Keywords
Computer Science and Digital Science
- A3.1.1. Modeling, representation
- A3.4. Machine learning and statistics
- A5.3. Image processing and analysis
- A5.4. Computer vision
- A5.10. Robotics
- A9. Artificial intelligence
- A9.1. Knowledge
- A9.2. Machine learning
- A9.3. Signal analysis
- A9.5. Robotics
Other Research Topics and Application Domains
- B9.5.1. Computer science
- B9.5.6. Data science
1 Team members, visitors, external collaborators
Research Scientists
- Ivan Laptev [Team leader, Inria, Senior Researcher, HDR]
- Justin Carpentier [Inria, Researcher]
- Jean Paul Laumond [CNRS, Senior Researcher, HDR]
- Jean Ponce [Inria, Senior Researcher, on leave from Ecole Normale Supérieure, HDR]
- Cordelia Schmid [Inria, Senior Researcher, HDR]
Post-Doctoral Fellows
- Alexandre Araujo [Inria, from Oct 2021]
- Shizhe Chen [Inria, from Mar 2021]
- Olivier Flasseur [Univ de Provence, from Apr 2021]
PhD Students
- Minttu Alakuijala [Google, CIFRE]
- Antoine Bambade [Corps des Ponts et Chaussées]
- Adrien Bardes [Facebook, CIFRE]
- Theo Bodrito [Inria, from Nov 2021]
- Oumayma Bounou [Inria]
- Thomas Chabal [Inria, from Oct 2021]
- Nicolas Chahine [DXOMARK, CIFRE, from Aug 2021]
- Elliot Chane-Sane [Inria]
- Zerui Chen [Inria, from Sep 2021]
- Hugo Cisneros [CTU Prague]
- Yann Dubois De Mont-Marin [Inria]
- Thomas Eboli [École Normale Supérieure de Paris, until Sep 2021]
- Aamr El Kazdadi [Inria]
- Alaaeldin El-Nouby [Facebook, CIFRE]
- Matthieu Futeral-Peter [Inria, from Nov 2021]
- Ricardo Jose Garcia Pinel [Inria]
- Pierre Louis Guhur [Univ Paris-Saclay]
- Yana Hasson [Inria, until Aug 2021]
- Marie Heurtevent [Inria, until Mar 2021]
- Wilson Jallet [Inria]
- Yann Labbe [École Normale Supérieure de Paris]
- Quentin Le Lidec [Inria]
- Guillaume Le Moing [Inria]
- Bruno Lecouat [Inria]
- Zongmian Li [Inria]
- Louis Montaut [CTU Prague, ENS]
- Adel Nabli [Inria, until Aug 2021]
- Alexander Pashevich [Inria, until Mar 2021]
- Ronan Riochet [Inria, Jan 2021]
- Robin Strudel [École Normale Supérieure de Paris]
- Lucas Ventura [École Nationale des Ponts et Chaussées, from Sep 2021]
- Elliot Vincent [Ministère de la Transition écologique et solidaire, from Sep 2021]
- Van Huy Vo [Valeo, CIFRE]
- Antoine Yang [Inria]
- Dimitri Zhukov [Inria, until Aug 2021]
Technical Staff
- Etienne Arlaud [Inria, Engineer, from Apr 2021]
- Rohan Budhiraja [Inria, Engineer, from Feb 2021]
- Wilson Jallet [Inria, Engineer, from Mar 2021 until Sep 2021, Pre-thèse]
- Igor Kalevatykh [Inria, Engineer, until June 2021]
- Quentin Le Lidec [Inria, Engineer, from Apr 2021 until Sep 2021, Pre-thèse]
- Ignacio Rocco Spremolla [Inria, Engineer, until Mar 2021]
Interns and Apprentices
- Louis Bodot [École Normale Supérieure de Paris, from Feb 2021 until Jul 2021]
- Thomas Chabal [Inria, from Apr 2021 until Sep 2021]
- Charlotte Perlant [Louis Vuitton, from Apr 2021 until Sep 2021]
- Charles Raude [Inria, from Jun 2021 until Sep 2021]
Administrative Assistants
- Mathieu Mourey [Inria, until Oct 2021]
- Scheherazade Rouag [Inria, from Oct 2021]
External Collaborators
- Mathieu Aubry [ENPC, HDR]
- Josef Sivic [CTU Prague, HDR]
- Gul Varol Simsekli [ENPC]
2 Overall objectives
2.1 Statement
Building machines that can automatically understand complex visual inputs is one of the central scientific challenges in artificial intelligence. Truly successful visual understanding technology will have a broad impact in application domains as varied as defense, entertainment, healthcare, human-computer interaction, image retrieval and data mining, industrial and personal robotics, manufacturing, scientific image analysis, surveillance and security, and transportation.
The problem is, however, very difficult due to the large variability of the visual world and the high complexity of the underling physical phenomena. For example, people easily learn how to perform complex tasks such as changing a car tire or performing resuscitation by observing other people. This involves advanced visual perception and interaction capabilities including interpreting sequences of human actions, learning new visuomotor skills from only a few example demonstrations, grounding instructions in appropriate scene elements and actions, and applying the learned skills in new environments and situations. Currently, however, there is no artificial system with a similar level of cognitive visual competence. Our goal for the next 10 years is to develop models, methods and algorithms providing sufficient level of visual intelligence to enable applications such as personal visual assistants or home robots that will, for example, prepare a meal in response to a chat request.
Despite the tremendous progress in visual recognition in the last decade, current visual recognition systems still require large amounts of carefully annotated training data, often use black-box architectures that do not model the 3D physical nature of the visual world, are typically limited to simple pattern recognition tasks such as detecting and recognizing objects from a predefined vocabulary, and do not capture real-world semantics. We plan to address these limitations with an ambitious research program that aims at developing models of the entire visual understanding process from image acquisition to the high-level embodied interpretation of visual scenes. We target learnable models that require minimal to no supervision, support complex reasoning about visual data, and are grounded in interactions with the physical world. More concretely, we will address fundamental scientific challenges along three research axes: (i) visual recognition in images and videos with an emphasis on weakly supervised learning and models grounded in the physical 3D world; (ii) learning embodied visual representations for robotic manipulation and locomotion; and (iii) image restoration and enhancement. These challenges will be tackled by a team of researchers with core expertise in computer vision and robotics, who will simultaneously advance both fields towards convergence. The complementary expertise in areas such as machine learning and natural language understanding will be gained through collaboration with relevant research teams.
We believe that foundational research should be grounded in applications and we plan to pursue applications with high scientific, societal, and/or economic impact in domains such as transportation; augmented reality; education; advanced manufacturing; and quantitative visual analysis in sciences, humanities and healthcare.
3 Research program
3.1 Visual recognition and reconstruction of images and videos
It is now possible to efficiently detect individual objects and people in cluttered images and videos. Current methods, however, rely on large-scale, manually-annotated image collections, often use black-box architectures that do not model the 3D physical nature of the visual world, and are typically limited to simple pattern recognition tasks. In this part of research program, we address these fundamental limitations. In particular, we address the following three key open challenges: (i) how to leverage available but weak annotations including text, audio and speech, (ii) how to enable automatic reasoning about visual data, and (iii) how to develop models grounded in the physical 3D world including learnable models for 3D object and scene reconstruction. We also continue theoretical work aimed at understanding the geometric underpinnings of computer vision.
Our current efforts in this area are outlined in detail in Section. 8.1.
3.2 Learning embodied representations
Computer vision has come a long way toward understanding images and videos in terms of scene geometry, object labels, locations and poses of people or classes of human actions. This “understanding”, however, remains largely disconnected from reasoning about the physical world. For example, what will happen when removing a tablecloth from a set table? What actions will be needed to resume an interrupted meal? We believe that a true embodied understanding of dynamic scenes from visual observations is the next major research challenge. We address this challenge by developing new models and algorithms with an emphasis on the synergy between vision, learning, robotics and natural language understanding. To this end, we study learning methods for motion planning and optimal control for known environments in state space. At the same time, we develop models and algorithms for learning visio-motor policies that do not rely on the known structure of environments and instead integrate visual perception directly into control algorithms. We also address natural language providing additional modality for more efficient learning and communication with emodied agents.
Our current efforts in this area are outlined in detail in Section 8.2.
3.3 Image restoration and enhancement
Although image processing is a mature field, it is more important than ever with the advent of high-quality camera phones, scientific applications in microscopy and astronomy and, recently, the emergence of multi-modal sensing for autonomous cars for example. In addition, it is an excellent proving ground for learning-based techniques since (a) it is in general (relatively) easy to generate realistic corrupted images from clean ones since reasonable models of the physical image corruption problem as often available (Abdelhamed et al., 2019; Nah et al., 2017), and (b) it is possible to incorporate natural image priors such as self-similarities (Buades et al., 2005) and sparsity (Mairal et al., 2009) in the modelling and optimization processes. We have conducted work on image restoration since the time of Julien Mairal's PhD thesis, addressing problems such as demosaicking, denoising, inpainting, and inverse half-toning with a combination of sparse coding/dictionary learning methods and non-local means, then moving on to blind deblurring including motion segmentation and, more recently, deep-learning methods. In our on-going efforts we address several challenges for learning-based approaches to image restoration: (i) how to combine different modalities such as depth and RGB images to improve the quality of the joint observations; (ii) how to construct tunable, fully interpretable approaches to image restoration in a functional framework; and (iii) how to incorporate machine learning methods that go beyond the traditional fully supervised setting into the image restoration pipeline.
Our current work in this area is outlined in detail in Section 8.3.
4 Application domains
4.1 Introduction
We believe that foundational modeling work should be grounded in applications. This includes (but is not restricted to) the following high-impact domains.
4.2 Automated visual assistants
The modern seamless video communication has enabled new applications in education, medicine and manufacturing, such as remote surgery or remotely-supervised product assembly. The abundance of online instructional videos further confirms the high demand of assistance including daily tasks such as cooking and gardening. Our work on embodied video understanding and on the joint modeling of vision and language will support automatic visual assistants. Similar to existing driving navigation assistants, such applications will guide people in daily living, inspection and manufacturing tasks. Some of these applications will be pursued in our MSR-Inria collaboration.
4.3 Robotics
In 2021, the Willow team has pursued the development of the Pinocchio library both from a scientific and software perspective. The recent versions of Pinocchio now accounts for closed-loop mechanisms (based on a proximal optimization), code source generation on GPUs, etc. All these new features make Pinocchio a unique tool to efficiently control complex robotic systems such as legged robots or industrial robots. We are now closely collaborating with Pal Robotics which plan to use Pinocchio to control its next generation of humanoid robots called Kangaroo. In the near future, the plan is to extend Pinocchio to become a generic-purposed and efficient robotic simulator simulating both rigid and compliant contact interactions between a robot and its environment, with the ambition of making Pinocchio the next golden framework for simulation in robotics, offering advanced features for optimal control, reinforcement learning, like differentiable simulation. Such features should position Pinocchio as the central simulator in Robotics.
4.4 Image restoration
We are pursuing applications of our image restoration work to personal photography, to enhance the images taken by consumer cameras and smartphones by deblurring and denoising them, and improving their spatial resolution and dynamic range. In this context, we are collaborating with DXOMark, the world leader in smartphone camera evaluation, through a CIFRE thesis. Two of the objectives are to develop a public database of portraits fully compliant with European GDRP regulations with informed consent from the models, and to automate the rating of image quality using this dataset. We also apply the mixture of physical image formation model and machine learning principles that has made our image restoration work successful to scientific fields: We collaborate with Anne-Marie Lagrange (Observatoire de Paris), Maud Langlois (SNRS/Observatoire de Lyon) and Julien Mairal (Inria) on direct exoplanet detection from ground-based telescope imagery. This work also involves a post-doc, Olivier Flasseur, and a PhD Student, Théo Bodrito. We will apply next year the same principles to molecular microscopy, in collaboration with Jean-Baptiste Masson (Institut Pasteur).
5 Social and environmental responsibility
Artificial intelligence holds great potential for improving our environment, for example, by reducing energy consumption and optimizing energy production. Computer vision, in particular, can be used to monitor emissions from coal plants and to track forest growth using satellite imagery. Autonomous drones can monitor and prevent failures of pipelines, power lines, power plants and other remote installations. However, as larger and more powerful AI models require increased compute power at training and inference, AI itself stands for an increasingly high carbon footprint. One direction of our research aims to develop efficient and low-resource neural network models. To this end we have proposed Cross-Covariance Image Transformers 16 that avoid quadratic complexity in terms of image size. We have also developed efficient text-to-visual retrieval with transformers in 24.
6 Highlights of the year
6.1 Awards
- PAMI Distinguished Researcher Award, 2021 for C. Schmid.
- Winner of the REVERIE / Soon Challenge, in conjunction with ICCV 2021 for S. Chen, I. Laptev and C. Schmid.
- AFRIF PhD Thesis Prize 2021 for Ignacio Rocco.
- Outstanding reviewer at ICCV 2021 for Y. Hasson.
- Best poster prize at the 2nd International Workshop on AI for Robotics, Naver Labs Europe 2021 for J. Sivic and J. Carpentier.
7 New software and platforms
7.1 New software
7.1.1 Pinocchio
-
Name:
Pinocchio
-
Keywords:
Robotics, Biomechanics, Mechanical multi-body systems
-
Functional Description:
Pinocchio instantiates state-of-the-art Rigid Body Algorithms for poly-articulated systems based on revisited Roy Featherstone's algorithms. In addition, Pinocchio instantiates analytical derivatives of the main Rigid-Body Algorithms like the Recursive Newton-Euler Algorithms or the Articulated-Body Algorithm. Pinocchio is first tailored for legged robotics applications, but it can be used in extra contexts. It is built upon Eigen for linear algebra and FCL for collision detection. Pinocchio comes with a Python interface for fast code prototyping.
- URL:
-
Contact:
Justin Carpentier
-
Partner:
CNRS
7.1.2 Segmenter
-
Name:
Segmenter: Transformer for Semantic Segmentation
-
Keywords:
Semantic Segmentation, Image segmentation
-
Functional Description:
Image segmentation aims at precisely localizing objects in an image. Each pixel of an image is associated to a class, typically a person, a car, a bike etc. This is a fundamental problem in computer vision that has numerous applications such as autonomous driving, robotics or medical imagery.
- Publication:
-
Contact:
Robin Strudel
-
Participants:
Robin Strudel, Ricardo Garcia Pinel, Ivan Laptev, Cordelia Schmid
7.1.3 VLN-HAMT
-
Name:
History Aware Multimodal Transformer for Vision-and-Language Navigation
-
Keyword:
Computer vision
-
Functional Description:
Open source release of the software package for the NeurIPS'21 paper by Chen et al. "History Aware Multimodal Transformer for Vision-and-Language Navigation". This release provides a full implementation of the method, including code for training models, and testing on standard datasets, as well as trained models.
- URL:
- Publication:
-
Contact:
Shize Chen
-
Participants:
Shize Chen, Pierre-Louis Guhur, Cordelia Schmid, Ivan Laptev
7.1.4 Goal-Conditioned Reinforcement Learning with Imagined Subgoals
-
Keywords:
Robotics, Reinforcement learning, Deep learning
-
Functional Description:
Code associated with the paper "Goal-Conditioned Reinforcement Learning with Imagined Subgoals"
- URL:
- Publication:
-
Contact:
Elliot Chane-Sane
-
Participants:
Elliot Chane-Sane, Cordelia Schmid, Ivan Laptev
7.1.5 hybrid-nca-evocraft
-
Name:
Open-ended creation of hybrid creatures with Neural Cellular Automata
-
Keywords:
Cellular automaton, Evolution, Evolutionary Algorithms
-
Functional Description:
Our algorithm is based on Neural Cellular Automata (NCA), a CA-based neural network model inspired by morphogenesis. We chose to work with cellular automata, as we're interested in models where complexity can be spontaneously increasing over time, which is a property that traditional models (like neural networks) do not have. We train a NCA to grow different patterns from various seeds (or "genomes") in 2 or 3 dimensions. Once the training is done, we load the model in Minecraft and have players modify the genomes. They can be mutated or merged to create an endless stream of novel growing patterns. The resulting patterns depend both on the genome and the growth rules learned offline by the NCA, which can be unpredictable and surprising.
The repository contains link to pre-trained models in 2D and 3D as well as Colab notebooks links to train you own NCA.
The current codebase is very simplistic and therefore not very usable, because that's only what I needed to run my experiments and try several things. I plan to make it more usable in the near future.
- URL:
-
Contact:
Hugo Cisneros
7.1.6 CCVS: Context-aware Controllable Video Synthesis
-
Keywords:
Computer vision, Video synthesis, Deep learning
-
Functional Description:
Code, results and models associated with the paper "CCVS: Context-aware Controllable Video Synthesis"
- URL:
- Publication:
-
Contact:
Guillaume Le Moing
-
Participants:
Jean Ponce, Cordelia Schmid
7.1.7 BurstSR
-
Name:
Super-resolution from image bursts
-
Keyword:
Image processing
-
Functional Description:
This is a research prototpye allowing to take as input a sequence of raw or rgb images produced by a smartphone or digital camera. This code produces a high quality color images with higher resolution.
-
Contact:
Bruno Lecouat
7.1.8 LOD
-
Name:
Large-Scale Unsupervised Object Discovery
-
Keywords:
Unsupervised learning, Computer vision
-
Scientific Description:
Paper: Large-Scale Unsupervised Object Discovery Huy V. Vo, Elena Sizikova, Cordelia Schmid, Patrick Pérez, Jean Ponce In Proc. NeurIPS 2021, Canada. Hal: hal-03541587
-
Functional Description:
Implementation of the method proposed in Large-Scale Unsupervised Object Discovery. Huy V. Vo, Elena Sizikova, Cordelia Schmid, Patrick Pérez, Jean Ponce. In Proc. NeurIPS 2021, Canada.
-
Contact:
Van Vo
7.1.9 LOST
-
Name:
Localizing Objects with Self-Supervised Transformers and no Labels
-
Keyword:
Computer vision
-
Scientific Description:
Localizing Objects with Self-Supervised Transformers and no Labels. Oriane Siméoni, Gilles Puy, Huy V. Vo, Simon Roburin, Spyros Gidaris, Andrei Bursuc, Patrick Pérez, Renaud Marlet, Jean Ponce. In Proc. BMVC 2021, United Kingdom.
-
Functional Description:
Implementation of the method proposed in Localizing Objects with Self-Supervised Transformers and no Labels, Oriane Siméoni, Gilles Puy, Huy V. Vo, Simon Roburin, Spyros Gidaris, Andrei Bursuc, Patrick Pérez, Renaud Marlet, Jean Ponce, in Proc. BMVC 2021, United Kingdom.
- Publication:
-
Contact:
Van Vo
7.1.10 LeSegWeakMultiTask
-
Name:
Improving Weakly Supervised Lesion Segmentation using Multi-Task Learning
-
Keyword:
Computer vision
-
Scientific Description:
Improving Weakly Supervised Lesion Segmentation using Multi-Task Learning. Tianshu Chu, Xinmeng Li, Huy V. Vo, Ronald Summers, Elena Sizikova MIDL 2021 - Medical Imaging with Deep Learning, Jul 2021, Lubeck, Germany
hal: hal-03478040
-
Functional Description:
Implementation of the method proposed in Improving Weakly Supervised Lesion Segmentation using Multi-Task Learning. Tianshu Chu, Xinmeng Li, Huy V. Vo, Ronald Summers, Elena Sizikova MIDL 2021 - Medical Imaging with Deep Learning, Jul 2021, Lubeck, Germany
- Publication:
-
Contact:
Van Vo
7.1.11 airbert
-
Name:
Airbert
-
Keywords:
Computer vision, Natural language processing, Machine learning, Robotics
-
Functional Description:
Open source release of the software package for the ICCV'21 paper by Guhur et al. "Airbert: In-domain Pretraining for Vision-and-Language Navigation". This release provides a full implementation of the method, including code for training models, and testing on standard datasets, as well as trained models.
-
Contact:
Pierre-Louis Guhur
7.1.12 Just Ask: Learning to Answer Questions from Millions of Narrated Videos
-
Keywords:
Computer vision, Natural language processing, Deep learning
-
Functional Description:
Code, datasets and models associated with the paper "Just Ask: Learning to Answer Questions from Millions of Narrated Videos"
- URL:
-
Contact:
Antoine Yang
7.2 New platforms
Together with SED we are bulding the new robotics laboratory at Inria Paris located on the 5th floor of the C building. This laboratory is currently composed of two robotic anthropomorphic arms for manipulation experiments mounted on a fixed frame basement, as well as the Tigao++ robot. In 2021 we have aquired two SOLO quadruped robots that will enable our future research and experiments with locomotion and navigation.
8 New results
8.1 Visual recognition and reconstruction of images and videos
8.1.1 Large-Scale Unsupervised Object Discovery
Participants: Huy V. Vo, Elena Sizikova, Cordelia Schmid, Patrick Perez, Jean Ponce.
Existing approaches to unsupervised object discovery (UOD) do not scale up to large datasets without approximations that compromise their performance. We propose in 31 a novel formulation of UOD as a ranking problem, amenable to the arsenal of distributed methods available for eigenvalue problems and link analysis. Through the use of self-supervised features, we also demonstrate the first effective fully unsupervised pipeline for UOD. Extensive experiments on COCO and OpenImages show that, in the single-object discovery setting where a single prominent object is sought in each image, the proposed LOD (Large-scale Object Discovery) approach is on par with, or better than the state of the art for medium-scale datasets (up to 120K images), and over 37% better than the only other algorithms capable of scaling up to 1.7M images. In the multi-object discovery setting where multiple objects are sought in each image, the proposed LOD is over 14% better in average precision (AP) than all other methods for datasets ranging from 20K to 1.7M images. Using self-supervised features, we also show that the proposed method obtains state-of-the-art UOD performance on OpenImages. Figure 1 shows some qualitative results of LOD on the Open Images dataset. Our code is publicly available at https://github.com/huyvvo/LOD.
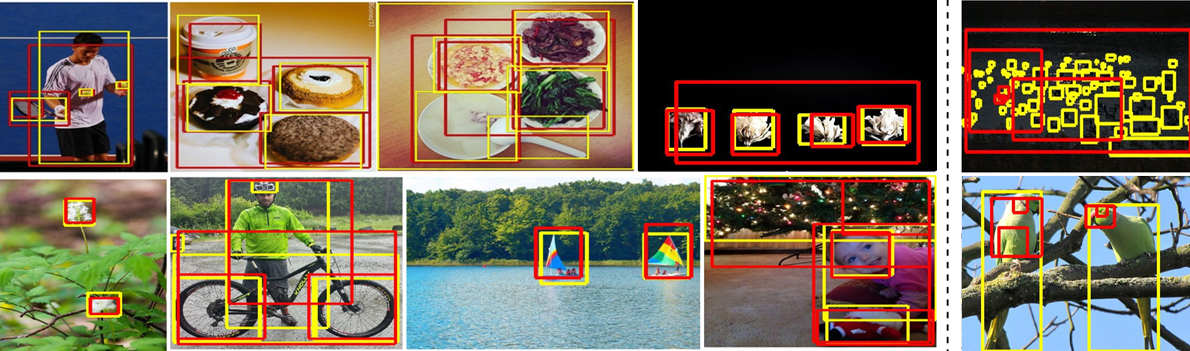
8.1.2 Localizing Objects with Self-Supervised Transformers and no Labels
Participants: Oriane Simeoni, Gilles Puy, Huy V. Vo, Simon Roburin, Spyros Gidaris, Andrei Bursuc, Patrick Perez, Renaud Marlet, Jean Ponce.
Localizing objects in image collections without supervision can help to avoid expensive annotation campaigns. In 29 we propose a simple approach to this problem, that leverages the activation features of a vision transformer pre-trained in a self-supervised manner. Our method, LOST, does not require any external object proposal nor any exploration of the image collection; it operates on a single image. Yet, we outperform state-of-the-art object discovery methods by up to 8 CorLoc points on PASCAL VOC 2012. We also show that training a class-agnostic detector on the discovered objects boosts results by another 7 points. Moreover, we show promising results on the unsupervised object discovery task. Figure 2 shows some qualitative results of LOST on unsupervised single-object discovery, multi-object discovery and object detection. The code to reproduce our results can be found at https://github.com/valeoai/LOST.

8.1.3 VICReg: Variance-Invariance-Covariance Regularization for Self-Supervised Learning
Participants: Adrien Bardes, Jean Ponce, Yann LeCun.
Recent self-supervised methods for image representation learning maximize the agreement between embedding vectors produced by encoders fed with different views of the same image. The main challenge is to prevent a collapse in which the encoders produce constant or non-informative vectors. In our work 9, we introduce VICReg (Variance-Invariance-Covariance Regularization), a method that explicitly avoids the collapse problem with two regularizations terms applied to both embeddings separately: (1) a term that maintains the variance of each embedding dimension above a threshold, (2) a term that decorrelates each pair of variables. Unlike most other approaches to the same problem, VICReg does not require techniques such as: weight sharing between the branches, batch normalization, feature-wise normalization, output quantization, stop gradient, memory banks, etc., and achieves results on par with the state of the art on several downstream tasks. The architecture of VICReg is presented in Figure 3.
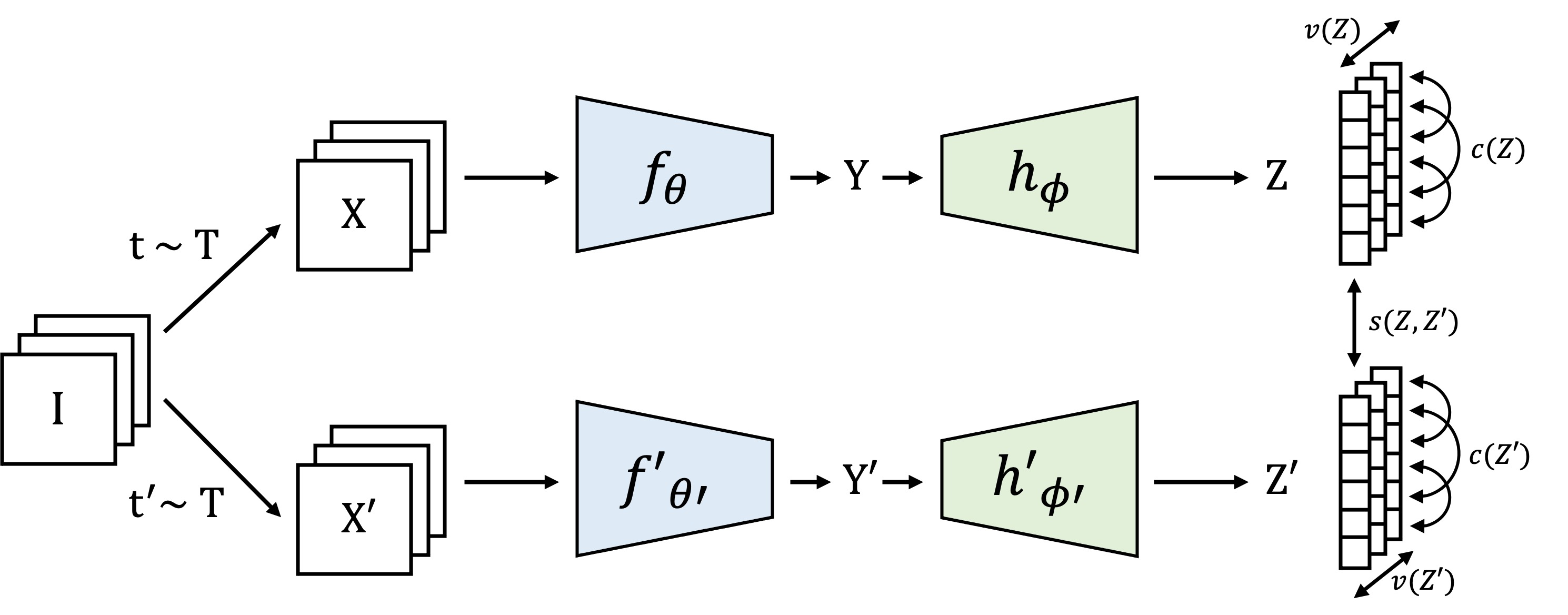
VICReg: joint embedding architecture with variance, invariance and covariance regularization. Given a batch of images I, two batches of different views XX and X'X^{\prime } are mapped to embeddings ZZ and Z'Z^{\prime }. The distance between two embeddings from the same image is minimized, the variance of each embedding variable over a batch is maintained above a threshold, and the covariance between pairs of embedding variables over a batch are attracted to zero, decorrelating the variables from each other.
8.1.4 XCiT: Cross-Covariance Image Transformers
Participants: Alaaeldin El-Nouby, Hugo Touvron, Mathilde Caron, Piotr Bojanowski, Matthijs Douze, Armand Joulin, Ivan Laptev, Natalia Neverova, Gabriel Synnaeve, Jakob Verbeek, Herve Jegou.
Following their success in natural language processing, transformers have recently shown much promise for computer vision. The self-attention operation underlying transformers yields global interactions between all tokens, i.e. words or image patches, and enables flexible modelling of image data beyond the local interactions of convolutions. This flexibility, however, comes with a quadratic complexity in time and memory, hindering application to long sequences and high-resolution images. In 16 we propose a "transposed" version of self-attention that operates across feature channels rather than tokens, where the interactions are based on the cross-covariance matrix between keys and queries. The resulting cross-covariance attention (XCA) has linear complexity in the number of tokens, and allows efficient processing of high-resolution images. Our cross-covariance image transformer (XCiT) is built upon XCA. It combines the accuracy of conventional transformers with the scalability of convolutional architectures. An overview of XCiT is presented in Figure 4. We validate the effectiveness and generality of XCiT by reporting excellent results on multiple vision benchmarks, including image classification and self-supervised feature learning on ImageNet-1k, object detection and instance segmentation on COCO, and semantic segmentation on ADE20k.
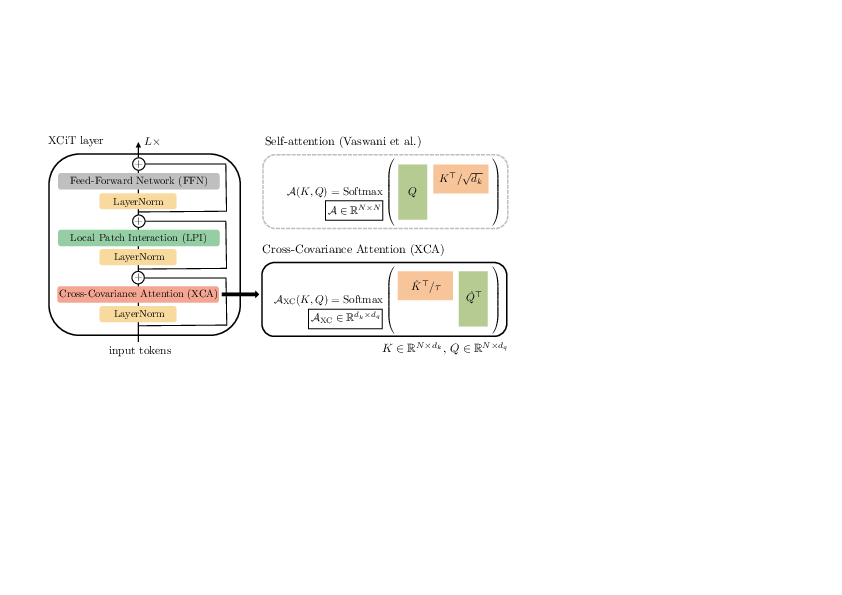
8.1.5 Just Ask: Learning to Answer Questions from Millions of Narrated Videos
Participants: Antoine Yang, Antoine Miech, Josef Sivic, Ivan Laptev, Cordelia Schmid.
Recent methods for visual question answering rely on large-scale annotated datasets. Manual annotation of questions and answers for videos, however, is tedious, expensive and prevents scalability. In 32, we propose to avoid manual annotation and generate a large-scale training dataset for video question answering making use of automatic cross-modal supervision. We leverage a question generation transformer trained on text data and use it to generate question-answer pairs from transcribed video narrations. Given narrated videos, we then automatically generate the HowToVQA69M dataset with 69M video-question-answer triplets. Figure 5 presents some examples of generated samples. To handle the open vocabulary of diverse answers in this dataset, we propose a training procedure based on a contrastive loss between a video-question multi-modal transformer and an answer transformer. We introduce the zero-shot VideoQA task and show excellent results, in particular for rare answers. Furthermore, we demonstrate our method to significantly outperform the state of the art on MSRVTT-QA, MSVD-QA, ActivityNet-QA and How2QA. Finally, for a detailed evaluation we introduce iVQA, a new VideoQA dataset with reduced language biases and high-quality redundant manual annotations.
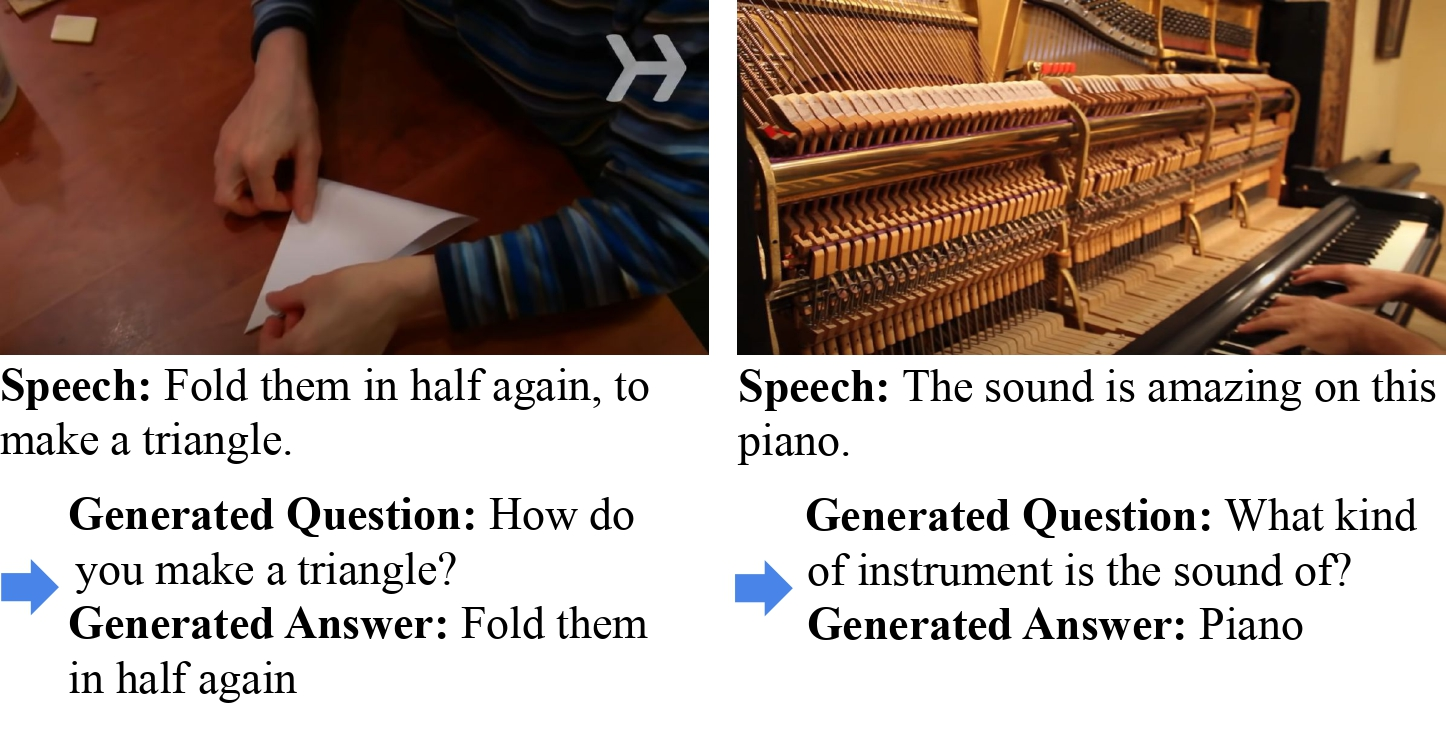
Two examples from our automatically generated HowToVQA69M dataset. Given videos with transcribed narration, we leverage language models and cross-modal supervision to obtain large-scale VideoQA data.
8.1.6 Thinking Fast and Slow: Efficient Text-to-Visual Retrieval with Transformers
Participants: Antoine Miech, Jean-Baptiste Alayrac, Ivan Laptev, Josef Sivic, Andrew Zisserman.
Our objective in 24 is language-based search of large-scale image and video datasets. The mapping of text and vision to a joint embedding space, a.k.a. dual encoders, is an attractive approach for this task as it enables scalable and efficient retrieval for billions of images using approximate nearest neighbour search. An alternative approach of using vision-text transformers with cross-attention gives considerable improvements in accuracy over the joint embeddings, but is often inapplicable in practice for large-scale retrieval given the cost of the cross-attention mechanisms required for each sample at test time. This work combines the best of both worlds. We make the following three contributions. First, we equip transformer-based models with a new fine-grained cross-attention architecture, providing significant improvements in retrieval accuracy whilst preserving scalability. Second, we introduce a generic approach for combining a Fast dual encoder model with our Slow but accurate transformer-based model via distillation and re-ranking. Finally, we validate our approach on the Flickr30K image dataset where we show an increase in inference speed by several orders of magnitude while having results competitive to the state of the art. We also extend our method to the video domain, improving the state of the art on the VATEX dataset. An overview of our approach is presented in Figure 6.
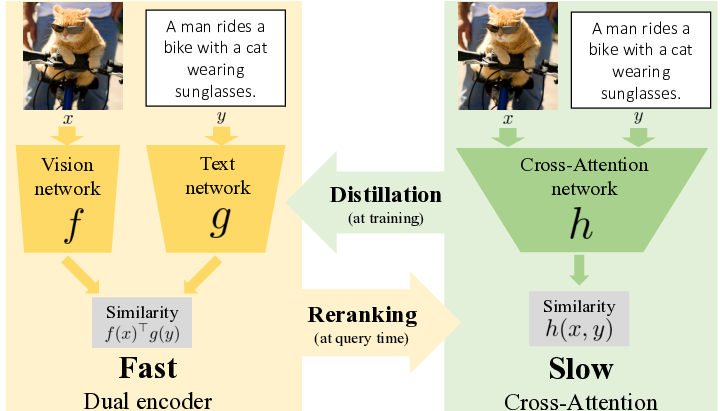
On the left, the Fast models, a.k.a dual encoders, independently process the input image and text to compute a similarity score via a single dot product, which can be efficiently indexed and is thus amenable to large-scale search. On the right, the Slow models, a.k.a cross-attention models, jointly process the input image and text with cross-modal attention to compute a similarity score. Fast and indexable models are improved by Slow models via distillation at training time (offline). Slow models are accelerated and improved with the distilled Fast approaches using a re-ranking strategy at query time.
8.1.7 Segmenter: Transformer for Semantic Segmentation
Participants: Robin Strudel, Ricardo Garcia, Ivan Laptev, Cordelia Schmid.
Semantic Segmentation as a sequence-to-sequence problem with Vision Transformers. Image segmentation is often ambiguous at the level of individual image patches and requires contextual information to reach label consensus. In 30 we introduce Segmenter, a transformer model for semantic segmentation. In contrast to convolution-based methods, our approach allows to model global context already at the first layer and throughout the network. We build on the recent Vision Transformer (ViT) and extend it to semantic segmentation. To do so, we rely on the output embeddings corresponding to image patches and obtain class labels from these embeddings with a point-wise linear decoder or a mask transformer decoder. We leverage models pre-trained for image classification and show that we can fine-tune them on moderate sized datasets available for semantic segmentation. The linear decoder allows to obtain excellent results already, but the performance can be further improved by a mask transformer generating class masks. We conduct an extensive ablation study to show the impact of the different parameters, in particular the performance is better for large models and small patch sizes. Segmenter attains excellent results for semantic segmentation. It outperforms the state of the art on both ADE20K and Pascal Context datasets and is competitive on Cityscapes. Figure 7 presents an overview of our method.
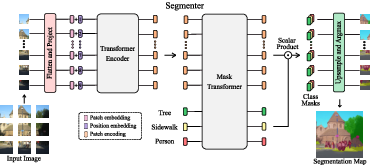
8.1.8 Synthetic Humans for Action Recognition from Unseen Viewpoints
Participants: Gul Varol, Ivan Laptev, Cordelia Schmid, Andrew Zisserman.
In 5 we use synthetic training data to improve the performance of human action recognition for viewpoints unseen during training. Although synthetic data has been shown to be beneficial for tasks such as human pose estimation, its use for RGB human action recognition is relatively unexplored. We make use of the recent advances in monocular 3D human body reconstruction from real action sequences to automatically render synthetic training videos for the action labels. We make the following contributions: (i) we investigate the extent of variations and augmentations that are beneficial to improving performance at new viewpoints. We consider changes in body shape and clothing for individuals, as well as more action relevant augmentations such as non-uniform frame sampling, and interpolating between the motion of individuals performing the same action; (ii) We introduce a new dataset, SURREACT, that allows supervised training of spatio-temporal CNNs for action classification; (iii) We substantially improve the state-of-the-art action recognition performance on the NTU RGB+D and UESTC standard human action multi-view benchmarks; Finally, (iv) we extend the augmentation approach to in-the-wild videos from a subset of the Kinetics dataset to investigate the case when only one-shot training data is available, and demonstrate improvements in this case as well. Figure 8 illustrates our approach.
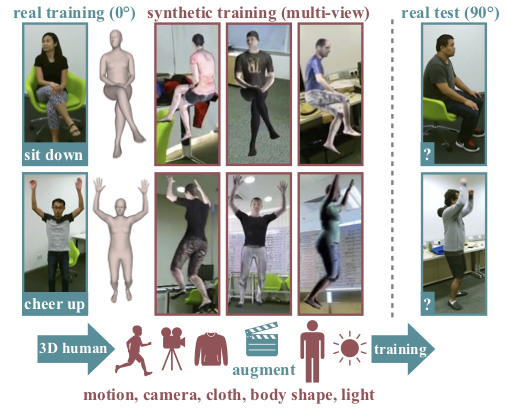
8.1.9 Differentiable Rendering with Perturbed Optimizers
Participants: Quentin Le Lidec, Ivan Laptev, Cordelia Schmid, Justin Carpentier.
Reasoning about 3D scenes from their 2D image projections is one of the core problems in computer vision. Solutions to this inverse and ill-posed problem typically involve a search for models that best explain observed image data. Notably, images depend both on the properties of observed scenes and on the process of image formation. Hence, if optimization techniques should be used to explain images, it is crucial to design differentiable functions for the projection of 3D scenes into images, also known as differentiable rendering. Previous approaches to differentiable rendering typically replace non-differentiable operations by smooth approximations, impacting the subsequent 3D estimation. In our work 22 we take a more general approach and study differentiable renderers through the prism of randomized optimization and the related notion of perturbed optimizers. In particular, our work highlights the link between some well-known differentiable renderer formulations and randomly smoothed optimizers, and introduces differentiable perturbed renderers. We also propose a variance reduction mechanism to alleviate the computational burden inherent to perturbed optimizers and introduce an adaptive scheme to automatically adjust the smoothing parameters of the rendering process. We apply our method to 3D scene reconstruction and demonstrate its advantages on the tasks of 6D pose estimation and 3D mesh reconstruction. By providing informative gradients that can be used as a strong supervisory signal, we demonstrate the benefits of perturbed renderers to obtain more accurate solutions when compared to the state-of-the-art alternatives using smooth gradient approximations. An overview of our approach is illustrated in Figure 9.
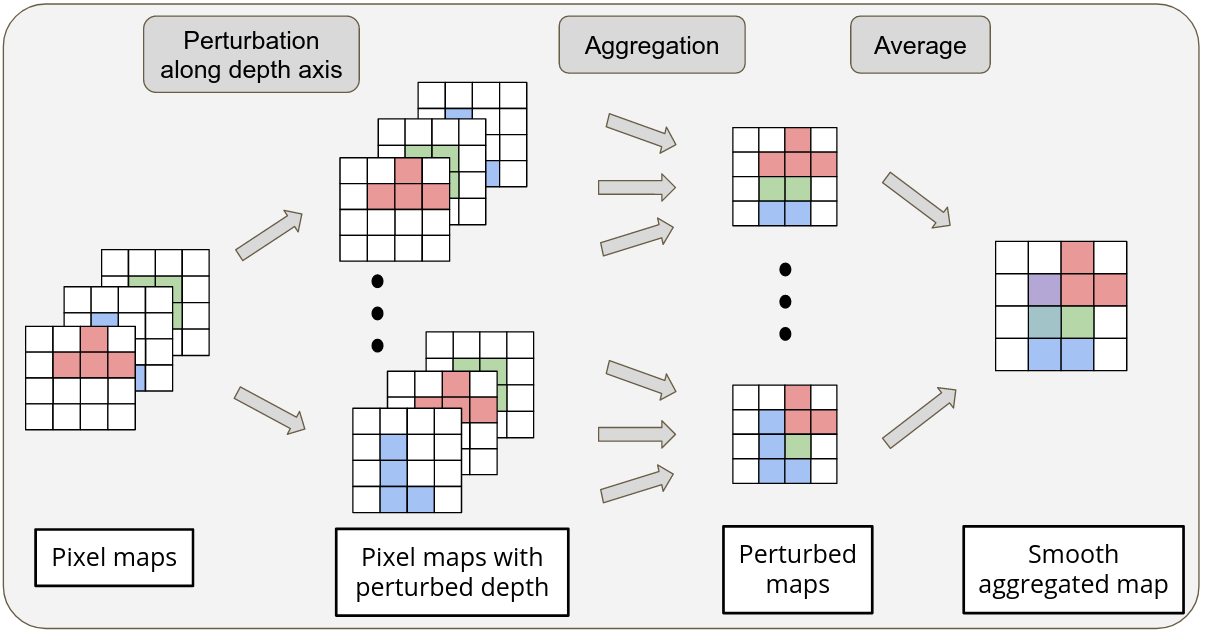
8.1.10 CCVS: Context-aware Controllable Video Synthesis
Participants: Guillaume Le Moing, Jean Ponce, Cordelia Schmid.
Feeding machines with extensive video content, and teaching them to create new samples on their own, may deepen their understanding of both the physical and social worlds. In 25 we introduces a self-supervised learning approach to the synthesis of new video clips from old ones, with several new key elements for improved spatial resolution and realism: It conditions the synthesis process on contextual information for temporal continuity and ancillary information for fine control. The prediction model is doubly autoregressive, in the latent space of an autoencoder for forecasting, and in image space for updating contextual information, which is also used to enforce spatio-temporal consistency through a learnable optical flow module. Adversarial training of the autoencoder in the appearance and temporal domains is used to further improve the realism of its output. A quantizer inserted between the encoder and the transformer in charge of forecasting future frames in latent space (and its inverse inserted between the transformer and the decoder) adds even more flexibility by affording simple mechanisms for handling multimodal ancillary information for controlling the synthesis process (e.g., a few sample frames, an audio track, a trajectory in image space) and taking into account the intrinsically uncertain nature of the future by allowing multiple predictions. Experiments with an implementation of the proposed approach give very good qualitative and quantitative results on multiple tasks and standard benchmarks. Some video samples synthesized by our approach can be found in Figure 10. Code, pretrained models, and additional video samples are available at https://16lemoing.github.io/ccvs.
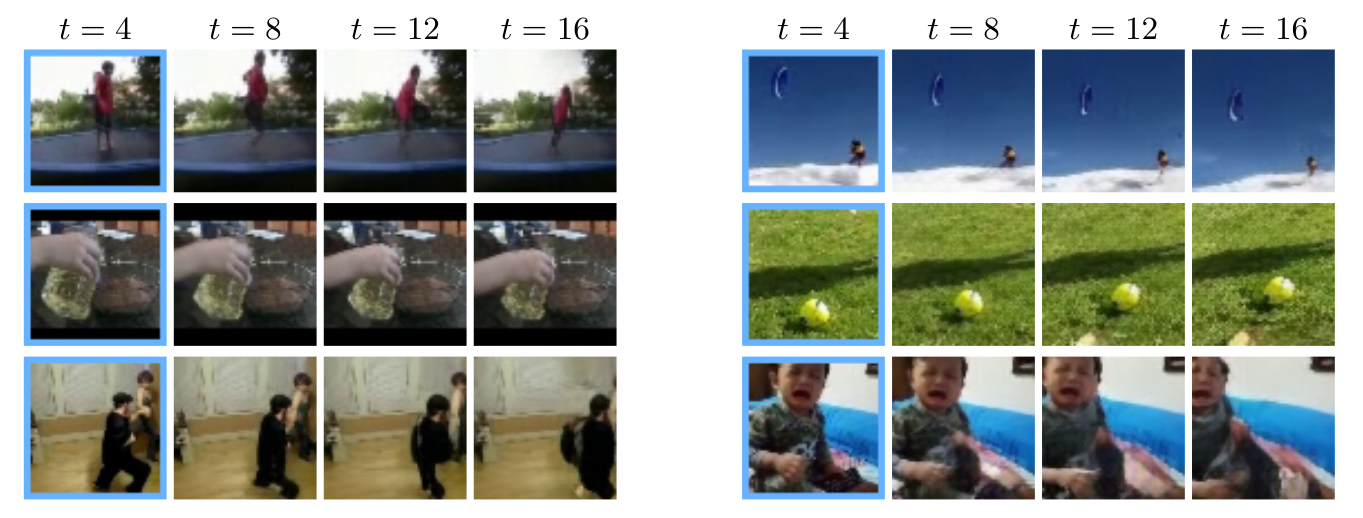
8.1.11 Estimating 3D Motion and Forces of Human-Object Interactions from Internet Videos
Participants: Zongmian Li, Jiri Sedlar, Justin Carpentier, Ivan Laptev, Nicolas Mansard, Josef Sivic.
In 4, we introduce a method to automatically reconstruct the 3D motion of a person interacting with an object from a single RGB video. As shown in Figure 11, our method estimates the 3D poses of the person together with the object pose, the contact positions and the contact forces exerted on the human body. The main contributions of this work are three-fold. First, we introduce an approach to jointly estimate the motion and the actuation forces of the person on the manipulated object by modeling contacts and the dynamics of the interactions. This is cast as a large-scale trajectory optimization problem. Second, we develop a method to automatically recognize from the input video the 2D position and timing of contacts between the person and the object or the ground, thereby significantly simplifying the complexity of the optimization. Third, we validate our approach on a recent video+MoCap dataset capturing typical parkour actions, and demonstrate its performance on a new dataset of Internet videos showing people manipulating a variety of tools in unconstrained environments.
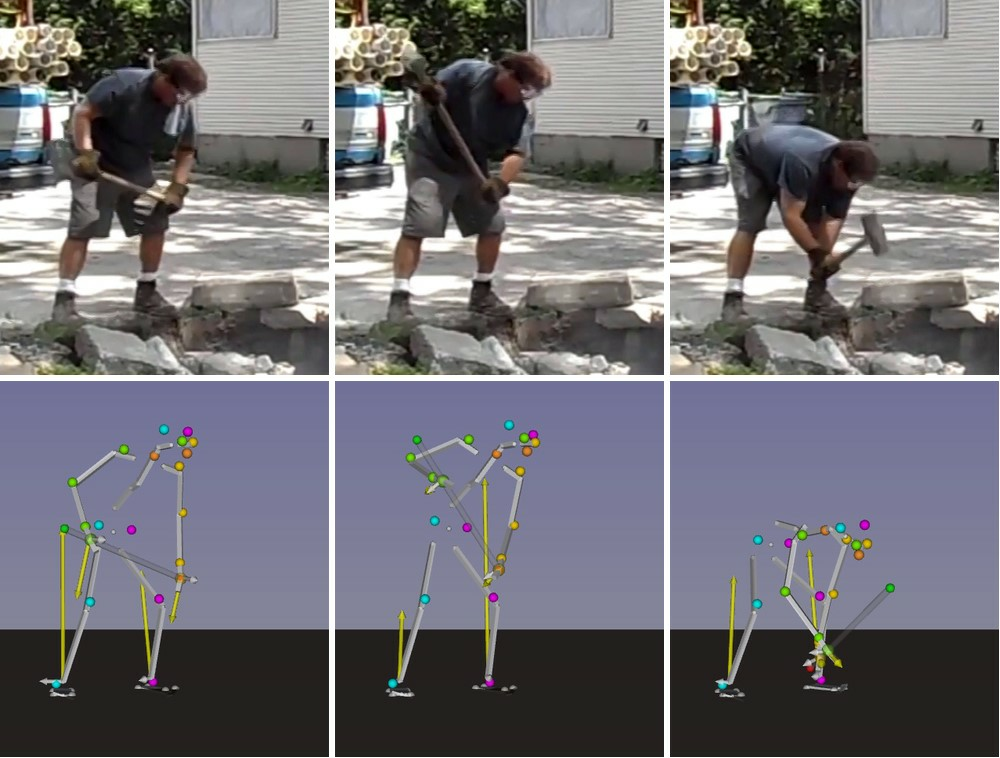
8.2 Learning embodied representations
8.2.1 Online Learning and Control of Dynamical Systems from Sensory Input
Participants: Oumayma Bounou, Jean Ponce, Justin Carpentier.
Identifying an effective model of a dynamical system from sensory data and using it for future state prediction and control is challenging. Recent data-driven algorithms based on Koopman theory are a promising approach to this problem, but they typically never update the model once it has been identified from a relatively small set of observation, thus making long-term prediction and control difficult for realistic systems, in robotics or fluid mechanics for example. This paper introduces a novel method for learning an embedding of the state space with linear dynamics from sensory data. Unlike previous approaches, the dynamics model can be updated online and thus easily applied to systems with non-linear dynamics in the original configuration space. Our approach proposed in 11 is evaluated empirically on several classical dynamical systems and sensory modalities, with good performance on long-term prediction and control. An overview of our approach is illustrated in Figure 12.

General overview of the proposed pipeline: the measurements [d1,⋯,dT][d_{1}, \dots , d_{T}] are first encoded with Φθ\Phi _{\theta } to codes [z1,⋯,zT][z_{1}, \dots , z_{T}]. Only the mm first codes are used to estimate the linear system dynamics AA. Using this linear dynamics AA and the last code zmz_{m}, the last T-mT - m codes are predicted. The resulting reconstructed measurements [d^1,⋯,d^T][\hat{d}_{1}, \dots , \hat{d}_{T}] are obtained by decoding with Ψθ\Psi _{\theta } the embeddings of the actual measurements [z1,⋯,zm][z_{1}, \dots , z_{m}] and the predicted embeddings [zm+1,⋯,zT][z_{m+1}, \dots , z_{T}].
8.2.2 Goal-Conditioned Reinforcement Learning with Imagined Subgoals
Participants: Elliot Chane-Sane, Cordelia Schmid, Ivan Laptev.
Goal-conditioned reinforcement learning endows an agent with a large variety of skills, but it often struggles to solve tasks that require more temporally extended reasoning. In 13, we propose to incorporate imagined subgoals into policy learning to facilitate learning of complex tasks. Imagined subgoals are predicted by a separate high-level policy, which is trained simultaneously with the policy and its critic. This high-level policy predicts intermediate states halfway to the goal using the value function as a reachability metric. We don't require the policy to reach these subgoals explicitly. Instead, we use them to define a prior policy, and incorporate this prior into a KL-constrained policy iteration scheme to speed up and regularize learning. Imagined subgoals are used during policy learning, but not during test time, where we only apply the learned policy. We evaluate our approach on complex robotic navigation and manipulation tasks and show that it outperforms existing methods by a large margin. Figure 13 presents an illustration of the approach.
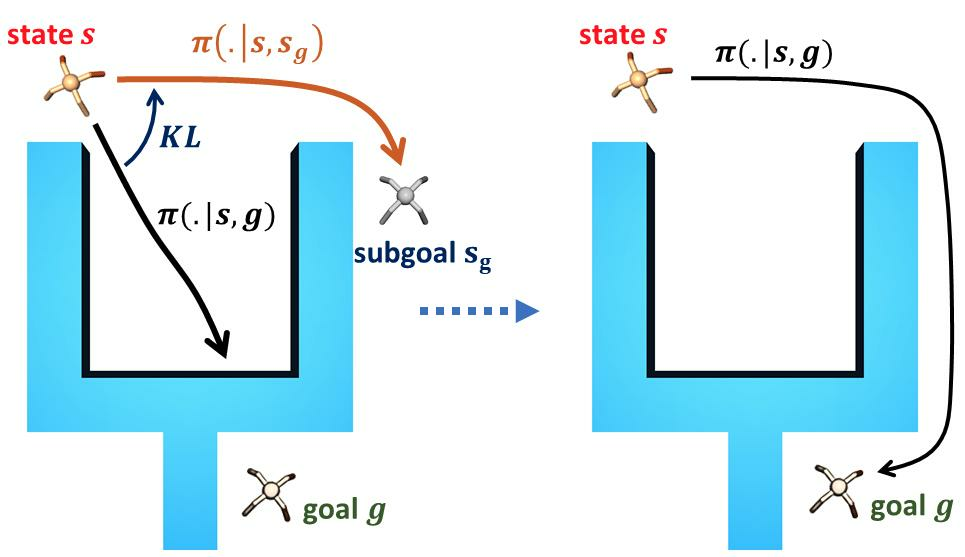
8.2.3 Single-view robot pose and joint angle estimation via render & compare
Participants: Yann Labbe, Justin Carpentier, Mathieu Aubry, Josef Sivic.
In 21, we introduce RoboPose, a method to estimate the joint angles and the 6D camera-to-robot pose of a known articulated robot from a single RGB image. This is an important problem to grant mobile and itinerant autonomous systems the ability to interact with other robots using only visual information in non-instrumented environments, especially in the context of collaborative robotics. It is also challenging because robots have many degrees of freedom and an infinite space of possible configurations that often result in self-occlusions and depth ambiguities when imaged by a single camera. The contributions of this work are three-fold. First, we introduce a new render & compare approach for estimating the 6D pose and joint angles of an articulated robot that can be trained from synthetic data, generalizes to new unseen robot configurations at test time, and can be applied to a variety of robots. Second, we experimentally demonstrate the importance of the robot parametrization for the iterative pose updates and design a parametrization strategy that is independent of the robot structure. Finally, we show experimental results on existing benchmark datasets for four different robots and demonstrate that our method significantly outperforms the state of the art. Code and pre-trained models are available on the project webpage https://www.di.ens.fr/willow/research/robopose/. The method is illustrated in Figure 14.
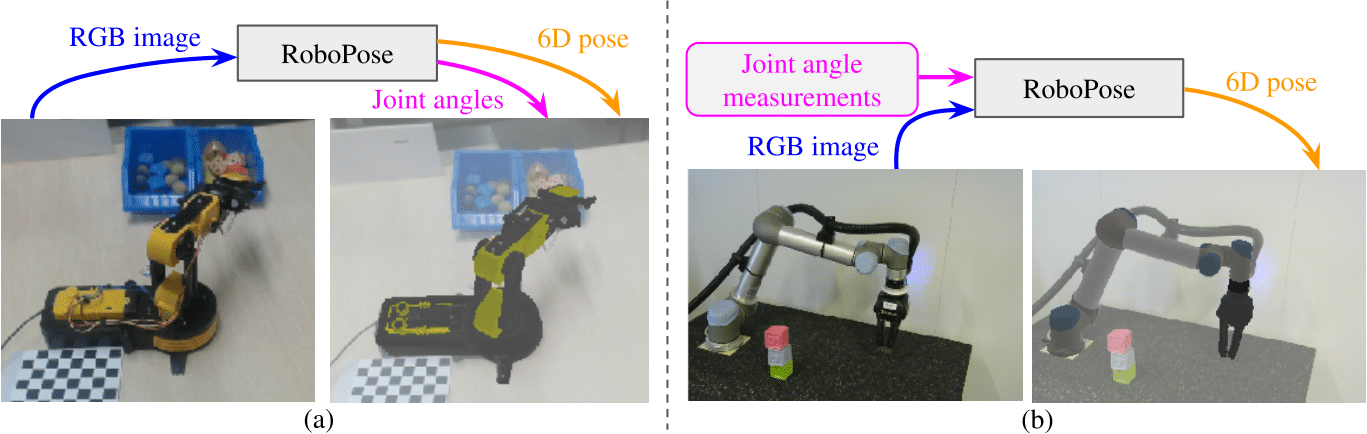
8.2.4 Airbert: In-domain Pretraining for Vision-and-Language Navigation
Participants: Pierre-Louis Guhur, Makarand Tapaswi, Shizhe Chen, Ivan Laptev, Cordelia Schmid.
Vision-and-language navigation (VLN) aims to enable embodied agents to navigate in realistic environments using natural language instructions. Given the scarcity of domain-specific training data and the high diversity of image and language inputs, the generalization of VLN agents to unseen environments remains challenging. Recent methods explore pretraining to improve generalization, however, the use of generic image-caption datasets or existing small scale VLN environments is suboptimal and results in limited improvements. In 17, we introduce BnB, a large scale and diverse in-domain VLN dataset. Figure 15 illustrates our approach to the generation of path-instruction pairs using BnB. We further propose a shuffling loss that improves the learning of temporal order inside PI pairs. We use BnB to pretrain our Airbert model that can be adapted to discriminative and generative settings and show that it outperforms state of the art for Room-to-Room (R2R) navigation and Remote Referring Expression (REVERIE) benchmarks. Moreover, our in-domain pretraining significantly increases performance on a challenging few-shot VLN evaluation, where we train the model only on VLN instructions from a few houses.
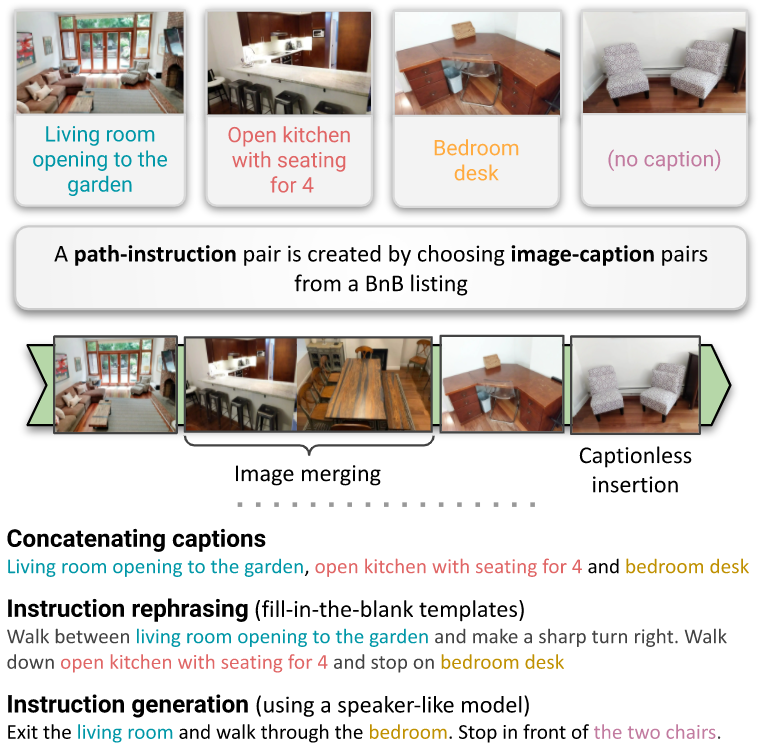
8.2.5 History Aware Multimodal Transformer for Vision-and-Language Navigation
Participants: Shizhe Chen, Pierre-Louis Guhur, Cordelia Schmid, Ivan Laptev.
Vision-and-language navigation (VLN) aims to build autonomous visual agents that follow instructions and navigate in real scenes. To remember previously visited locations and actions taken, most approaches to VLN implement memory using recurrent states. In this work 14, we introduce a History Aware Multimodal Transformer (HAMT) to incorporate a long-horizon history into multimodal decision making. HAMT efficiently encodes all the past panoramic observations via a hierarchical vision transformer (ViT), which first encodes individual images with ViT, then models spatial relation between images in a panoramic observation and finally takes into account temporal relation between panoramas in the history. It, then, jointly combines text, history and current observation to predict the next action. We first train HAMT end-to-end using several proxy tasks including single step action prediction and spatial relation prediction, and then use reinforcement learning to further improve the navigation policy. HAMT achieves new state of the art on a broad range of VLN tasks, including VLN with fine-grained instructions (R2R, RxR), high-level instructions (R2R-Last, REVERIE), dialogs (CVDN) as well as long-horizon VLN (R4R, R2R-Back). We demonstrate HAMT to be particularly effective for navigation tasks with longer trajectories. Figure 16 presents qualitative results of our method.
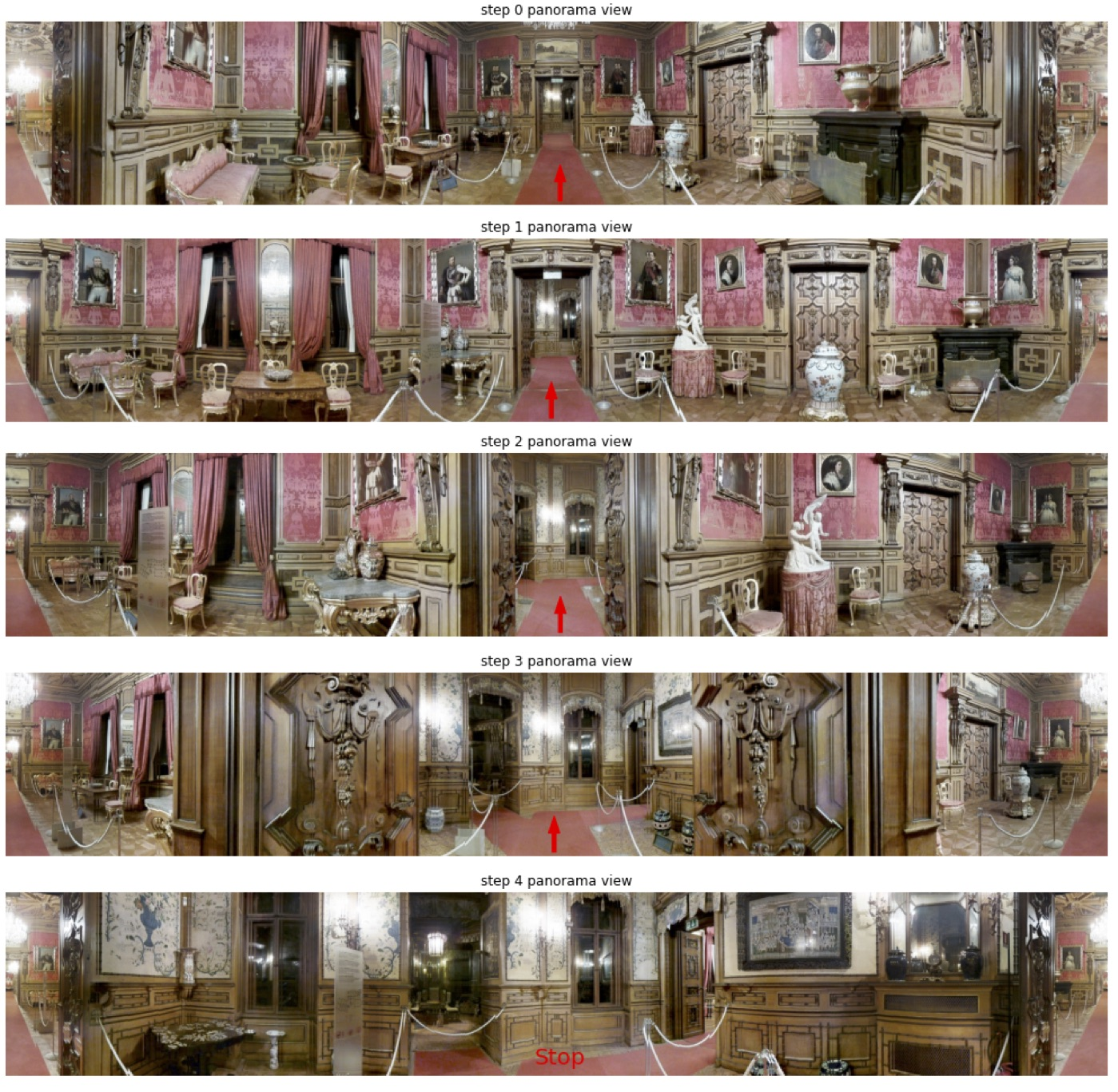
8.2.6 Differentiable simulation for physical system identification
Participants: Quentin Le Lidec, Igor Kalevatykh, Ivan Laptev, Cordelia Schmid, Justin Carpentier.
Simulating frictional contacts remains a challenging research topic in robotics. Recently, differentiable physics emerged and has proven to be a key element in model-based Reinforcement Learning (RL) and optimal control fields. However, most of the current formulations deploy coarse approximations of the underlying physical principles. Indeed, the classic simulators loose precision by casting the Nonlinear Complementarity Problem (NCP) of frictional contact into a Linear Complementarity Problem (LCP) to simplify computations. Moreover, such methods deploy non-smooth operations and cannot be automatically differentiated. In 3, we propose (i) an extension of the staggered projections algorithm for more accurate solutions of the problem of contacts with friction. Based on this formulation, we introduce (ii) a differentiable simulator and an efficient way to compute the analytical derivatives of the involved optimization problems. Finally, (iii) we validate the proposed framework with a set of experiments to present a possible application of our differentiable simulator. In particular, using our approach we demonstrate accurate estimation of friction coefficients and object masses both in synthetic and real experiments. An overview of our approach is presented in Figure 17.
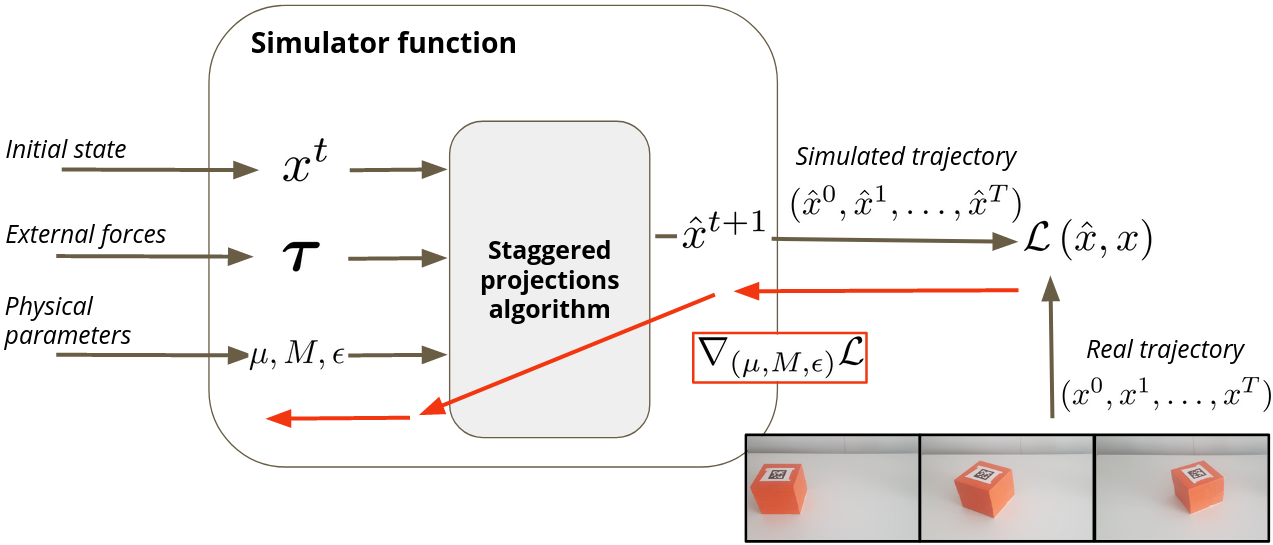
Overview of our differentiable simulator. The differentiability of the simulator allows to integrate it into a larger learning architecture and infer physical parameters such as friction coefficients μ\mu and mass MM of the objects, from real trajectories of these objects.
8.2.7 Leveraging Randomized Smoothing for Optimal Control of Nonsmooth Dynamical Systems
Participants: Quentin Le Lidec, Louis Montaut, Ivan Laptev, Cordelia Schmid, Justin Carpentier.
Optimal Control (OC) algorithms such as Differential Dynamic Programming (DDP) take advantage of the derivatives of the dynamics to efficiently control physical systems. Yet, in the presence of nonsmooth dynamical systems, such class of algorithms are likely to fail due, for instance, to the presence of discontinuities in the dynamics derivatives or because of non-informative gradient during the solving. On the contrary, Reinforcement Learning (RL) algorithms have shown better empirical results in scenarios exhibiting non-smooth effects (contacts, frictions, etc). Our approach in 46 leverages recent works on Randomized Smoothing (RS) to tackle non-smoothness issues commonly encountered in Optimal Control, and provides key insights on the interplay between RL and OC through the prism of RS methods, see Figure 18. This naturally leads us to introduce the Randomized Differential Dynamic Programming (R-DDP) algorithm accounting for deterministic but non-smooth dynamics in a very sample-efficient way. The experiments demonstrate that our method is able to solve classic robotic problems with dry friction and frictional contacts, where classical OC algorithms are likely to fail and RL algorithms require in practice a prohibitive number of samples to find an optimal solution.
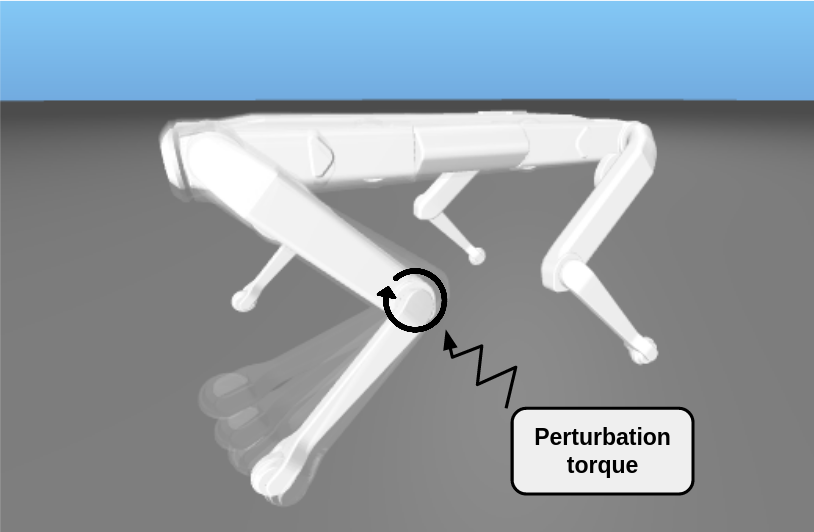
8.2.8 Implicit Differential Dynamic Programming
Participants: Wilson Jallet, Nicolas Mansard, Justin Carpentier.
Over the past decade, the Differential Dynamic Programming (DDP) method has gained in maturity and popularity within the robotics community. Several recent contributions have led to the integration of constraints within the original DDP formulation, hence enlarging its domain of application while making it a strong and easy-to-implement competitor against alternative methods of the state of the art such as collocation or multiple-shooting approaches. Yet, and similarly to its competitors, DDP remains unable to cope with high-dimensional dynamics within a receding horizon fashion, such as in the case of online generation of athletic motion on humanoid robots. In our work 19, we propose to make a step toward this objective by reformulating classic DDP as an implicit optimal control problem, allowing the use of more advanced integration schemes such as implicit or variational integrators. To that end, we introduce a primal-dual proximal Lagrangian approach capable of handling dynamic and path constraints in a unified manner, while taking advantage of the time sparsity inherent to optimal control problems. We show that his reformulation enables us to relax the dynamics along the optimization process by solving it inexactly: far from the optimality conditions, the dynamics are only partially fulfilled, but continuously enforced as the solver gets closer to the local optimal solution. This inexactness enables our approach to robustly handle large time steps (100 ms or more), unlike other state-of-the-art DDP solvers. We experimentally validate our approach inh different robotic scenarios. Some results of our method are illustrated in Figure 19.
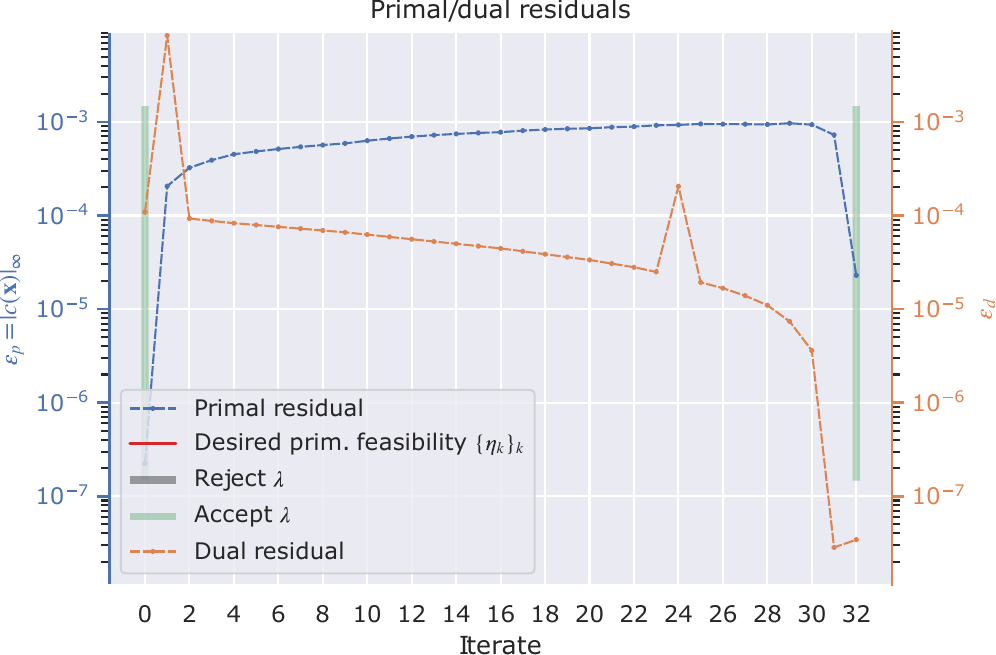
8.2.9 Fast and Robust Stability Region Estimation for Nonlinear Dynamical Systems
Participants: Eloïse Berthier, Justin Carpentier, Francis Bach.
A linear quadratic regulator can stabilize a nonlinear dynamical system with a local feedback controller around a linearization point, while minimizing a given performance criteria. An important practical problem is to estimate the region of attraction of such a controller, that is, the region around this point where the controller is certified to be valid. This is especially important in the context of highly nonlinear dynamical systems. In 10 we propose two stability certificates that are fast to compute and robust when the first, or second derivatives of the system dynamics are bounded. Associated with an efficient oracle to compute these bounds, this provides a simple stability region estimation algorithm compared to classic approaches of the state of the art. We experimentally validate that it can be applied to both polynomial and non-polynomial systems of various dimensions, including standard robotic systems, for estimating region of attractions around equilibrium points, as well as for trajectory tracking. This work is a joint contribution between Willow and Sierra teams.
8.3 Image restoration and enhancement
8.3.1 Lucas-Kanade Reloaded: End-to-End Super-Resolution from Raw Image Bursts
Participants: Bruno Lecouat, Jean Ponce, Julien Mairal.
In 23 we address the problem of reconstructing a high-resolution image from multiple lower-resolution snapshots captured from slightly different viewpoints in space and time. Key challenges for solving this super-resolution problem include (i) aligning the input pictures with sub-pixel accuracy, (ii) handling raw (noisy) images for maximal faithfulness to native camera data, and (iii) designing/learning an image prior (regularizer) well suited to the task. We address these three challenges with a hybrid algorithm building on the insight from Wronski et al. that aliasing is an ally in this setting, with parameters that can be learned end to end, while retaining the interpretability of classical approaches to inverse problems. The effectiveness of our approach is demonstrated on synthetic and real image bursts, setting a new state of the art on several benchmarks and delivering excellent qualitative results on real raw bursts captured by smartphones and prosumer cameras. Figure 20 presents some example results of our method.
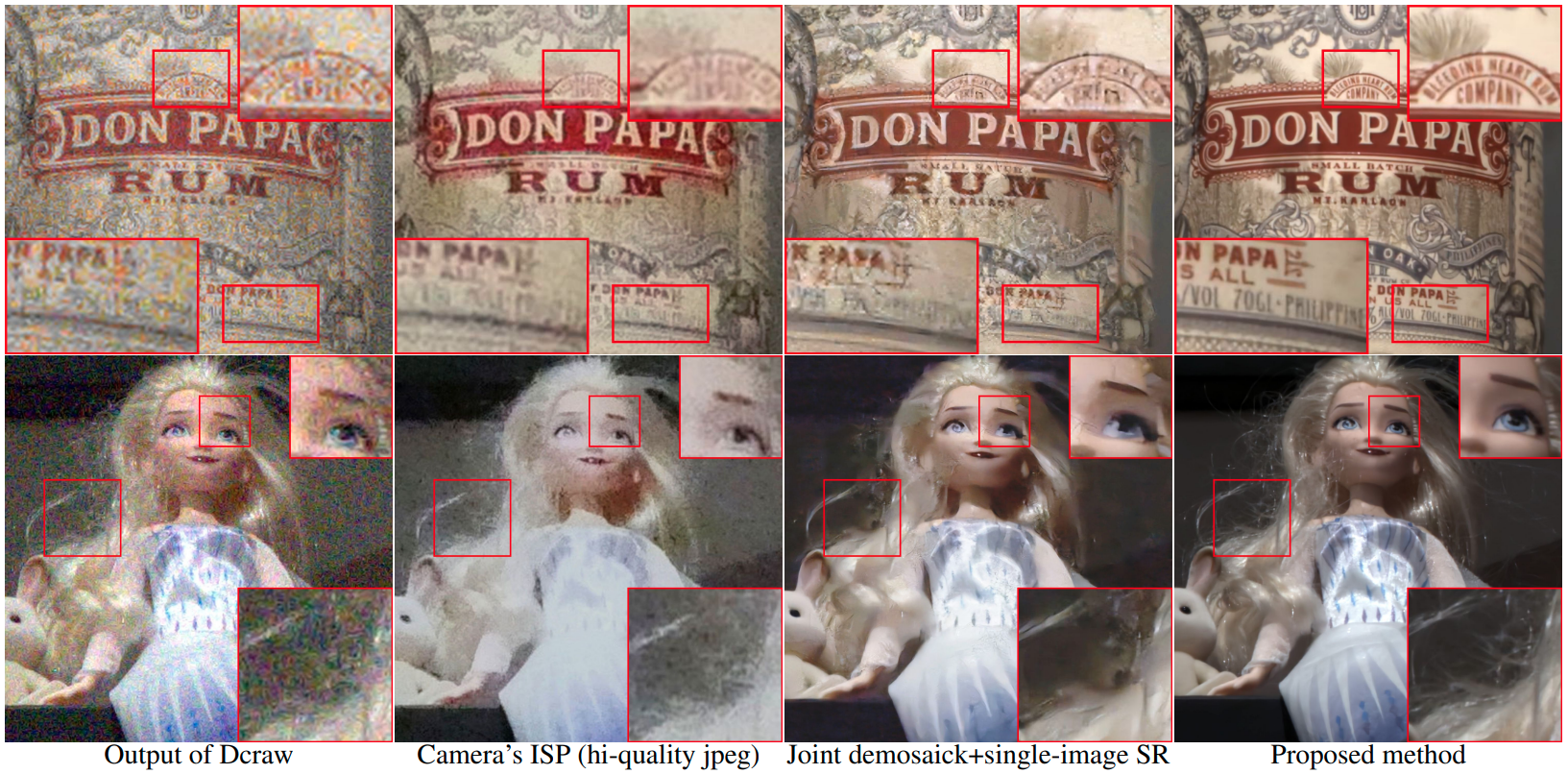
×\times 4 super-resolution results obtained from a burst of 30 raw images acquired with a handheld Panasonic Lumix GX9 camera at 12800 ISO for the top image and 25600 for the bottom image. Dcraw performs basic demosaicking.
9 Bilateral contracts and grants with industry
9.1 Bilateral contracts with industry
9.1.1 MSR-Inria joint lab: Image and video mining for science and humanities (Inria)
Participants: Yana Hasson, Ivan Laptev, Jean Ponce, Josef Sivic, Dimitri Zhukov, Cordelia Schmid.
This collaborative project brings together the WILLOW with MSR researchers in Zurich and elsewhere. The concept builds on several ideas articulated in the 2020 Science report, including the importance of data mining and machine learning in computational science. Rather than focusing only on natural sciences, however, we propose here to expand the breadth of e-science to include humanities and social sciences. The project we propose will focus on fundamental computer science research in computer vision and machine learning, and its application to archaeology, cultural heritage preservation, environmental science, and sociology, and it will be validated by collaborations with researchers and practitioners in these fields.
In 2018 a new agreement has been signed with a new focus on video understanding for personal assistants. The scientific objectives are to develop models, representations and learning algorithms for (i) automatic understanding of task-driven complex human activities from videos narrated with natural language in order to (ii) give people instructions in a new environment via an augmented reality device such as the Microsoft HoloLens. Besides the clear scientific interest of automatically understanding human activities in video streams, the main high-impact motivation of this project it to develop virtual assistants that may guide a child through simple games to improve his/her manipulation and language skills; help an elderly person to achieve everyday tasks; or facilitate the training of a new worker for highly-specialized machinery maintenance.
9.1.2 Louis Vuitton/ENS chair on artificial intelligence
Participants: Ivan Laptev, Jean Ponce, Josef Sivic.
The scientific chair Louis Vuitton - École normale supérieure in Artificial Intelligence has been created in 2017 and inaugurated on April 12, 2018 by the ENS Director Marc Mézard and the LV CEO Michael Burke. The goal of the chair is to establish a close collaboration between LV and ENS in the area of Artificial Intelligence. The chair enjoys the generous annual contribution of 200K Euros provided by LV in support of research activities in statistical learning and computer vision. In particular, the chair supports the costs of researchers, students, missions, computational resources as well as seminars and meetings, including the two days of meeting annually organized by LV and ENS. During 2020 ENS and LV have organized several joint meetings with the participation of researchers from SIERRA and WILLOW teams. The chair has also supported the hiring of one PhD student at the WILLOW team, missions to conferences and international research labs as well as data collection for research projects. In 2020 the chair has been extended to the next three-year period until 2023.
9.2 Bilateral grants with industry
9.2.1 Facebook AI Research Paris: Weakly-supervised interpretation of image and video data (Inria)
Participants: Jean Ponce, Minsu Cho, Ivan Laptev, Josef Sivic.
We will develop in this project (Facebook gift) new models of image and video content, as well as new recognition architectures and algorithms, to address the problem of understanding the visual content of images and videos using weak forms of supervision, such as the fact that multiple images contain instances of the same objects, or the textual information available in television or film scripts.
9.2.2 Google: Multimodal video representation with cross-modal learning (Inria)
Participants: Ivan Laptev.
The proposed project (Google gift) aims to learn a detailed correspondence between the text and the visual content of the video from large-scale unlabeled video collections. It will significantly extend current representations which rely on frame/clip based features and at best learn correlation based on transformers, but fail to provide the in-depth understanding of spatial and temporal structure of the visual content in the video. This will enable advanced multimodal video representations and hence will improve downstream tasks such as video captioning, search and summarization. The main challenge of the project is to build new state-of-the-art models and methods for self-supervised learning based on large-scale but imprecise textual information obtained from video transcripts and other video metadata. The project includes the collection of a dataset allowing a detailed analysis of the visual representation by extending the HowTo100Million dataset with manual annotations.
9.2.3 Google: Structured learning from video and natural language (Inria)
Participants: Simon Lacoste-Julien, Ivan Laptev, Josef Sivic.
People can easily learn how to change a flat tire of a car or assemble an IKEA shelve by observing other people doing the same task, for example, by watching a narrated instruction video. In addition, they can easily perform the same task in a different context, for example, at their home. This involves advanced visual intelligence abilities such as recognition of objects and their function as well as interpreting sequences of human actions that achieve a specific task. However, currently there is no artificial system with a similar cognitive visual competence. The goal of this proposal is to develop models, representations and learning algorithms for automatic understanding of complex human activities from videos narrated with natural language.
10 Partnerships and cooperations
10.1 International initiatives
10.1.1 Associate team GAYA
Participants: Jean Ponce, Cordelia Schmid.
GAYA is a joint research team bringing together two Inria project-teams (Thoth, Grenoble and WILLOW, Paris) and Carnegie Mellon University, USA. It focuses on two research themes: (i) semantic structured interpretation of videos, and (ii) studying the geometric properties of object shapes to enhance state-of-the-art object recognition approaches.
Interpreting videos semantically in a general setting, involving various types of video content like home video clips, news broadcasts, feature films, which contain a lot of clutter, non-rigid motion, many “actors” performing actions, person-object and person-person interactions, varying viewpoints, is challenging. This task is being examined increasingly over the past decade, with the availability of large video resources, e.g., YouTube. Despite this progress, an effective video representation for recognizing actions is still missing. To address this critical challenge, we propose a joint optimization framework, wherein we learn the video representation and also develop models for action recognition. Specifically, we aim to exploit the spatio-temporal relations among pixels in a video through graphical models and novel deep learning feature representations.
The second research theme explores geometric aspects of computer vision, in particular how to model three-dimensional objects from their two-dimensional projections, and how the appearance of these objects evolves with changes in viewpoint. Beyond its theoretical interest, this work is critical for developing object recognition algorithms that take into account the three-dimensional nature of the visual world and go beyond the template-matching approaches dominant today. Duality is an important concept in this area, and we are investigating its application to the construction of visual hulls as well as the characterization of the topology of image contours using the Gauss map. Existing results are essentially limited to the Euclidean setting, and we are investigating their generalization to the general projective case.
Partners: CMU (Deva Ramanan, Martial Hebert, Abhinav Gupta, Pavel Tokmakov), INRIA Thoth (Karteek Alahari).
10.2 International research visitors
Most of our international visits in 2021 have been cancelled due to the COVID-19 pandemic. We have nevertheless continued close remote collaboration with universities and companies including CTU in Prague (J. Sivic), DeepMind in London (J.-B. Alayrac, A. Miech, A. Zisserman), POSTECH in Pohang (M. Cho), Xi’an Jiaotong University in Xi’an (J. Sun) and Yonsei University in Seoul (B. Ham, B. Kim). Moreover, J. Ponce spends most of his time at New York University.
10.3 European initiatives
10.3.1 IMPACT: Intelligent machine perception
Participants: Joaef Sivic, Jean Ponce, Ivan Laptev.
IMPACT is a collaborative project with Czech Technical University, Center for Robotics, Informatics and Cybernetics (CIIRC) (2017-2023). The IMPACT project focuses on fundamental and applied research in computer vision, machine learning and robotics to develop machines that learn to perceive, reason, navigate and interact with complex dynamic environments. For example, people easily learn how to change a flat tire of a car or perform resuscitation by observing other people doing the same task. This involves advanced visual intelligence abilities such as interpreting sequences of human actions that manipulate objects to achieve a specific task. Currently, however, there is no artificial system with a similar level of cognitive visual competence. Breakthrough progress in intelligent machine perception will have profound implications on our everyday lives as well as science and commerce, with smart assistive robots that automatically learn new skills from the Internet, safer cars that autonomously navigate in difficult changing conditions, or intelligent glasses that help people navigate never seen before environments.
10.4 National initiatives
10.4.1 PRAIRIE
Participants: Ivan Laptev, Jean-Paul Laumond, Jean Ponce, Cordelia Schmid.
The Prairie Institute (PaRis AI Research InstitutE) is one of the four French Institutes for Interdisciplinary Artificial Intelligence Research (3IA), which were created as part of the national French initiative on AI announced by President Emmanuel Macron on May 29, 2018. It brings together five academic partners (CNRS, Inria, Institut Pasteur, PSL University, and University of Paris) as well as 17 industrial partners, large corporations which are major players in AI at the French, European and international levels, as well as 45 Chair holders, including four of the members of WILLOW (Laumond, Laptev, Ponce, Sivic). Ponce is the scientific director of PRAIRIE.
10.4.2 VideoPredict: Predicting future video content
Participants: Cordelia Schmid, Jean Ponce.
Predicting future video content is a challenging problem with high potential impact in downstream tasks such as self-driving cars and robotics, but also much promise for the learning process itself, from self-supervised learning to data augmentation. Existing approaches range from predicting future actions with semantic labels to creating realistic renderings of future frames. Most of them use straight predictions from convolutional features of previous frames. We propose instead to model the causality effects involved in the video formation process, and disentangle motion and appearance factors. This will result in better prediction, but also and maybe more importantly in a better, more structured understanding of the video content, leading to explicable and interpretable results, and eventually to more trustworthy learning systems. The German and French partners are, respectively, experts in machine learning and computer vision, with complementary research threads in causality and disentangled data models on the one hand, and video understanding and action recognition on the other hand, that are ideally suited for this collaborative project
11 Dissemination
11.1 Promoting scientific activities
11.1.1 Scientific events: organisation
- I. Laptev, co-organizer of Machines Can See on-line summit on computer vision and machine learning, July 2021.
- J.P. Laumond, main organizer of the conference Mythes et machines - Robotique et Intelligence Artificielle : penser la technologie aujourd’hui, Académie des Sciences, Paris, November 2021.
- J.P. Laumond, main organizer of the workshop Robotics and Optimal Control, IHP Paris, December 2021.
- J. Ponce, co-organizer of the French-German workshop on Machine Learning, May 10-11, 2021.
- J. Ponce, co-organizer of PRAIRIE workshop, Paris, Nov. 10, 2021.
- C. Schmid, co-organizer of the workshop, Structured Representations for Video Understanding, in conjunction with ICCV 2021, virtual, October 2021.
- C. Schmid was co-organizer of the Ellis Workshop for Computer Vision and Machine Learning, virtual, July 2021.
11.1.2 Scientific events: selection
Area chairs
- IEEE Conference on Computer Vision and Pattern Recognition (CVPR), 2021 (I. Laptev).
- International Conference on Computer Vision (ICCV), 2021 (C. Schmid).
- Conference and Workshop on Neural Information Processing Systems (NeurIPS), 2021 (C. Schmid).
Member of the conference program committees / Reviewer
- IEEE Conference on Computer Vision and Pattern Recognition (CVPR), 2021 (S. Chen, A. El-Nouby, P.-L. Guhur, Y. Hasson, Y. Labbe, J. Sivic, R. Strudel, V.-H. Vo).
- International Conference on Computer Vision (ICCV), 2021 (S. Chen, A. El-Nouby, Y. Hasson, Y. Labbe, J. Sivic).
- International Conference on Learning Representations (ICLR), 2021 (R. Strudel).
- International Conference on Intelligent Robots and Systems (IROS), 2021 (Y. Labbe).
- International Conference on Robotics and Automation (ICRA), 2021 (M. Alakuijala).
11.1.3 Journal
Member of the editorial boards
- International Journal of Computer Vision (I. Laptev, J. Ponce, J. Sivic).
- Foundations and Trends in Computer Graphics and Vision (J. Ponce).
Reviewer - reviewing activities
- International Journal of Computer Vision (T. Eboli).
- IEEE Transactions on Pattern Analysis and Machine Intelligence (T. Eboli, V.-H. Vo).
11.1.4 Invited talks
- M. Alakuijala, Invited talk, MIPT-UGA young researchers workshop, July 2021.
- J. Carpentier, Invited talk, Bordeaux Robotics Workshop, Bordeaux, Janurary 2021.
- J. Carpentier, Invited talk, Covariant, Los Angeles, June 2021.
- J. Carpentier, Invited talk, Toyota Research Institute, Boston, August 2021
- J. Carpentier, Pinocchio: a universal framework for robotics and beyond, Pal Robotics, Barcelona, October 2021.
- J. Carpentier, Invited talk, DEFROST, INRIA Lille, November 2021.
- J. Carpentier, Invited talk, IRCOM conference, Iran, November 2021.
- J. Carpentier, Invited talk, Prairie Workshop, Paris, November 2021.
- S. Chen, Invited talk at ICCV 2021 Workshop Human Interaction for Robotic Navigation
- I. Laptev, Invited talk, CVPR Workshop Long-form Video Understanding, June 2021.
- I. Laptev, Invited talk, ELLIS Workshop, July 2021.
- I. Laptev, Invited talk, RAAI Summer School, July 2021.
- I. Laptev, Invited talk, Conseild'Etat, July 2021.
- I. Laptev, Invited speaker, International Conference on Information Technology and Nanotechnology, September 2021.
- I. Laptev, Invited talk, INRIA Grenoble, September 2021.
- I. Laptev, Invited talk, Ecole navale, Brest, October 2021.
- J. Ponce, Invited talk, Belgian AI week, March 2021.
- J. Ponce, Invited talk, Idemia, Paris, 2021.
- J. Ponce, Invited talk, NYU Capstone seminar, 2021.
- J. Ponce, Invited talk, Ecole navale, Brest, October 2021.
- C. Schmid, Invited speaker at Prairie workshop, Paris, November 2021.
- C. Schmid, Keynote ACM Multimedia 2021, virtual, October 2021.
- C. Schmid, Invited speaker at 4th Workshop on Closing the Loop Between Vision and Language, in conjunction with ICCV’21, virtual, October 2021.
- C. Schmid, Invited speaker at 2nd Autonomous Vehicle Vision Workshop, in conjunction with ICCV’21, virtual, October 2021.
- C. Schmid, Invited speaker at Workshop on Computer Vision for the Factory Floor, in conjunction with ICCV’21, virtual, October 2021.
- C. Schmid, Invited lecture at Pairie artificial intelligence summer school (PAISS), virtual, July 2021.
- C. Schmid, Invited speaker at Workshop on Large-Scale Holistic Video Understanding, in conjunction with CVPR’21, virtual, June 2021.
- C. Schmid, Invited speaker at Frontiers of Monocular 3D Perception workshop, in conjunction with CVPR’21, virtual, June 2021.
- C. Schmid, Invited speaker at 2nd Comprehensive Tutorial in Video Modeling, in conjunction with CVPR’21, virtual, June 2021.
- C. Schmid, Invited speaker at French-German Machine Learning Symposium, virtual, May 2021.
- C. Schmid, Turing lecture, virtual, May 2021.
- C. Schmid, Talk at Academie des technologies, virtual, March 2021.
- C. Schmid, Talk at EPFL Center of Intelligent Systems - CIS Colloquium, virtual, March 2021.
- J. Sivic, Invited talk, 2nd International Workshop on AI for Robotics Naver Labs Europe, 2021.
- J. Sivic, Invited talk, ICCV Workshop on Structured Representations for Video Understanding, 2021.
- J. Sivic, Invited talk, AI Journey Conference, Moscow, 2021;
- J. Sivic, Invited seminar, ELLIS Unit Nijmegen, NL 2021.
- J. Sivic, Invited seminar, Samsung AI Center, Cambridge, UK 2021.
- J. Sivic, Invited seminar, ANITI Toulouse AI Institute seminar, 2021.
- V.-H. Vo, Invited talk, ENPC, December 2021.
- A. Yang, Invited talk, CVPR Holistic Video Understanding Workshop, June 2021.
11.1.5 Leadership within the scientific community
- Member, advisory board, Computer Vision Foundation (J. Sivic).
- Board Member Deputy, European Laboratory for Learning and Intelligent Systems (J. Sivic).
- Global Member of the Bavarian AI Council (J. Sivic).
- Member of TPAMI EIC search committee (C. Schmid).
- Helmholtz award committee (C. Schmid).
- Member of Technical Activities Board for International Foundation of Robotics Research (C. Schmid).
- Member of Scientific Advisory Committee of the Helmholtz AI Cooperation Unit (C. Schmid).
- Member of scientific advisory board for the German Competence Centers for AI Research (C. Schmid).
- Director of Ellis program on Computer Vision and Machine Learning (C. Schmid).
- Member of board of directors of the Computer Vision Foundation (CVF) (C. Schmid)..
- Member of the PAMI-TC executive committee (C. Schmid).
- Member of the PAMI-TC awards committee (C. Schmid).
11.1.6 Scientific expertise
- J. Ponce, coordinator of the AI theme for the joint French-American Committee on Science and Technology, 2018–.
- I. Laptev, head of scientific board at VisionLabs, 2019–.
11.1.7 Research administration
- Member, INRIA Cordi-S and postdoc selection committee, 2019— (I. Laptev).
- Member, INRIA Commission des emplois scientifiques (CES), 2019— (I. Laptev).
11.2 Teaching - Supervision - Juries
11.2.1 Teaching
- Master: M. Aubry, K. Alahari, I. Laptev and J. Sivic "Introduction to computer vision", M1, Ecole normale supérieure, 36h.
- Master: I. Laptev, J. Ponce, J. Sivic and C. Schmid "Object recognition and computer vision", M2, Ecole normale superieure, and MVA, Ecole normale superieure Paris-Saclay, 36h.
- Master: J-P. Laumond and J. Carpentier, "Robotics", M1 MPRI, Ecole normale supérieure and Ecole normale superieure Paris-Saclay, 48h.
- Master: J. Ponce, Introduction to computer vision to medical students, 15h.
- Master: J. Ponce, Introduction to computer vision, NYU.
- Master: I. Laptev, Fundamentals of Machine Learning, Master IASD, PSL University, 9h.
- Master: J-P. Laumond, "Robotics", Ecole des Mines de Paris, 4h.
- P.-L. Guhur, Reinforcement Learning, EPITA.
- P.-L. Guhur, Data Science for Business, EM Lyon.
- A. Yang, Teacher Assistant, Differential Equations, Sorbonne, 38h.
- A. Yang, Teacher Assistant, Differential Equations, Sorbonne, 38h.
- J. Sivic, Three lectures (3 x 1.5h) in the 3D computer vision class of V. Hlavac at Charles University in Prague.
- J. Sivic, Lecture on multi-modal self-supervised learning, Eastern European Summer School, 2021.
11.2.2 Supervision
- PhD in progress : Nicolas Chahine, started in Aug 2021, J. Ponce.
- PhD in progress : Matthieu Futeral-Peter, started in Nov 2021, I. Laptev and C. Schmid.
- PhD in Progress : Wilson Jallet, started in March 2021, J. Carpentier.
- PhD in progress : Guillaume Le Moing, “Learning robust representations for improved visual understanding”, started in Nov 2020, J. Ponce and C. Schmid.
- PhD in progress : Antoine Bambade, started in Oct. 2020, J. Carpentier, A. Taylor (Sierra) and J. Ponce.
- PhD in progress : Adrien Bardes, started in Oct. 2020, J. Ponce.
- PhD in progress : Oumayma Bounou, started in Oct. 2020, J. Ponce and J. Carpentier.
- PhD in progress : Antoine Yang, “Multimodal video representation with cross-modal learning”, started in Oct. 2020, I. Laptev, C. Schmid, J. Sivic.
- PhD in progress : Elliot Chane-Sane, “Learning long-horizon robotics manipulations”, started in Oct. 2020, I. Laptev and C. Schmid.
- PhD in progress : Yann Dubois De Mont-Marin, started in Sept. 2020, J.-P. Laumond.
- PhD in progress : Alaaeldin Ali, “Object centric visual retrieval in the wild”, started in Aug. 2020, I. Laptev.
- PhD in progress : Vo Van Huy, started in Dec 2018, J. Ponce.
- PhD in progress : Pierre-Louis Guhur, “Learning Visual Language Manipulation”, started in Oct 2019, I. Laptev and C. Schmid.
- PhD in progress : Aamr El Kazdadi, started in Oct 2019, J. Carpentier and J. Ponce.
- PhD in progress : Bruno Lecouat, started in Sept 2019, J. Ponce and J. Mairal (Inria Grenoble).
- PhD in progress : Robin Strudel, “Learning and transferring complex robot skills from human demonstrations”, started in Oct 2018, I. Laptev, C. Schmid and J. Sivic.
- PhD in progress : Yann Labbe, “Generalizing robotic sensorimotor skills to new tasks and environments”, started in Oct 2018, J. Sivic and I. Laptev.
- PhD in progress : Minttu Alakuijala, started in Feb 2019, J. Ponce and C. Schmid.
- PhD in progress : Thomas Eboli, graduated in Sept 2021, J. Ponce.
- PhD in progress : Zongmian Li, “Learning to manipulate objects from instructional videos”, started in Oct 2017, I. Laptev, J. Sivic and N. Mansard (LAAS/CNRS, Toulouse).
- PhD in progress : Yana Hasson, “Reconstruction and recognition of hand-object manipulations”, graduated in Oct 2021, I. Laptev and C. Schmid.
- PhD in progress : Alexander Pashevich, “Learning to grasp”, Graduated in Sept 2021, C. Schmid.
- PhD in progress : Ronan Riochet, “Unsupervised Learning of Intuitive Physics from Videos”, graduated in June 2021, E. Dupoux, I. Laptev and J. Sivic.
- PhD in progress : Dmitry Zhukov, “Learning from instruction videos for personal assistants”, graduated in Dec 2021, I. Laptev and J. Sivic.
11.2.3 Juries
- PhD thesis committee:
- W. Liu, Swiss Federal Institute of Technology Lausanne, 2021 (I. Laptev, rapporteur).
- V. Sydorov, Université Grenoble Alpes, 2021 (I. Laptev, rapporteur).
- Y. Wang, Université Côte d’Azur, 2021 (I. Laptev, rapporteur).
- A. Pashevich, Université Grenoble Alpes, 2021 (I. Laptev, examiner).
- M. Caron, Université Grenoble Alpes, 2021 (C. Schmid).
- C. Kervadec, University of Lyon, 2021 (C. Schmid).
- Yang Xiao, Ecole des Ponts ParisTech (J. Sivic, rapporteur).
- Othman Sbai, Ecole des Ponts ParisTech (J. Sivic, examinateur).
- Hugo Germain, Ecole des Ponts ParisTech (J. Sivic, examinateur).
- HDR committee:
- JS Franco, University of Grenoble, 2021 (C. Schmid).
11.3 Popularization
J.P. Laumond is the scientific curator of the permanent exhibition at Cité des Sciences et de l'Industrie. The aim of this exhibition is to help the general audience to understand the concepts of robotics. Indeed, the notion of robotics today is packed with many preconceived notions, phobias, and utopias, all fed by literature and a rich film culture. The real challenge of the exhibition is the presentation of authentic working robots that raises awareness of our relationship to these singular machines. How do they work? What are they for? What are their performances today and what will they be tomorrow? The exhibition lays bare the actual capabilities of robots and provides insight into the current issues.
12 Scientific production
12.2 Publications of the year
International journals
International peer-reviewed conferences
Doctoral dissertations and habilitation theses
Reports & preprints