioral traits such as facial expression, gaze, gestures, body behavior, and body language. To cope with the limited amount of available data and the privacy issues of medical data, we propose data generation for data augmentation and anonymization. Another important challenge is to make the link between collected data, medical diagnosis, and ultimately treatments. To validate our research, we will work closely with our clinical partners, in particular those of the Nice Hospital.
- Antitza Dantcheva was promoted to Directrice de Recherche (DR2).
7 New software, platforms, open data
7.1 Open data
We have provided two benchmark datasets.
Stress ID Dataset: a Multimodal Dataset for Stress Identification
-
Contributors:
Hava Chaptoukaev, Valeriya Strizhkova, Michele Panariello, Bianca Dalpaos, Aglind Reka, Valeria Manera, Susanne Thummler, Esma Ismailova, Nicholas W., Francois Bremond, Massimiliano Todisco, Maria A Zuluaga and Laura M. Ferrari
-
Description:
It contains RGB facial video, audio and physiological signals (ECG, EDA, Respiration). Different stress-inducing stimuli are used: emotional video-clips, cognitive tasks and public speaking. The total dataset consists of recordings from 65 participants that performed 11 tasks. Each task is labeled by the subjects in terms of stress, relaxation, arousal, and valence. The experimental set-up ensures synchronized, high-quality, and low noise data.
- Project link:
-
Publications:
https://nips.cc/virtual/2023/poster/73454
-
Contact:
stressid.dataset@inria.fr
-
Release contributions:
The Dataset is licensed for non-commercial scientific research purposes.
Toyota Smarthome Datasets: Real-World Activities of Daily Living.
-
Contributors:
R. Dai, S. Das, S. Sharma, L. Minciullo, L. Garattoni, F. Bremond and G. Francesca
-
Description:
Smarthome has been recorded in an apartment equipped with 7 Kinect v1 cameras. It contains the common daily living activities of 18 subjects. The subjects are senior people in the age range 60-80 years old. The dataset has a resolution of 640×480 and offers 3 modalities: RGB + Depth + 3D Skeleton. The 3D skeleton joints were extracted from RGB. For privacy-preserving reasons, the face of the subjects is blurred. Currently, two versions of the dataset are provided: Toyota Smarthome Trimmed and Toyota Smarthome Untrimmed.
-
Dataset DOI:
10.1109/TPAMI.2022.3169976
- Project link:
-
Publications:
Toyota Smarthome Untrimmed: Real-World Untrimmed Videos for Activity Detection, PAMI 2022.
-
Contact:
toyotasmarthome@inria.fr
-
Release contributions:
The Dataset is licensed for non-commercial scientific research purposes.
8 New results
8.1 Introduction
This year Stars has proposed new results related to its three main research axes: (i) perception for activity recognition, (ii) action recognition and (iii) semantic activity recognition.
Perception for Activity Recognition
Participants: François Brémond, Antitza Dantcheva, Michal Balazia, Baptiste Chopin, Di Yang, Abid Ali, Olivier Huynh, Tomasz Stanczyk, Sanya Sinha.
The new results for perception for activity recognition are:
- Identifying Surgical Instruments in Pedagogical Cataract Surgery Videos through an Optimized Aggregation Network (see 8.2)
- Temporally Propagated Masks as an Association Cue for Multi-Object Tracking (see 8.3)
- Re-Evaluating Re-ID in Multi-Object Tracking (see 8.4)
- Anti-forgetting adaptation for unsupervised person re-identification (see 8.5)
- Enhancing age estimation by regularizing with look-alike references and ensuring ordinal continuity (see 8.6)
- P-Age: Pexels Dataset for Robust Spatio-Temporal Apparent Age Classification (see 8.7)
- Synthetic data in human analysis (see 8.8)
- LEO: Generative latent image animator for human video synthesis (see 8.9)
- LIA: Latent image animator (see 8.10)
- Local Distributional Smoothing for Noise-invariant Fingerprint Restoration (see 8.11)
- DiffTV: Identity-Preserved Thermal-to-Visible Face Translation via Feature Alignment and Dual-Stage Conditions (see 8.12)
- GHNeRF: Learning Generalizable Human Features with Efficient Neural Radiance Fields (see 8.13)
- HFNeRF: Learning Human Biomechanical Features with Neural Radiance Fields (see 8.14)
- Bipartite Graph Diffusion Model for Human Interaction Generation (see 8.15)
- Dimitra: Audio-driven Diffusion model for Expressive Talking Head Generation (see 8.16)
Action Recognition
Participants: François Brémond, Antitza Dantcheva, Michal Balazia, Monique Thonnat, Mohammed Guermal, Tanay Agrawal, Abid Ali, Di Yang, Aglind Reka, Valeriya Strizhkova.
The new results for action recognition are:
- Learning Effective Video Representations for Action Recognition (see 8.17)
- CM3T: Framework for Efficient Multimodal Learning for Inhomogeneous Interaction Datasets (see 8.18)
- Scaling Action Detection: AdaTAD++ with Transformer-Enhanced Temporal-Spatial Adaptation (see 8.19)
- AM Flow: Adapters for Temporal Processing in Action Recognition (see 8.20)
- View-invariant Skeleton Action Representation Learning via Motion Retargeting (see 8.21)
- Human Activity Recognition in Videos (see 8.22)
- Introducing Gating and Context into Temporal Action Detection (see 8.23)
- MAURA: Video Representation Learning for Conversational Facial Expression Recognition Guided by Multiple View Reconstruction (see 8.24)
- MVP: Multimodal Emotion Recognition based on Video and Physiological Signals (see 8.25)
Semantic Activity Recognition
Participants: François Brémond, Monique Thonnat, Snehashis Majhi, Mohammed Guermal, Mahmoud Ali, Abid Ali, Alexandra Konig, Rachid Guerchouche, Michal Balazia, Utkarsh Tiwari, Yoann Torrado.
For this research axis, the contributions are:
- MultiMediate'24: Multimodal Behavior Analysis for Artificial Mediation (see 8.26)
- OE-CTST: Outlier-Embedded Cross Temporal Scale Transformer for Weakly-supervised Video Anomaly Detection (see 8.27)
- Guess Future Anomalies from Normalcy: Forecasting Abnormal Behavior in Real-World Videos (see 8.28)
- What Matters in Autonomous Driving Anomaly Detection: A Weakly Supervised Horizon (see 8.29)
- Classification of Rare Diseases on Facial Videos (see 8.30)
- Weakly-supervised Autism Severity Assessment in Long Videos (see 8.31)
- Video Analysis Using Deep Neural Networks: An Application for Autism (see 8.32)
- Quo Vadis, Video Understanding with Vision-Language Foundation Models? (see 8.33)
8.2 Identifying Surgical Instruments in Pedagogical Cataract Surgery Videos through an Optimized Aggregation Network
Participants: Sanya Sinha, Michal Balazia, Francois Bremond.
Instructional cataract surgery videos are crucial for ophthalmologists and trainees to observe surgical details repeatedly. In 35, we present a deep learning model for real-time identification of surgical instruments in these videos, using a custom dataset scraped from open-access sources. Inspired by the architecture of YOLOv9, the model employs a Programmable Gradient Information (PGI) mechanism and a novel Generally-Optimized Efficient Layer Aggregation Network (Go-ELAN) to address the information bottleneck problem, enhancing Minimum Average Precision (mAP) at higher Non-Maximum Suppression Intersection over Union (NMS IoU) scores.
Go-ELAN YOLOv9 Architecture (see Figure 4) contains an auxiliary block which works on the Programmable Gradient Information (PGI) concept by creating an auxiliary reverse branch for enabling reliable gradient calculation by avoiding potential semantic loss. The GELAN block in the backbone feature extractor is replaced by the Go-ELAN block proposed in this paper. The Spatial Pyramid Pooling block SPPELAN removes the fixed size limitation of the backbone. The ADown block downsamples the generated feature maps to target sizes. The CBLinear blocks extract higher level features from the images, and the CBFuse block fuses these extracted features. The Neck combines the acquired features and the Head predicts the final bounding bound outputs with their respective probabilities.
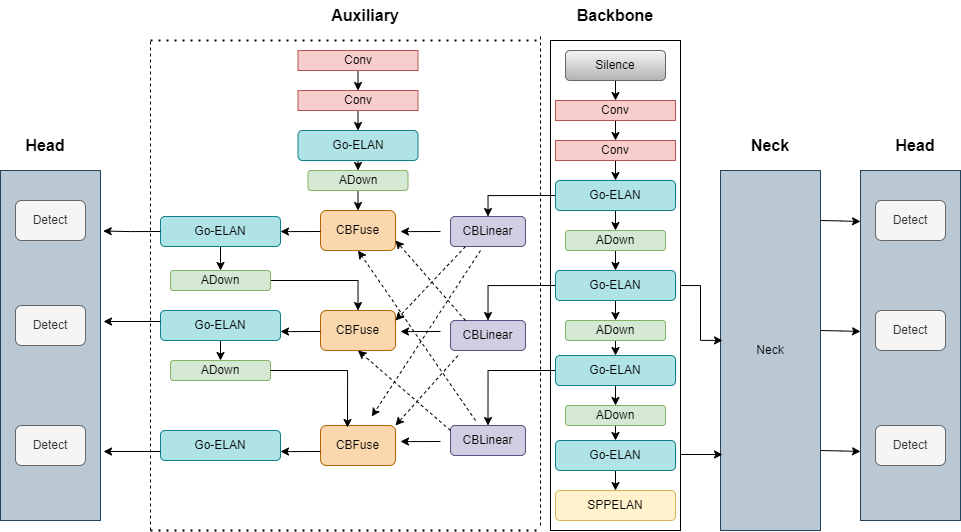
Our Go-ELAN YOLOv9 model, evaluated against YOLO v5, v7, v8, v9 vanilla, Laptool and DETR, achieves a superior mAP of 73.74 at IoU 0.5 on a dataset of 615 images with 10 instrument classes, demonstrating the effectiveness of the proposed model. To illustrate the visual and qualitative superiority of our model, we have compared 12 ground-truth images with their respective model predictions in Figure 5.
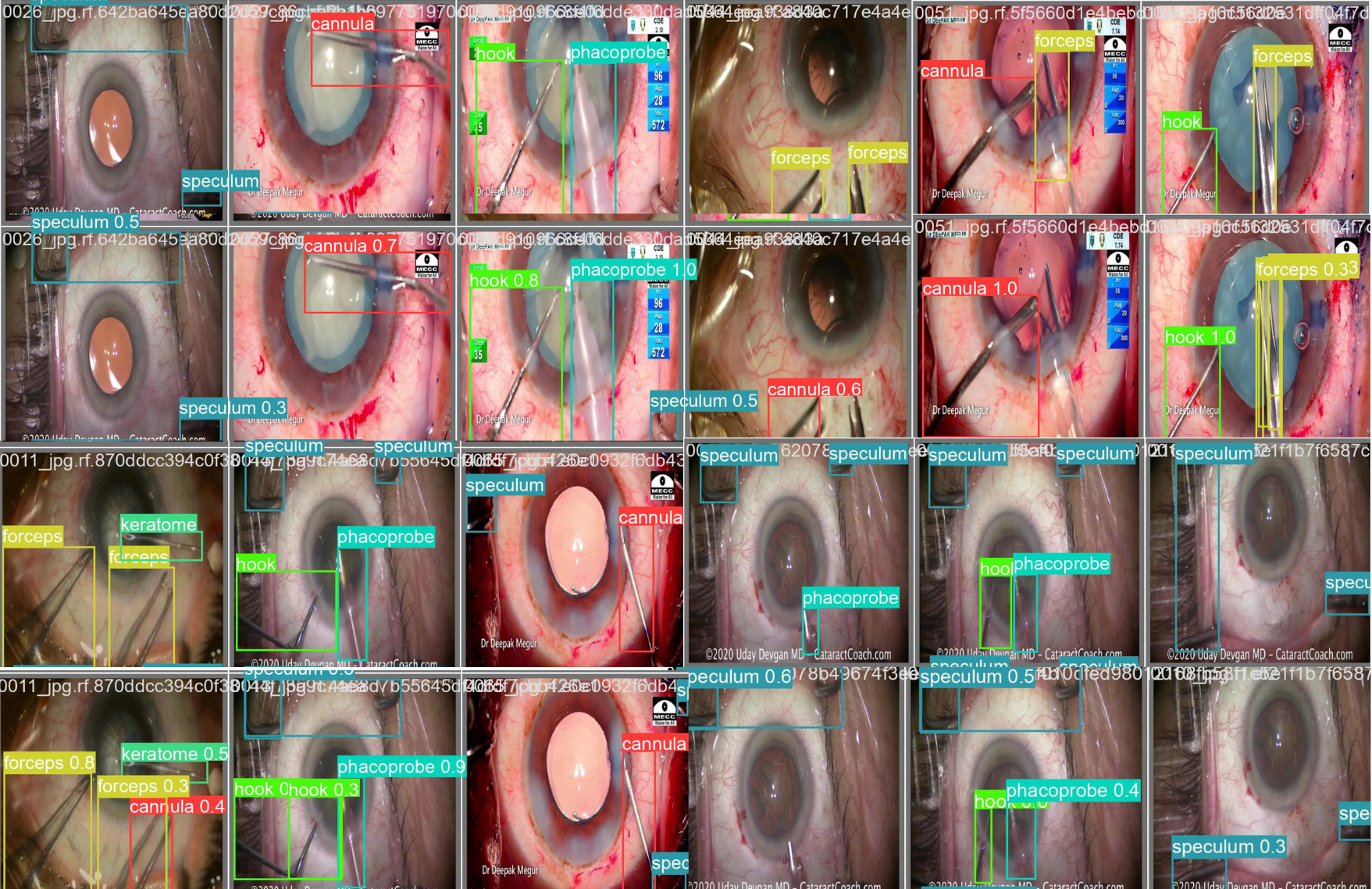
8.3 Temporally Propagated Masks as an Association Cue for Multi-Object Tracking
Participants: Tomasz Stanczyk, Francois Bremond.
The second contribution involved the development of McByte, a novel tracking-by-detection algorithm that introduces temporally propagated segmentation masks as a robust association cue. Traditional MOT methods often rely solely on bounding boxes, which can struggle under conditions such as heavy occlusion or abrupt motion. McByte addresses these challenges by combining bounding box information with the additional guidance provided by temporally propagated masks, improving object association accuracy and generalizability.
McByte eliminates the need for per-sequence tuning, a common limitation in many existing methods, making it more practical and adaptable across diverse datasets. Evaluations on DanceTrack, MOT17, SoccerNet, and KITTI-tracking demonstrated its consistent superiority over state-of-the-art approaches. The algorithm showed significant improvements in critical tracking metrics, such as HOTA and IDF1, while effectively handling complex scenarios like occlusion and tracking objects across extended sequences. These results highlight McByte's potential as a robust solution for diverse applications, including surveillance, autonomous driving, and sports analytics. Visual example of our designed tracker utilizing the temporally propagated mask as an association cue improving over the baseline algorithm are presented in Figure 6.
![]() |
![]() |
Frame 319 (baseline) | Frame 401 (baseline) |
![]() |
![]() |
Frame 319 (McByte) | Frame 401 (McByte) |
8.4 Re-Evaluating Re-ID in Multi-Object Tracking
Participants: Tomasz Stanczyk, Francois Bremond.
Re-identification (Re-ID) algorithms are widely used in tracking-by-detection pipelines for multi-object tracking (MOT) to maintain consistent object identities across frames. A detailed study was conducted to evaluate the effectiveness of Re-ID when integrated with BoT-SORT, focusing on its impact across diverse datasets and conditions. The analysis revealed that, while Re-ID can provide performance gains in controlled scenarios, its overall impact is inconsistent and highly dependent on factors such as dataset characteristics, detection quality, and the extent of fine-tuning.
In several cases, particularly with datasets featuring low-quality detections or a lack of parameter optimization, Re-ID integration negatively affected performance. This study highlighted that the benefits of Re-ID are not universally applicable and emphasized the importance of tailoring Re-ID strategies to specific datasets. These findings serve as a caution for over-reliance on Re-ID and encourage more rigorous evaluation when incorporating it into MOT systems.
8.5 Anti-forgetting adaptation for unsupervised person re-identification
Participants: Francois Bremond.
Regular unsupervised domain adaptive person re-identification (ReID) focuses on adapting a model from a source domain to a fixed target domain. However, an adapted ReID model can hardly retain previously-acquired knowledge and generalize to unseen data. In this work 16, we propose a Dual-level Joint Adaptation and Anti-forgetting (DJAA) framework, which incrementally adapts a model to new domains without forgetting source domain and each adapted target domain. We explore the possibility of using prototype and instance-level consistency to mitigate the forgetting during the adaptation. Specifically, we store a small number of representative image samples and corresponding cluster prototypes in a memory buffer, which is updated at each adaptation step. With the buffered images and prototypes, we regularize the image-to-image similarity and image-to-prototype similarity to rehearse old knowledge. After the multi-step adaptation, the model is tested on all seen domains and several unseen domains to validate the generalization ability of our method. Extensive experiments demonstrate that our proposed method significantly improves the anti-forgetting, generalization and backward-compatible ability of an unsupervised person ReID model.
8.6 Enhancing age estimation by regularizing with look-alike references and ensuring ordinal continuity
Participants: Olivier Huynh, Francois Bremond.
Age estimation is a classic problem in computer vision and can be used by Law Enforcement Agencies (LEAs) to detect minors in Child Sexual Abuse Material (CSAM). We present and aim to address two essential challenges in age estimation: the continuity of the aging process and the proper regularization of the latent space. The latter is particularly hindered by the imbalance of publicly available datasets, which is recognized as a challenge in the field.
To improve prediction results, we propose that the model leverage contextual information related to a cross-age sequence of a similar reference individual. This approach implicitly groups people of the same ethnicity, gender, etc., and provides insight into how a particular person ages. One associated challenge is to indicate to the system the labels of these references. We propose adding them as embeddings to our tokens while simultaneously training an image embedding estimator for the queried image, implemented with self-attention. We also explore the possibility of simulating this embedding across all ages and learning the target label with cross-entropy loss. Regarding regularization, we studied several ways to separate trajectories of distinct individuals using objectives such as ArcFace (CVPR'19) or triplet losses. Since our association criterion is based on look-alike features and to ensure high-quality references, we have manually annotated in FGNET and CAF datasets the association of each image from a trajectory to the images of a look-alike person. We also conducted experiments to automatically enrich our sequences with an unconstrained dataset (APPA-Real) by using a policy trained with the REINFORCE algorithm.
Simultaneously, related to the continuity of the aging process, we propose a novel constraint objective based on the reconstruction of the representation of the queried image from the reference sequence. We found that reconstruction could either collapse if poorly placed in the network or lead to learning difficulties. The optimal and most effective level is to work on the feature map given directly by the encoder, preserving a local feature scale (e.g., wrinkles).
Finally, to showcase the research work and as part of the European project HEROES, a demo tool was developed that integrates face detection and tracking. Experiments were conducted on adult content data, and the tool was used by LEAs on CSAM data with good performance.
8.7 P-Age: Pexels Dataset for Robust Spatio-Temporal Apparent Age Classification
Participants: Abid Ali.
Age estimation is a challenging task that has numerous applications. In this work 23, we propose a new direction for age classification that utilizes a video-based model to address challenges such as occlusions, low-resolution, and lighting conditions. To address these challenges, we propose AgeFormer which utilizes spatio-temporal information on the dynamics of the entire body dominating face-based methods for age classification. Our novel two-stream architecture uses TimeSformer and EfficientNet as backbones, to effectively capture both facial and body dynamics information for efficient and accurate age estimation in videos. Furthermore, to fill the gap in predicting age in real-world situations from videos, we construct a video dataset called Pexels Age (P-Age) for age classification. The proposed method achieves superior results compared to existing face-based age estimation methods and is evaluated in situations where the face is highly occluded, blurred, or masked. The method is also cross-tested on a variety of challenging video datasets such as Charades, Smarthome, and Thumos-14. The code and dataset is available on GitHub.
8.8 Synthetic data in human analysis
Participants: Francois Bremond, Antitza Dantcheva.
Deep neural networks have become prevalent in human analysis, boosting the performance of applications, such as biometric recognition, action recognition, as well as person re-identification. However, the performance of such networks scales with the available training data. In human analysis, the demand for large-scale datasets poses a severe challenge, as data collection is tedious, time-expensive, costly and must comply with data protection laws. Current research investigates the generation of synthetic data as an efficient and privacy-ensuring alternative to collecting real data in the field. This survey 17 introduces the basic definitions and methodologies, essential when generating and employing synthetic data for human analysis. We summarize current state-of-the-art methods and the main benefits of using synthetic data. We also provide an overview of publicly available synthetic datasets and generation models. Finally, we discuss limitations, as well as open research problems in this field. This survey is intended for researchers and practitioners in the field of human analysis.
8.9 LEO: Generative latent image animator for human video synthesis
Participants: Yaohui Wang, Antitza Dantcheva.
Spatio-temporal coherency is a major challenge in synthesizing high-quality videos, particularly in synthesizing human videos that contain rich global and local deformations. To resolve this challenge, previous approaches have resorted to different features in the generation process aimed at representing appearance and motion. However, in the absence of strict mechanisms to guarantee such disentanglement, a separation of motion from appearance has remained challenging, resulting in spatial distortions and temporal jittering that break the spatio-temporal coherency. Motivated by this, we here propose LEO 18, a novel framework for human video synthesis, placing emphasis on spatio-temporal coherency. Our key idea is to represent motion as a sequence of flow maps in the generation process, which inherently isolate motion from appearance. We implement this idea via a flow-based image animator and a Latent Motion Diffusion Model (LMDM). The former bridges a space of motion codes with the space of flow maps, and synthesizes video frames in a warp-and-inpaint manner. LMDM learns to capture motion prior in the training data by synthesizing sequences of motion codes. Extensive quantitative and qualitative analysis suggests that LEO significantly improves coherent synthesis of human videos over previous methods on the datasets TaichiHD, FaceForensics and CelebV-HQ. In addition, the effective disentanglement of appearance and motion in LEO allows for two additional tasks, namely infinite-length human video synthesis, as well as content-preserving video editing.
8.10 LIA: Latent image animator
Participants: Yaohui Wang, Francois Bremond, Antitza Dantcheva.
Previous animation techniques mainly focus on leveraging explicit structure representations (e.g., meshes or keypoints) for transferring motion from driving videos to source images. However, such methods are challenged with large appearance variations between source and driving data, as well as require complex additional modules to respectively model appearance and motion. Towards addressing these issues, we introduce the Latent Image Animator (LIA) 19, streamlined to animate high-resolution images. LIA is designed as a simple autoencoder that does not rely on explicit representations. Motion transfer in the pixel space is modeled as linear navigation of motion codes in the latent space. Specifically such navigation is represented as an orthogonal motion dictionary learned in a self-supervised manner based on proposed Linear Motion Decomposition (LMD). Extensive experimental results demonstrate that LIA outperforms state-of-the-art on VoxCeleb, TaichiHD, and TED-talk datasets with respect to video quality and spatio-temporal consistency. In addition, LIA is well equipped for zero-shot high-resolution image animation. Code, models, and demo video are available on GitHub.
8.11 Local Distributional Smoothing for Noise-invariant Fingerprint Restoration
Participants: Antitza Dantcheva.
Existing fingerprint restoration models fail to generalize on severely noisy fingerprint regions. To achieve noise-invariant fingerprint restoration, this work 31 proposes to regularize the fingerprint restoration model by enforcing local distributional smoothing by generating similar output for clean and perturbed fingerprints. Notably, the perturbations are learnt by virtual adversarial training so as to generate the most difficult noise patterns for the fingerprint restoration model. Improved generalization on noisy fingerprints is obtained by the proposed method on two publicly available databases of noisy fingerprints.
8.12 DiffTV: Identity-Preserved Thermal-to-Visible Face Translation via Feature Alignment and Dual-Stage Conditions
Participants: Antitza Dantcheva.
The thermal-to-visible (T2V) face translation task is essential for enabling face verification in low-light or dark conditions by converting thermal infrared faces into their visible counterparts. However, this task faces two primary challenges. First, the inherent differences between the modalities hinder the effective use of thermal information to guide RGB face reconstruction. Second, translated RGB faces often lack the identity details of the corresponding visible faces, such as skin color. To tackle these challenges, we introduce DiffTV 39, the first Latent Diffusion Model (LDM) specifically designed for T2V facial image translation with a focus on preserving identity. Our approach proposes a novel heterogeneous feature alignment strategy that bridges the modal gap and extracts both coarse- and fine-grained identity features consistent with visible images. Furthermore, a dual-stage condition injection strategy introduces control information to guide identity-preserved translation. Experimental results demonstrate the superior performance of DiffTV, particularly in scenarios where maintaining identity integrity is critical.
8.13 GHNeRF: Learning Generalizable Human Features with Efficient Neural Radiance Fields
Participants: Di Yang, Antitza Dantcheva.
Recent advances in Neural Radiance Fields (NeRF) have demonstrated promising results in 3D scene representations, including 3D human representations. However, these representations often lack crucial information on the underlying human pose and structure, which is crucial for AR/VR applications and games. In this work 28, we introduce a novel approach, termed GHNeRF, designed to address these limitations by learning 2D/3D joint locations of human subjects with NeRF representation. GHNeRF uses a pre-trained 2D encoder streamlined to extract essential human features from 2D images, which are then incorporated into the NeRF framework in order to encode human biomechanical features. This allows our network to simultaneously learn biomechanical features, such as joint locations, along with human geometry and texture. To assess the effectiveness of our method, we conduct a comprehensive comparison with state-of-the-art human NeRF techniques and joint estimation algorithms. Our results show that GHNeRF can achieve state-of-the-art results in near real-time.
8.14 HFNeRF: Learning Human Biomechanical Features with Neural Radiance Fields
Participants: Di Yang, Antitza Dantcheva.
In recent advancements in novel view synthesis, generalizable Neural Radiance Fields (NeRF) based methods applied to human subjects have shown remarkable results in generating novel views from few images. However, this generalization ability cannot capture the underlying structural features of the skeleton shared across all instances. Building upon this, we introduce HFNeRF 29: a novel generalizable human feature NeRF aimed at generating human biomechanical features using a pre-trained image encoder. While previous human NeRF methods have shown promising results in the generation of photorealistic virtual avatars, such methods lack underlying human structure or biomechanical features such as skeleton or joint information that are crucial for downstream applications including Augmented Reality (AR)/Virtual Reality (VR). HFNeRF leverages 2D pre-trained foundation models toward learning human features in 3D using neural rendering, and then volume rendering towards generating 2D feature maps. We evaluate HFNeRF in the skeleton estimation task by predicting heatmaps as features. The proposed method is fully differentiable, allowing to successfully learn color, geometry, and human skeleton in a simultaneous manner. This paper presents preliminary results of HFNeRF, illustrating its potential in generating realistic virtual avatars with biomechanical features using NeRF.
8.15 Bipartite Graph Diffusion Model for Human Interaction Generation
Participants: Baptiste Chopin.
The generation of natural human motion interactions is a hot topic in computer vision and computer animation. It is a challenging task due to the diversity of possible human motion interactions. Diffusion models, which have already shown remarkable generative capabilities in other domains, are a good candidate for this task. In this work 27, we introduce a novel bipartite graph diffusion method (BiGraphDiff) to generate human motion interactions between two persons. Specifically, bipartite node sets are constructed to model the inherent geometric constraints between skeleton nodes during interactions. The interaction graph diffusion model is transformer-based, combining some state-of-the-art motion methods. We show that the proposed method achieves new state-of-the-art results on leading benchmarks for the human interaction generation task.
8.16 Dimitra: Audio-driven Diffusion model for Expressive Talking Head Generation
Participants: Baptiste Chopin, Antitza Dantcheva.
We propose Dimitra, a novel framework for audio-driven talking head generation, streamlined to learn lip motion, facial expression, as well as head pose motion. Specifically, we train a conditional Motion Diffusion Transformer (cMDT) by modeling facial motion sequences with 3D representation. We condition the cMDT with only two input signals, an audio- sequence, as well as a reference facial image. By extracting additional features directly from audio, Dimitra is able to increase quality and realism of generated videos. In particular, phoneme sequences contribute to the realism of lip motion, whereas text transcript to facial expression and head pose realism. Quantitative and qualitative experiments on two widely employed datasets, VoxCeleb2 and HDTF, showcase that Dimitra is able to outperform existing approaches for generating realistic talking heads imparting lip motion, facial expression, and head pose. This work was submitted to FG 2025. We extend Dimitra by minimizing the number of features extracted from the audio. Instead of text, phoneme and deep features from wav2vec, we only use deep features from Wavlm. The high-quality features from Wavlm are able to better encode the audio resulting in features that contains information about the phonemes and the semantics. This leads to better results quantitatively and qualitatively. Additionally we modify our training scheme by training on the HDTF dataset and finetuning on the LRW dataset. The LRW dataset contains a large number of samples representing 500 common english word, allowing Dimitra to learn better representation for the lips motion of these words increasing the overall quality of the generated videos. Finally, in an effort to propose an alternative to the widely used, but inherently flawed, syncnet based metric for lips synchronization evaluation, we propose a new way to evaluate lips synchronization. This new evaluation consists of several metrics, each evaluating an aspect of lips synchronization (e.g., the lips do not move during silence, the correct mouth shape are being generated). To extend this work beyond talking head generation, we are looking into co-speech gesture generation using 3D meshes. With this we can generate both face and full body motion related to speech resulting in more realistic videos.
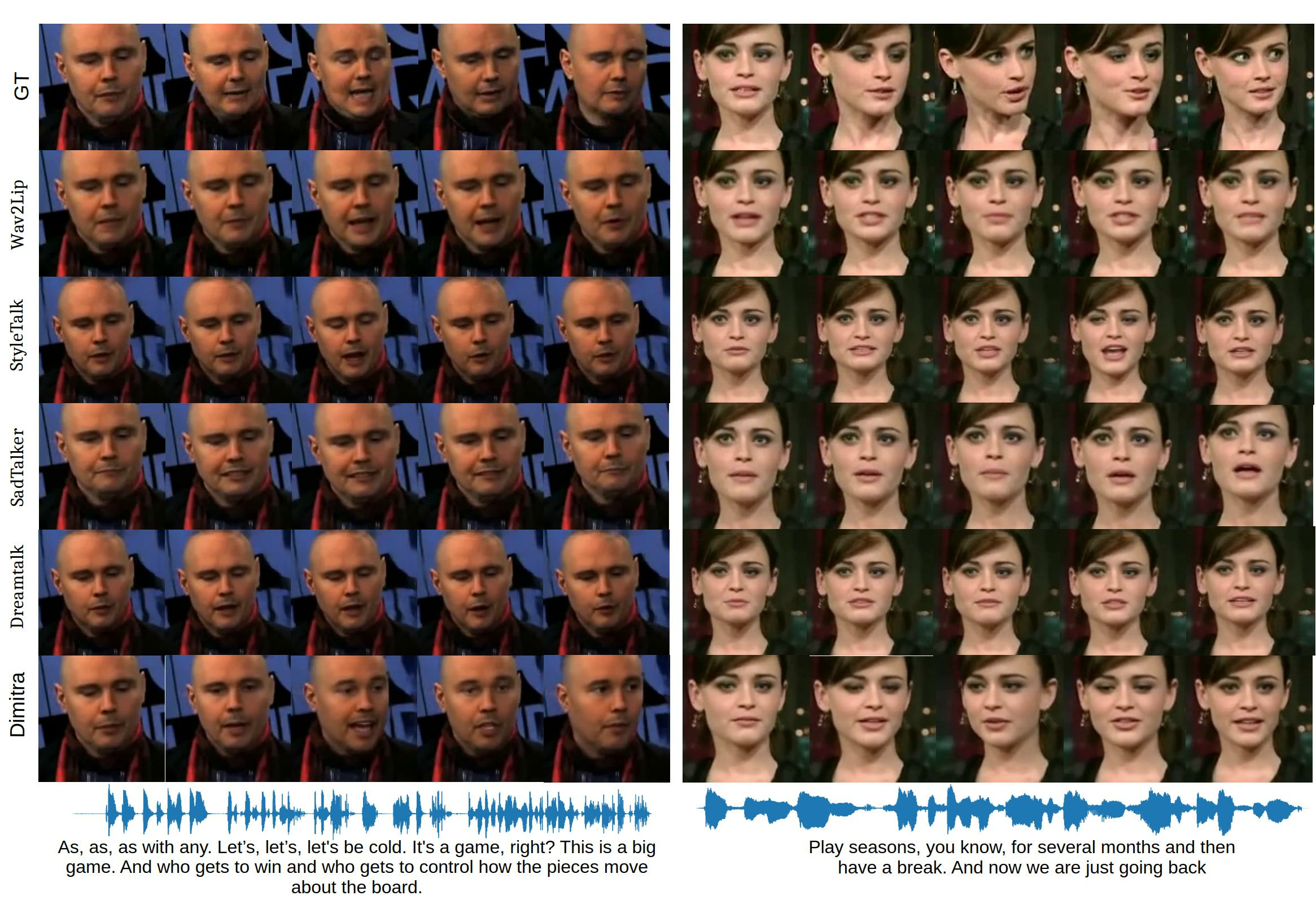
8.17 Learning Effective Video Representations for Action Recognition
Participants: Di Yang, Francois Bremond.
Human action recognition is an active research field with significant contributions to applications such as home-care monitoring, human-computer interaction, and game control. However, recognizing human activities in real-world videos remains challenging, especially when learning effective video representations with a high expressive power to represent human spatio-temporal motion, view-invariant actions, complex composable actions, etc. To address this challenge, this thesis 42 makes three contributions toward learning effective video representations that can be applied and evaluated in real-world human action classification and segmentation tasks by transfer learning.
The first contribution is to improve the generalizability of human skeleton motion representation models. We propose a unified framework for real-world skeleton human action recognition. The framework includes a novel skeleton model that not only effectively learns spatio-temporal features on human skeleton sequences but also generalizes across datasets.
The second contribution extends the proposed framework by introducing two novel joint skeleton action generation and representation learning frameworks for different downstream tasks. The first is a self-supervised framework for learning from synthesized composable motions for skeleton-based action segmentation. The second is a View-invariant model for self-supervised skeleton action representation learning that can deal with large variations across subjects and camera viewpoints.
The third contribution targets general RGB-based video action recognition. Specifically, a time-parameterized contrastive learning strategy is proposed. It captures time-aware motions to improve the performance of action classification in fine-grained and human-oriented tasks. Experimental results on benchmark datasets demonstrate that the proposed approaches achieve state-of-the-art performance in action classification and segmentation tasks. The proposed frameworks improve the accuracy and interpretability of human activity recognition and provide insights into the underlying structure and dynamics of human actions in videos.
Overall, this thesis contributes to the field of video understanding by proposing novel methods for skeleton-based action representation learning, and general RGB video representation learning. Such representations benefit both action classification and segmentation tasks.
8.18 CM3T: Framework for Efficient Multimodal Learning for Inhomogeneous Interaction Datasets
Participants: Tanay Agrawal, Mohammed Guermal, Michal Balazia, Francois Bremond.
(Accepted for WACV 2025)
Challenges in cross-learning involve inhomogeneous or even inadequate amount of training data and lack of resources for retraining large pretrained models. Inspired by transfer learning techniques in NLP, adapters and prefix tuning, this work presents a new model-agnostic plugin architecture for cross-learning, called CM3T, that adapts transformer-based models to new or missing information. We introduce two adapter blocks 21: multi-head vision adapters for transfer learning and cross-attention adapters for multimodal learning. Training becomes substantially efficient as the backbone and other plugins do not need to be finetuned along with these additions. Comparative and ablation studies on three datasets Epic-Kitchens-100, MPIIGroupInteraction and UDIVA v0.5 show efficacy of this framework on different recording settings and tasks. With only 12.8% trainable parameters compared to the backbone to process video input and only 22.3% trainable parameters for two additional modalities, we achieve comparable and even better results than the state-of-the-art. CM3T has no specific requirements for training or pretraining and is a step towards bridging the gap between a general model and specific practical applications of video classification. Figure 8 illustrates a representation of the main problem CM3T aims to solve.
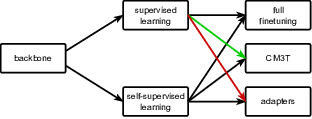
8.19 Scaling Action Detection: AdaTAD++ with Transformer-Enhanced Temporal-Spatial Adaptation
Participants: Tanay Agrawal, Abid Ali, Francois Bremond.
Temporal Action Detection (TAD) is essential for understanding long-form videos by detecting and segmenting actions within untrimmed sequences. Although recent innovations, such as Temporal Informative Adapters (TIA), have advanced the scalability of TAD, memory constraints persist, specifically during training, hindering the handling of larger video inputs. This paper presents an enhanced TIA framework that introduces independently trainable temporal and spatial adapters. Our two-step training protocol initially trains low spatial and high temporal resolution, followed by high spatial and low temporal resolution, allowing higher input resolutions during inference. We further replace the 1D convolutional layers with a transformer encoder for temporal feature extraction to capture long-range dependencies more effectively. Extensive experiments on ActivityNet-1.3, THUMOS14, and EPIC-Kitchens 100 datasets demonstrate our approach's ability to balance performance gains with computational feasibility, achieving state-of-the-art results in both detection accuracy and scalability, see Figure 9. This work provides a pathway for future TAD models to scale efficiently, leveraging high-resolution data while minimizing memory overhead.
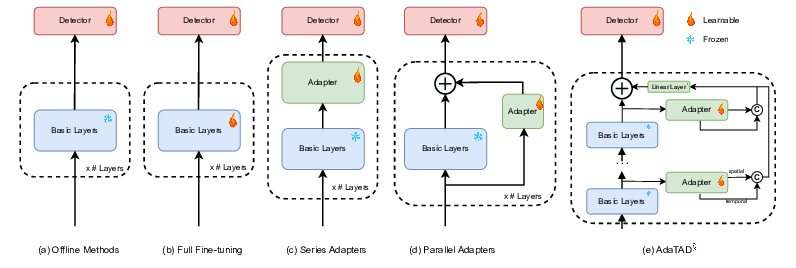
8.20 AM Flow: Adapters for Temporal Processing in Action Recognition
Participants: Tanay Agrawal, Abid Ali, Antitza Dantcheva, Francois Bremond.
Deep learning models, in particular image models, have recently gained generalizability and robustness. In this work, we propose to exploit such advances in the realm of video classification. Video foundation models suffer from the requirement of extensive pretraining and a large training time. Towards mitigating such limitations, we propose "Attention Map (AM) Flow" for image models, a method for identifying pixels relevant to motion in each input video frame. In this context, we propose two methods to compute AM flow, depending on camera motion. AM flow allows the separation of spatial and temporal processing, while providing improved results over combined spatio-temporal processing (as in video models). Adapters, one of the popular techniques in parameter efficient transfer learning, facilitate the incorporation of AM flow into pretrained image models, mitigating the need for full-finetuning. We extend adapters to "temporal processing adapters" by incorporating a temporal processing unit into the adapters, see Figure 10. Our work achieves faster convergence, therefore reducing the number of epochs needed for training. Moreover, we endow an image model with the ability to achieve state-of-the-art results on popular action recognition datasets. This reduces training time and simplifies pretraining. We present experiments on Kinetics-400, Something-Something v2, and Toyota Smarthome datasets, showcasing state-of-the-art or comparable results.
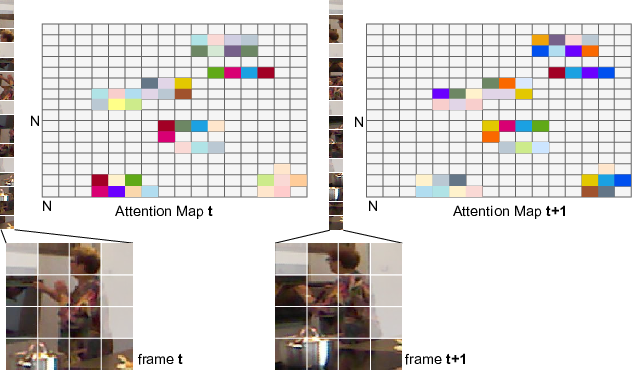
8.21 View-invariant Skeleton Action Representation Learning via Motion Retargeting
Participants: Di Yang, Antitza Dantcheva, Francois Bremond.
Current self-supervised approaches for skeleton action representation learning often focus on constrained scenarios, where videos and skeleton data are recorded in laboratory settings. When dealing with estimated skeleton data in real-world videos, such methods perform poorly due to the large variations across subjects and camera viewpoints. To address this issue, we introduce ViA 20, a novel View-Invariant Autoencoder for self-supervised skeleton action representation learning. ViA leverages motion retargeting between different human performers as a pretext task, in order to disentangle the latent action-specific `Motion' features on top of the visual representation of a 2D or 3D skeleton sequence. Such `Motion' features are invariant to skeleton geometry and camera view and allow ViA to facilitate both, cross-subject and cross-view action classification tasks. We conduct a study focusing on transfer-learning for skeleton-based action recognition with self-supervised pre-training on real-world data (e.g., Posetics). Our results showcase that skeleton representations learned from ViA are generic enough to improve upon state-of-the-art action classification accuracy, not only on 3D laboratory datasets such as NTU-RGB+D 60 and NTU-RGB+D 120, but also on real-world datasets where only 2D data are accurately estimated, e.g., Toyota Smarthome, UAV-Human and Penn Action.
8.22 Human Activity Recognition in Videos
Participants: Mohammed Guermal, Francois Bremond.
Understanding actions in videos is a pivotal aspect of computer vision with profound implications across various domains. As our reliance on visual data continues to surge, the ability to comprehend and interpret human actions in videos is necessary for advancing technologies in surveillance, healthcare, autonomous systems, and human-computer interaction. Moreover, there is an unprecedented economic and societal demand for robots that can assist humans in their industrial work and daily life activities. Hence, understanding human behavior and their activities would be very helpful and would facilitate the development of such robots. The accurate interpretation of actions in videos serves as a cornerstone for the development of intelligent systems that can navigate and respond effectively to the complexities of the real world. Computer Vision has made huge progress with the rise of deep learning methods such as convolutional neural networks (CNNs) and more lately transformers. However, when it comes to video processing, it is still limited compared to static images. In this thesis 41, we focus on action understanding and we divide it into two main parts: action recognition and action detection. Mainly, action understanding algorithms face the following challenges: 1) temporal and spatial analysis, 2) fine grained actions, and 3) temporal modeling.
In this thesis, we introduce in more detail the different aspects and key challenges of action understanding. After that, we introduce our contributions and solutions on how to deal with these challenges. We focus mainly on recognizing fine-grained actions using spatio-temporal object semantics and their dependencies in space and time. We tackle also action detection in real-time and anticipation by introducing a new joint model of action anticipation and online action detection for real-life scenarios applications of action detection. Finally, we discuss some ongoing and future works. All our contributions were extensively evaluated on challenging benchmarks and outperformed previous works.
8.23 Introducing Gating and Context into Temporal Action Detection
Participants: Aglind Reka, Diana Borza, Michal Balazia, Francois Bremond.
Temporal Action Detection (TAD), the task of localizing and classifying actions in untrimmed video, remains challenging due to action overlaps and variable action durations. Recent findings suggest that TAD performance is dependent on the structural design of transformers rather than on the self-attention mechanism. Building on this insight, we propose a refined feature extraction process through lightweight, yet effective operations. First, we employ a local branch that employs parallel convolutions with varying window sizes to capture both fine-grained and coarse-grained temporal features. This branch incorporates a gating mechanism to select the most relevant features. Second, we introduce a context branch that uses boundary frames as key-value pairs to analyze their relationship with the central frame through cross-attention. The proposed method 34 captures temporal dependencies and improves contextual understanding.
This work introduces a one-stage TAD model, built on top of the TriDet architecture. As its predecessor, the model comprises three modules: a video feature extractor, a feature pyramid extractor that progressively down-samples the video features to effectively handle actions of different lengths, and a boundary-oriented Trident-head for action localization and classification. Figure 11 shows the model consisting of a video feature extractor, a feature pyramid extractor, and a boundary-oriented head for action localization and classification. Figure 11 also depicts the structure of the proposed Temporal Attention Gating layer.
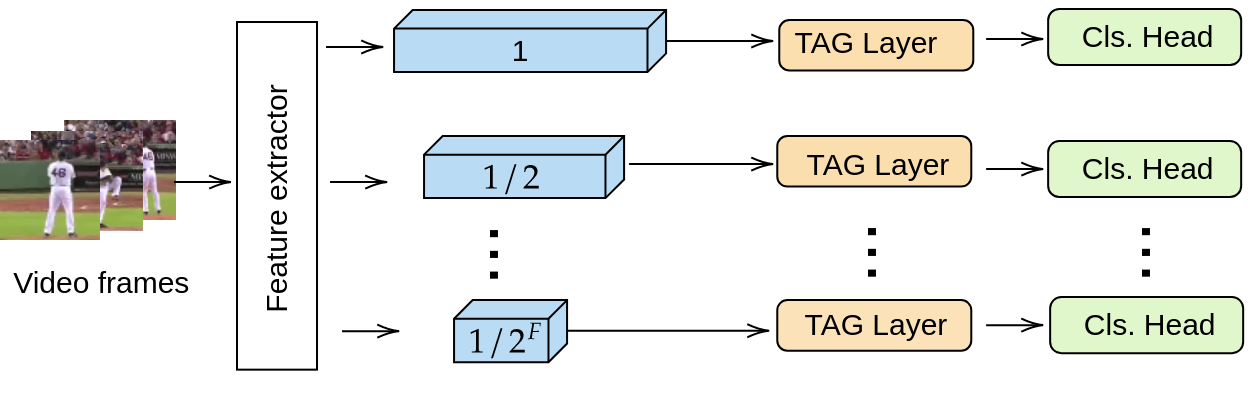
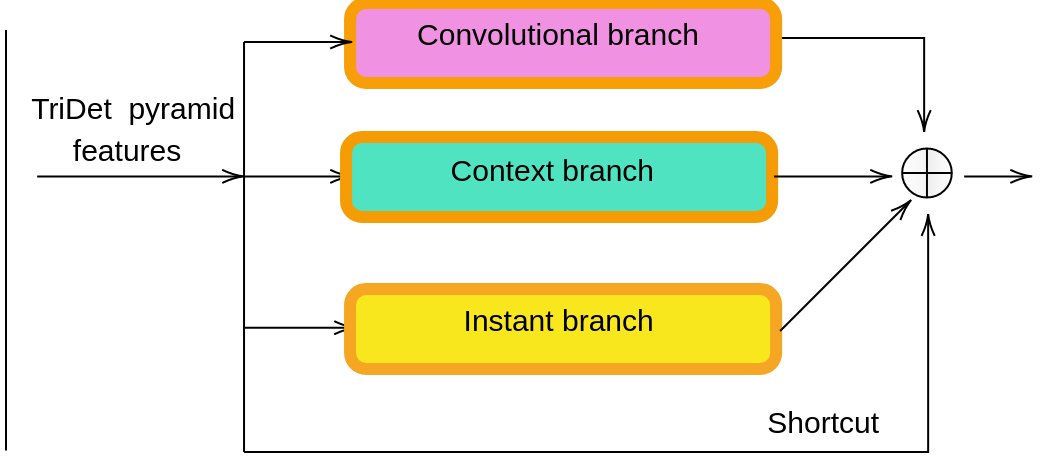
Conducted on two TAD benchmarks, EPIC-KITCHEN 100 and THUMOS14, our experiments confirm the efficacy of our proposed model. The results demonstrate an improvement in detection performance compared to existing methods, underlining the benefits of the proposed architectural design.
8.24 MAURA: Video Representation Learning for Conversational Facial Expression Recognition Guided by Multiple View Reconstruction
Participants: Valeriya Strizhkova, Antitza Dantcheva, Francois Bremond.
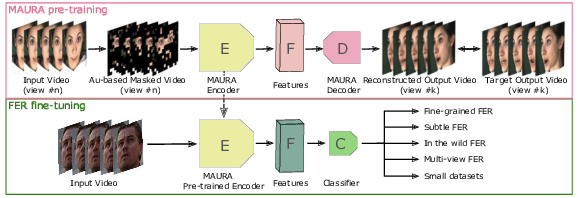
The paper 36 introduces MAURA (Masking Action Units and Reconstructing Multiple Angles), a novel self-supervised pre-training method for conversational facial expression recognition (cFER), see Figure 12. MAURA addresses challenges such as fine-grained emotional expressions, low-intensity emotions, extreme face angles, and limited datasets. Unlike existing methods, MAURA incorporates an innovative masking and reconstruction strategy that focuses on active facial muscle movements (Action Units) and reconstructs synchronized multi-view videos to capture dependencies between muscle movements.
The MAURA framework uses an asymmetric encoder-decoder architecture, where the encoder processes videos with masked Action Units, and the decoder reconstructs videos from different angles. This approach enables the model to learn robust, transferable video representations, capturing subtle emotional expressions even in challenging conditions.
The method demonstrates superior performance over state-of-the-art techniques in various tasks, including low-intensity, fine-grained, multi-view, and in-the-wild facial expression recognition. Experiments on datasets such as MEAD, DFEW, CMU-MOSEI, and MFA highlight MAURA’s capability to improve emotion classification accuracy, particularly in cases with limited training data and complex visual conditions.
MAURA’s contributions include a novel masking strategy, multi-view representation learning, and significant improvements in emotion recognition tasks. The study concludes with future directions, including integrating additional modalities (e.g., audio and language) and exploring other applications like lip synchronization and DeepFake detection.
8.25 MVP: Multimodal Emotion Recognition based on Video and Physiological Signals
Participants: Valeriya Strizhkova, Michal Balazia, Antitza Dantcheva, Francois Bremond.
The paper 37 introduces the Multimodal for Video and Physio (MVP) architecture, designed to advance emotion recognition by fusing video and physiological signals. Unlike traditional methods that rely on classic machine learning, MVP leverages deep learning and transformer-based architectures to handle long input sequences (1-2 minutes). The model integrates video features (Action Units or deep learning-extracted representations) with physiological signals (e.g., ECG and EDA), employing cross-attention to learn dependencies across modalities effectively.
Key contributions include (1) evaluating video and physiological backbones for long sequence inputs, (2) proposing a transformer-based multimodal fusion method, and (3) demonstrating MVP's superior performance over state-of-the-art approaches in emotion recognition tasks on datasets like AMIGOS and DEAP. Experimental results show MVP's ability to better capture nuanced behavioral and physiological changes, offering robust emotion classification even with limited datasets.
The study highlights MVP's innovative mid-fusion strategy and emphasizes its capacity to improve human emotion understanding, paving the way for further research in multimodal affective computing. Future directions include refining physiological signal handling and developing domain-specific pretraining techniques.
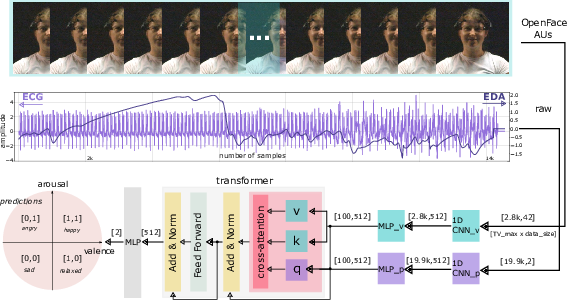
8.26 MultiMediate'24: Multimodal Behavior Analysis for Artificial Mediation
Participants: Michal Balazia, Francois Bremond.
Automatic analysis of human behavior is a fundamental prerequisite for the creation of machines that can effectively interact with and support humans in social interactions. In the MultiMediate Grand Challenge, we address key human social behavior analysis tasks in a controlled environment: eye contact detection, next speaker prediction, backchannel detection, agreement estimation, body behavior recognition and engagement estimation.
The latter two, body behavior recognition and engagement estimation, have gained significant interest from the participants. For engagement estimation, we collected novel annotations on the NOvice eXpert Interaction database (NOXI, see Figure 14 left). For body behavior recognition, we annotated test recordings of the MPIIGroupInteraction corpus (MPIIGI, see Figure 14 right). We also present baseline results for both challenge tasks.
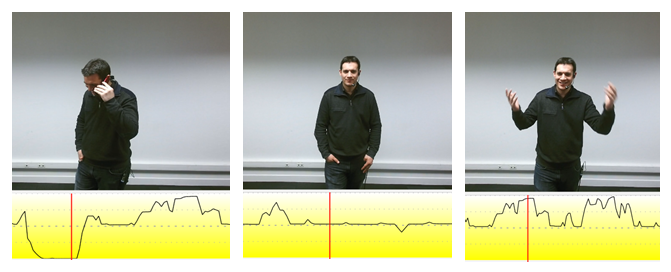
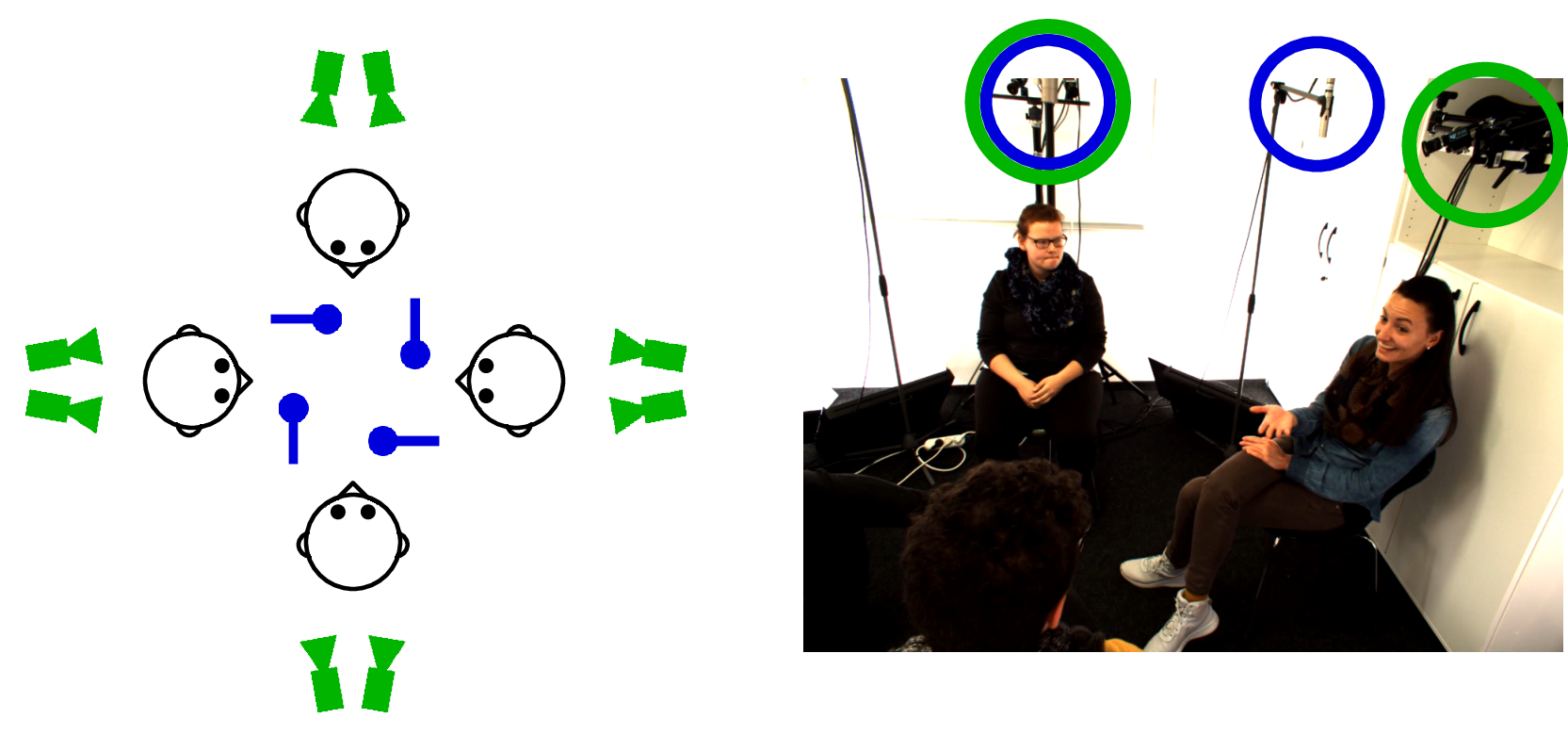
Estimating the momentary level of participant's engagement is an important prerequisite for assistive systems that support human interactions. Previous work has addressed this task in within-domain evaluation scenarios, i.e. training and testing on the same dataset. This is in contrast to real-life scenarios where domain shifts between training and testing data frequently occur. Our latest edition of MultiMediate, this year's MultiMediate'24 33, expands the single-domain nature of engagement estimation into multiple domains. MultiMediate'24 continues all previously defined tasks and creates another task: Multi-Domain Engagement Estimation.
As training data, we utilize the NOXI database of dyadic novice-expert interactions. In addition, to within-domain test data, we add two new test domains. First, we introduce recordings following the NOXI protocol but covering languages that are not present in the NOXI training data. Second, we collected novel engagement annotations on the MPIIGroupInteraction dataset which consists of group discussions between three to four people. In this way, MultiMediate'24 evaluates the ability of approaches to generalize across factors such as language and cultural background, group size, task, and screen-mediated versus face-to-face interaction.
The task includes the continuous, frame-wise prediction of the level of conversational engagement of each participant in the social interaction on a continuous scale from 0 (lowest) to 1 (highest). Challenge participants are encouraged to investigate multimodal as well as reciprocal behavior of all interlocutors. We use the Concordance Correlation Coefficient (CCC) to evaluate predictions on our test set annotations. We provide a multi-modal set of pre-computed features to participants. From the audio signal, we provide transcripts generated with the Whisper model. Additionally, we supply GeMAPS features along with wav2vec 2.0 embeddings. From the video, we provide OpenFace and OpenPose outputs to cover facial as well as bodily behavior.
8.27 OE-CTST: Outlier-Embedded Cross Temporal Scale Transformer for Weakly-supervised Video Anomaly Detection
Participants: Snehashis Majhi, Francois Bremond.
Video anomaly detection in real-world scenarios is challenging due to the complex temporal blending of long and short-length anomalies with normal ones. Further, it is more difficult to detect those due to : (i) Distinctive features characterizing the short and long anomalies with sharp and progressive temporal cues respectively; (ii) Lack of precise temporal information (i.e. weak-supervision) limits the temporal dynamics modeling of anomalies from normal events. In this work 32, we propose a novel 'temporal transformer' framework for weakly-supervised anomaly detection: OE-CTST. The proposed framework has two major components: (i) Outlier Embedder (OE) and (ii) Cross Temporal Scale Transformer (CTST). First, OE generates anomaly-aware temporal position encoding to allow the transformer to effectively model the temporal dynamics among the anomalies and normal events. Second, CTST encodes the cross-correlation between multi-temporal scale features to benefit short and long length anomalies by modeling the global temporal relations. The proposed OE-CTST is validated on three publicly available datasets i.e. UCF-Crime, XD-Violence, and IITB-Corridor, outperforming recently reported state-of-the-art approaches.
8.28 Guess Future Anomalies from Normalcy: Forecasting Abnormal Behavior in Real-World Videos
Participants: Snehashis Majhi, Mohammed Guermal, Antitza Dantcheva, Francois Bremond.
Forecasting Abnormal Human Behavior (AHB) aims to predict unusual behavior in advance by analyzing early patterns of normal human interactions. Unlike typical action prediction methods, this task focuses on observing only normal interactions to predict both, short and long term future abnormal behavior. Despite its affirmative impact on society, AHB prediction remains under-explored in current research. This is primarily due to the challenges involved in anticipating complex human behaviors and interactions with surrounding agents in real-world situations. Further, there exists an underlying uncertainty between the early normal patterns and the future abnormal behavior, thereby making the prediction harder. To address these challenges, we introduce a novel transformer model that improves early interaction modeling by accounting for uncertainties in both, observations and future outcomes. To the best of our knowledge, we are the first to explore the task. Therefore, we present a new comprehensive dataset referred to as “AHB-F", which features real-world scenarios with complex human interactions. The AHB-F has a deterministic evaluation protocol that ensures only normal frames to be observed for long- and short-term future prediction. We extensively evaluate and compare competitive action anticipation methods on our benchmark. Our results show that our method consistently outperforms existing action anticipation approaches, both in quantitative and qualitative evaluations.
Forecasting abnormal human behavior (AHB) incorporates two main challenges: (I) understanding complex AHB, and (II) overcoming uncertainty between current observations and future events. First, real-world scenarios are unpredictable and ever-changing, rendering it difficult to analyze complex human behavior and interactions to predict abnormal behavior. These situations differ significantly from everyday life, where interactions are simpler. In abnormal scenarios, there is a wide range of interactions, from subtle cues (such as shoplifting, which involves a few human-object interactions) to more intuitive ones (such as protests, where there occur dense human-to-human and human-to-object interactions). Abnormal behavior is often accompanied by normal activities and occurs rarely, which challenges prediction. Second, predicting future AHB based on normal interactions is naturally uncertain in both, short and long-term behavior. For instance, the same normal observation can result in multiple plausible continuations. Despite the importance of forecasting AHB, few methods exist that can handle these uncertainties and complexities in real-world, dynamic situations.
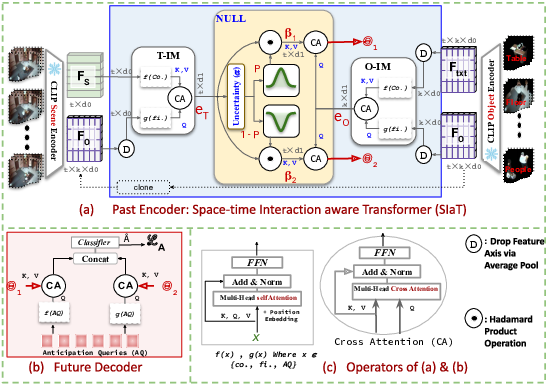
Motivated by this, we use an encoder-decoder framework shown in Figure 15 to process past interactions and predict future AHB. Our past encoder introduces a new transformer model, referred to as “Space-time Interaction aware Transformer (SIaT)", with two key components: (i) Interaction Modules (TIM/OIM) and (ii) Normalcy Uncertainty Latent Learner (NULL). These components help for capturing early human interaction patterns and handle the uncertainty between normal interactions to future abnormal behavior. Deviating from previous methods, our interaction modules separately encode scene-level temporal context (TIM) and object-level spatial interactions (OIM), providing a detailed understanding from broader scene dynamics to fine object interactions. We use panoptic object masks and raw RGB frames to represent objects and scenes level agents, thereby making both spatially coherent. This enables SIaT to effectively capture correlations between scene and object interactions. Next, the correlation encoded normal scene and object semantics are associated via NULL by considering the uncertainties associated with normal observations. The NULL module handles the uncertainty by distinguishing between relevant and non-relevant scene-object associations. Further, it adjusts the flow of information from the past encoder to the future decoder by learning latent features that is aligned with future predictions.
8.29 What Matters in Autonomous Driving Anomaly Detection: A Weakly Supervised Horizon
Participants: Utkarsh Tiwari, Snehashis Majhi, Michal Balazia, Francois Bremond.
Video anomaly detection (VAD) in autonomous driving scenarios is an important task, however, it involves several challenges due to the ego-centric views and moving camera. Due to this, it remains largely under-explored. While recent developments in weakly-supervised VAD methods have shown remarkable progress in detecting critical real-world anomalies in static camera scenarios, the development and validation of such methods are yet to be explored for moving camera VAD. This is mainly due to existing datasets like DoTA not following training pre-conditions of weakly-supervised learning. In this work 38, we aim to promote the development of weakly supervised methods for autonomous driving VAD. We reorganize the DoTA dataset and aim to validate recent powerful weakly-supervised VAD methods on moving camera scenarios. Further, we provide a detailed analysis of which modifications to state-of-the-art methods can significantly improve the detection performance. Towards this, we propose a “feature transformation block” and through experimentation, we show that our propositions can empower existing weakly-supervised VAD methods significantly in improving the VAD in autonomous driving.
While video anomaly detection (VAD) in static CCTV scenarios has been extensively studied in recent research, egocentric vehicle view anomaly detection (ego-VAD) remains largely unexplored. This is due to the complexity involved in ego-VAD as it poses several unique challenges. These include: (i) complex dynamic scenarios due to moving cameras, (ii) low camera field of view, (iii) little to no prior cues before anomaly occurrence. Recent static camera VAD approaches adopt a weakly-supervised binary classification paradigm where both normal and anomaly videos are used during training. In this setting, for a long untrimmed video sequence, only coarse video-level labels (i.e. normal and anomaly) are required for training instead of frame-level annotations. Another, critical aspect of weakly-supervised VAD lies in effective temporal modeling to discriminate anomalies from normal events. To promote this, previous classical methods adopt conventional temporal modeling networks like TCN, LSTM to discriminate short anomalies from normal events. In contrast, authors in Robust Temporal Feature Magnitude learning (RTFM) proposed a multi-scale temporal convolution network (MTN) for global temporal dependency modeling between normal and anomaly segments. Recently, URDMU and MGFN adopt transformer-based global-local and focus-glance blocks respectively to capture long and short-term temporal dependencies in normal and anomalous videos. Distinctively, OE-CTST proposes an Outlier-Embedded Cross Temporal Scale Transformer that first generates anomaly-aware temporal information for both long and short anomalies and hence allows the transformer to effectively model the global temporal relation among the normal and anomalies. Recent weakly-supervised VAD methods empowered by effective temporal modeling ability and strong optimization ability with limited supervision have gained popularity in static camera conditions, however, their adaptation to moving ego-camera settings is still unexplored.
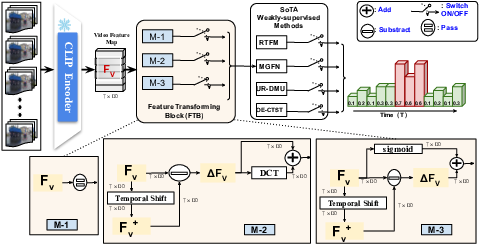
Motivated by this, in this work, we aim to provide an extensive exploration of recent popular weakly-supervised methods on ego-centric VAD tasks. We choose four state-of-the-art (SoTA) reproducible methods: RTFM(ICCV'21), MGFN(AAAI'23), UR-DMU(AAAI'23), and OE-CTST(WACV'24) for quantitative and qualitative analysis. Further, as weakly-supervised methods majorly rely on pre-computed input feature maps, we leverage recent popular vision-language model CLIP for backbone feature extraction. Next, we proceed to propose a feature transformation block (FTB) to enhance the temporal saliency which can enable better temporal modeling and optimization with feature magnitude supervision in SoTA methods, see Figure 16. Further, as existing ego-centric VAD datasets like DoTA do not have normal samples in the training split, the official DoTA dataset is not useful for weakly-supervised training (requires both normal and anomaly samples for training). Thus, we reorganized the training split of the DoTA dataset to fulfill the weakly-supervised training regime and kept the test split as in the official DoTA dataset for a fair comparison with previous unsupervised methods. We declare this reorganized DoTA dataset as WS-DoTA to promote weakly supervised research exploration on ego-centric VAD tasks. Through experimentation, we have shown that what matters in weakly supervised learning of anomalies in ego-centric autonomous driving videos. Further, we show how the proposed FTB enhances the SoTA methods' performance significantly on the WS-DoTA dataset.
8.30 Classification of Rare Diseases on Facial Videos
Participants: Yoann Torrado, Francois Bremond.
Facio-scapulo-humeral muscular dystrophy (FSHD) is a genetic muscle disorder in which the muscles of the body and the face are affected. The diagnosis of this disease needs to be done at a medical center through long medical examinations. To help clinicians speed up the process and avoid long travels for patients, we explore computer vision solutions that can be used in telemedicine services.
The dataset consists of a set of multiple facial exercises done by patients. These exercises try to create different facial movements to enhance the disease's visual symptoms. The facial features of the disease are hard to find even for human beings; this is why we explore state-of-the-art methods to extract those features, like I3D, DinoV2, and masked auto-encoders with VideoMAE and MARLIN.
We enhanced the performance of VideoMAE by incorporating adapters to facilitate fine-tuning on our small dataset after pretraining the model on a large facial video dataset, VoxCeleb. Compared to pretraining on Kinetics-400, VoxCeleb significantly improved results by helping the model learn a stronger representation of facial features. To further improve performance, we introduced an UpConv Feature Pooling module, which enhances the model's ability to capture movement dynamics. Additionally, we have integrated a multi-head transformer classifier to classify both the disease and its severity. These modules collectively enable the model to operate in an end-to-end manner, reducing the overall number of parameters while achieving more accurate predictions. This design not only leverages stronger facial representations, but also effectively captures temporal motion and disease-specific details, leading to improved outcomes on our dataset.
8.31 Weakly-supervised Autism Severity Assessment in Long Videos
Participants: Abid Ali, Mahmoud Ali, Francois Bremond.
Autism Spectrum Disorder (ASD) is a diverse collection of neurobiological conditions marked by challenges in social communication and reciprocal interactions, as well as repetitive and stereotypical behaviors. Atypical behavior patterns in a long, untrimmed video can serve as biomarkers for children with ASD. In this work 22, we propose a video-based weakly-supervised method that takes spatio-temporal features of long videos to learn typical and atypical behaviors for autism detection. On top of that, we propose a shallow TCN-MLP network, which is designed to further categorize the severity score. We evaluate our method on actual evaluation videos of children with autism collected and annotated (for severity score) by clinical professionals. Experimental results demonstrate the effectiveness of behavioral biomarkers that could help clinicians in autism spectrum analysis.
8.32 Video Analysis Using Deep Neural Networks: An Application for Autism
Participants: Abid Ali, Francois Bremond, Monique Thonnat.
Understanding actions in videos is a crucial element of computer vision with significant implications across various fields. As our dependence on visual data grows, comprehending and interpreting human actions in videos becomes essential for advancing technologies in surveillance, healthcare, autonomous systems, and human-computer interaction. The accurate interpretation of actions in videos is fundamental for creating intelligent systems that can effectively navigate and respond to the complexities of the real world. In this context, advances in action understanding push the boundaries of computer vision and play a crucial role in shaping the landscape of cutting-edge applications that impact our daily lives. Computer vision has made significant progress with the rise of deep learning methods such as convolutional neural networks (CNNs) pushing the boundaries of computer vision and enabling the computer vision community to advance in many domains, including image segmentation, object detection, scene understanding, and more. However, video processing remains limited compared to static images. In this work 40, we focus on action understanding, dividing it into two main parts: action recognition and action detection, and their application in the medical domain for autism analysis.
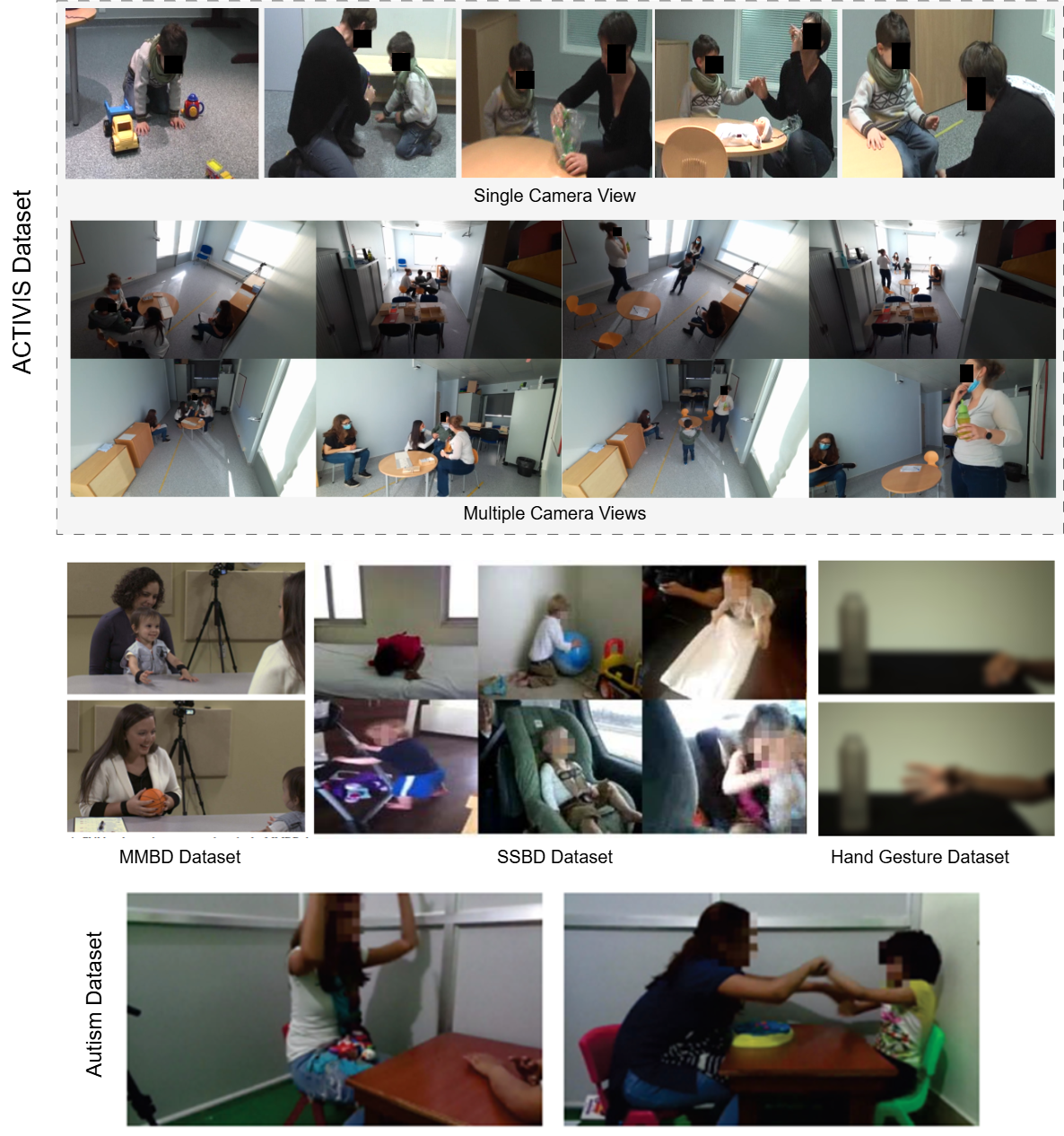
We explore the various aspects and challenges of video understanding from a general and an application-specific perspective. We then present our contributions and solutions to address these challenges. In addition, we introduce the ACTIVIS dataset (Figure 17), designed to diagnose autism in young children. Our work is divided into two main parts: generic modeling and applied models. Initially, we focus on adapting image models for action recognition tasks by incorporating temporal modeling using parameter-efficient fine-tuning (PEFT) techniques. We also address real-time action detection and anticipation by proposing a new joint model for action anticipation and online action detection in real-life scenarios 30. Furthermore, we introduce a new task called 'loose-interaction' in dyadic situations and its applications in autism analysis 24. Finally, we concentrate on the applied aspect of video understanding by proposing an action recognition model for repetitive behaviors in videos of autistic individuals. We conclude by proposing a weakly-supervised method to estimate the severity score of autistic children in long videos.
8.33 Quo Vadis, Video Understanding with Vision-Language Foundation Models?
Participants: Mahmoud Ali, Francois Bremond.
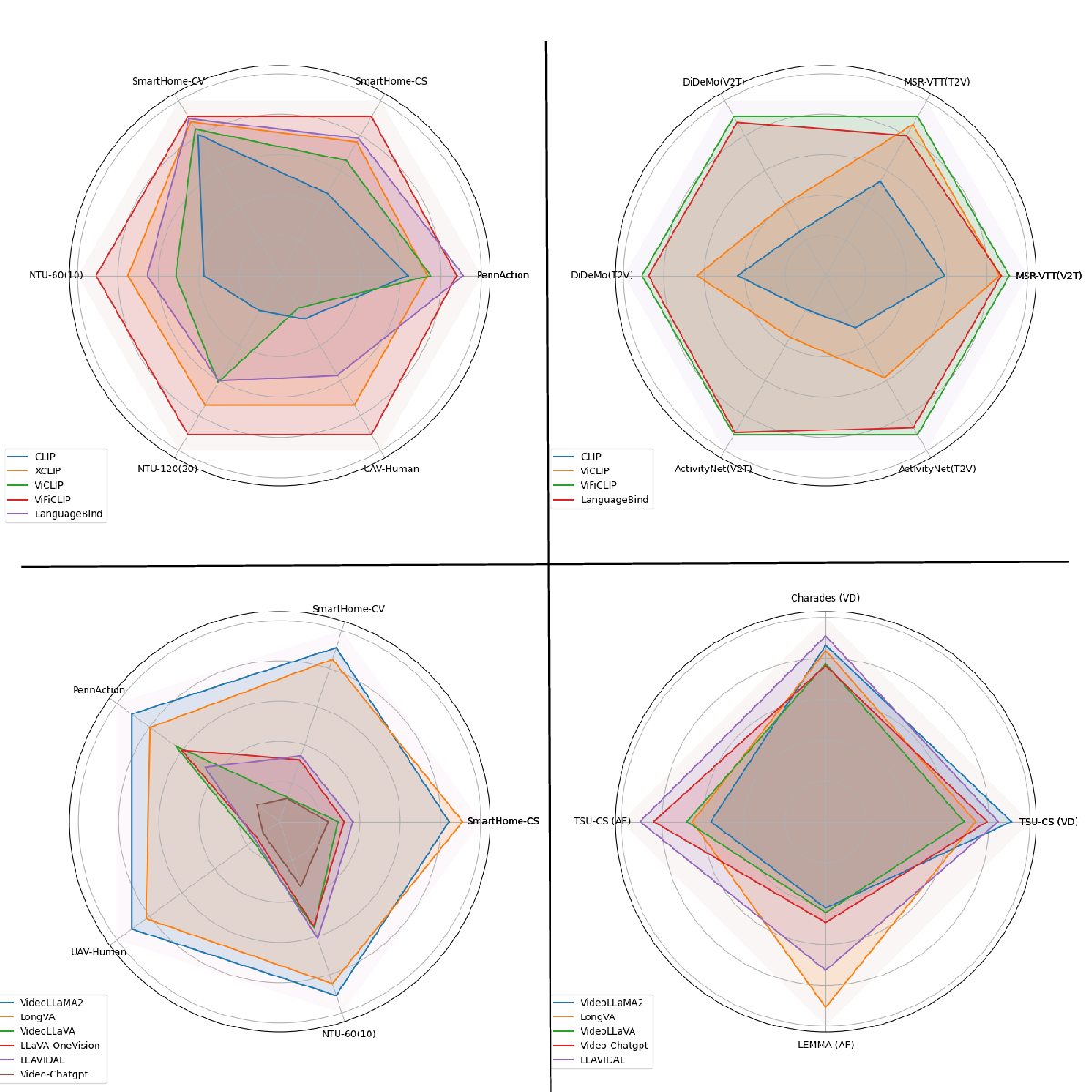
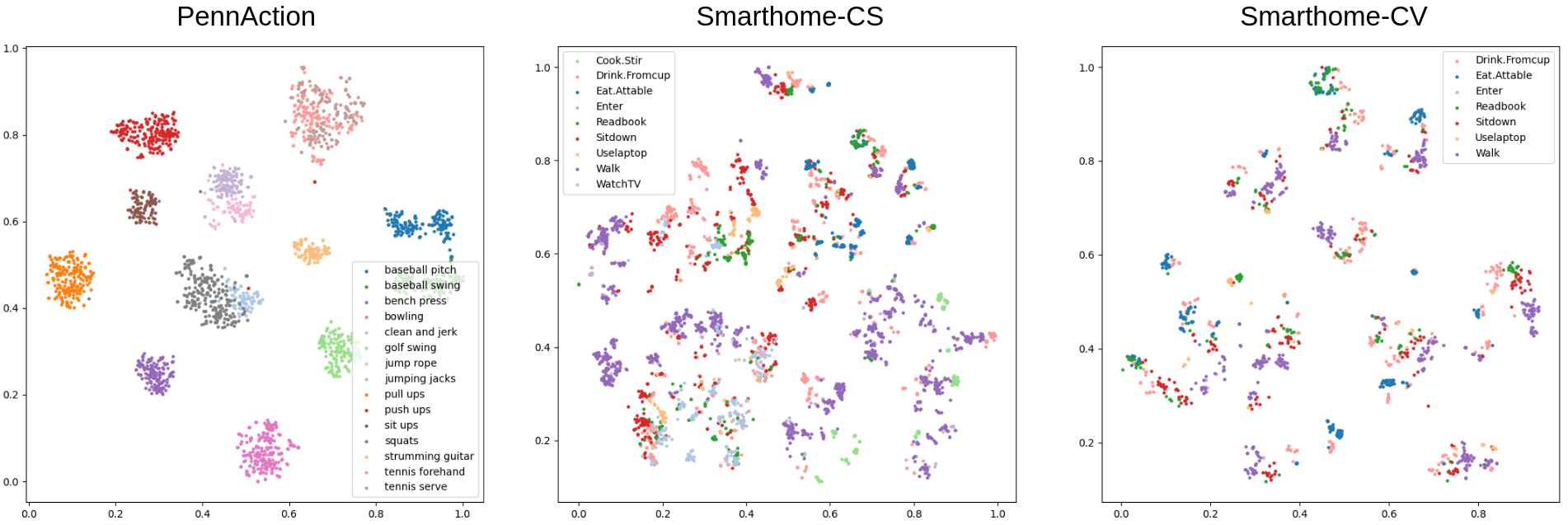
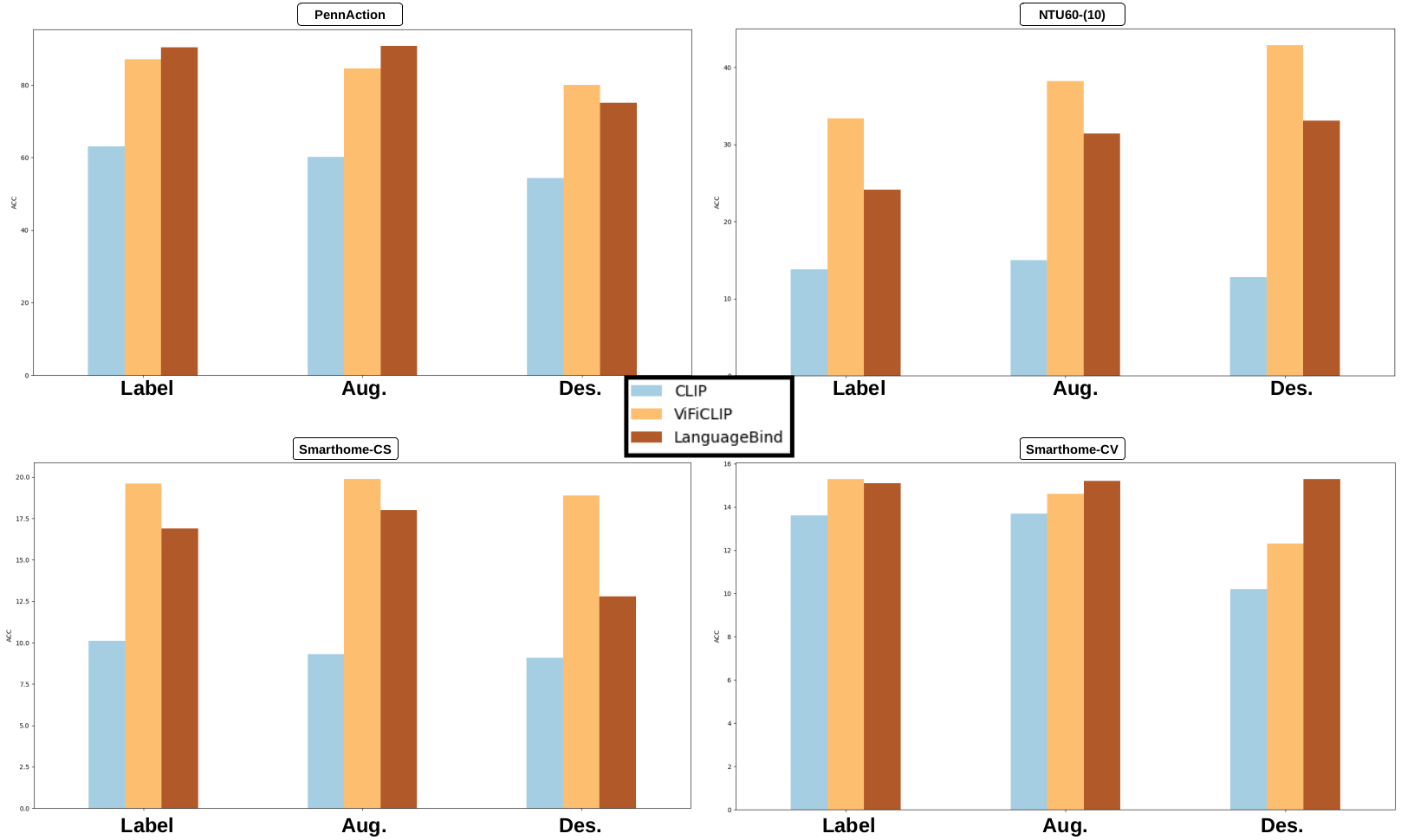
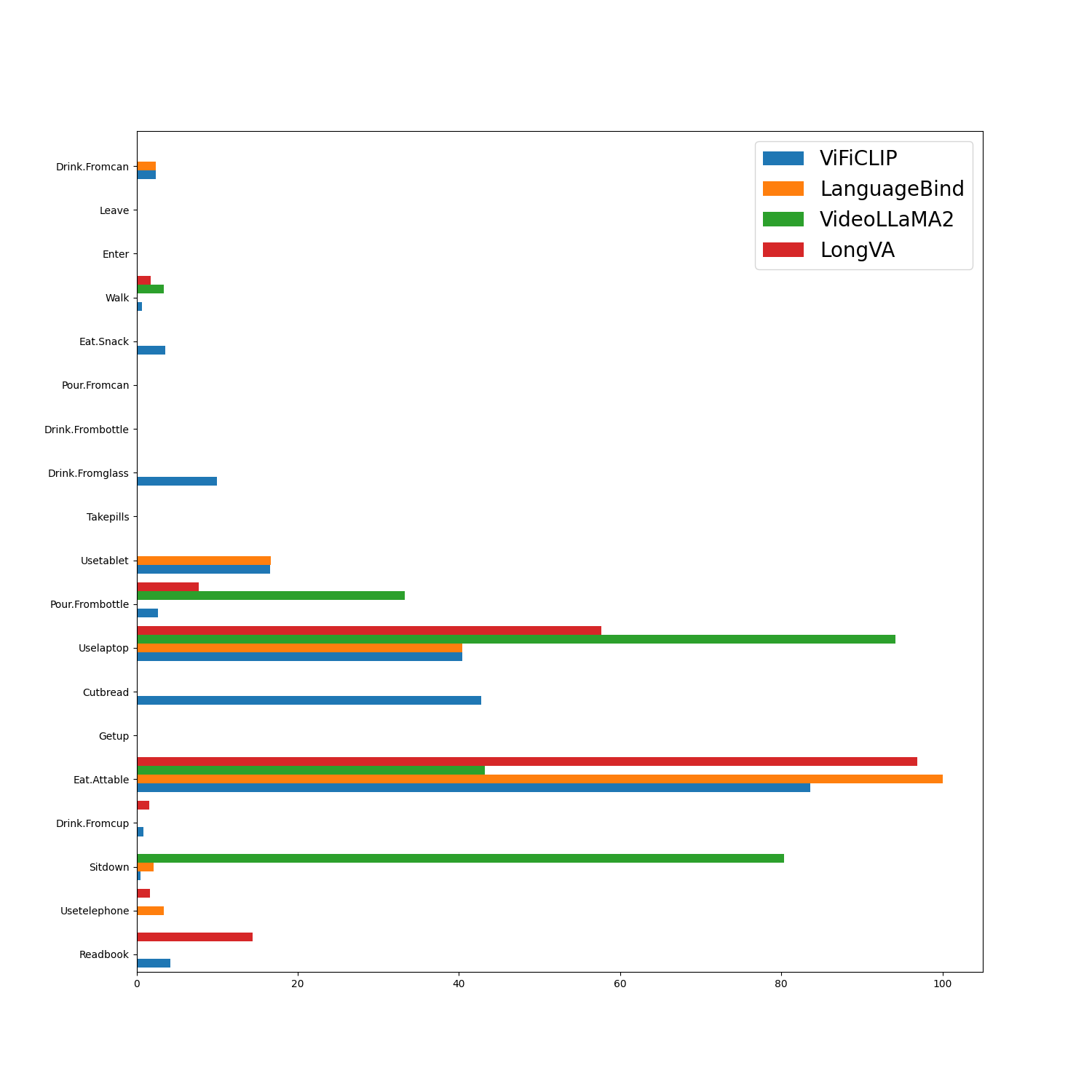
Vision-language foundation models (VLFMs), including vision-language models (VLMs) and vision-large language models (VLLMs), have evolved rapidly and demonstrated strong performance across various downstream video understanding tasks, particularly on web datasets. However, it remains an open question how well these models perform in more challenging scenarios, such as Activities of Daily Living (ADL).
In 25, we investigate the effectiveness of VLMs like CLIP for action recognition and segmentation tasks, focusing on their generalizability in zero-shot settings. In 26, we extend our study to VLLMs, evaluating them on five video understanding tasks across eleven datasets. We provide a comprehensive comparison of VLMs and VLLMs, examining their zero-shot transferability to five downstream tasks: action classification, video retrieval, video description, action forecasting, and frame-wise action segmentation. Extensive experiments were conducted on eleven real-world, human-centric video understanding datasets, including Toyota Smarthome, Penn Action, UAV-Human, EgoExo4D, TSU, and Charades. We provide in-depth analysis to understand the strengths and limitations of these models in zero-shot settings, such as identifying optimal video sampling strategies, analyzing the impact of action label descriptions on zero-shot action classification, and exploring the effects of fine-grained labels and diverse viewpoints on vision-language alignment.
The findings from our studies indicate that VLFMs are still far from achieving satisfactory performance in all evaluated tasks, as shown in Figure 18, particularly in densely labeled and long video datasets with fine-grained activities in complex, real-world scenarios, such as those in the TSU and Toyota Smarthome datasets. These models perform well on small, controlled datasets like PennAction, where they achieve an accuracy of 90.4%. However, their performance significantly drops on real-world datasets like Toyota Smarthome, where they achieve only 22.7%. This highlights their difficulty in discriminating between fine-grained and complex real-world actions, as shown in the t-SNE visualization in Figure 19. Most models struggle to detect motion-based actions like "walk," "leave," and "enter," focusing instead on stable actions with less motion, as depicted in Figure 21. VLFMs are also sensitive to text embeddings, as shown in Figure 20. For example, action descriptions can improve text features for zero-shot classification on datasets like NTU10. However, on datasets like Smarthome, where the original labels already provide significant contextual information (e.g., "people make coffee on the table"), augmenting action labels does not lead to performance improvements.
9 Bilateral contracts and grants with industry
Participants: Antitza Dantcheva, Francois Bremond.
Stars team has currently several experiences in technological transfer towards industries, which have permitted to exploit research result.
9.1 Bilateral contracts with industry
9.1.1 Toyota
This project runs from the 1st of August 2013 up to December 2025. It aims at detecting critical situations in the daily life of older adults living home alone.
Toyota is working with Stars on action recognition software to be integrated on their robot platform. This project aims at detecting critical situations in the daily life of older adults alone at home. This will require not only recognition of ADLs but also an evaluation of the way and timing in which they are being carried out. The system we want to develop is intended to help them and their relatives to feel more comfortable because they know that potentially dangerous situations will be detected and reported to caregivers if necessary. The system is intended to work with a Partner Robot - HSR - (to send real-time information to the robot) to better interact with the older adult.
9.1.2 Fantastic Sourcing
Fantastic Sourcing is a French SME specialized in micro-electronics, it develops e-health technologies. Fantastic Sourcing is collaborating with Stars through the UniCA Solitaria project, by providing their Nodeus system. Nodeus is an IoT (Internet of Things) system for home support for the elderly, which consists of a set of small sensors (without video cameras) to collect precious data on the habits of isolated people. Solitaria project performs a multi-sensor activity analysis for monitoring and safety of older and isolated people. With the increase of the ageing population in Europe and in the rest of the world, keeping elderly people at home, in their usual environment, as long as possible, becomes a priority and a challenge of modern society. A system for monitoring activities and alerting in case of danger, in permanent connection with a device (an application on a phone, a surveillance system ...) to warn relatives (family, neighbors, friends ...) of isolated people still living in their natural environment could save lives and avoid incidents that cause or worsen the loss of autonomy. In this R
10 Partnerships and cooperations
10.1 International initiatives
10.1.1 Inria associate team not involved in an IIL or an international program
GDD
Participants: Antitza Dantcheva, Francois Bremond.
-
Title:
Generalizable Deepfake Detection
-
Duration:
2022 - 2024
-
Coordinator:
Abhijit Das (abhijit.das@hyderabad.bits-pilani.ac.in)
-
Partners:
- Bits Pilani Hyderabad, India
-
Inria contact:
Antitza Dantcheva
-
Summary:
In this project, we will focus on Manipulated facial videos (deepfakes) which have become highly realistic due to the tremendous progress of deep convolutional neural networks (CNNs). While intriguing, such progress raises a number of social concerns related to fake news. We propose in GDD the design of deepfake detection algorithms, which can generalize in order to detect unknown manipulations.
10.1.2 Visits of international scientists
- Diana Borza visited STARS from May 2024 - July 2024.
- Giacomo D'Amicantonio visited STARS from August 2024 - November 2024.
- Yaohui Wang from the Shanghai AI lab visited STARS in November 2024.
10.1.3 Visits to international teams
Research stays abroad:
Francois Bremond and Monique Thonnat visited the Birla Institute of Technology and Science (BITS), Pilani, Hyderabad Campus, India within the framework of our associated team GDD December 9-13, 2024.
Antitza Dantcheva visited the Birla Institute of Technology and Science (BITS), Pilani, Hyderabad Campus, India within the framework of our associated team GDD October 28 - November 1, 2024.
10.2 European initiatives
10.2.1 Horizon Europe
GAIN
Participants: François Brémond.
-
Title:
Georgian Artificial Intelligence Networking and Twinning Initiative
-
Duration:
From October 1, 2022 to September 30, 2025
-
Partners:
- Institut National De Recherche En Informatique Et Automatique (Inria), France
- Exolaunch Gmbh (Exo), Germany
- Deutsches Forschungszentrum Fur Kunstliche Intelligenz Gmbh (Dfki), Germany
- Georgian Technical University (GTU), Georgia
-
Inria contact:
François Bremond
-
Coordinator:
George Giorgobiani, Deputy Director of Muskhelishvili Institute of Computational Mathematics (MICM), GTU.
-
Summary:
GAIN will take a strategic step towards integrating Georgia, one of the Widening countries, into the system of European efforts aimed at ensuring the Europe's leadership in one of the most transformative technologies of today and tomorrow - Artificial Intelligence (AI). It will be achieved by research profile adjusting and linking the central Georgian ICT research institute - Muskhelishvili Institute of Computational Mathematics (MICM), to the European AI research and innovation community. Two absolutely leading European research organizations (DFKI and INRIA) supported by the high-tech company EXOLAUNCH will support MICM in this endeavor. The Strategic Research and Innovation Programme (SRIP) designed by the partnership will provide the environment for the Georgian colleagues to get involved in the research projects of the European partners addressing a clearly delineated set of AI topics. Jointly, the partners will advance in capacity building and networking within the area of AI Methods and Tools for Human Activities Recognition and Evaluation, which also will contribute to strengthening core competences in such fundamental technologies as e.g. Machine (Deep) Learning. The results of the cooperation presented through the series of scientific publications and events will inform the European AI community about the potential of MICM and trigger new partnerships building, addressing e.g. Horizon Europe. The project will contribute to career development of a cohort of young researchers at MICM through joint supervision and targeted capacity building measures. Innovation and Research Administration and Management capacities of MICM will also be strengthen to allow the Institute to be better connected to the local, regional and European innovation activities. Using their extensive research and innovation networking capacities DFKI and INRIA will introduce MICM to the European AI research community by connecting to such networks as CLAIRE, ELLIS, ADRA, AI NoEs, etc.
10.2.2 H2020 projects
HEROES
Participants: Francois Bremond.
-
Title:
Novel Strategies to Fight Child Sexual Exploitation and Human Trafficking Crimes and Protect their Victims
-
Duration:
From December 1, 2021 to November 30, 2024
-
Partners:
- Institut National De Recherche En Informatique Et Automatique (Inria), France
- Policia Federal, Brazil
- Elliniko Symvoulio Gai Tous Prosfyges (Greek Council For Refugees), Greece
- International Centre For Migration Policy Development (ICMPD), Austria
- Universidade Estadual De Campinas (UNICAMP), Brazil
- Associacao Brasileira De Defesa Da Muhler Da Infancia E Da Juventude (ASBRAD), Brazil
- Kovos Su Prekyba Zmonemis Ir Isnaudojimu Centras Vsi (KOPZI), Lithuania
- Fundacion Renacer, Colombia
- Trilateral Research Limited (TRI IE), Ireland
- Vrije Universiteit Brussel (VUB), Belgium
- Athina-Erevnitiko Kentro Kainotomias Stis Technologies Tis Pliroforias, Ton Epikoinonion Kai Tis Gnosis (ATHENA - RESEARCH AND INNOVATION CENTER), Greece
- The Global Initiative Verein Gegen Transationale Organisierte Kriminalitat, Austria
- Esphera - Cultural,Ambiental E Social, Brazil
- Fundacao Universidade De Brasilia (Universidade De Brasília), Brazil
- Idener Research & Development Agrupacion De Interes Economico (Idener Research & Development AIE), Spain
- Universidad Complutense De Madrid (UCM), Spain
- University Of Kent (UNIKENT), United Kingdom
- Kentro Meleton Asfaleias (Center Forsecurity Studies Centre D'etudes De Securite), Greece
- Trilateral Research LTD, United Kingdom
- Policia Rodoviaria Federal (Federa Highway Police), Brazil
- Ministerio Del Interior (Ministry Of The Interior), Spain
- Iekslietu Ministrijas Valsts Policija State Police Of The Ministry Of Interior (State Police Of Latvia), Latvia
- Secretaria De Inteligencia Estrategica De Estado - Presidencia De La Republica Oriental Del Uruguay (SIEE), Uruguay
- Associacao Portuguesa De Apoio A Vitima, Portugal
- Comando Conjunto De Las Fuerzas Armadas Del Peru (Comando Conjunto De Las Fuerzas Armadas Del Peru), Peru
- International Center For Missing And Exploited Children Switzerland, Switzerland
- Hellenic Police (Hellenic Police), Greece
- Centre For Women And Children Studies (CWCS), Bangladesh
- Glavna Direktsia Borba S Organiziranata Prestupnost (Chief Directorate Fight With Organised Crime), Bulgaria
-
Inria contact:
François Bremond
-
Summary:
Trafficking of human beings (THB) and child sexual abuse and exploitation (CSA/CSE) are two big problems in our society. Inadvertently, new information and communication technologies (ICTs) have provided a space for these problems to develop and take new forms, made worse by the lockdown caused by the COVID-19 pandemic. At the same time, technical and legal tools available to stakeholders that prevent, investigate, and assist victims – such as law enforcement agencies (LEAs), prosecutors, judges, and civil society organizations (CSOs) – fail to keep up with the pace at which criminals use new technologies to continue their abhorrent acts. Furthermore, assistance to victims of THB and CSA/CSE is often limited by the lack of coordination among these stakeholders. In this sense, there is a clear and vital need for joint work methodologies and the development of new strategies for approaching and assisting victims. In addition, due to the cross-border nature of these crimes, harmonization of legal frameworks from each of the affected countries is necessary for creating bridges of communication and coordination among all those stakeholders to help victims and reduce the occurrence of these horrendous crimes. To address these challenges, the HEROES project comes up with an ambitious, interdisciplinary, international, and victim-centred approach. The HEROES project is structured as a comprehensive solution that encompasses three main components: Prevention, Investigation and Victim Assistance. Through these components, our solution aims to establish a coordinated contribution with LEAs by developing an appropriate, victim-centered approach that is capable of addressing specific needs and providing protection. The HEROES project's main objective is to use technology to improve the way in which help and support can be provided to victims of THB and CSA/CSE.
10.3 National initiatives
3IA
Participants: François Brémond.
-
Title:
Video Analytics for Human Behavior Understanding (axis 2),
-
Duration:
From 2019
-
Chair holder:
François Brémond
-
Summary:
The goal of this chair is to design novel modern AI methods (including Computer Vision and Deep Learning algorithms) to build real-time systems for improving health and well-being as well as people safety, security and privacy. Behavior disorders affect the mental health of a growing number of people and are hard to handle, leading to a high cost in our modern society. New AI techniques can enable a more objective and earlier diagnosis, by quantifying the level of disorders and by monitoring the evolution of the disorders. AI techniques can also learn the relationships between the symptoms and their true causes, which are often hard to identify and measure.
ACTIVIS
Participants: François Brémond, Monique Thonnat.
-
Title:
ACTIVIS: Video-based analysis of autism behavior
-
Duration:
From 2020 - 2025
-
Partners:
Inria, Aix-Marseille Université - LIS, Hôpitaux Pédiatriques Nice CHU-Lenval - CoBTeK, Nively
-
Inria contact:
François Brémond
-
Coordinator:
Aix-Marseille Université - LIS
-
Summary:
The ACTIVIS project is an ANR project (CES19: Technologies pour la santé) started in January 2020 and will end in December 2025. This project is based on an objective quantification of the atypical behaviors on which the diagnosis of autism is based, with medical (diagnostic assistance and evaluation of therapeutic programs) and computer scientific (by allowing a more objective description of atypical behaviors in autism) objectives. This quantification requires video analysis of the behavior of people with autism. In particular, we propose to explore the issues related to the analysis of ocular movement, gestures and posture to characterize the behavior of a child with autism.
11 Dissemination
11.1 Promoting scientific activities
11.1.1 Scientific events: organisation
General chair, scientific chair:
François Brémond was General Chair at IPAS 2025 (Web-site) [130 people], the IEEE International Conference on Image Processing, Applications and Systems, Lyon, Jan 2025.
Member of the organizing committees:
- François Brémond was Publication Chair, for the IEEE ECCV'24 [8000 people], Milan, Italy, Oct 2024.
- Antitza Dantcheva was Workshop Chair at CVPR'24.
- Antitza Dantcheva was Area Chair at ECCV'24.
- Michal Balazia was organizer of the ACM Grand Challenge MultiMediate'24 at ACMMM'24.
11.1.2 Scientific events: selection
Member of the conference program committees
Monique Thonnat was member of conference program committee IJCAI-2024 and ICPRAM 2025.
Reviewer:
- François Brémond was reviewer in major Computer Vision and Machine Learning conferences including ICCV (International Conference on Computer Vision), ECCV (European Conference on Computer Vision), WACV (Winter Conference on Applications of Computer Vision), CVPR (Computer Vision and Pattern Recognition), NeurIPS (Neural Information Processing Systems), AAAI (Association for the Advancement of Artificial Intelligence), ICLR (International Conference on Learning Representations).
- Michal Balazia was reviewer at WACV'25, ICPR'24, ACMMM'24, T-CAP'24 and one ANR project.
- Michal Balazia was in the Technical committee at T-CAP'24.
11.1.3 Invited talks:
- François Brémond was invited to give a tutorial, part of a workshop on "Privacy" invited by Kate Kaye from the World Privacy Forum.
- Francois Bremond was invited to give a virtual talk at Birla Institute of Technology and Science, Pilani, Hyderabad Campus, India on October 30, 2024 at Raise Workshop in the framework of our associated team GDD.
- François Brémond was invited to give an invited talk (40min) at IPAS 2025 , IEEE International Conference on Image Processing, Applications and Systems, Lyon, January 9-11, 2025.
- François Brémond was invited to give a talk part of a Japan - JST Delegation Nice/Sophia September 26-27, 2024.
- François Brémond was invited to give a talk part of DiEpiMED2024: International Conference on Digital Epidemiology September 12, 2024.
- Antitza Dantcheva was invited to give talks
- at the University of Cagliari on February 28, 2024.
- on "Generative Models" for the Ministère de l'intérieur France, March 12, 2024.
- for the visit of the Député Eric Pauget March 15, 2024 at Inria.
- on "Deciphering human faces with the means of artificial intelligence" at centenary of the syndrome "Doppelganger Illusion" organized by the Société Medico-Psychologique, on April 24, 2024 in Paris, France.
- at the "Generative AI" session of the Journées Scientifiques Inria August 29, 2024 in Grenoble.
- for the visit of the Japan Society for Research (JST) on September 27, 2024.
- for the visit of Comité d'Administration Régionale (with 6 Préfets of the region) on October 16, 2024.
- in the premises of VIGINUM on October 18, 2024.
- at the Birla Institute of Technology and Science (BITS), Pilani, Hyderabad Campus, India on October 30, 2024 at the Raise Workshop in the framework of our associated team GDD.
- Michal Balazia was invited to give a talk at the CoBTeK meeting, ICP Nice, February 15, 2024 and December 12, 2024.
- Michal Balazia was invited to give a talk at the Journées scientifiques Handicap et numérique, Inria Paris.
11.1.4 Scientific expertise
- François Brémond was a member of the ANR Scientific Evaluation Committee - CES 23 “Artificial Intelligence and Data Science” on June 12-14, 2024 and of the ANR Scientific Committee WISG 2024 “Interdisciplinary Workshop on Global Security” on March 14-15, 2024.
- Monique Thonnat evaluated ANR projects in the framework of comité d'évaluation “CE45 - Interfaces : mathématiques, sciences du numérique - biologie, santé”.
- Antitza Dantcheva was in the hiring committee for MC UNICAEN IUT GON & GREYC at the Université de Caen, and in the hiring committee CRCN and ISFP at Inria, Sophia Antipolis.
11.2 Teaching - Supervision - Juries
11.2.1 Teaching
- François Brémond organized and lectured AI courses on Computer Vision & Deep Learning for the Data Science and AI - MSc program at Université Côte d'Azur: 30h class at Université Côte d'Azur in 2024. Web-site
- Antitza Dantcheva taught 2 classes at Polytech Nice Sophia - Univ Côte d'Azur (Applied Artificial Intelligence, Master 2).
- Snehashis Majhi taught two lectures for MSc. Data Science and Artificial Intelligence, UniCA.
- Tomasz Stanczyk taught one lecture for MSc. Data Science and Artificial Intelligence, UniCA.
- Valeriya Strizhkova taught one lecture for MSc. Data Science and Artificial Intelligence, UniCA and one research project for DSAI.
- Baptiste Chopin taught one lecture for the course Applied Artificial Intelligence, Master 2, Polytech Nice-Sophia, September 2022.
11.2.2 Supervision
- François Brémond has (co-)supervised 8 PhD students and 6 master students.
- Antitza Dantcheva has (co-)supervised 4 PhD students and 2 master students.
- Michal Balazia supervised the undergraduate students Aglind Reka, Utkarsh Tiwari, Sanya Sinha, Sripadam Sujith Sai.
11.2.3 Juries
François Brémond was
- HDR committee examiner : Stéphane Lathuilière, Telecom-Paris Multimedia, October 9, 2024,
- Selection committee for MCF position in Speech Therapy - Université Côte d'Azur, September 11, 2024.
François Brémond was in the PhD committees of
- Romain Guesdon from Université Lumière - Lyon 2 on February 14, 2024.
- Ziming Liu from Université Côte d'Azur on February 28, 2024
- Mehran Hatamzadeh, Université Côte d'Azur on November 4, 2024.
- Huiyu Li at Université Côte d'Azur on the November 28, 2024
- Nima Mehdi, at Université de Lorraine on December 17, 2024.
Antitza Dantcheva was PhD committees
- Guillaume Jeanneret from Université de Caen on September 25, 2024 (Reviewer).
- Baptiste Pouthier from Université Côte d'Azur on the June 3, 2024 (Examiner).
Monique Thonnat was PhD committee chair: PhD of Jules Colennes University Aix Marseille, on December 10, 2024.
François Brémond was CS (Scientific Committee) of
- Kaushik Bhowmik, Université Grenoble-alpes, July 8, 2024,
- Marc Chapus, Laboratory LIRIS, Lyon, June 10, 2024,
- Fabien Lionti, Université Côte d'Azur, May 6, 2024.
- Franz Franco Gallo, Université Côte d'Azur, May 23, 2024.
- Yannick Porto, University Bourgogne Franche Comté, August 27, 2023.
- Idir Chatar, Université Côte d'Azur, September 8, 2024,
- Sofia Alexopoulou, Université Côte d'Azur, July 23, 2024,
- Monica Fossati, Université Côte d'Azur, May 21, 2024,
- Federica Facente, Université Côte d'Azur, June 11,2024,
- Keqi Chen, University of Strasbourg, May 22, 2024.
Antitza Dantcheva was in the CSs(Scientific Committees) of
- Sahar Husseini, Eurecom, October 17, 2024,
- Mehdi Atamna, Université Lumière Lyon 2, May 28, 2024.
11.3 Popularization
11.3.1 Specific official responsibilities in science outreach structures
- François Brémond was invited to participate to the "Fête de la science à Antibes : Reconnaissance d'activité humaine", October 2024.
- François Brémond and Antitza Dantcheva were invited to discuss "Intelligence artificielle, le vrai et le Fake" at an "apéritif des sciences sur l'IA" on the 21st February 2024.
- François Brémond was invited to host 12 interns "3ème" part of Terra Numerica, on the 21st February.
- Monique Thonnat was invited to "Journées scientifiques Handicap et numérique" at Inria Paris on 7-8 March 2024.
11.3.2 Participation in Live events
Antitza Dantcheva gave a talk at the Lycée du Parc Impérial in Nice in the course of the CHICHE Programme on the March 26, 2024.
Antitza Dantcheva gave a talk at the Ecole des 3 collines in Mougins on the June 28, 2024.
Antitza Dantcheva attended the Bits & Pretzels Founders Festival in Munich, 29 Sep - 01 oct 2024.
12 Scientific production
12.1 Major publications
- 1 inproceedingsLearning to match appearances by correlations in a covariance metric space.European Conference on Computer VisionSpringer2012, 806--820
- 2 articleExploiting Feature Correlations by Brownian Statistics for People Detection and Recognition.IEEE transactions on systems, man, and cybernetics2016HAL
- 3 inproceedingsVideo Covariance Matrix Logarithm for Human Action Recognition in Videos.IJCAI 2015 - 24th International Joint Conference on Artificial Intelligence (IJCAI)Buenos Aires, ArgentinaJuly 2015HAL
- 4 articleSemantic Event Fusion of Different Visual Modality Concepts for Activity Recognition.IEEE Transactions on Pattern Analysis and Machine Intelligence382016, 1598 - 1611HALDOI
- 5 articleGender estimation based on smile-dynamics.IEEE Transactions on Information Forensics and Security2016, 11HALDOI
- 6 inproceedingsToyota Smarthome: Real-World Activities of Daily Living.ICCV 2019 -17th International Conference on Computer VisionSeoul, South KoreaOctober 2019HAL
- 7 inproceedingsVPN: Learning Video-Pose Embedding for Activities of Daily Living.ECCV 2020 - 16th European Conference on Computer VisionGlasgow (Virtual), United KingdomAugust 2020HAL
- 8 inproceedingsGesture Recognition by Learning Local Motion Signatures.CVPR 2010 : IEEE Conference on Computer Vision and Pattern RecognitionSan Franscico, CA, United StatesIEEE Computer Society PressJune 2010HAL
- 9 articleRecognizing Gestures by Learning Local Motion Signatures of HOG Descriptors.IEEE Transactions on Pattern Analysis and Machine Intelligence2012HAL
- 10 inproceedingsKnowledge Representation for Program Reuse.European Conference on Artificial Intelligence (ECAI)Lyon, FranceJuly 2002, 240-244
- 11 articleBlocks, a Component Framework with Checking Facilities for Knowledge-Based Systems.Informatica, Special Issue on Component Based Software Development254November 2001, 501-507
- 12 articleCompilation Modulaire d'un Langage Synchrone.Revue des sciences et technologies de l'information, série Théorie et Science Informatique430June 2011, 441-471URL: http://hal.inria.fr/inria-00524499/en
- 13 article What Can Program Supervision Do for Software Re-use? IEE Proceedings - Software Special Issue on Knowledge Modelling for Software Components Reuse 147 5 2000
- 14 inproceedingsAutomatic Video Interpretation: A Novel Algorithm based for Temporal Scenario Recognition.The Eighteenth International Joint Conference on Artificial Intelligence (IJCAI'03)Acapulco, Mexico9-15 September 2003
- 15 inproceedingsG3AN: Disentangling Appearance and Motion for Video Generation.CVPR 2020 - IEEE Conference on Computer Vision and Pattern RecognitionSeattle / Virtual, United StatesJune 2020HAL
12.2 Publications of the year
International journals
- 16 articleAnti-Forgetting Adaptation for Unsupervised Person Re-identification.IEEE Transactions on Pattern Analysis and Machine IntelligenceNovember 2024HALDOIback to text
- 17 articleSynthetic Data in Human Analysis: A Survey.IEEE Transactions on Pattern Analysis and Machine Intelligence2024, 1-20HALDOIback to text
- 18 articleLEO: Generative Latent Image Animator for Human Video Synthesis.International Journal of Computer VisionSeptember 2024HALDOIback to text
- 19 articleLIA: Latent Image Animator.IEEE Transactions on Pattern Analysis and Machine Intelligence4612December 2024, 10829-10844HALDOIback to text
- 20 articleView-invariant Skeleton Action Representation Learning via Motion Retargeting.International Journal of Computer VisionJanuary 2024HALDOIback to text
International peer-reviewed conferences
- 21 inproceedingsCM3T: Framework for Efficient Multimodal Learning for Inhomogeneous Interaction Datasets.Winter Conference on Applications of Computer VisionTucson, United StatesMarch 2025HALback to text
- 22 inproceedingsWeakly-supervised Autism Severity Assessment in Long Videos.CBMI 2024 - International Conference on Content-based Multimedia IndexingReyjavik, Iceland2024HALback to text
- 23 inproceedingsP-Age: Pexels Dataset for Robust Spatio-Temporal Apparent Age Classification.WACV 2024 - Winter Conference on Applications of Computer VisionHAWAII, United StatesJanuary 2024HALback to text
- 24 inproceedingsLoose Social-Interaction Recognition in Real-world Therapy Scenarios.WACV 2025 - IEEE/CVF Winter Conference on Applications of Computer VisionTucson, United StatesarXivFebruary 2025HALback to text
- 25 inproceedingsAre Visual-Language Models Effective Action Recognition? A Comparative Study.Lecture notes in computer scienceECCVW 2024 - 18th European Conference on Computer VisionMilan, ItalySeptember 2024HALback to text
- 26 inproceedings Quo Vadis, Video Understanding with Vision-Language Foundation Models? NeurIPS Proceedings Neurips 2024 - 38th Annual Conference on Neural Information Processing Systems Vancouver (Canada), Canada December 2024 HAL back to text
- 27 inproceedingsBipartite Graph Diffusion Model for Human Interaction Generation: The generation of natural human motion interactions is a hot topic in computer vision and computer animation. It is a challenging task due to the diversity of possible human motion interactions. Diffusion models, which have already shown remarkable generative capabilities in other domains, are a good candidate for this task. In this paper, we introduce a novel bipartite graph diffusion method (BiGraphDiff) to generate human motion interactions between two persons. Specifically, bipartite node sets are constructed to model the inherent geometric constraints between skeleton nodes during interactions. The interaction graph diffusion model is transformer-based, combining some state-of-the-art motion methods. We show that the proposed achieves new state-of-the-art results on leading benchmarks for the human interaction generation task.WACV 2024 - IEEE/CVF Winter Conference on Applications of Computer VisionWAIKOLOA (Hawaii), United StatesJanuary 2024HALback to text
- 28 inproceedingsGHNeRF: Learning Generalizable Human Features with Efficient Neural Radiance Fields.IEEE XploreCVPR 2024 - IEEE/CVF Conference on Computer Vision and Pattern Recognition Workshops2024 IEEE/CVF Conference on Computer Vision and Pattern Recognition (CVPR)Seattle (USA), United StatesJune 2024, 2812-2821HALDOIback to text
- 29 inproceedingsHFNeRF: Learning Human Biomechanic Features with Neural Radiance Fields.IEEE XploreIEEE CAI 2025 - IEEE Conference on Artificial Intelligence2024 IEEE Conference on Artificial Intelligence (CAI)Singapore, SingaporeJune 2024HALDOIback to text
- 30 inproceedingsJOADAA: joint online action detection and action anticipation.WACV 2024 - IEEE/CVF Winter Conference on Applications of Computer VisionWaikoloa (Hawaii), United StatesJanuary 2024HALback to text
- 31 inproceedingsLocal Distributional Smoothing for Noise-invariant Fingerprint Restoration.International Conference of the Biometrics Special Interest Group (BIOSIG)Darmstadt (Germany), GermanySeptember 2024HALback to text
- 32 inproceedingsOE-CTST: Outlier-Embedded Cross Temporal Scale Transformer for Weakly-supervised Video Anomaly Detection.WACV 2024 - IEEE/CVF Winter Conference on Applications of Computer VisionHawaii, United StatesJanuary 2024HALback to text
- 33 inproceedingsMultiMediate'24: Multi-Domain Engagement Estimation.ACM Digital LibraryMM 2024 : The 32nd ACM International Conference on MultimediaMM '24: Proceedings of the 32nd ACM International Conference on MultimediaMelbourne, AustraliaACMOctober 2024, 11377-11382HALDOIback to text
- 34 inproceedingsIntroducing Gating and Context into Temporal Action Detection.SpringerlinkECCVW 2024Milan, ItalySeptember 2024HALback to text
- 35 inproceedingsIdentifying Surgical Instruments in Pedagogical Cataract Surgery Videos through an Optimized Aggregation Network.IPAS 2025 - Sixth IEEE International Conference on Image Processing Applications and SystemsLyon, FranceJanuary 2025HALback to text
- 36 inproceedingsVideo Representation Learning for Conversational Facial Expression Recognition Guided by Multiple View Reconstruction.Conference on Computer Vision and Pattern Recognition (CVPR) WorkshopsSeattle (USA), Washington, United StatesJune 2024, 4693-4702HALback to text
- 37 inproceedingsMVP: Multimodal Emotion Recognition based on Video and Physiological Signals.SpringerlinkECCVW 2024Milan, ItalySeptember 2024HALback to text
- 38 inproceedingsWhat Matters in Autonomous Driving Anomaly Detection: A Weakly Supervised Horizon.Lecture Notes in Computer ScienceECCVW 2024 - 18th European Conference on Computer VisionLNCS-15059 up to 15147Computer Vision – ECCV 2024 18th European Conference, Milan, Italy, September 29–October 4, 2024, ProceedingsMilan, ItalySeptember 2024HALback to text
Conferences without proceedings
- 39 inproceedingsDiffTV: Identity-Preserved Thermal-to-Visible Face Translation via Feature Alignment and Dual-Stage Conditions.ACM MultimediaMelbourne (AUS), AustraliaOctober 2024HALback to text
Doctoral dissertations and habilitation theses
- 40 thesisVideo analysis using deep neural networks : an application for autism.Université Côte d'AzurDecember 2024HALback to text
- 41 thesisHuman activity recognition in videos.Université Côte d'AzurMay 2024HALback to text
- 42 thesisLearning effective video representations for action recognition.Université Côte d'AzurFebruary 2024HALback to text