Keywords
Computer Science and Digital Science
- A3.4. Machine learning and statistics
- A5.1. Human-Computer Interaction
- A5.3. Image processing and analysis
- A5.4. Computer vision
- A5.7. Audio modeling and processing
- A5.10.2. Perception
- A5.10.5. Robot interaction (with the environment, humans, other robots)
- A9.2. Machine learning
- A9.5. Robotics
Other Research Topics and Application Domains
- B5.6. Robotic systems
1 Team members, visitors, external collaborators
Research Scientists
- Radu Horaud [Team leader, Inria, Senior Researcher, HDR]
- Xavier Alameda-Pineda [Inria, Researcher, HDR]
- Chris Reinke [Inria, Starting Research Position, from Mar 2020]
- Mostafa Sadeghi [Inria, Starting Research Position, from Aug 2020 until Oct 2020]
- Timothee Wintz [Inria, Starting Research Position, from Apr 2020]
Post-Doctoral Fellow
- Mostafa Sadeghi [Inria, until Jul 2020]
PhD Students
- Anand Ballou [Univ Grenoble Alpes]
- Xiaoyu Bie [Univ Grenoble Alpes]
- Guillaume Delorme [Inria]
- Wen Guo [Univ Grenoble Alpes]
- Gaetan Lepage [Inria, from Oct 2020]
- Xiaoyu Lin [Inria, from Nov 2020]
- Yihong Xu [Inria]
Technical Staff
- Soraya Arias [Inria, Engineer]
- Alex Auternaud [Inria, Engineer]
- Luis Gomez Camara [Inria, Engineer, from Sep 2020]
- Zhiqi Kang [Inria, Engineer, from Sep 2020]
- Matthieu Py [Inria, Engineer, from Feb 2020]
Interns and Apprentices
- Alvaro Gonzalez Jimenez [ENSIMAG, from Feb 2020 until Jul 2020]
- Zhiqi Kang [Inria, from Feb 2020 until Aug 2020]
- Viet Nhat Nguyen [Inria, from Feb 2020 until Aug 2020]
- Predrag Pilipovic [Inria, from Feb 2020 until Jul 2020]
Administrative Assistant
- Nathalie Gillot [Inria]
External Collaborators
- Yutong Ban [MIT, Boston Ma, US, until May 2020]
- Laurent Girin [Institut polytechnique de Grenoble, HDR]
- Simon Leglaive [CNRS, until Aug 2020]
- Xiaofei Li [Université WestLake Shangaï - Chine]
2 Overall objectives
2.1 Audio-Visual Machine Perception
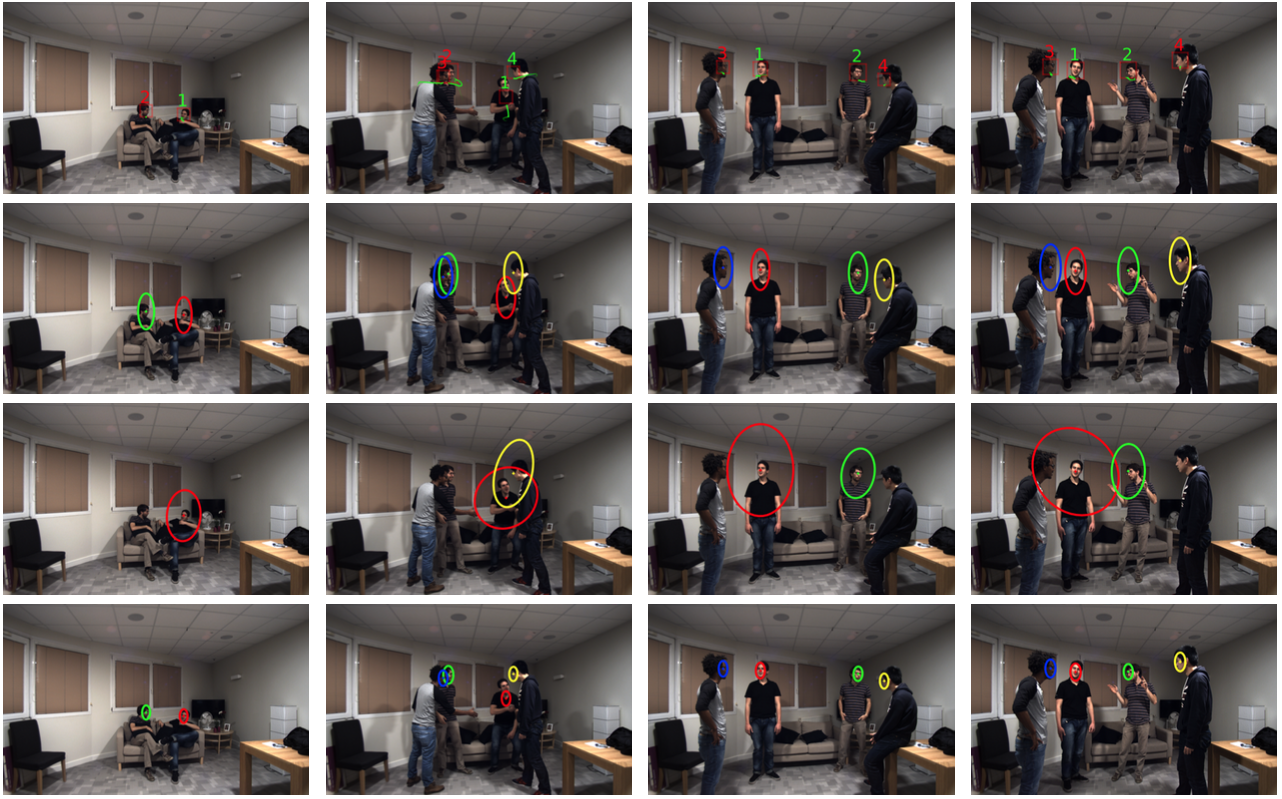
This figure illustrates the audio-visual multiple-person tracking that has been developed by the team 43, 30. The tracker is based on variational inference 5 and on supervised sound-source localization 11, 34. Each person is identified with a digit. Green digits denote speaking persons, while red digits denote silent ones. The next rows show the covariances (uncertainties) associated with the visual (second row), audio (third row) and dynamic (fourth row) contributions for tracking a varying number of persons. Notice the large uncertainty associated with audio and the small uncertainty associated with the dynamics of the tracker. In the light of this example, one may notice the complementary roles played by vision and audio: vision data are more accurate while audio data provide speech information (who speaks when). These developments have been supported by the European Union via the FP7 STREP project “Embodied Audition for Robots" (EARS), the ERC advanced grant “Vision and Hearing in Action" (VHIA) and ERC Proof of Concept grand VHIALab.
Auditory and visual perception play a complementary role in human interaction. Perception enables people to communicate based on verbal (speech and language) and non-verbal (facial expressions, visual gaze, head movements, hand and body gesturing) communication. These communication modalities have a large degree of overlap, in particular in social contexts. Moreover, the modalities disambiguate each other whenever one of the modalities is weak, ambiguous, or corrupted by various perturbations. Human-computer interaction (HCI) has attempted to address these issues, e.g., using smart & portable devices. In HCI the user is in the loop for decision taking: images and sounds are recorded purposively in order to optimize their quality with respect to the task at hand.
However, the robustness of HCI based on speech recognition degrades significantly as the microphones are located a few meters away from the user. Similarly, face detection and recognition work well under limited lighting conditions and if the cameras are properly oriented towards a person. Altogether, the HCI paradigm cannot be easily extended to less constrained interaction scenarios which involve several users and whenever is important to consider the social context.
The PERCEPTION team investigates the fundamental role played by audio and visual perception in human-robot interaction (HRI). The main difference between HCI and HRI is that, while the former is user-controlled, the latter is robot-controlled, namely it is implemented with intelligent robots that take decisions and act autonomously. The mid term objective of PERCEPTION is to develop computational models, methods, and applications for enabling non-verbal and verbal interactions between people, analyze their intentions and their dialogue, extract information and synthesize appropriate behaviors, e.g., the robot waves to a person, turns its head towards the dominant speaker, nods, gesticulates, asks questions, gives advices, waits for instructions, etc. The following topics are thoroughly addressed by the team members: audio-visual sound-source separation and localization in natural environments, for example to detect and track moving speakers, inference of temporal models of verbal and non-verbal activities (diarisation), continuous recognition of particular gestures and words, context recognition, and multimodal dialogue.
3 Research program
3.1 Audio-Visual Scene Analysis
From 2006 to 2009, R. Horaud was the scientific coordinator of the collaborative European project POP (Perception on Purpose), an interdisciplinary effort to understand visual and auditory perception at the crossroads of several disciplines (computational and biological vision, computational auditory analysis, robotics, and psychophysics). This allowed the PERCEPTION team to launch an interdisciplinary research agenda that has been very active for the last five years. There are very few teams in the world that gather scientific competences spanning computer vision, audio signal processing, machine learning and human-robot interaction. The fusion of several sensorial modalities resides at the heart of the most recent biological theories of perception. Nevertheless, multi-sensor processing is still poorly understood from a computational point of view. In particular and so far, audio-visual fusion has been investigated in the framework of speech processing using close-distance cameras and microphones. The vast majority of these approaches attempt to model the temporal correlation between the auditory signals and the dynamics of lip and facial movements. Our original contribution has been to consider that audio-visual localization and recognition are equally important. We have proposed to take into account the fact that the audio-visual objects of interest live in a three-dimensional physical space and hence we contributed to the emergence of audio-visual scene analysis as a scientific topic in its own right. We proposed several novel statistical approaches based on supervised and unsupervised mixture models. The conjugate mixture model (CMM) is an unsupervised probabilistic model that allows to cluster observations from different modalities (e.g., vision and audio) living in different mathematical spaces 26, 2. We thoroughly investigated CMM, provided practical resolution algorithms and studied their convergence properties. We developed several methods for sound localization using two or more microphones 1. The Gaussian locally-linear model (GLLiM) is a partially supervised mixture model that allows to map high-dimensional observations (audio, visual, or concatenations of audio-visual vectors) onto low-dimensional manifolds with a partially known structure 10. This model is particularly well suited for perception because it encodes both observable and unobservable phenomena. A variant of this model, namely probabilistic piecewise affine mapping has also been proposed and successfully applied to the problem of sound-source localization and separation 9. The European projects HUMAVIPS (2010-2013) coordinated by R. Horaud and EARS (2014-2017), applied audio-visual scene analysis to human-robot interaction.
3.2 Stereoscopic Vision
Stereoscopy is one of the most studied topics in biological and computer vision. Nevertheless, classical approaches of addressing this problem fail to integrate eye/camera vergence. From a geometric point of view, the integration of vergence is difficult because one has to re-estimate the epipolar geometry at every new eye/camera rotation. From an algorithmic point of view, it is not clear how to combine depth maps obtained with different eyes/cameras relative orientations. Therefore, we addressed the more general problem of binocular vision that combines the low-level eye/camera geometry, sensor rotations, and practical algorithms based on global optimization 21, 38. We studied the link between mathematical and computational approaches to stereo (global optimization and Markov random fields) and the brain plausibility of some of these approaches: indeed, we proposed an original mathematical model for the complex cells in visual-cortex areas V1 and V2 that is based on steering Gaussian filters and that admits simple solutions 18. This addresses the fundamental issue of how local image structure is represented in the brain/computer and how this structure is used for estimating a dense disparity field. Therefore, the main originality of our work is to address both computational and biological issues within a unifying model of binocular vision. Another equally important problem that still remains to be solved is how to integrate binocular depth maps over time. Recently, we have addressed this problem and proposed a semi-global optimization framework that starts with sparse yet reliable matches and proceeds with propagating them over both space and time. The concept of seed-match propagation has then been extended to TOF-stereo fusion 13.
3.3 Audio Signal Processing
Audio-visual fusion algorithms necessitate that the two modalities are represented in the same mathematical space. Binaural audition allows to extract sound-source localization (SSL) information from the acoustic signals recorded with two microphones. We have developed several methods, that perform sound localization in the temporal and the spectral domains. If a direct path is assumed, one can exploit the time difference of arrival (TDOA) between two microphones to recover the position of the sound source with respect to the position of the two microphones. The solution is not unique in this case, the sound source lies onto a 2D manifold. However, if one further assumes that the sound source lies in a horizontal plane, it is then possible to extract the azimuth. We used this approach to predict possible sound locations in order to estimate the direction of a speaker 2. We also developed a geometric formulation and we showed that with four non-coplanar microphones the azimuth and elevation of a single source can be estimated without ambiguity 1. We also investigated SSL in the spectral domain. This exploits the filtering effects of the head related transfer function (HRTF): there is a different HRTF for the left and right microphones. The interaural spectral features, namely the ILD (interaural level difference) and IPD (interaural phase difference) can be extracted from the short-time Fourier transforms of the two signals. The sound direction is encoded in these interaural features but it is not clear how to make SSL explicit in this case. We proposed a supervised learning formulation that estimates a mapping from interaural spectral features (ILD and IPD) to source directions using two different setups: audio-motor learning 9 and audio-visual learning 11.
We also addressed the difficult problem of audio signal processing in reverberant environments. We thoroughly studied the convolutive transfer function (CTF) and developed several methods and associated algorithms for the localization, separation and tracking of audio sources 34, 32, 33, 35, 31
3.4 Visual Reconstruction With Multiple Color and Depth Cameras
For the last decade, one of the most active topics in computer vision has been the visual reconstruction of objects, people, and complex scenes using a multiple-camera setup. The PERCEPTION team has pioneered this field and by 2006 several team members published seminal papers in the field. Recent work has concentrated onto the robustness of the 3D reconstructed data using probabilistic outlier rejection techniques combined with algebraic geometry principles and linear algebra solvers 41. Subsequently, we proposed to combine 3D representations of shape (meshes) with photometric data 40. The originality of this work was to represent photometric information as a scalar function over a discrete Riemannian manifold, thus generalizing image analysis to mesh and graph analysis. Manifold equivalents of local-structure detectors and descriptors were developed 39. The outcome of this pioneering work has been twofold: the formulation of a new research topic now addressed by several teams in the world, and allowed us to start a three year collaboration with Samsung Electronics. We developed the novel concept of mixed camera systems combining high-resolution color cameras with low-resolution depth cameras 22, 19,17. Together with our start-up company 4D Views Solutions and with Samsung, we developed the first practical depth-color multiple-camera multiple-PC system and the first algorithms to reconstruct high-quality 3D content 13.
3.5 Registration, Tracking and Recognition of People and Actions
The analysis of articulated shapes has challenged standard computer vision algorithms for a long time. There are two difficulties associated with this problem, namely how to represent articulated shapes and how to devise robust registration and tracking methods. We addressed both these difficulties and we proposed a novel kinematic representation that integrates concepts from robotics and from the geometry of vision. In 2008 we proposed a method that parameterizes the occluding contours of a shape with its intrinsic kinematic parameters, such that there is a direct mapping between observed image features and joint parameters 27. This deterministic model has been motivated by the use of 3D data gathered with multiple cameras. However, this method was not robust to various data flaws and could not achieve state-of-the-art results on standard dataset. Subsequently, we addressed the problem using probabilistic generative models. We formulated the problem of articulated-pose estimation as a maximum-likelihood with missing data and we devised several tractable algorithms 25, 24. We proposed several expectation-maximization procedures applied to various articulated shapes: human bodies, hands, etc. In parallel, we proposed to segment and register articulated shapes represented with graphs by embedding these graphs using the spectral properties of graph Laplacians 8. This turned out to be a very original approach that has been followed by many other researchers in computer vision and computer graphics.
4 Application domains
The research topics of Perception have strong social impact with great economic value. In more detail, Perception has put strong emphasis on the development of socially assistive robots SARs. In particular we apply social robot methodologies to gerontological healthcare. Several recently released demographic studies have emphasized that the number of very old people (over 80) will considerably increase, reaching 13% (66 million people) of the EU population by 2080. For the same period of time the working-age population will shrink, thus raising the critical issue of how the elderly will be taken care of in the coming century. This is a major concern for our civilisation that has been largely under-estimated. There is a risk that the elderly are being left behind the digitization of the society. Consequently, the development of robotic technologies addressing their needs and their expectations is mandatory. Team members will work hand-in-hand with healthcare professionals to apprehend the current limitations of SARs, to evaluate their potential, and to establish several use-cases.
The deployment of SARs, from academic laboratories to hospitals and to retirement homes, is far from being a trivial issue and it should not be done in isolation. The team is committed to work in collaboration with industrial partners, able to develop, commercialize, maintain and update SAR technologies. The Perception team has a great deal of collaborative experience with the robotics industry. In particular we have directly collaborated with Softbank Robotics Europe (formerly Aldebaran Robotics) and conducted several technology transfer actions. Currently, we work in collaboration with PAL Robotics (Spain), a leading R&D robot manufacturer, and with ERM Automatismes (France), an SME specialized in the development of service robots. These two companies are part of the EU project SPRING. It is worth to be mentioned that the Broca Hospital (Assistance Publique Hôpitaux de Paris) is a member of SPRING as well, and is highly committed to provide ethical guidance, recommendations for robot-technology acceptance by very old people, and in-depth experimental validation.
5 Highlights of the year
5.1 Awards
Xavier Alameda-Pineda and his collaborators from the University of Trento received the ACM-TOMM 2020 Nicolas D. Georganas Best Paper Award, that recognizes the most significant work in ACM Transactions on Multimedia Computing, Communications, and Applications (ACM TOMM) in a given calendar year: Increasing image memorability with neural style transfer, vol. 15 Issue 2, January 2019 by A. Siarohin, G. Zen, C. Majtanovic, X. Alameda-Pineda, E. Ricci, N. Sebe.
5.2 New Projects and Collaborations
5.2.1 Collaboration with Facebook Reality Research Lab, USA
In this project, we investigate visually assisted speech processing. In particular we plan to go beyond the current paradigm that systematically combines a noisy speech signal with clean lip images and which delivers a clean speech signal. The rationale of this paradigm is based on the fact that lip images are free of any type of noise. This hypothesis is merely verified in practice. Indeed, speech production is often accompanied by head motions that considerably modify the patterns of the observed lip movements. As a consequence, currently available audio-visual speech processing technologies are not usable in practice. In this project we develop a methodology that separates non-rigid face- and lip movements from rigid head movements, and we build a deep generative architecture that combines audio and visual features based on their relative merits, rather than making systematic recourse to their concatenation. The core methodology is based on robust mixture modeling and on variational auto-encoders, two methodologies that have been thoroughly investigated by the team.
5.2.2 H2020 Project SPRING
Started on Januray 1st, 2020 and finalising on May 31st, 2024, SPRING is a research and innovation action (RIA) with eight partners: Inria Grenoble (coordinator), Università degli Studi di Trento, Czech Technical University Prague, Heriot-Watt University Edinburgh, Bar-Ilan University Tel Aviv, ERM Automatismes Industriels Carpentras, PAL Robotics Barcelona, and Hôpital Broca Paris.. The main objective of SPRING (Socially Pertinent Robots in Gerontological Healthcare) is the development of socially assistive robots with the capacity of performing multimodal multiple-person interaction and open-domain dialogue. In more detail:
- The scientific objective of SPRING is to develop a novel paradigm and novel concept of socially-aware robots, and to conceive innovative methods and algorithms for computer vision, audio processing, sensor-based control, and spoken dialog systems based on modern statistical- and deep-learning to ground the required social robot skills.
- The technological objective of SPRING is to create and launch a brand new generation of robots that are flexible enough to adapt to the needs of the users, and not the other way around.
- The experimental objective of SPRING is twofold: to validate the technology based on HRI experiments in a gerontology hospital, and to assess its acceptability by patients and medical staff.
Website: https://
5.2.3 ANR JCJC Project ML3RI
Starting on March 1st 2020 and finalising on February 28th 2024, ML3RI is an ANR JCJC that has been awarded to Xavier Alameda-Pineda. Multi-person robot interaction in the wild (i.e. unconstrained and using only the robot's resources) is nowadays unachievable because of the lack of suitable machine perception and decision-taking models. Multi-Modal Multi-person Low-Level Learning models for Robot Interaction (ML3RI) has the ambition to develop the capacity to understand and react to low-level behavioral cues, which is crucial for autonomous robot communication. The main scientific impact of ML3RI is to develop new learning methods and algorithms, thus opening the door to study multi-party conversations with robots. In addition, the project supports open and reproducible research.
Website: https://
5.3 The Social Robot ARI
The team participated to the specifications of a social-robot prototype manufactured by PAL Robotics, an industrial partner of the SPRING project. ARI is a non-holonomic differential-drive wheeled robot equipped with a pan and tilt head, with both color and depth cameras and with a microphone array that embeds the latest audio signal processing technologies. The challenge is to devolop a software suite, from low-level control to high-level planning, such that the robot has a socially-aware behaviour while it safely navigates in an ever changing environment.
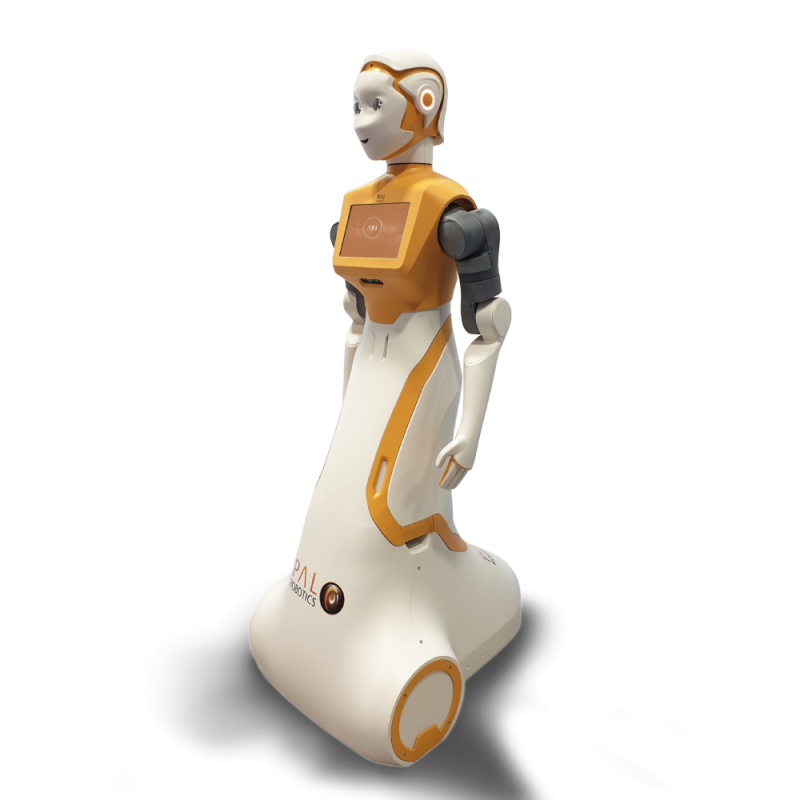
ARI is a robot prototype (not yet a commercially available product) designed and manufactured by PAL Robotics, located in Barcelona, and a member of the SPRING consortium.
6 New software and platforms
6.1 New software
6.1.1 deepMot
- Name: A Differentiable Framework for Training Multi-Object Trackers
- Keywords: Deep learning, Computer vision, Multi-Object Tracking
- Scientific Description: The recent trend in vision-based multi-object tracking (MOT) is heading towards leveraging the representational power of deep learning to jointly learn to detect and track objects. However, existing methods train only certain sub-modules using loss functions that often do not correlate with established tracking evaluation measures such as Multi-Object Tracking Accuracy (MOTA) and Precision (MOTP). As these measures are not differentiable, the choice of appropriate loss functions for end-to-end training of multi-object tracking methods is still an open research problem. We bridge this gap by proposing a differentiable proxy of MOTA and MOTP, which we combine in a loss function suitable for end-to-end training of deep multi-object trackers. As a key ingredient, we propose a Deep Hungarian Net (DHN) module that approximates the Hungarian matching algorithm. DHN allows to estimate the correspondence between object tracks and ground truth objects to compute differentiable proxies of MOTA and MOTP, which are in turn used to optimize deep trackers directly. We experimentally demonstrate that the proposed differentiable framework improves the performance of existing multi-object trackers, and we establish a new state-of-the-art on the MOTChallenge benchmark.
- Functional Description: We develop a differentiable proxy of MOTA and MOTP (Multi-Object Tracking Accuracy -MOTA and Precision-MOTP), which we combine in a loss function suitable for end-to-end training of deep multi-object trackers. As a key ingredient, we propose a Deep Hungarian Net (DHN) module that approximates the Hungarian matching algorithm. DHN allows to estimate the correspondence between object tracks and ground truth objects to compute differentiable proxies of MOTA and MOTP, which are in turn used to optimize deep trackers directly. We experimentally demonstrate that the proposed differentiable framework improves the performance of existing multi-object trackers, and we establish a new state-of-the-art on the MOTChallenge benchmark.
-
URL:
https://
team. inria. fr/ perception/ research/ deepmot/ - Publication: hal-02534894
- Contact: Xavier Alameda-pineda
- Participants: Yihong Xu, Xavier Alameda-pineda
- Partner: Technical University of Munich (TUM)
6.1.2 dvae-speech
- Name: dynamic variational auto-encoder for speech re-synthesis
- Keywords: Variational Autoencoder, Deep learning, Pytorch, Speech Synthesis
- Functional Description: It can be considered an library for speech community, to use different dynamic VAE models for speech re-synthesis (potentially for other speech application)
- Authors: Xiaoyu Bie, Xavier Alameda-pineda, Laurent Girin
- Contact: Xavier Alameda-pineda
6.1.3 AVSE-VAE
- Name: Audio-visual speech enhancement based on variational autoencoder speech modeling
- Keywords: Variational Autoencoder, Speech, Deep learning
- Scientific Description: Variational auto-encoders (VAEs) are deep generative latent variable models that can be used for learning the distribution of complex data. VAEs have been successfully used to learn a probabilistic prior over speech signals, which is then used to perform speech enhancement. One advantage of this generative approach is that it does not require pairs of clean and noisy speech signals at training. We propose audio-visual variants of VAEs for single-channel and speaker-independent speech enhancement. We develop a conditional VAE (CVAE) where the audio speech generative process is conditioned on visual information of the lip region. At test time, the audio-visual speech generative model is combined with a noise model based on nonnegative matrix factorization, and speech enhancement relies on a Monte Carlo expectation-maximization algorithm. Experiments are conducted with the recently published NTCD-TIMIT dataset. The results confirm that the proposed audio-visual CVAE effectively fuse audio and visual information, and it improves the speech enhancement performance compared with the audio-only VAE model, especially when the speech signal is highly corrupted by noise. We also show that the proposed unsupervised audio-visual speech enhancement approach outperforms a state-of-the-art supervised deep learning method.
- Functional Description: This library contains PyTorch implementations of the audio-visual speech enhancement methods based on variational autoencoder (VAE), presented in the following publications : - M. Sadeghi, S. Leglaive, X. Alameda-Pineda, L. Girin, and R. Horaud, “Audio-visual Speech Enhancement Using Conditional Variational Auto-Encoder,” August 2019. - M. Sadeghi and X. Alameda-Pineda, “Robust Unsupervised Audio-visual Speech Enhancement Using a Mixture of Variational Autoencoders,” in IEEE International Conference on Acoustics Speech and Signal Processing (ICASSP), Barcelona, Spain, May 2020. - M. Sadeghi and X. Alameda-Pineda, “Mixture of Inference Networks for VAE-based Audio-visual Speech Enhancement,” December 2019.
- Publications: hal-02364900, hal-02534911
- Contact: Xavier Alameda-pineda
- Participants: Mostafa Sadeghi, Xavier Alameda-pineda, Laurent Girin, Simon Leglaive, Radu Horaud
6.1.4 upa3dfa
- Name: Unsupervised Performance Analysis of 3D Face Alignment
- Keywords: Computer vision, Deep learning, 3D Face alignment, Pattern matching
- Scientific Description: We address the problem of analyzing the performance of 3D face alignment (3DFA) algorithms. Traditionally, performance analysis relies on carefully annotated datasets. Here, these annotations correspond to the 3D coordinates of a set of pre-defined facial landmarks. However, this annotation process, be it manual or automatic, is rarely error-free, which strongly biases the analysis. In contrast, we propose a fully unsupervised methodology based on robust statistics and a parametric confidence test. We revisit the problem of robust estimation of the rigid transformation between two point sets and we describe two algorithms, one based on a mixture between a Gaussian and a uniform distribution, and another one based on the generalized Student's t-distribution. We show that these methods are robust to up to 50% outliers, which makes them suitable for mapping a face, from an unknown pose to a frontal pose, in the presence of facial expressions and occlusions. Using these methods in conjunction with large datasets of face images, we build a statistical frontal facial model and an associated parametric confidence metric, eventually used for performance analysis. We empirically show that the proposed pipeline is neither method-biased nor data-biased, and that it can be used to assess both the performance of 3DFA algorithms and the accuracy of annotations of face datasets
- Functional Description: This library contains codes and data for unsupervised performance analysis of 3D face alignment algorithms explained in the following publication : "Sadeghi M, Guy S, Raison A, Alameda-Pineda X, Horaud R (2020) Unsupervised Performance Analysis of 3D Face Alignment. Submitted to International Journal of Computer Vision"
- Publication: hal-02543069
- Contact: Xavier Alameda-pineda
- Participants: Mostafa Sadeghi, Sylvain Guy, Xavier Alameda-pineda
6.1.5 CANUReID
- Name: CANU-ReID: A Conditional Adversarial Network for Unsupervised person Re-IDentification
- Keywords: Computer vision, Deep learning, Identification, Unsupervised learning
- Scientific Description: Unsupervised person re-ID is the task of identifying people on a target data set for which the ID labels are unavailable during training. In this paper, we propose to unify two trends in unsupervised person re-ID: clustering & fine-tuning and adversarial learning. On one side, clustering groups training images into pseudo-ID labels, and uses them to fine-tune the feature extractor. On the other side, adversarial learning is used, inspired by domain adaptation, to match distributions from different domains. Since target data is distributed across different camera viewpoints, we propose to model each camera as an independent domain, and aim to learn domain-independent features. Straightforward adversarial learning yields negative transfer, we thus introduce a conditioning vector to mitigate this undesirable effect. In our framework, the centroid of the cluster to which the visual sample belongs is used as conditioning vector of our conditional adversarial network, where the vector is permutation invariant (clusters ordering does not matter) and its size is independent of the number of clusters. To our knowledge, we are the first to propose the use of conditional adversarial networks for unsupervised person re-ID. We evaluate the proposed architecture on top of two state-of-the-art clustering-based unsupervised person re-identification (re-ID) methods on four different experimental settings with three different data sets and set the new state-of-the-art performance on all four of them.
- Functional Description: We propose to unify two trends in unsupervised person re-ID: clustering & fine-tuning and adversarial learning. On one side, clustering groups training images into pseudo-ID labels, and uses them to fine-tune the feature extractor. On the other side, adversarial learning is used, inspired by domain adaptation, to match distributions from different domains. Since target data is distributed across different camera viewpoints, we propose to model each camera as an independent domain, and aim to learn domain-independent features. Straightforward adversarial learning yields negative transfer, we thus introduce a conditioning vector to mitigate this undesirable effect. In our framework, the centroid of the cluster to which the visual sample belongs is used as conditioning vector of our conditional adversarial network, where the vector is permutation invariant (clusters ordering does not matter) and its size is independent of the number of clusters. To our knowledge, we are the first to propose the use of conditional adversarial networks for unsupervised person re-ID. We evaluate the proposed architecture on top of two state-of-the-art clustering-based unsupervised person re-identification (re-ID) methods on four different experimental settings with three different data sets and set the new state-of-the-art performance on all four of them.
- Contact: Xavier Alameda-pineda
- Participants: Guillaume Delorme, Yihong Xu, Xavier Alameda-pineda
7 New results
7.1 Speech Denoising and Enhancement with LTSMs
We address the problems of single- and multichannel speech denoising 36 and enhancement 63, 53, 51 in the short-time Fourier transform (STFT) domain and in the framework of sequence-to-sequence deep learning. In the case of denoising, the magnitude of noisy speech is mapped onto the noise power spectral density. In the case of speech enhancement, the noisy speech is mapped onto clean speech. A long short-time memory (LSTM) network takes as input a sequence of STFT coefficients associated with a frequency bin of multichannel noisy-speech signals. The network's output is a sequence of single-channel clean speech at the same frequency bin. We propose several clean-speech network targets, namely, the magnitude ratio mask, the complex ideal ratio mask, the STFT coefficients and spatial filtering 63. A prominent feature of the proposed model is that the same LSTM architecture, with identical parameters, is trained across frequency bins. The proposed method is referred to as narrow-band deep filtering. This choice stays in contrast with traditional wide-band speech enhancement methods. The proposed deep filter is able to discriminate between speech and noise by exploiting their different temporal and spatial characteristics: speech is non-stationary and spatially coherent while noise is relatively stationary and weakly correlated across channels. This is similar in spirit with unsupervised techniques, such as spectral subtraction and beamforming. We describe extensive experiments with both mixed signals (noise is added to clean speech) and real signals (live recordings). We empirically evaluate the proposed architecture variants using speech enhancement and speech recognition metrics, and we compare our results with the results obtained with several state of the art methods. In the light of these experiments we conclude that narrow-band deep filtering has very good performance, and excellent generalization capabilities in terms of speaker variability and noise type, e.g. Figure 3.
Website: https://
![]() |
![]() |
![]() |
Noisy speech input | Filtered output | Ground-truth speech signal |
7.2 Speech Enhancement with a Recurrent Variational Auto-Encoder
We investigate a generative approach to speech enhancement based on a recurrent variational autoencoder (RVAE). The deep generative speech model is trained using clean speech signals only, and it is combined with a nonnegative matrix factorization (NMF) noise model for speech enhancement. We propose a variational expectation-maximization algorithm where the encoder of the RVAE is fine-tuned at test time, to approximate the distribution of the latent variables given the noisy speech observations. Compared with previous approaches based on feed-forward fully-connected architectures, the proposed recurrent deep generative speech model induces a posterior temporal dynamic over the latent variables, which is shown to improve the speech enhancement results. 52.
Website: https://
7.3 Audio-visual Speech Enhancement with Conditional Variational Auto-Encoder
Variational auto-encoders (VAEs) are deep generative latent variable models that can be used for learning the distribution of complex data. VAEs have been successfully used to learn a probabilistic prior over speech signals, which is then used to perform speech enhancement. One advantage of this generative approach is that it does not require pairs of clean and noisy speech signals at training. In this work, we propose audio-visual variants of VAEs for single-channel and speaker-independent speech enhancement. We developed a conditional VAE (CVAE) where the audio speech generative process is conditioned on visual information of the lip region, e.g. Figure 4. At test time, the audio-visual speech generative model is combined with a noise model, based on nonnegative matrix factorization, and speech enhancement relies on a Monte Carlo expectation-maximization algorithm. Experiments were conducted with the recently published NTCD-TIMIT dataset. The results confirm that the proposed audio-visual CVAE effectively fuse audio and visual information, and it improves the speech enhancement performance compared with the audio-only VAE model, especially when the speech signal is highly corrupted by noise. We also showed that the proposed unsupervised audio-visual speech enhancement approach outperforms a state-of-the-art supervised deep learning method 46.
Website: https://
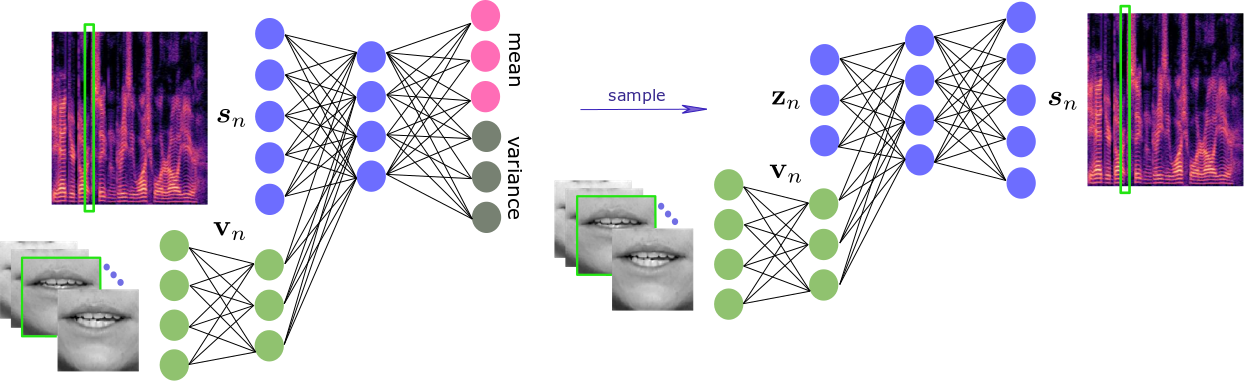
7.4 Mixture of Inference Networks for VAE-based Audio-visual Speech Enhancement
We are interested in unsupervised (unknown noise) audio-visual speech enhancement based on variational autoencoders (VAEs), where the probability distribution of clean speech spectra is simulated via an encoder-decoder architecture. The trained generative model (decoder) is then combined with a noise model at test time to estimate the clean speech. In the speech enhancement phase (test time), the initialization of the latent variables, which describe the generative process of clean speech via decoder, is crucial, as the overall inference problem is non-convex. This is usually done by using the output of the trained encoder where the noisy audio and clean video data are given as input. Current audio-visual models do not provide an effective initialization because the two modalities are tightly coupled (concatenated) in the associated architectures 46, Figure 4. To overcome this issue, we inspire from mixture models, and introduce the mixture of inference networks variational autoencoder (MIN-VAE), e.g. Figure 5. Two encoder networks input, respectively, audio and visual data, and the posterior of the latent variables is modeled as a mixture of two Gaussian distributions output from each encoder network. The mixture variable is also latent, and therefore the inference of learning the optimal balance between the audio and visual inference networks is unsupervised as well. By training a shared decoder, the overall network learns to adaptively fuse the two modalities. Moreover, at test time, the visual encoder, which takes (clean) visual data, is used for initialization. A variational inference approach is derived to train the proposed generative model. Thanks to the novel inference procedure and the robust initialization, the proposed audio-visual VAE exhibits superior performance on speech enhancement than using the standard audio-only as well as audio-visual counterparts 45, 57, 56.
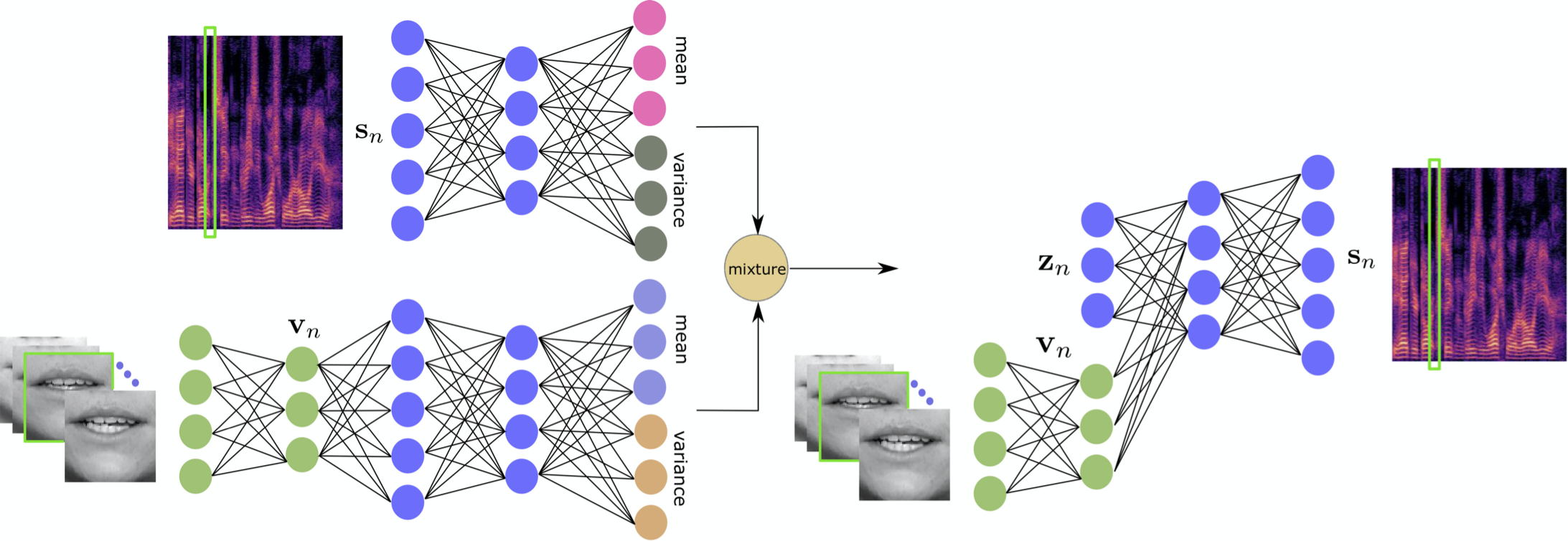
Architecture of the proposed mixture of inference networks VAE (MIN-VAE). A mixture of an audio- and a visual-based encoder is used to approximate the intractable posterior distribution of the latent variables.
7.5 A Comprehensive Analysis of Deep Regression
Deep learning revolutionized data science, and recently its popularity has grown exponentially, as did the amount of papers employing deep networks. Vision tasks, such as human pose estimation, did not escape from this trend. There is a large number of deep models, where small changes in the network architecture, or in the data pre-processing, together with the stochastic nature of the optimization procedures, produce notably different results, making extremely difficult to sift methods that significantly outperform others. This situation motivates the current study, in which we perform a systematic evaluation and statistical analysis of vanilla deep regression, i.e. convolutional neural networks with a linear regression top layer. This is the first comprehensive analysis of deep regression techniques. We perform experiments on four vision problems, and report confidence intervals for the median performance as well as the statistical significance of the results, if any. Surprisingly, the variability due to different data pre-processing procedures generally eclipses the variability due to modifications in the network architecture. Our results reinforce the hypothesis according to which, in general, a general-purpose network (e.g. VGG-16 or ResNet-50) adequately tuned can yield results close to the state-of-the-art without having to resort to more complex and ad-hoc regression models, 44.
Website: https://
7.6 Variational Inference and Learning of Piecewise-linear Dynamical Systems
Modeling the temporal behavior of data is of primordial importance in many scientific and engineering fields. Baseline methods assume that both the dynamic and observation equations follow linear-Gaussian models. However, there are many real-world processes that cannot be characterized by a single linear behavior. Alternatively, it is possible to consider a piecewise-linear model which, combined with a switching mechanism, is well suited when several modes of behavior are needed. Nevertheless, switching dynamical systems are intractable because their computational complexity increases exponentially with time. In this paper, we propose a variational approximation of piecewise linear dynamical systems. We provide full details of the derivation of two variational expectation-maximization algorithms, a filter and a smoother. We show that the model parameters can be split into two sets, static and dynamic parameters, and that the former parameters can be estimated off-line together with the number of linear modes, or the number of states of the switching variable. We apply the proposed method to the head-pose tracking, e.g. Figure 6 , and we thoroughly compare our algorithms with several state of the art trackers, 42.
Website: https://
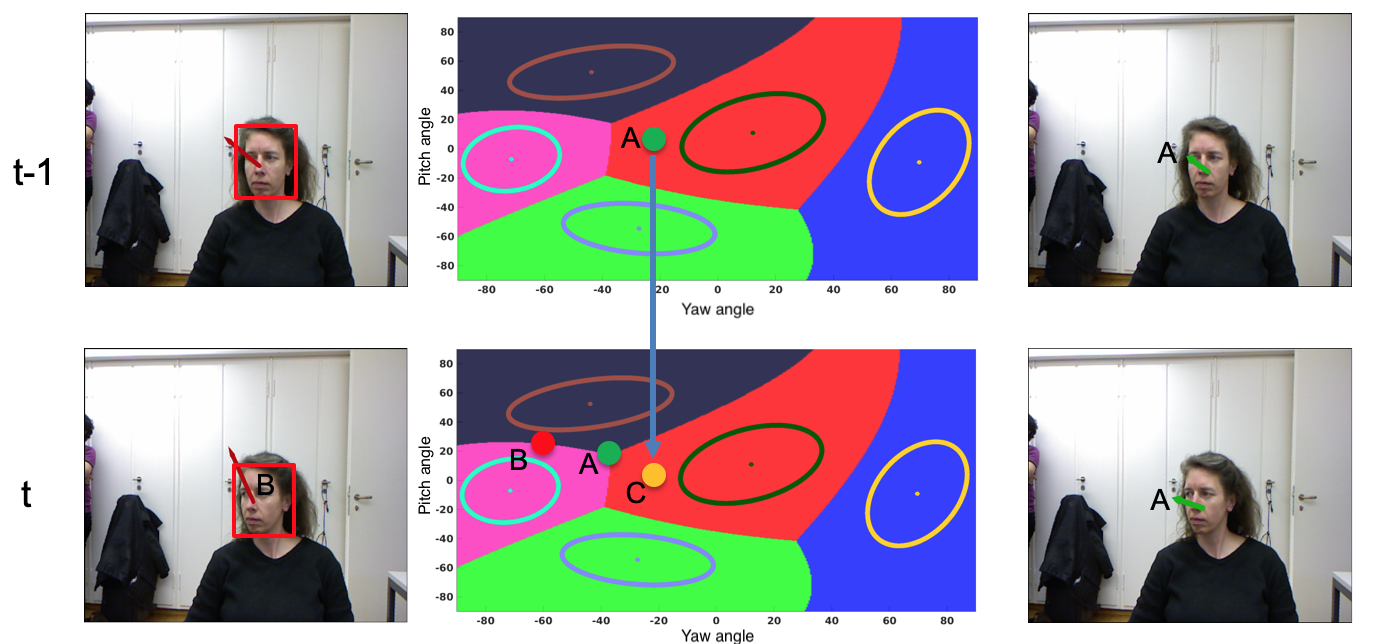
The proposed variational piecewise linear dynamical system (P-LDS) algorithm applied to the problem of head pose tracking (HPT). The central column shows the Gaussian mixture that models the latent space. The parameters of this mixture don't vary over time and they are learnt from a training set of input-output instances of the observed and latent variables. In this example we show the likelihood function associated with the latent variables of head pose, namely the yaw and pitch angles. The observed pose at (red dot denoted B) is estimated from a high-dimensional feature vector that describes a face (left column). The variational means (green dots denoted A and shown with green arrows onto the right column) are inferred by the E-X step of the algorithm based on the current dynamic prediction (orange dot denoted C) and the current observation (red dot denoted B).
7.7 Visual Voice Activity Detection
Visual voice activity detection (V-VAD) uses visual features to predict whether a person is speaking or not. V-VAD is useful whenever audio VAD (A-VAD) is inefficient either because the acoustic signal is difficult to analyze or because it is simply missing. We propose two deep architectures for V-VAD, one based on facial landmarks and one based on optical flow, Figure 7. Moreover, available datasets, used for learning and for testing V-VAD, lack content variability. We introduce a novel methodology to automatically create and annotate very large datasets in-the-wild – WildVVAD – based on combining A-VAD with face detection and tracking. A thorough empirical evaluation shows the advantage of training the proposed deep V-VAD models with this dataset, 50.
Website: https://
[Land-LSTM] ![]() |
![]() |
Architectures of the two proposed V-VAD models, based on facial landmarks (Land-LSTM) and based on optical flow (OF-ConvNet). Both networks take as input a sequence of frames and predict as output an activity label, speaking or silent.
7.8 Deep Multi-Object tracking
The recent trend in vision-based multi-object tracking (MOT) is heading towards leveraging the representational power of deep learning to jointly learn to detect and track objects. However, existing methods train only certain sub-modules using loss functions that often do not correlate with established tracking evaluation measures such as Multi-Object Tracking Accuracy (MOTA) and Precision (MOTP). As these measures are not differentiable, the choice of appropriate loss functions for end-to-end training of multi-object tracking methods is still an open research problem. In this paper, we bridge this gap by proposing a differentiable proxy of MOTA and MOTP, which we combine in a loss function suitable for end-to-end training of deep multi-object trackers. As a key ingredient, we propose a Deep Hungarian Net (DHN) module that approximates the Hungarian matching algorithm, Figure 8. DHN allows estimating the correspondence between object tracks and ground truth objects to compute differentiable proxies of MOTA and MOTP, which are in turn used to optimize deep trackers directly. We experimentally demonstrate that the proposed differentiable framework improves the performance of existing multi-object trackers, and we establish a new state of the art on the MOTChallenge benchmark, 58.
Website: https://
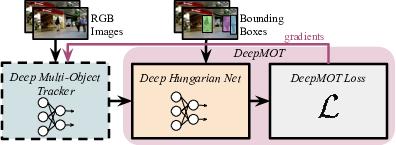
7.9 Multi-Person Monocular 3D Pose Estimation
Recent literature addressed the monocular 3D pose estimation task very satisfactorily. In these studies, different persons are usually treated as independent pose instances to estimate. However, in many every-day situations, people are interacting, and the pose of an individual depends on the pose of his/her interactees. In this paper, we investigate how to exploit this dependency to enhance current – and possibly future – deep networks for 3D monocular pose estimation. Our pose interacting network, or PI-Net, Figure 9, inputs the initial pose estimates of a variable number of interacting persons into a recurrent architecture used to refine the pose of the person-of-interest. Evaluating such a method is challenging due to the limited availability of public annotated multi-person 3D human pose datasets. We demonstrate the effectiveness of our method in the MuPoTS dataset, setting the new state-of-the-art on it. Qualitative results on other multi-person datasets (for which 3D pose ground-truth is not available) showcase the proposed PI-Net, 49.
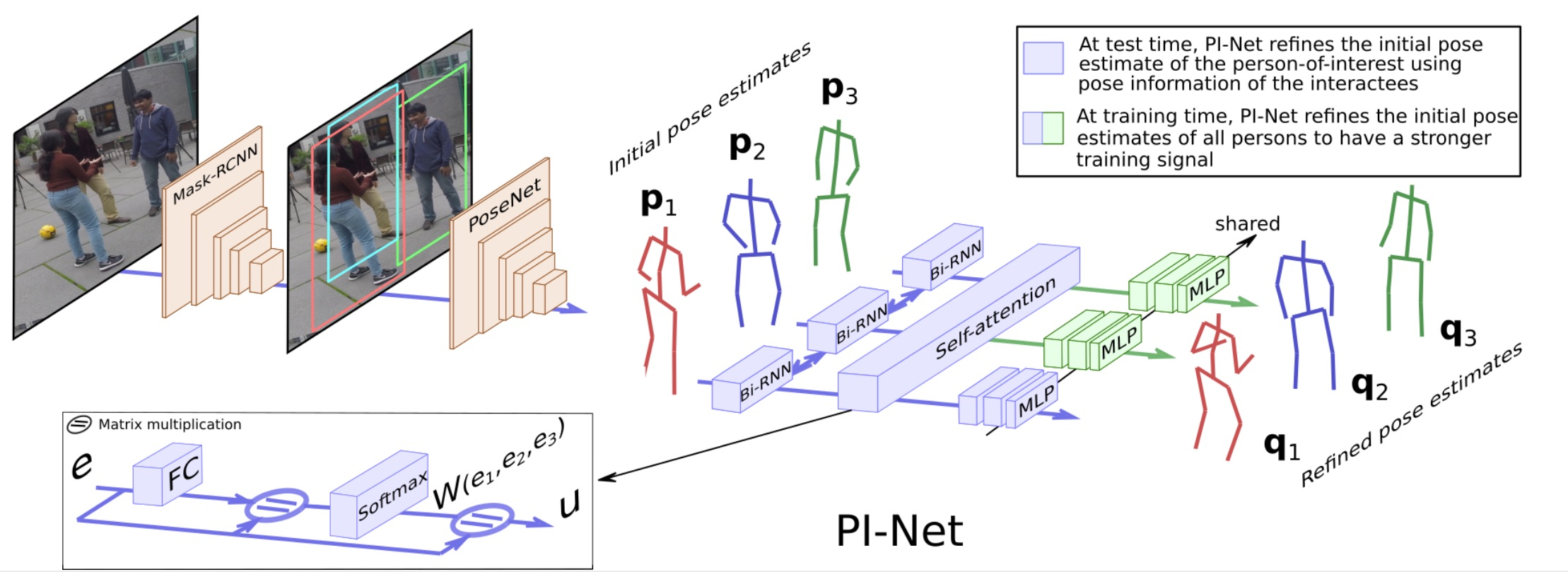
7.10 Expression-preserving face frontalization
Face frontalization consists of synthesizing a frontally-viewed face from an arbitrarily-viewed one. The main contribution of this paper is a robust frontalization method that preserves non-rigid facial deformations in order to boost the performance of expression analysis from videos of faces, e.g. lip reading. The method iteratively estimates the rigid transformation (scale, rotation, and translation) and the non-rigid deformation between 3D landmarks extracted from an arbitrarily-viewed face, and 3D vertices parameterized by a deformable shape model. An important merit of the method is its ability to deal with non-Gaussian errors in the data. For that purpose, we use the generalized Student-t distribution. The associated EM algorithm estimates a set of weights assigned to the observed landmarks, the higher the weight the more important the landmark, thus favoring landmarks that are only affected by rigid head movements. We propose to use the zero-mean normalized cross-correlation (ZNCC) score to evaluate the ability to preserve facial expressions. Moreover, we show that the method, when incorporated into a lip reading pipeline, considerably improves the word recognition score on an in-the-wild benchmark, 62.
https://
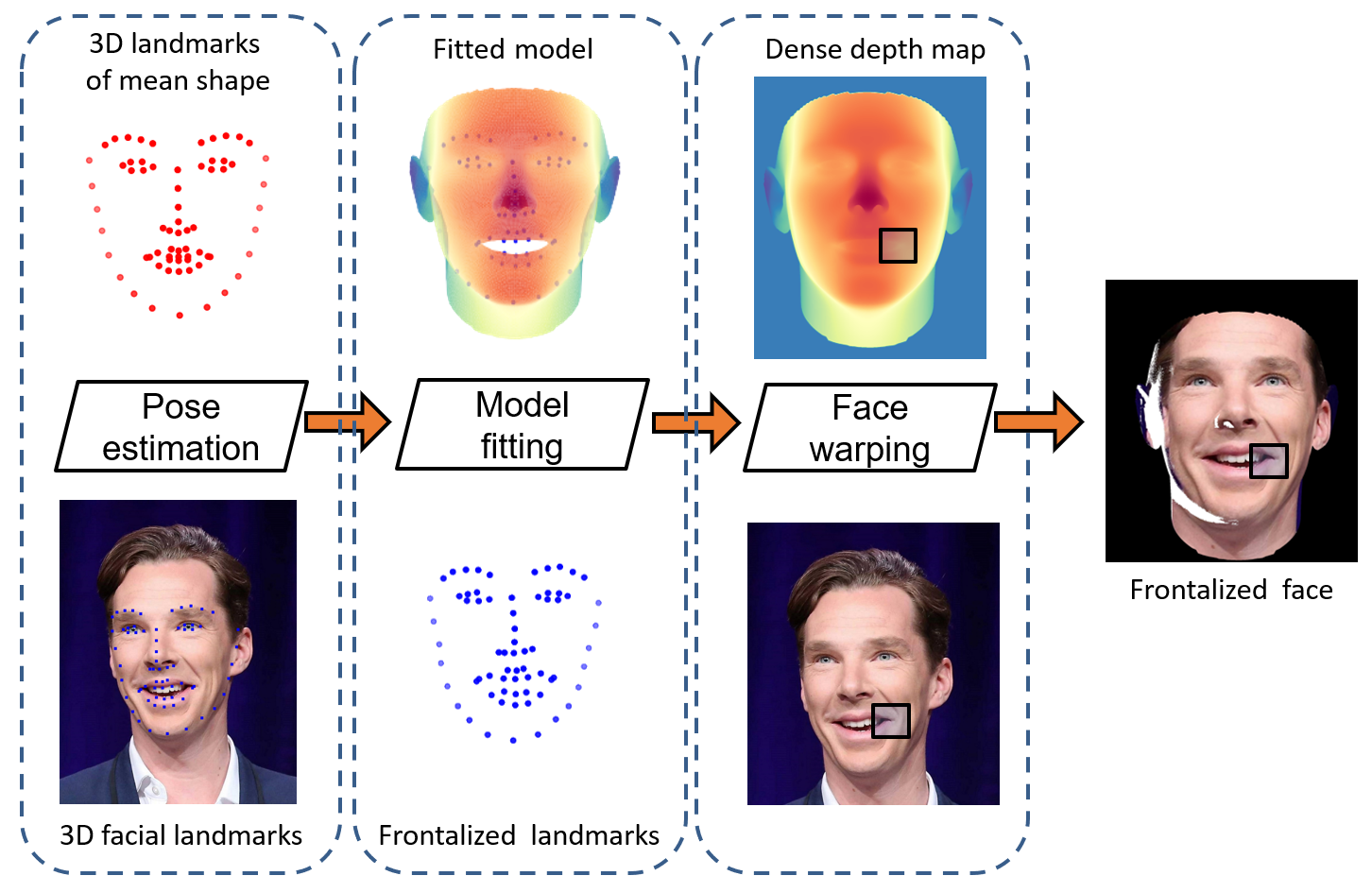
Overview of the proposed method. 3D landmarks extracted from a face (bottom-left) are aligned with 3D vertices associated with a frontal model (top-left). This deformable model is fitted to the frontalized landmarks (bottom-middle), yielding a deformed model aligned with the landmarks (top-middle). A dense depth map is computed by interpolating the 3D vertices of the triangulated mesh of the deformed model (top-right). This depth map is combined with the input face which is warped onto the frontal view (bottom-right).
7.11 Conditional Adversarial Network for Person Re-id
Unsupervised person re-ID is the task of identifying people on a target data set for which the ID labels are unavailable during training. In this paper, we propose to unify two trends in unsupervised person re-ID: clustering & fine-tuning and adversarial learning. On one side, clustering groups training images into pseudo-ID labels, and uses them to fine-tune the feature extractor. On the other side, adversarial learning is used, inspired by domain adaptation, to match distributions from different domains. Since target data is distributed across different camera viewpoints, we propose to model each camera as an independent domain, and aim to learn domain-independent features. Straightforward adversarial learning yields negative transfer, we thus introduce a conditioning vector to mitigate this undesirable effect. In our framework, the centroid of the cluster to which the visual sample belongs is used as conditioning vector of our conditional adversarial network, where the vector is permutation invariant (clusters ordering does not matter) and its size is independent of the number of clusters. To our knowledge, we are the first to propose the use of conditional adversarial networks for unsupervised person re-ID. We evaluate the proposed architecture on top of two state-of-the-art clustering-based unsupervised person re-identification (re-ID) methods on four different experimental settings with three different data sets and set the new state-of-the-art performance on all four of them, 48.
Website: https://
7.12 Online Deep Appearance Network for Identity-Consistent Multi-Person Tracking
The analysis of effective states through time in multi-person scenarii is very challenging, because it requires to consistently track all persons over time. This requires a robust visual appearance model capable of re-identifying people already tracked in the past, as well as spotting newcomers. In real-world applications, the appearance of the persons to be tracked is unknown in advance, and therefore on must devise methods that are both discriminative and flexible. Previous work in the literature proposed different tracking methods with fixed appearance models. These models allowed, up to a certain extent, to discriminate between appearance samples of two different people. We propose an online deep appearance network (ODANet), a method able to simultaneously track people and update the appearance model with the newly gathered annotation-less images. Since this task is specially relevant for autonomous systems, we also describe a platform-independent robotic implementation of ODANet. Our experiments show the superiority of the proposed method with respect to the state of the art, and demonstrate the ability of ODANet to adapt to sudden changes in appearance, to integrate new appearances in the tracking system and to provide more identity-consistent tracks 47.
7.13 Socially Aware Robot Navigation
While deep learning has signifantly advanced the state-of-the art in a number of domains, it has also been successful to solve more complex tasks that involve online decision taking and control, e.g. end-to-end learning of self-driving cars. This raises the question of applying such end-to-end frameworks for robot navigation taking into account social constraints. However, compared to self-driving cars, socially-aware navigation does not have such well defined tasks, and the behavior of the other entities (people in our case) present in the scene follows much more complex patterns than in the self-driving car scenarios. Furthermore, data on social interactions is hard to acquire, both for ethical and for practical reasons. Therefore we turn our attention towards more tranditional robot control techniques to address this problem. More precisely, we exploit the well established framework of Model Predictive Control (MPC), and combine it with a social cost map to take into account conversational groups (called F-formations). For example, a group organized in an F-formation shares a private space, called the o-space, reserved to the group that should not be occupied (by a social robot). Another example of social constraints would be to respect privacy spaces of people the robot is interacting with and depending on the level of interaction. These constraints should be integrated in the control of the robot, for example in the form of a velocity map or a social cost map. Our first simulated experiments demonstrate the capabilities of the proposed MPC-based framework to address a variety of generic scenarios (joining a group, guiding or following a person) meaningful for social robotics in general.
7.14 Meta Reinforcement Learning for Robust Action Policies
We have also started investigating the use of deep reinforcement learning for socially acceptable robot action policies. A problematic point of deep reinforcement learning is the amount of data that is required to learn appropriate policies. The agent needs to explore a lot of the state space and to observe the outcomes of different actions to identify the best action per state. In the context of robotics, this learning process takes a long time. Furthermore, the learned behavior depends on the reward function that has to be defined by the user. However, it is often not foreseeable what behavior will result from a reward function. For example, in a navigation task where the robot has to approach a human, the reward function could have a component which punishes strong movements. This should ensure that the robot is not learning a policy that behaves erratic or approaches a human with a too high velocity. How strong this component influences the whole reward function can lead to vastly different behaviors. If the punishment is too small, then the robot might approach a human too fast and is perceived as threatening. If the punishment is too large, then the robot might approach too slow or does not move around obstacles to reach the human. Often, the reward function needs to be adapted to learn an appropriate behavior. For classical reinforcement learning the task would have to be learned from scratch for each new reward function costing a lot of time. As a solution to these problems the SPRING project will utilize transfer learning and meta learning techniques. Our first series of experiments demonstrate the interest of exploiting meta reinforcement learning strategies when combining tasks such as facing the prominent speaker, looking at the people involved in the social interaction and limiting the robot movements (to have a more natural beahvior).
The studies on socially aware robot navigation and meta-reinforcement learning must be confirmed beyond simulation, via a real robotic platform in a real multi-person environment. The pandemic put these experiments on hold, and we are looking forward to evaluating our developments with the physical robotic platform which will soon be available in the team's laboratory, Figure 2.
8 Bilateral contracts and grants with industry
8.1 Bilateral contracts with industry
8.1.1 VASP
- Title: Visually-assisted speech processing
- Duration: 1 October 2020 - 30 September 2021
- Principal investigator: Radu Horaud
- Partner: Facebook Reality Labs Research, Redmond WA, USA
- Summary: We investigate audio-visual speech processing. In particular we plan to go beyond the current paradigm that systematically combines a noisy speech signal with clean lip images and which delivers a clean speech signal. The rationale of this paradigm is based on the fact that lip images are free of any type of noise. This hypothesis is merely verified in practice. Indeed, speech production is often accompanied by head motions that considerably modify the patterns of the observed lip movements. As a consequence, currently available audio-visual speech processing technologies are not usable in practice. In this project we develop a methodology that separates non-rigid face- and lip movements from rigid head movements, and we build a deep generative architecture that combines audio and visual features based on their relative merits, rather than making systematic recourse to their concatenation. It is also planned to record and annotate an audio-visual dataset that contains realistic face-to-face and multiparty conversations. The core methodology is based on robust mixture modeling and on variational auto-encoders.
9 Partnerships and cooperations
9.1 European initiatives
9.1.1 FP7 & H2020 Projects
SPRING
- Title: Socially Pertinent Robots in Gerontological Healthcare
- Duration: 1 January 2020 - 31 December 2023
- Coordinator: Xavier Alameda-Pineda, Inria
-
Partners:
- BAR ILAN UNIVERSITY (Israel)
- CESKE VYSOKE UCENI TECHNICKE V PRAZE (Czech Republic)
- ERM AUTOMATISMES INDUSTRIELS (France)
- HERIOT-WATT UNIVERSITY ((missing:COUNTRY))
- PAL ROBOTICS SL (Spain)
- UNIVERSITA DEGLI STUDI DI TRENTO (Italy)
- Inria contact: Xavier Alameda-Pineda
- Summary: SPRING is an EU H2020-ICT research and innovation action (RIA) whose main objective is the development of socially assistive robots with the capacity of performing multimodal multiple-person interaction and open-domain dialogue. SPRING explores new methods at the crossroads of machine learning, computer vision, audio signal processing, spoken dialog and robotics for enhancing the interaction and communication capabilities of companion robots. The paramount application of SPRING is the use of robots in gerontological healthcare.
9.2 National initiatives
9.2.1 ANR JCJC MLRI
- Title: Multi-modal multi-person low-level learning for robot interactions
- Duration: 1 March 2020 - 29 February 2024
- Principal investigator: Xavier Alameda-Pineda, Inria
- Summary: Robots with autonomous communication capabilities interacting with multiple persons at the same time in the wild are both a societal mirage and a scientific Ithaca. Indeed, despite the presence of various companion robots on the market, their social skills are derived from machine learning techniques functioning mostly under laboratory conditions. Moreover, current robotic platforms operate in confined environments, where on one side, qualified personnel received detailed instructions on how to interact with the robot as part of their technical training, and on the other side, external sensors and actuators may be available to ease the interaction between the robot and the environment. Trespassing these two constraints would allow a robotic platform to freely interact with multiple humans in a wide variety of every-day situations, e.g. as an office assistant, a health-care helper, a janitor or a waiter/waitress. To our understanding, interacting in the wild means that the environment is natural (unscripted conversation, regular lighting and acoustic conditions, people freely moving, etc.) and the robot is self-sufficient (uses only its sensing, acting and computing resources).
9.2.2 Multidisciplinary Institute of Artificial Intelligence (MIAI)
- Title: MIAI chair: Audio-visual machine perception and interaction for companion robots
- Duration: 1 October 2019 - 30 September 2023
- Principal investigators: Xavier Alameda-Pined and Radu Horaud, Inria
-
Participants:
- Florence Forbes, Inria
- Jean-Charles Quinton, UGA
- Laurent Girin, Grenoble INP
- Summary: We are particularly interested in the development of a robot able to achieve such tasks as exploring a populated space, understanding human behavior, and engaging multimodal dialog with one or several users. These tasks require audio and visual features (e.g. clean speech, prosody, eye-gaze, head-gaze, facial expressions, lip movements, head movements, and hand gestures) to be robustly retrieved from the raw sensor data. These features cannot be reliably extracted with a static robot that listens, looks and communicates with people from a distance, because of acoustic noise and reverberation, overlapping audio sources, bad lighting, limited image resolution, limited camera field of view, non-frontal views of people, visual occlusions, etc. Audio and visual perception and communication must therefore be performed actively: given a particular task, such as face-to-face dialog, the robot should be able to learn how to collect clean data (e.g. frontal videos of faces and audio signals with high speech-to-noise ratios) and how to react appropriately to human verbal and non-verbal solicitations (e.g. taking speech turns in a multi-party conversation). We plan to achieve a fine coupling between scientific findings and technological developments and to demonstrate this with a companion robot that assists and entertains the elderly in healthcare facilities.
9.2.3 ANR project MUDialbot
- Title: MUlti-party perceptually-active situated DIALog for human-roBOT interaction
- Duration:
- Coordinator: Fabrice Lefevre, Avignon Unibersity
-
Partners:
- Avignon University
- Inria
- Hubert Curien laboratory
- Broca Hospital
- Inria contact: Radu Horaud
- Summary: The overall goal is to actively incorporate human-behavior cues in spoken human-robot communication. We intend to reach a new level in the exploitation of the rich information available with audio and visual data flowing from humans when interacting with robots. In particular, extracting highly informative verbal and non-verbal perceptive features will enhance the robot's decision-making ability such that it can take speech turns more naturally and switch between multi-party/group interactions and face-to-face dialogues where required. Recently there has been an increasing interest in companion robots that are able to assist people in their everyday life and to communicate with them. These robots are perceived as social entities and their utility for healthcare and psychological well being for the elderly has been acknowledged by several recent studies. Patients, their families and medical professionals appreciate the potential of robots, provided that several technological barriers would be overcome in the near future, most notably the ability to move, see and hear in order to naturally communicate with people, well beyond touch screens and voice commands. The scientific and technological results of the project will be implemented onto a commercially available social robot and they will be tested and validated with several use cases in a day-care hospital unit. Large-scale data collection will complement in-situ tests to fuel further researches.
9.2.4 IDEX-UGA PIMPE
Physical complex Interactions and Multi-person Pose Estimation (PIMPE) is an International Strategic Partnerships (ISP) three-year project between our team and Universitat Politècnica de Catalunya (UPC). The scientific challenges of PIMPE are the followings: (i) Modeling multi-person interactions in full-body pose estimation, (ii) Estimating human poses in complex multi-person physical interactions, and (iii) Generating controlled and realistic multi-person complex pose images.
Participants: Xavier Alameda-Pineda (PI), Francesc Moreno-Noguer (UPC, Co-PI).
9.2.5 IDEX-UGA MIDGen
Multimodal Interaction Data Generation (MIDGen) is an Initiatives de Recherche Stratégiques (IRS) three-year project between our team and Pervasive Interaction team. The scientific challenges of MIDGen is the development of multimodal perception algorithms capable of understanding social signals emitted by humans with a high degree of precision.
Participants: Dominique Vaufreydaz (PI, UGA/LIG), Xavier Alameda-Pineda (co-PI).
10 Dissemination
10.1 Promoting scientific activities
10.1.1 Scientific events: selection
Xavier Alameda-Pineda was the main co-organiser of the Fairness Accountability Transparency and Ethics in Multimedia workshop, co-located with ACM International Conference on Multimedia 2020.
Member of the conference program committees
Xavier Alameda-Pineda was Area Chair for the following conferences:
- ACM International Conference on Multimedia 2020
- IAPR International Conference on Pattern Recognition 2020
- IEEE Winter Conference on Applications of Computer Vision 2021
10.1.2 Journal
Member of the editorial boards
Xavier Alameda-Pineda is Associated Editor of the ACM Transactions on Multimedia Tools and Applications.
Reviewer - reviewing activities
Xavier Alameda-Pineda reviewed for IEEE Transactions on Pattern Analysis and Machine Intelligence, IEEE Transactions on Audio, Language and Signal Processing and for IEEE Transactions on Multimedia.
10.1.3 Invited talks
Xavier Alameda-Pineda was invited to give the following talks:
- Towards audio-visual speech enhancement in robotic platforms (Dec'20) at Journée “perception et interaction homme-robot” du Groupe de Travail GT5 Interactions Personnes / Systèmes Robotiques du GDR Robotique
- Audio-visual variational speech enhancement (Sep'20) at Intelligent Sensing Summer School
- Choosing wisely your deep training loss (March'20) at Universidade NOVA de Lisboa
- Artificial Intelligence for Social Robots in Gerontological Healthcare (March'20) at European Robotics Forum
10.1.4 Teaching
- Master : Xavier Alameda-Pineda, Fundamentals of Probabilistic Data Mining, 19.5h, M2, UGA, France.
- Master : Xavier Alameda-Pineda, Machine Learning for Computer Vision and Audio Processing, 12h, M2, UGA, France.
10.1.5 Supervision
- PhD in progress: Guillaume Delorme, Deep Person Re-identification, October 2017, Xavier Alameda-Pineda and Radu Horaud,
- PhD in progress: Yihong Xu, Deep Multiple-person Tracking, October 2018, Xavier Alameda-Pineda and Radu Horaud,
- PhD in progress: Wen Guo, Deep Human Pose, October 2019, Xavier Alameda-Pineda and Radu Horaud,
- PhD in progress: Anand Ballou, Deep Reinforcement Learning for Robot Control, November 2019, Xavier Alameda-Pineda and Radu Horaud,
- PhD in progress: Louis Airale, Data Generation for Deep Multimodal Interaction Algorithms, October 2019, Xavier Alameda-Pineda and Dominique Vaufreydaz,
- PhD in progress: Xiaoyu Bie, Deep Generative Methods for Audio and Vision, December 2019, Xavier Alameda-Pineda and Laurent Girin.
- PhD in progress: Gaetan Lepage, Deep Reinforcement Learning for Robot Perception Enhancement, October 2020, Xavier Alameda-Pineda and Laurent Girin.
- PhD in progress: Xiaoyu Lin, Deep Generative Methods for Multi-Person Multi-Modal Tracking, November 2020, Xavier Alameda-Pineda and Laurent Girin.
10.1.6 Juries
Xavier Alameda-Pineda participated to the following PhD Juries as “rapporteur”:
- Daniel Michelsanti, University of Aalborg. Supervisors: Zheng-Hua Tan and Jesper Jensen.
11 Scientific production
11.1 Major publications
- 1 articleA Geometric Approach to Sound Source Localization from Time-Delay EstimatesIEEE Transactions on Audio, Speech and Language Processing226June 2014, 1082--1095
- 2 articleVision-Guided Robot HearingInternational Journal of Robotics Research344--5April 2015, 437--456
- 3 book Multimodal behavior analysis in the wild: Advances and challenges Academic Press (Elsevier) December 2018
- 4 articleVisual Servoing from LinesInternational Journal of Robotics Research2182002, 679--700URL: http://hal.inria.fr/hal-00520167
- 5 articleAn On-line Variational Bayesian Model for Multi-Person Tracking from Cluttered ScenesComputer Vision and Image Understanding153December 2016, 64--76
- 6 inproceedings Tracking a Varying Number of People with a Visually-Controlled Robotic Head IEEE/RSJ International Conference on Intelligent Robots and Systems Vancouver, Canada September 2017
- 7 articleTracking Multiple Audio Sources with the Von Mises Distribution and Variational EMIEEE Signal Processing Letters266June 2019, 798 - 802
- 8 articleRobust Temporally Coherent Laplacian Protrusion Segmentation of 3D Articulated BodiesInternational Journal of Computer Vision1121March 2015, 43--70
- 9 articleAcoustic Space Learning for Sound-Source Separation and Localization on Binaural ManifoldsInternational Journal of Neural Systems251February 2015, 21
- 10 articleHigh-Dimensional Regression with Gaussian Mixtures and Partially-Latent Response VariablesStatistics and Computing255September 2015, 893--911
- 11 articleCo-Localization of Audio Sources in Images Using Binaural Features and Locally-Linear RegressionIEEE Transactions on Audio, Speech and Language Processing234April 2015, 718--731
- 12 articleRobust Head-Pose Estimation Based on Partially-Latent Mixture of Linear RegressionsIEEE Transactions on Image Processing263March 2017, 1428--1440
- 13 articleFusion of Range and Stereo Data for High-Resolution Scene-ModelingIEEE Transactions on Pattern Analysis and Machine Intelligence3711November 2015, 2178--2192
- 14 articleJoint Alignment of Multiple Point Sets with Batch and Incremental Expectation-MaximizationIEEE Transactions on Pattern Analysis and Machine Intelligence406https://arxiv.org/abs/1609.01466June 2018, 1397--1410
- 15 articleEM Algorithms for Weighted-Data Clustering with Application to Audio-Visual Scene AnalysisIEEE Transactions on Pattern Analysis and Machine Intelligence3812December 2016, 2402--2415
- 16 articleAudio-Visual Speaker Diarization Based on Spatiotemporal Bayesian FusionIEEE Transactions on Pattern Analysis and Machine Intelligence405https://arxiv.org/abs/1603.09725July 2018, 1086--1099
- 17 articleCross-Calibration of Time-of-flight and Colour CamerasComputer Vision and Image Understanding134April 2015, 105--115
- 18 articleA Differential Model of the Complex CellNeural Computation239September 2011, 2324--2357URL: http://hal.inria.fr/inria-00590266
- 19 articleAutomatic Detection of Calibration Grids in Time-of-Flight ImagesComputer Vision and Image Understanding121April 2014, 108--118
- 20 articleCyclopean geometry of binocular visionJournal of the Optical Society of America A259September 2008, 2357--2369URL: http://hal.inria.fr/inria-00435548
- 21 articleCyclorotation Models for Eyes and CamerasIEEE Transactions on Systems, Man, and Cybernetics, Part B: Cybernetics401March 2010, 151--161URL: http://hal.inria.fr/inria-00435549
- 22 bookTime of Flight Cameras: Principles, Methods, and ApplicationsSpringer Briefs in Computer ScienceSpringerOctober 2012, 95URL: http://hal.inria.fr/hal-00725654
- 23 articleStereo Calibration from Rigid MotionsIEEE Transactions on Pattern Analysis and Machine Intelligence2212December 2000, 1446--1452URL: http://hal.inria.fr/inria-00590127
- 24 articleRigid and Articulated Point Registration with Expectation Conditional MaximizationIEEE Transactions on Pattern Analysis and Machine Intelligence333March 2011, 587--602URL: http://hal.inria.fr/inria-00590265
- 25 articleHuman Motion Tracking by Registering an Articulated Surface to 3-D Points and NormalsIEEE Transactions on Pattern Analysis and Machine Intelligence311January 2009, 158--163URL: http://hal.inria.fr/inria-00446898
- 26 articleConjugate Mixture Models for Clustering Multimodal DataNeural Computation232February 2011, 517--557URL: http://hal.inria.fr/inria-00590267
- 27 articleHuman Motion Tracking with a Kinematic Parameterization of Extremal ContoursInternational Journal of Computer Vision793September 2008, 247--269URL: http://hal.inria.fr/inria-00590247
- 28 articleA Variational EM Algorithm for the Separation of Time-Varying Convolutive Audio MixturesIEEE/ACM Transactions on Audio, Speech and Language Processing248August 2016, 1408--1423
- 29 articleNeural Network Based Reinforcement Learning for Audio-Visual Gaze Control in Human-Robot InteractionPattern Recognition Letters118February 2019, 61-71
- 30 articleOnline Localization and Tracking of Multiple Moving Speakers in Reverberant EnvironmentsIEEE Journal of Selected Topics in Signal Processing131March 2019, 88-103
- 31 inproceedingsReverberant Sound Localization with a Robot Head Based on Direct-Path Relative Transfer FunctionIEEE/RSJ International Conference on Intelligent Robots and SystemsIEEEDaejeon, South KoreaIEEEOctober 2016, 2819--2826
- 32 articleMultichannel Online Dereverberation based on Spectral Magnitude Inverse FilteringIEEE/ACM Transactions on Audio, Speech and Language Processing279May 2019, 1365-1377
- 33 articleMultichannel Speech Separation and Enhancement Using the Convolutive Transfer FunctionIEEE/ACM Transactions on Audio, Speech and Language Processing273March 2019, 645-659
- 34 articleEstimation of the Direct-Path Relative Transfer Function for Supervised Sound-Source LocalizationIEEE/ACM Transactions on Audio, Speech and Language Processing2411November 2016, 2171--2186
- 35 articleMultiple-Speaker Localization Based on Direct-Path Features and Likelihood Maximization with Spatial Sparsity RegularizationIEEE/ACM Transactions on Audio, Speech and Language Processing251016 pages, 4 figures, 4 tablesOctober 2017, 1997--2012
- 36 articleAudio-noise Power Spectral Density Estimation Using Long Short-term MemoryIEEE Signal Processing Letters266June 2019, 918-922
- 37 articleTracking Gaze and Visual Focus of Attention of People Involved in Social InteractionIEEE Transactions on Pattern Analysis and Machine Intelligence4011https://arxiv.org/abs/1703.04727November 2018, 2711--2724
- 38 articleReal-time Visuomotor Update of an Active Binocular HeadAutonomous Robots341January 2013, 33--45URL: http://hal.inria.fr/hal-00768615
- 39 articleKeypoints and Local Descriptors of Scalar Functions on 2D ManifoldsInternational Journal of Computer Vision1001October 2012, 78--98URL: http://hal.inria.fr/hal-00699620
- 40 articleTopology-Adaptive Mesh Deformation for Surface Evolution, Morphing, and Multi-View ReconstructionIEEE Transactions on Pattern Analysis and Machine Intelligence334April 2011, 823--837URL: http://hal.inria.fr/inria-00590271
- 41 articleRobust Factorization Methods Using A Gaussian/Uniform Mixture ModelInternational Journal of Computer Vision813March 2009, 240--258URL: http://hal.inria.fr/inria-00446987
11.2 Publications of the year
International journals
International peer-reviewed conferences
Doctoral dissertations and habilitation theses
Reports & preprints