Keywords
Computer Science and Digital Science
- A1.3.2. Mobile distributed systems
- A1.5.2. Communicating systems
- A2.3.1. Embedded systems
- A3.4.1. Supervised learning
- A3.4.2. Unsupervised learning
- A3.4.3. Reinforcement learning
- A3.4.4. Optimization and learning
- A3.4.5. Bayesian methods
- A3.4.6. Neural networks
- A3.4.8. Deep learning
- A5.1. Human-Computer Interaction
- A5.4.2. Activity recognition
- A5.4.4. 3D and spatio-temporal reconstruction
- A5.4.5. Object tracking and motion analysis
- A5.4.6. Object localization
- A5.4.7. Visual servoing
- A5.10.2. Perception
- A5.10.3. Planning
- A5.10.4. Robot control
- A5.10.5. Robot interaction (with the environment, humans, other robots)
- A5.10.6. Swarm robotics
- A5.10.7. Learning
- A5.11.1. Human activity analysis and recognition
- A6.1.2. Stochastic Modeling
- A6.1.3. Discrete Modeling (multi-agent, people centered)
- A6.2.3. Probabilistic methods
- A6.2.6. Optimization
- A6.4.1. Deterministic control
- A6.4.2. Stochastic control
- A6.4.3. Observability and Controlability
- A6.4.6. Optimal control
- A8.2. Optimization
- A8.2.1. Operations research
- A8.2.2. Evolutionary algorithms
- A8.11. Game Theory
- A8.12. Optimal transport
- A9.2. Machine learning
- A9.5. Robotics
- A9.6. Decision support
- A9.7. AI algorithmics
- A9.9. Distributed AI, Multi-agent
- A9.10. Hybrid approaches for AI
Other Research Topics and Application Domains
- B5.2.1. Road vehicles
- B5.6. Robotic systems
- B7.1.2. Road traffic
- B8.4. Security and personal assistance
1 Team members, visitors, external collaborators
Research Scientists
- Ozgur Erkent [Inria, Starting Research Position]
- Christian Laugier [Inria, Emeritus, HDR]
- Agostino Martinelli [Inria, Researcher, until Jun 2020]
- David Sierra Gonzalez [Inria, Starting Research Position]
Faculty Members
- Olivier Simonin [Team leader, INSA Lyon, Professor, HDR]
- Jilles Dibangoye [INSA Lyon, Associate Professor]
- Samba Ndojh Ndiaye [Univ Claude Bernard, Associate Professor]
- Christine Solnon [INSA Lyon, Professor, from Feb 2020, HDR]
- Anne Spalanzani [Univ Grenoble Alpes, Associate Professor, HDR]
Post-Doctoral Fellows
- Lotfi Abdi [Inria, until Aug 2020]
- Andres Gomez Hernandez [Inria, until Oct 2020]
- Mohamad Hobballah [INSA Lyon, until Feb 2020]
- Luc Libralesso [Université Clermont Auvergne, from Oct 2020]
PhD Students
- Edward Beeching [Inria]
- Alexandre Bonnefond [Inria]
- Guillaume Bono [INSA Lyon, until Oct 2020]
- Esteban Carvalho [Univ Grenoble Alpes]
- Aurelien Delage [INSA Lyon, from Oct 2020]
- Niranjan Deshpande [Inria]
- Manuel Diaz Zapata [Inria, from Nov 2020]
- Romain Fontaine [INSA Lyon, from Oct 2020]
- Remy Grunblatt [Inria]
- Maria Kabtoul [Inria, until Mar 2020]
- Xiao Peng [INSA Lyon, from Oct 2020]
- Mihai-Ioan Popescu [INSA ]
- Manon Prédhumeau [Univ Grenoble Alpes]
- Benoit Renault [INSA Lyon]
- Loic Rouquette [INSA Lyon]
- Luiz Serafim Guardini [Renault, CIFRE]
- Samuele Zoboli [INSA Lyon, from Sep 2020]
Technical Staff
- David Albert [INSA Lyon, Engineer, from Nov 2020]
- Rabbia Asghar [Inria, Engineer]
- Jean-Alix David [Inria, Engineer, until Mar 2020]
- Vincent Dufour [Inria, Engineer, from Nov 2020]
- Thomas Genevois [Inria, Engineer]
- Andres Gomez Hernandez [Inria, Engineer, from Nov 2020]
- Andres Gonzalez Moreno [Inria, Engineer, from Nov 2020]
- Vincent Le Doze [Inria, Engineer]
- Jerome Lussereau [Inria, Engineer]
- Anshul Paigwar [Inria, Engineer]
- Alessandro Renzaglia [Inria, Engineer]
- Lukas Rummelhard [Inria, Engineer, from Feb 2020]
- Alexis Saget [Inria, Engineer, from Nov 2020]
- Amrita Suresh [Inria, Engineer, from Nov 2020]
- Stephane d'Alu [INSA Lyon, Engineer]
Interns and Apprentices
- David Albert [INSA Rouen, from Mar 2020 until Sep 2020]
- Maxime Bernard [Inria, from May 2020 until Aug 2020]
- Aurelien Delage [INSA Lyon, from Mar 2020 until Aug 2020]
- Manuel Diaz Zapata [Inria, from Feb 2020 until Jul 2020]
- Christopher Hunt Rubinstein [Inria, from Feb 2020 until Jun 2020]
- Damien Jauneau [Inria, from Feb 2020 until Aug 2020]
- Kseniia Menzorova [Univ Grenoble Alpes, from Feb 2020 until Aug 2020]
- Unmesh Patil [Inria, from Oct 2020]
- Vlaldislav Shlenskii [Institut polytechnique de Grenoble, from Feb 2020 until Aug 2020]
- Yuxuan Xie [INSA Lyon, until Jun 2020]
Administrative Assistant
- Anouchka Ronceray [Inria]
External Collaborators
- Fabrice Jumel [École supérieure de chimie physique électronique de Lyon]
- Jacques Saraydaryan [École supérieure de chimie physique électronique de Lyon]
2 Overall objectives
2.1 Origin of the project
Chroma is a bi-localized project-team at Inria Grenoble Rhône-Alpes in Grenoble and Lyon cities. The project was launched at the beginning of the year 2015 (March) before it became an Inria project-team on December 1st, 2017. It brings together experts in perception and decision-making for mobile robotics, all of them sharing common approaches that mainly relate to the field of Artificial Intelligence. It was originally founded by members of Inria project-team eMotion (2002-2014) led by Christian Laugier and teacher-researchers from INSA Lyon 1 working in the robotic group led by Prof. Olivier Simonin in CITI Lab. 2 (since 2013). Earlier members include Olivier Simonin (Prof. INSA Lyon), Christian Laugier (Inria researcher DR1), Jilles Dibangoye (Asso. Prof. INSA Lyon) and Agostino Martinelli (Inria researcher CR1). On December 2015, Anne Spalanzani (Asso. Prof. Univ. Grenoble, HDR) joined the team (she was previously member of eMotion and Prima Inria project-teams). On January 2020, Christine Solnon (Prof. INSA Lyon) joined the team, thanks to her transfert from LIRIS lab. to CITI lab.
The overall objective of Chroma is to address fundamental and open issues that lie at the intersection of the emerging research fields called “Human Centered Robotics” 3 and “Multi-Robot Systems 4".
More precisely, our goal is to design algorithms and develop models allowing mobile robots to navigate and cooperate in dynamic and human-populated environments. Chroma is involved in all decision aspects pertaining to single and multi robot navigation tasks, including perception and motion-planning.
The general objective is to build robotic behaviors that allow one or several robots to operate safely among humans in partially known environments, where time, dynamics and interactions play a significant role. Recent advances in embedded computational power, sensor and communication technologies, and miniaturized mechatronic systems, make the required technological breakthroughs possible (including from the scalability point of view).
Chroma is clearly positioned in the "Artificial Intelligence and Autonomous systems" research theme of the Inria 2018-2022 Strategic Plan. More specifically we refer to the "Augmented Intelligence" challenge (connected autonomous vehicles) and to the "Human centred digital world" challenge (interactive adaptation).
2.2 Research themes
To address the mentionned challenges, we take advantage of recent advances in all: probabilistic methods, machine learning, planning techniques, multi-agent decision making, and constraint programming. We also draw inspiration from other disciplines such as Sociology to take into account human models.
Two main research themes of mobile robotics are addressed : i) Perception and Situation Awareness ii) Navigation and Cooperation in Dynamic Environments. Next, we elaborate more about these themes.
-
Perception and Situation Awareness.
This theme aims at understanding complex dynamic scenes, involving mobile objects and human beings, by exploiting prior knowledge and streams of perceptual data coming from various sensors.
To this end, we investigate three complementary research problems:
- Bayesian Perception: How to take into account prior knowledge and uncertain sensory data in a dynamic context?
- Situation awareness : How to interpret the perceived scene and to predict their likely future motion (including near future collision risk) ?
- Robust state estimation: acquire a deep understanding on several sensor fusion problems and investigate their observability properties in the case of unknown inputs.
-
Navigation and Cooperation in Dynamic Environments.
This theme aims at designing models and algorithms allowing robots to move and to coordinate efficiently in dynamic environments.
We focus on two problems: navigation in human-populated environment (social navigation) and cooperation in large distributed fleet of robots (scalability and robustness issues).
- Motion-planning in human-populated environment. How to plan trajectories that take into account the uncertainty of human-populated environments and respect the social rules of human beings? Such a challenge requires models of human behavior to be learnt or designed as well as dedicated learning or planning algorithms.
- Multi-robot decision making in complex environments. How to design models and algorithms that can achieve both scalability and performance guarantees in real-world robotic systems? Our methodology builds upon advantages of three complementary approaches, Multi-Agent Sequential Decision Making (MA-SDM), Swarm Intelligence (SI), and Constraint programming (CP).
Chroma is also concerned with applications and transfer of the scientific results. Our main applications include autonomous and connected vehicles, service robotics, search&rescue tasks with ground and aerial robots. Chroma is currently involved in several projects in collaboration with automobile companies (Renault, Toyota and Volvo) and some startups.
3 Research program
3.1 Introduction
The Chroma team aims to deal with different issues of autonomous mobile robotics : perception, decision-making and cooperation. Figure 1 schemes the different themes and sub-themes investigated by Chroma.
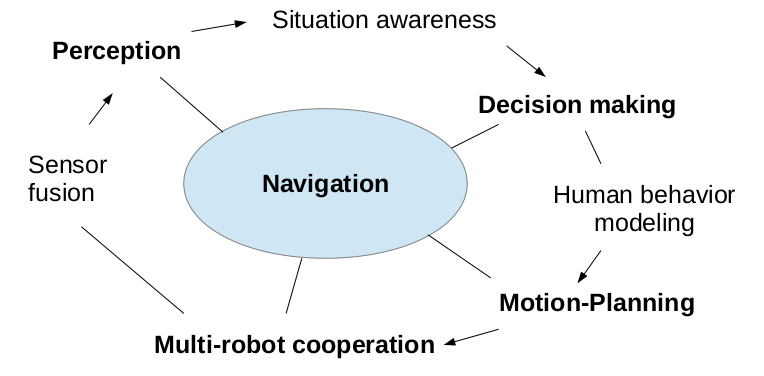
We present here after our approaches to address these different themes of research, and how they combine altogether to contribute to the general problem of robot navigation. Chroma pays particular attention to the problem of autonomous navigation in highly dynamic environments populated by humans and cooperation in multi-robot systems. We share this goal with other major robotic laboratories/teams in the world, such as Autonomous Systems Lab at ETH Zurich, Robotic Embedded Systems Laboratory at USC, KIT 5 (Prof Christoph Stiller lab and Prof Ruediger Dillmann lab), UC Berkeley, Vislab Parma (Prof. Alberto Broggi), and iCeiRA 6 laboratory in Taipei, to cite a few. Chroma collaborates at various levels (visits, postdocs, research projects, common publications, etc.) with most of these laboratories, see Section 9.2.
3.2 Perception and Situation Awareness
Robust perception in open and dynamic environments populated by human beings is an open and challenging scientific problem. Traditional perception techniques do not provide an adequate solution for these problems, mainly because such environments are uncontrolled 7 and exhibit strong constraints to be satisfied (in particular high dynamicity and uncertainty). This means that the proposed solutions have to simultaneously take into account characteristics such as real time processing, temporary occultations, dynamic changes or motion predictions.
Bayesian perception
Context. Perception is known to be one of the main bottlenecks for robot motion autonomy, in particular when navigating in open and dynamic environments is subject to strong real-time and uncertainty constraints. In order to overcome this difficulty, we have proposed in the scope of the former e-Motion team, a new paradigm in robotics called “Bayesian Perception”. The foundation of this approach relies on the concept of “Bayesian Occupancy Filter (BOF)” initially proposed in the Ph.D. thesis of Christophe Coue 61 and further developed in the team 8. The basic idea is to combine a Bayesian filter with a probabilistic grid representation of both the space and the motions. It allows the filtering and the fusion of heterogeneous and uncertain sensors data, by taking into account the history of the sensors measurements, a probabilistic model of the sensors and of the uncertainty, and a dynamic model of the observed objects motions.
In the scope of the Chroma team and of several academic and industrial projects (in particular the IRT Security for autonomous vehicle and Toyota projects), we went on with the development and the extension under strong embedded implementation constraints, of our Bayesian Perception concept. This work has already led to the development of more powerful models and more efficient implementations, e.g. the CMCDOT (Conditional Monte Carlo Dense Occupancy Tracker) framework 82 which is still under development.
This work is currently mainly performed in the scope of the “Security for Autonomous Vehicle (SAV)” project (IRT Nanoelec), and more recently in cooperation with some Industrial Companies (see section New Results for more details on the non confidential industrial cooperation projects).
Objectives. We aim at defining a complete framework extending the Bayesian Perception paradigm to the object level. The main objective is to be simultaneously more robust, more efficient for embedded implementations, and more informative for the subsequent scene interpretation step (Figure 2.a illustrates). Another objective is to improve the efficiency of the approach (by exploiting the highly parallel characteristic of our approach), while drastically reducing important factors such as the required memory size, the size of the hardware component, its price and the required energy consumption. This work is absolutely necessary for studying embedded solutions for the future generation of mobile robots and autonomous vehicles. We also aim at developing strong partnerships with non-academic partners in order to adapt and move the technology closer to the market.
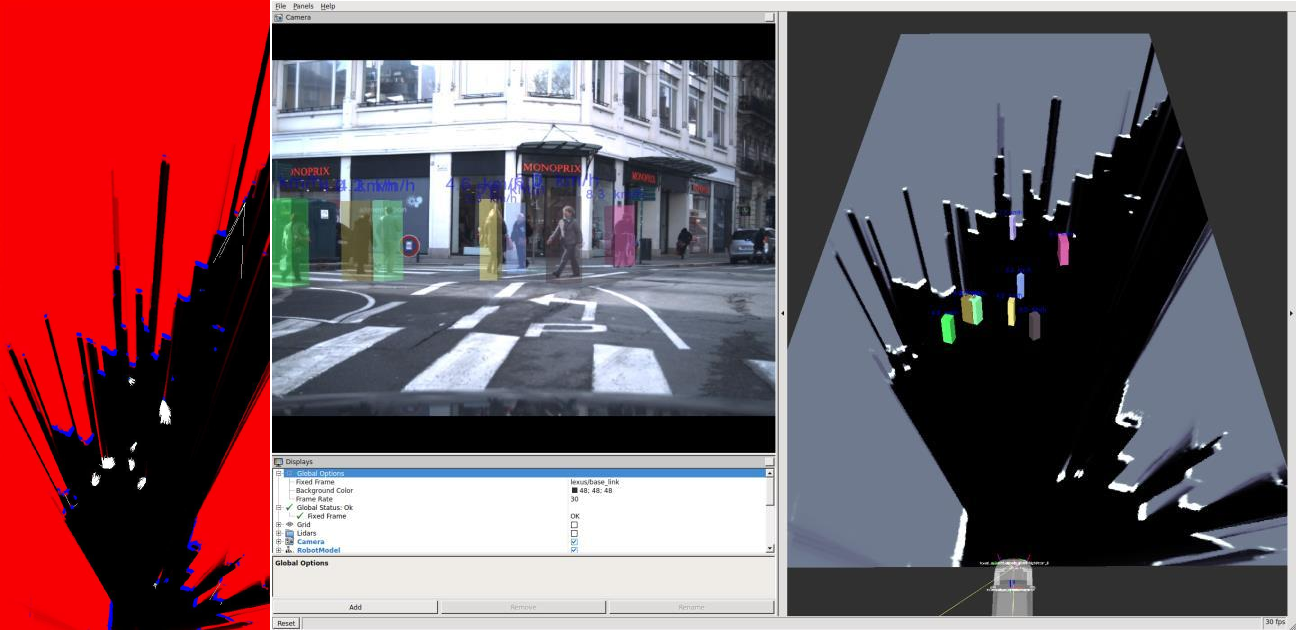
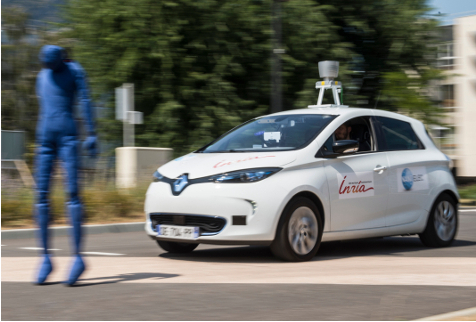
System validation
Context. Testing and validating Cyber Physical Systems which are designed for operating in various real world conditions, is both an open scientific question and a necessity for a future deployment of such systems. In particular, this is the case for Embedded Perception and Decision-making Systems which are designed for future ADAS 9 and Autonomous Vehicles. Indeed, it is unrealistic to try to be exhaustive by making a huge number of experiments in various real situations. Moreover, such experiments might be dangerous, highly time consuming, and expensive. This is why we have decided to develop appropriate realistic simulation and statistical analysis tools for being able to perform a huge number of tests based on some previously recorded real data and on random changes of some selected parameters (the “co-simulation” concept). Such an approach might also be used in a training step of a machine learning process. This is why simulation-based validation is getting more and more popular in automotive industry and research.
This work is performed in the scope of both the SAV 10 project (IRT Nanoelec) and of the EU Enable-S3 project; it is also performed in cooperation with the Inria team Tamis in Rennes, with the objective to integrate the Tamis “Statistical Model Checking” (SMC) approach into our validation process. We are also starting to work on this topic with the Inria team Convecs, with the objective to also integrate formal methods into our validation process.
Objectives. We started to work on this new research topic in 2017. The first objective is to build a “simulated navigation framework” for: (1) constructing realistic testing environments (including the possibility of using real experiments records), (2) developing for each vehicle a simulation model including various physical and dynamic characteristics (e.g. physics, sensors and motion control), and (3) evaluating the performances of a simulation run using appropriate statistical software tools.
The second objective is to develop models and tools for automating the Simulation & Validation process, by using a selection of relevant randomized parameters for generating large database of tests and statistical results. Then, a metric based on the use of some carefully selected “Key Performance Indicator” (KPI) has to be defined for performing a statistical evaluation of the results (e.g. by using the above-mentioned SMC approach).
Situation Awareness and Prediction
Context. Predicting the evolution of the perceived moving agents in a dynamic and uncertain environment is mandatory for being able to safely navigate in such an environment. We have recently shown that an interesting property of the Bayesian Perception approach is to generate short-term conservative 11 predictions on the likely future evolution of the observed scene, even if the sensing information is temporary incomplete or not available 78. But in human populated environments, estimating more abstract properties (e.g. object classes, affordances, agent’s intentions) is also crucial to understand the future evolution of the scene. This work is carried out in the scope of the Security of Autonomous Vehicle (SAV) project (IRT Nanoelec) and of several cooperative and PhD projects with Toyota and with Renault.
Objectives. The first objective is to develop an integrated approach for “Situation Awareness & Risk Assessment” in complex dynamic scenes involving multiples moving agents (e.g vehicles, cyclists, pedestrians ...), whose behaviors are most of the time unknown but predictable. Our approach relies on combining machine learning to build a model of the agent behaviors and generic motion prediction techniques (e.g. Kalman-based, GHMM , or Gaussian Processes). In the perspective of a long-term prediction we will consider the semantic level 12 combined with planning techniques.
The second objective is to build a general framework for perception and decision-making in multi-robot/vehicle environments. The navigation will be performed under both dynamic and uncertainty constraints, with contextual information and a continuous analysis of the evolution of the probabilistic collision risk. Interesting published and patented results 69 have already been obtained in cooperation with Renault and UC Berkeley, by using the “Intention / Expectation” paradigm and Dynamic Bayesian Networks. We are currently working on the generalization of this approach, in order to take into account the dynamics of the vehicles and multiple traffic participants. The objective is to design a new framework, allowing us to overcome the shortcomings of rules-based reasoning approaches which often show good results in low complexity situations, but which lead to a lack of scalability and of long terms predictions capabilities.
Robust state estimation (Sensor fusion)
Context. In order to safely and autonomously navigate in an unknown environment, a mobile robot is required to estimate in real time several physical quantities (e.g., position, orientation, speed). These physical quantities are often included in a common state vector and their simultaneous estimation is usually achieved by fusing the information coming from several sensors (e.g., camera, laser range finder, inertial sensors). The problem of fusing the information coming from different sensors is known as the Sensor Fusion problem and it is a fundamental problem which plays a major role in robotics.
Objective. A fundamental issue to be investigated in any sensor fusion problem is to understand whether the state is observable or not. Roughly speaking, we need to understand if the information contained in the measurements provided by all the sensors allows us to carry out the estimation of the state. If the state is not observable, we need to detect a new observable state. This is a fundamental step in order to properly define the state to be estimated. To achieve this goal, we apply standard analytic tools developed in control theory together with some new theoretical concepts we introduced in 75 (concept of continuous symmetry). Additionally, we want to account the presence of disturbances in the observability analysis.
Our approach is to introduce general analytic tools able to derive the observability properties in the nonlinear case when some of the system inputs are unknown (and act as disturbances). We recently obtained a simple analytic tool able to account the presence of unknown inputs 72, which extends a heuristic solution derived by the team of Prof. Antonio Bicchi 57 with whom we collaborate (Centro Piaggio at the University of Pisa).
Fusing visual and inertial data. A special attention is devoted to the fusion of inertial and monocular vision sensors (which have strong application for instance in UAV navigation). The problem of fusing visual and inertial data has been extensively investigated in the past. However, most of the proposed methods require a state initialization. Because of the system nonlinearities, lack of precise initialization can irreparably damage the entire estimation process. In literature, this initialization is often guessed or assumed to be known 65. Recently, this sensor fusion problem has been successfully addressed by enforcing observability constraints 68 and by using optimization-based approaches 70. These optimization methods outperform filter-based algorithms in terms of accuracy due to their capability of relinearizing past states. On the other hand, the optimization process can be affected by the presence of local minima. We are therefore interested in a deterministic solution that analytically expresses the state in terms of the measurements provided by the sensors during a short time-interval.
For some years we explore deterministic solutions as presented in 76 and 71. Our objective is to improve the approach by taking into account the biases that affect low-cost inertial sensors (both gyroscopes and accelerometers) and to exploit the power of this solution for real applications (see results of the ANR project VIMAD 13 involving experiments with a quadrotor UAV). We have a collaboration with Prof. Stergios Roumeliotis (the leader of the MARS lab at the University of Minnesota) and with Prof. Anastasios Mourikis from the University of California Riverside. Regarding the usage of our solution for real applications we have a collaboration with Prof. Davide Scaramuzza (the leader of the Robotics and Perception group at the University of Zurich) and with Prof. Roland Siegwart from the ETHZ.
3.3 Navigation and cooperation in dynamic environments
In his reference book Planning algorithms14 S. LaValle discusses the different dimensions that made the motion-planning problem complex, which are the number of robots, the obstacle region, the uncertainty of perception and action, and the allowable velocities. In particular, it is emphasized that complete algorithms require at least exponential time to deal with multiple robot planning in complex environments, preventing them to be scalable in practice. Moreover, dynamic and uncertain environments, as human-populated ones, expand this complexity.
In this context, we aim at scale up decision-making in human-populated environments and in multi-robot systems, while dealing with the intrinsic limits of the robots (computation capacity, limited communication).
Motion-planning in human-populated environment
Context. Motion planning in dynamic and human-populated environments is a current challenge of robotics. Many research teams work on this topic. We can cite the Institut of robotic in Barcelone 64, the MIT 55, the Autonomous Intelligent Systems lab in Freiburg 58, or the LAAS 79. In Chroma, we explore different issues : integrating the risk (uncertainty) in planning processes, modeling and taking into account human behaviors and flows.
Objective. We aim to give the robot some socially compliant behaviors by anticipating the near future (trajectories of mobile obstacle in the robot's surroundings) and by integrating knowledge from psychology, sociology and urban planning. In this context, we will focus on the following 3 topics.
Risk-based planning. Unlike static or controlled environments 15 where global path planning approaches are suitable, dealing with highly dynamic and uncertain environments requires to integrate the notion of risk (risk of collision, risk of disturbance). Then, we examine how motion planning approaches can integrate this risk in the generation and selection of the paths. An algorithm called RiskRRT was proposed in the previous eMotion team. This algorithm plans goal oriented trajectories that minimize the risk estimated at each instant. It fits environments that are highly dynamic and adapts to a representation of uncertainty 84 (see Figure 3.a for illustration). Now, we extend this principle to be adapted to various risk evaluation methods (proposed in 3.2) and various situation (highways, urban environments, even in dense traffic).
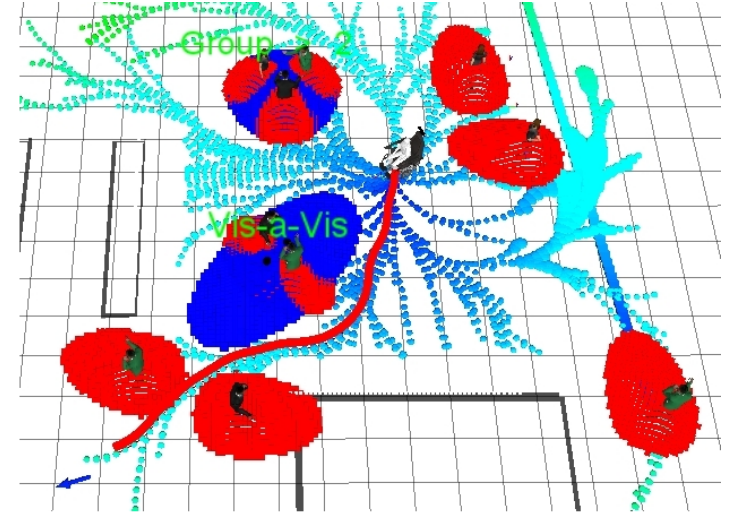
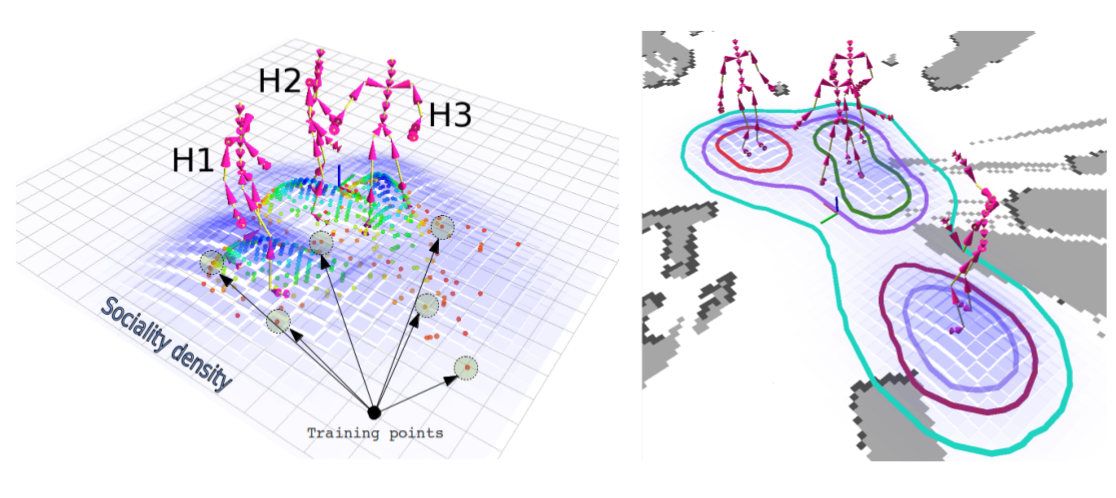
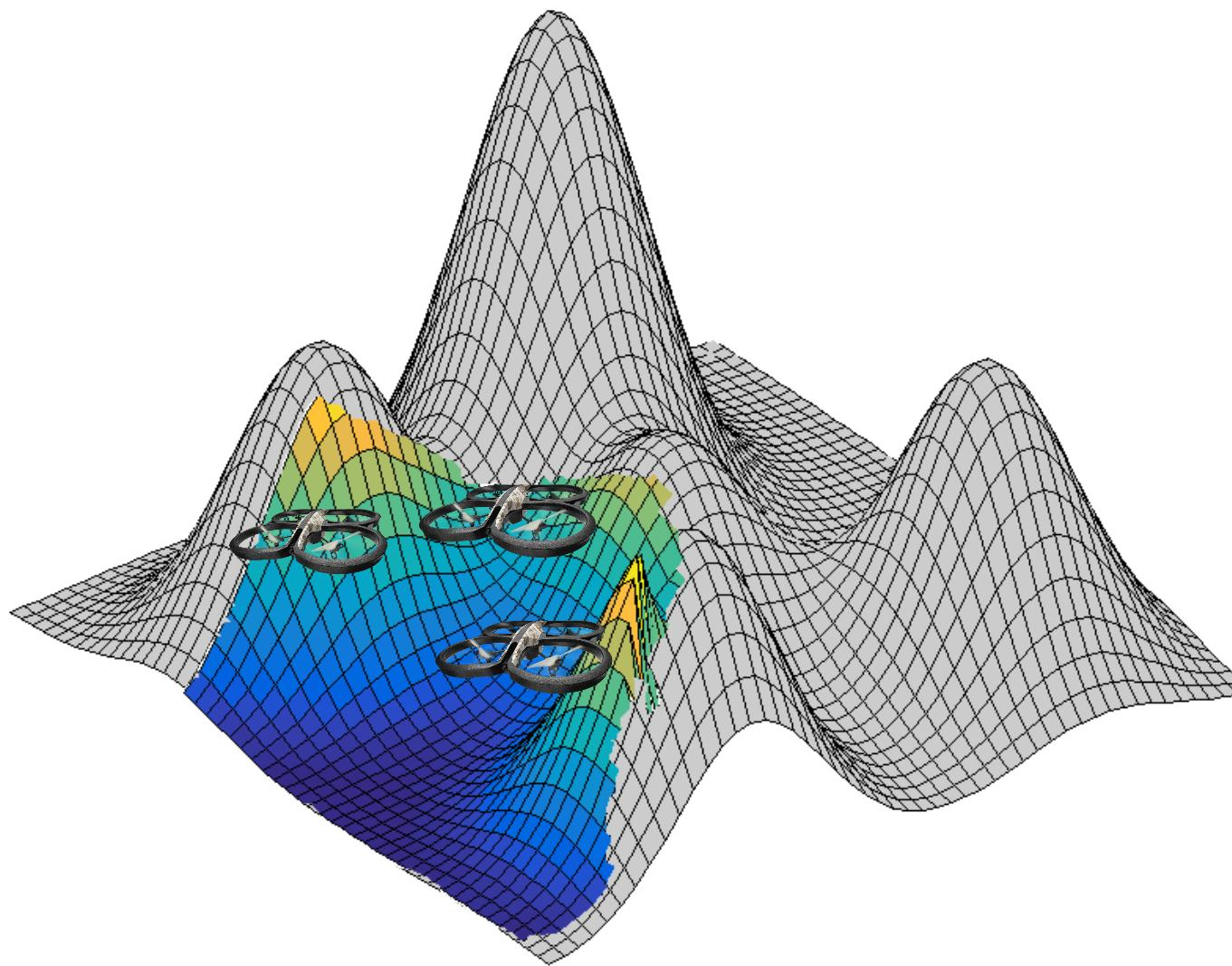
Recently we investigated the automatic learning of robot navigation in complex environments based on specific tasks and from visual input. We address this problem by combining computer vision, machine learning (deep-learning), and robotics path planning (see 7.5.1).
Sharing the physical space with humans. Robots are expected to share their physical space with humans. Hence, robots need to take into account the presence of humans and to behave in a socially acceptable way. Their trajectories must be safe but also predictable, that is why they must follow social conventions, respecting proximity constraints, avoiding people interacting or joining a group engaged in conversation without disturbing. For this purpose, we proposed earlier to integrate some knowledge from the psychology domain (i.e. proxemics theory), see figure 3.b. We aim now to integrate semantic knowledge 16 and psycho-social theories of human behavior 1718 in the navigation framework we have developed for a few years (i.e. the Risk-based navigation algorithms 66, 84, 88). These concepts were tested on our automated wheelchair (see figure 3.c) but they have and will be adapted to autonomous cars, telepresence robots and companion robots. This work was supported by the ANR Valet and since 2020 it continues by the ANR Hianic.
Decision Making in Multi-robot systems
Context. A central challenge in Chroma is to define decision-making algorithms that scale up to large multi-robot systems. This work takes place in the general framework of Multi-Agent Systems (MAS). The objective is to compute/define agent behaviors that provide cooperation and adaptation abilities. Solutions must also take into account the agent/robot computational limits.
We can abstract the challenge in four objectives :
i) mastering the complexity of large fleets of robots/vehicles (scalability),
ii) dealing with limited computational/memory capacity,
iii) building adaptive solutions (robustness),
iv) Integrating different kinds of contraints (related to geometry, for example).
Combining Decision-theoretic models and Swarm intelligence.
Over the past few years, our attempts to address multi-robot decision-making are mainly due to Multi-Agent Sequential Decision Making (MA-SDM) and Swarm Intelligence (SI). MA-SDM builds upon well-known decision-theoretic models (e.g., Markov decision processes and games) and related algorithms, that come with strong theoretical guarantees. In contrast, the expressiveness of MA-SDM models has limited scalability in face of realistic multi-robot systems 19, resulting in computational overload. On their side, SI methods, which rely on local rules – generally bio-inspired – and relating to Self-Organized Systems 20, can scale up to multiple robots and provide robustness to disturbances, but with poor theoretical guarantees 21. Swarm models can also answer to the need of designing tractable solutions 83, but they remain not geared to express complex realistic tasks or to handle (point-to-point) communication between robots. This motivates our work to go beyond these two approaches and to combine them.
First, we plan to investigate incremental expansion mechanisms in anytime decision-theoretic planning, starting from local rules (from SI) to complex strategies with performance guarantees (from MA-SDM) 62. This methodology is grounded into our research on anytime algorithms, that are guaranteed to stop at anytime while still providing a reliable solution to the original problem. It further relies on decision theoretical models and tools including: Decentralized and Partially Observable Markov Decision Processes and Games, Dynamic Programming, Distributed Reinforcement Learning and Statistical Machine Learning.
Second, we plan to extend the SI approach by considering the integration of optimization techniques at the local level. The purpose is to force the system to explore solutions around the current stabilized state – potentially a local optimum – of the system. We aim at keeping scalability and self-organization properties by not compromising the decentralized nature of such systems. Introducing optimization in this way requires to measure locally the performances, which is generally possible from local perception of robots (or using learning techniques). The main optimization methods we will consider are Local Search (Gradient Descent), Distributed Stochastic Algorithm and Reinforcement Learning. We have shown in 87 the interest of such an approach for driverless vehicle traffic optimization.
Both approaches must lead to master the complexity inherent to large and open multi-robot systems. Such systems are prone to combinatorial problems, in term of state space and communication, when the number of robots grows. To cope with this complexity we explore several approaches :
- Combining MA-SDM, machine learning and OR 22 techniques to deal with global-local optimization in multi-agent/robot systems. In 2016, we started a collaboration with the VOLVO Group, in Lyon, to deal with VRP problems and optimization of goods distribution using a fleet of autonomous vehicles. Since January 2020, we continue to develop our acitivies on such optimization problems by the arrival of Christine Solnon.
- Defining heuristics by decentralizing global exact solutions. For instance we explore online stochastic-optimization planning to deal with multi-robot coverage/exploration of 3D environments, see Fig 3.c and 81.
Beyond this methodological work, we aim to evaluate our models on benchmarks from the literature, by using simulation tools as a complement of robotic experiments. This will lead us to develop simulators, allowing to deploy tens to thousands robots in constrained environments.
Towards adaptive connected robots.
Mobile robots and autonomous vehicles are becoming more connected to one another and to other devices in the environment (concept of cloud of robots 23 and V2V/V2I connectivity in transportation systems). Such robotic systems are open systems as the number of connected entities is varying dynamically. Network of robots brought with them new problems, as the need of (online) adaption to changes in the system and to the variability of the communication.
In Chroma, we address the problem of adaptation by considering machine learning techniques and local mechanisms as discussed above (SI models). More specifically we investigate the problem of maintaining the connectivity between robots which perform dynamic version of tasks such as patrolling, exploration or transportation, i.e. where the setting of the problem is continuously changing and growing (see 80).
In Lyon, the CITI Laboratory conducts research in many aspects of telecommunication, from signal theory to distributed computation. In this context, Chroma develops cooperations with the Inria team Agora (eg. in BugWright2 H2020 project) (wireless communication architecture) and with Dynamid team 59 (middlleware and cloud aspects), that we wish to reinforce in the next years.
4 Application domains
4.1 Introduction
Applications in Chroma are organized in two main domains : i) Future cars and transportation of persons and goods in cities, ii) Service robotics with ground and aerial robots. These domains correspond to the experimental fields initiated in Grenoble (eMotion team) and in Lyon (CITI lab). However, the scientific objectives described in the previous sections are intended to apply equally to these applicative domains. Even our work on Bayesian Perception is today applied to the intelligent vehicle domain, we aim to generalize to any mobile robots. The same remark applies to the work on multi-agent decision making. We aim to apply algorithms to any fleet of mobile robots (service robots, connected vehicles, UAVs). This is the philosophy of the team since its creation.
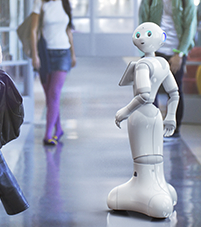
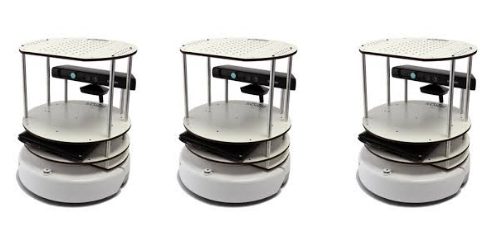
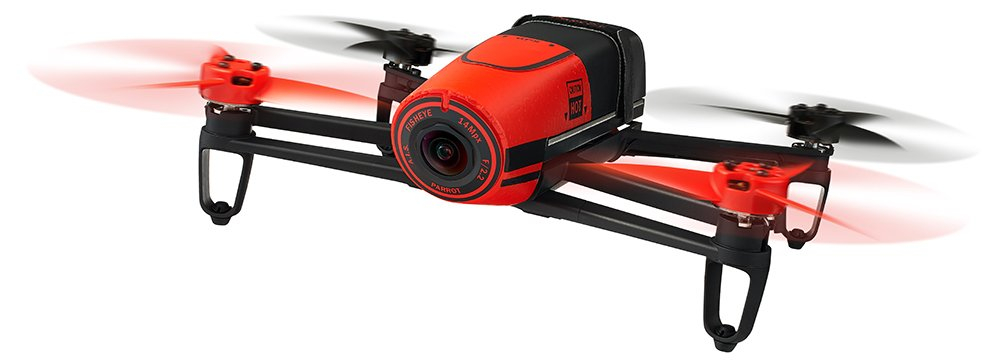
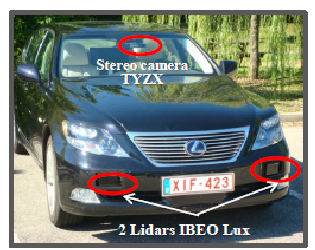
4.2 Future cars and transportation systems
Thanks to the introduction of new sensor and ICT technologies in cars and in mass transportation systems, and also to the pressure of economical and security requirements of our modern society, this application domain is quickly changing. Various technologies are currently developed by both research and industrial laboratories. These technologies are progressively arriving at maturity, as it is witnessed by the results of large scale experiments and challenges such as the Google’s car project and several future products announcements made by the car industry. Moreover, the legal issue starts to be addressed in USA (see for instance the recent laws in Nevada and in California authorizing autonomous vehicles on roads) and in several other countries (including France).
In this context, we are interested in the development of ADAS 24 systems aimed at improving comfort and safety of the cars users (e.g., ACC, emergency braking, danger warnings), and of Fully Autonomous Driving functions for controlling the displacements of private or public vehicles in some particular driving situations and/or in some equipped areas (e.g., automated car parks or captive fleets in downtown centers or private sites).
Since about 8 years, we are collaborating with Toyota and with Renault-Nissan on these applications (bilateral contracts, PhD Theses, shared patents), but also recently with Volvo group (PhD thesis started in 2016). We are also strongly involved (since 2012) in the innovation project Perfect then now Security for autonomous vehicle of the IRT 25 Nanoelec (transportation domain). In 2016, we have been awarded a European H2020 ECSEL project 26 involving major European automotive constructors and car suppliers. In this project, Chroma is focusing on the embedded perception component (models and algorithms, including the certification issue), in collaboration with Renault, Valeo and also with the Inria team TAMIS (Rennes). Chroma (A. Spalanzani) leads the ANR Hianic (2018-21) dealing with pedestrian-vehicle interaction for a safe navigation.
In this context, Chroma has two experimental vehicles equipped with various sensors (a Toyota Lexus and a Renault Zoe, see. Fig. 4 and Fig. 2.b), which are maintained by Inria-SED 27 and that allow the team to perform experiments in realistic traffic conditions (Urban, road and highway environments). The Zoe car has been automated in December 2016, through our collaboration with the team of P. Martinet (IRCCyN Lab, Nantes), that allow new experiments in the team.
4.3 Service robotics with ground and aerial robots
Service robotics is an application domain quickly emerging, and more and more industrial companies (e.g., IS-Robotics, Samsung, LG) are now commercializing service and intervention robotics products such as vacuum cleaner robots, drones for civil or military applications, entertainment robots ... One of the main challenges is to propose robots which are sufficiently robust and autonomous, easily usable by non-specialists, and marked at a reasonable cost. We are involved in developing observation and surveillance systems, by using ground robots and aerial ones, see Fig. 4. Since 2016, we develop solutions for 3D observation/exploration of complex scenes or environments with a fleet of UAVs (BugWright2 H2020 project, Dynaflock Inria/DGA project) or ground robots (COMODYS FIL project 77).
A more recent challenge for the coming decade is to develop robotized systems for assisting elderly and/or disabled people. In the continuity of our work in the IPL PAL 28, we aim to propose smart technologies to assist electric wheelchair users in their displacements and also to control autonomous cars in human crowds (see Figure 10 for illustration). This concerns our recent "Hianic" ANR project. Another emerging application is humanoid robots helping humans at their home or work. In this context, we address the problem of NAMO (Navigation Among Movable Obstacles) in human populated environments (eg. PhD of B. Renault started on 2018). More generally we address navigation and reconnaissance tasks with Pepper humanoids in the context of the RoboCup-Social League.
5 Highlights of the year
5.1 New Member
- In January 2020, Christine Solnon, Professor at INSA Lyon, joined the Chroma team following her request to transfert from LIRIS Lab. to CITI Lab. Chroma members interact with Christine since several years about optimization methodologies applied to intelligent transport.
5.2 ANR Projects & EquipEx+
-
EquipEx+ TIRREX 2021-2030
Title : "Technological Infrastructure for Robotics Research of Excellence" ( 12M€) (32 lab.).
Chroma is involved in two axes of research :
- "Aerial Robotics", Chroma/CITI lab. is a major partner of this axis (INSA Lyon & Inria)
- "Autonomous land robotics", Chroma/CITI lab. is a secondary partner of this axis (INSA Lyon & Inria).
-
ANR ASTRID ’CONCERTO’ (300K€) 2020-2023
Chroma is partner of this project which is led by Isabelle Guerin-Lassous (LIP/Inria Dante).
Title : mobilité Contrôlée et cOmmunicatioNs effiCaces dans une flottE de dRones auTo-Organisée
Partners : LIP (UCBL/Inria Dante), CITI (INSA/Inria Chroma), LS2N (CNRS, Nantes), LORIA (UL, Nancy), Alerion (company in Nancy).
-
Chaire IA "REMEMBER" (ANR) 2020-23 (Chair of research and teaching in artificial intelligence)
Title : Learning Reasoning, Memory and Behavior (funding 574K€)
This Chair is led by Christian Wolf (INSA Lyon/LIRIS lab.).
Partners : O. Simonin and J. Dibangoye from CHROMA, and L. Matignon from LIRIS/Lyon1.
5.3 Startups
Creation of two startups inside Chroma and founded by two engineers from the team :
- CrowFox, founded by Vincent Le Doze, supported by the Inria Startup studio, CITI lab. (Lyon).
- RoboChef, founded by Anshul Paigwar, supported by the Inria Startup studio, Montbonnot (Grenoble).
5.4 Publications
In 2020, Chroma published its works in 8 major international journals and 15 A/A+ international conferences in the fields of AI, Robotics and Intelligent Transport.
6 New software and platforms
6.1 New software
6.1.1 CMCDOT
- Keywords: Robotics, Environment perception
- Functional Description: CMCDOT is a Bayesian filtering system for dynamic occupation grids, allowing parallel estimation of occupation probabilities for each cell of a grid, inference of velocities, prediction of the risk of collision and association of cells belonging to the same dynamic object. Last generation of a suite of Bayesian filtering methods developed in the Inria eMotion team, then in the Inria Chroma team (BOF, HSBOF, ...), it integrates the management of hybrid sampling methods (classical occupancy grids for static parts, particle sets for parts dynamics) into a Bayesian unified programming formalism , while incorporating elements resembling the Dempster-Shafer theory (state "unknown", allowing a focus of computing resources). It also offers a projection system of the estimated scene in the near future, to reference potential collisions with the ego-vehicle or any other element of the environment, as well as very low cost pre-segmentation of coherent dynamic spaces (taking into account speeds). It takes as input instantaneous occupation grids generated by sensor models for different sources, the system is composed of a ROS package, to manage the connectivity of I / O, which encapsulates the core of the embedded and optimized application on GPU Nvidia (Cuda), allowing real-time analysis of the direct environment on embedded boards (Tegra X1, X2). ROS (Robot Operating System) is a set of open source tools to develop software for robotics. Developed in an automotive setting, these techniques can be exploited in all areas of mobile robotics, and are particularly suited to highly dynamic and uncertain environment management (e.g. urban scenario, with pedestrians, cyclists, cars, buses, etc.).
- Authors: Amaury Nègre, Lukas Rummelhard, Jean-Alix David, Christian Laugier
- Contact: Christian Laugier
- Partners: CEA, CNRS
6.1.2 Ground Elevation and Occupancy Grid Estimator (GEOG - Estimator)
- Keywords: Robotics, Environment perception
- Functional Description: GEOG-Estimator is a system of joint estimation of the shape of the ground, in the form of a Bayesian network of constrained elevation nodes, and the ground-obstacle classification of a pointcloud. Starting from an unclassified 3D pointcloud, it consists of a set of expectation-maximization methods computed in parallel on the network of elevation nodes, integrating the constraints of spatial continuity as well as the influence of 3D points, classified as ground-based or obstacles. Once the ground model is generated, the system can then construct a occupation grid, taking into account the classification of 3D points, and the actual height of these impacts. Mainly used with lidars (Velodyne64, Quanergy M8, IBEO Lux), the approach can be generalized to any type of sensor providing 3D pointclouds. On the other hand, in the case of lidars, free space information between the source and the 3D point can be integrated into the construction of the grid, as well as the height at which the laser passes through the area (taking into account the height of the laser in the sensor model). The areas of application of the system spread across all areas of mobile robotics, it is particularly suitable for unknown environments. GEOG-Estimator was originally developed to allow optimal integration of 3D sensors in systems using 2D occupancy grids, taking into account the orientation of sensors, and indefinite forms of grounds. The ground model generated can be used directly, whether for mapping or as a pre-calculation step for methods of obstacle recognition or classification. Designed to be effective (real-time) in the context of embedded applications, the entire system is implemented on Nvidia graphics card (in Cuda), and optimized for Tegra X2 embedded boards. To ease interconnections with the sensor outputs and other perception modules, the system is implemented using ROS (Robot Operating System), a set of opensource tools for robotics.
- Authors: Amaury Nègre, Lukas Rummelhard, Lukas Rummelhard, Jean-Alix David, Christian Laugier
- Contact: Christian Laugier
6.1.3 Zoe Simulation
- Name: Simulation of INRIA's Renault Zoe in Gazebo environment
- Keyword: Simulation
- Functional Description: This simulation represents the Renault Zoe vehicle considering the realistic physical phenomena (friction, sliding, inertia, ...). The simulated vehicle embeds sensors similar to the ones of the actual vehicle. They provide measurement data under the same format. Moreover the software input/output are identical to the vehicle's. Therefore any program executed on the vehicle can be used with the simulation and reciprocally.
- Authors: Christian Laugier, Nicolas Turro, Thomas Genevois
- Contact: Christian Laugier
6.1.4 Hybrid-state E*
- Name: Path planning with Hybrid-state E*
- Keywords: Planning, Robotics
-
Functional Description:
Considering a vehicle with the kinematic constraints of a car and an environment which is represented by a probabilistic occupancy grid, this software produces a path from the initial position of the vehicle to its destination. The computed path may include, if necessary, complex maneuvers. However the suggested path is often the simpler and the shorter.
This software is designed to take benefit from bayesian occupancy grids such as the ones computed by the CMCDOT software.
-
URL:
https://
team. inria. fr/ chroma/ en/ - Authors: Christian Laugier, Thomas Genevois
- Contact: Christian Laugier
- Partner: CEA
6.1.5 Pedsim_ros_AV
- Name: Pedsim_ros_AV
- Keywords: Simulator, Multi-agent, Crowd simulation, Autonomous Cars, Pedestrian
-
Scientific Description:
These ROS packages are useful to support robotic developments that require the simulation of pedestrians and an autonomous vehicle in various shared spaces scenarios. They allow: 1. in simulation, to pre-test autonomous vehicle navigation algorithms in various crowd scenarios, 2. in real crowds, to help online prediction of pedestrian trajectories around the autonomous vehicle.
Individual pedestrian model in shared space (perception, distraction, personal space, pedestrians standing, trip purpose). Model of pedestrians in social groups (couples, friends, colleagues, family). Autonomous car model. Pedestrian-autonomous car interaction model. Definition of shared space scenarios: 3 environments (business zone, campus, city centre) and 8 crowd configurations.
- Functional Description: Simulation of pedestrians and an autonomous vehicle in various shared space scenarios. Adaptation of the original Pedsim_ros model to simulate heterogeneous crowds in shared spaces (individuals, social groups, etc.). The car model is integrated into the simulator and the interactions between pedestrians and the autonomous vehicle are modeled. The autonomous vehicle can be controlled from inside the simulation or from outside the simulator by ROS commands.
-
URL:
https://
github. com/ maprdhm/ pedsim_ros_AV - Publications: hal-02194735, hal-02514963
- Contact: Manon Prédhumeau
- Participants: Manon Prédhumeau, Anne Spalanzani, Julie Dugdale, Lyuba Mancheva
- Partner: LIG
6.1.6 S-NAMO-SIM
- Name: S-NAMO Simulator
- Keywords: Simulation, Navigation, Robotics, Planning
- Functional Description: 2D Simulator of NAMO algorithms (Navigation Among Movable Obstacles) ROS compatible
- Release Contributions: Creation
- Author: Benoit Renault
- Contact: Benoit Renault
6.1.7 SimuDronesGR
- Name: Simultion of UAV fleets with Gazebo/ROS
- Keywords: Robotics, Simulation
- Functional Description: The simulator includes the following functionality : 1) Simulation of the mechanical behavior of an Unmanned Aerial Vehicle : * Modeling of the body's aerodynamics with lift, drag and moment * Modeling of rotors' aerodynamics using the forces and moments' expressions from Philppe Martin's and Erwan Salaün's 2010 IEEE Conference on Robotics and Automation paper "The True Role of Accelerometer Feedback in Quadrotor Control". 2) Gives groundtruth informations : * Positions in East-North-Up reference frame * Linear velocity in East-North-Up and Front-Left-Up reference frames * Linear acceleration in East-North-Up and Front-Left-Up reference frames * Orientation from East-North-Up reference frame to Front-Left-Up reference frame (Quaternions) * Angular velocity of Front-Left-Up reference frame expressed in Front-Left-Up reference frame. 3) Simulation of the following sensors : * Inertial Measurement Unit with 9DoF (Accelerometer + Gyroscope + Orientation) * Barometer using an ISA model for the troposphere (valid up to 11km above Mean Sea Level) * Magnetometer with the earth magnetic field declination * GPS Antenna with a geodesic map projection.
- Release Contributions: Initial version
- Author: Vincent Le Doze
- Contact: Vincent Le Doze
- Partner: Insa de Lyon
6.1.8 spank
- Name: Swarm Protocol And Navigation Kontrol
- Keyword: Protocoles
- Functional Description: Communication and distance measurement in an uav swarm
-
URL:
https://
gitlab. inria. fr/ dalu/ spank - Author: Stéphane d'Alu
- Contact: Stéphane d'Alu
7 New results
7.1 Robust state estimation (Sensor fusion)
This research is the follow up of Agostino Martinelli's investigations carried out during the last six years. It is focused on two main topics which are the visual and inertial sensor fusion problem and the unknown input observability problem.
7.1.1 Visual-inertial structure from motion
Participant: Agostino Martinelli.
Since 2018 we are studying the visual inertial sensor fusion problem in the cooperative case. In 2019 we obtained the analytic solution of this important problem 73, 74. This solution is based on a fundamental equivalence that has been established. This sensor fusion problem is equivalent to a polynomial equation system which consists of three polynomials of second degree and many polynomials of first degree. Starting from this equivalence, during the last part of 2019, we carried out a thorough analysis of the problem singularities. In the first part of 2020 we concluded this analysis. We obtained an exhaustive analysis that includes all the singularities, degeneracies, and minimal cases. The usefulness of this analysis has also been illustrated with simulations. In particular, we have quantitatively obtained how the performance of the state estimation worsens near a singularity. The results of this research have been published by the robotics and automation letter journal 6. They were also presented at ICRA 2020.
7.1.2 Unknown Input Observability
Participant: Agostino Martinelli.
This research is the follow-up of the study conducted during the last six years, which mainly consisted in the derivation of the analytical solution of the unknown input observability problem. This was an open problem, formulated during the 1960's by the automatic control community. In December 2018, I was invited by the Society for Industrial and Applied Mathematics (SIAM) to write a book with the solution of this problem. This was the main work during 2019. As this general solution is based on tensorial calculus (Ricci algebra) and many mathematics procedures and tricks borrowed from theoretical physics, the scope of this book has gone much more beyond the presentation of the analytical solution of the unknown input observability problem. Basically, I introduced a new theory of observability, based on the group of invariance. The activity, during 2020, took place along the following three guidelines:
- Finalize the writing of the book also taking into account all the issues raised during the review process.
- Extend the solution to more general systems.
- Exploit the new theory introduced in the book to obtain the solution of further problems.
Regarding the second point, we exhaustively dealt with the systems that do not belong to the category of the systems that are canonical with respect to their unknown inputs. The solution introduced in book 43 (2020) applies only to systems that satisfy this fundamental assumption. We provided new definitions of this concept and we obtained a more general solution that can also be applied to systems that are not canonical with respect to their unknown inputs. In addition, we showed that, in some cases, we can transform a non canonical system into a canonical system. We called these systems canonizable. The new proposed solution can deal with the case where the system is not canonical and not even canonizable. This new solution is also provided in the form of a new algorithm. Finally, as a simple consequence of these results , we also provided the answer to the problem of unknown input reconstruction which is intimately related to the problem of state observability. All these new results have been published on arXiv 29.
Regarding the third point, we extended the controllability rank condition to nonlinear, time-variant systems. This extension follows the same steps to extend the observability rank condition. This result was published on arXiv 30.
7.2 Bayesian Perception
Participants: Christian Laugier, Lukas Rummelhard, Jean-Alix David, Jerome Lussereau, Thomas Genevois, Alessandro Renzaglia, Nicolas Turro, Rabbia Asghar.
Recognized as one of the core technologies developed within the team over the years (see related sections in previous activity report of Chroma, and previously e-Motion reports), the CMCDOT framework is a generic Bayesian Perception framework, designed to estimate a dense representation of dynamic environments and the associated risks of collision, by fusing and filtering multi-sensor data. This whole perception system has been developed, implemented and tested on embedded devices, incorporating over time new key modules. In 2020, this framework, and the corresponding software, has continued to be the core of many important industrial partnerships and academic contributions, and to be the subject of important developments, both in terms of research and engineering. Some of those recent evolutions are detailed below.
7.2.1 Path planning relying on bayesian occupancy grids
Participants: Thomas Genevois, Christian Laugier.
Willing to create a new opening for its Bayesian occupancy grids technologies, CHROMA studied their application to path planning. The state of the art of path planning shows few solutions working with a non-binary occupancy representation. Furthermore almost none of these solutions can consider a non-holonomic vehicle.
Therefore CHROMA decided to develop its own path planning solution based on Bayesian occupancy grids and dedicated to a car or similar vehicle. This path planning method has been built on the basis of the E* algorithm with a custom interpolation kernel. It also uses a hybrid-state representation in order to have an accurate path representation at various resolutions. The resulting path planning method has been called Hybrid-State E*. It has been successfully tested in simulation and on our experimental Zoe platform. Figure 5 shows several examples of paths computed with this method.
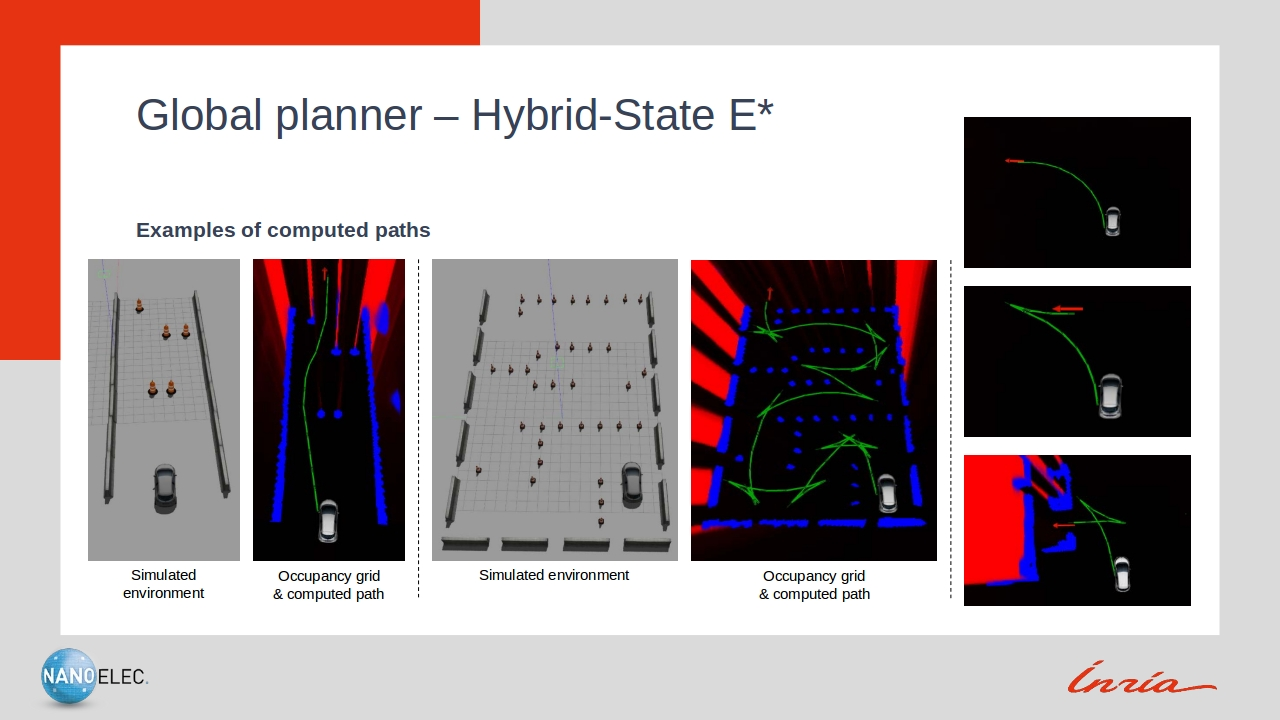
7.2.2 Testing of the experimental platform
Participants: Thomas Genevois, Rabbia Asghar, Jerome Lussereau, Lukas Rummelhard, Christian Laugier.
Series of tests of the experimental Zoe platform have been performed on a dedicated circuit (see Fig. 6). These tests allowed a complete validation and characterization of the basic automation of the vehicle. The behavior of the vehicle has been challenged at different speed, up to 60km/h, with various steering, acceleration and deceleration commands.
Then several perception and navigation capabilities of the vehicle have been tested. These tests included obstacle detection and avoidance at 30km/h and path following at 50km/h. During these tests, all data of the vehicle have been recorded. These data are now studied to analyze the performance of the software and improve it.
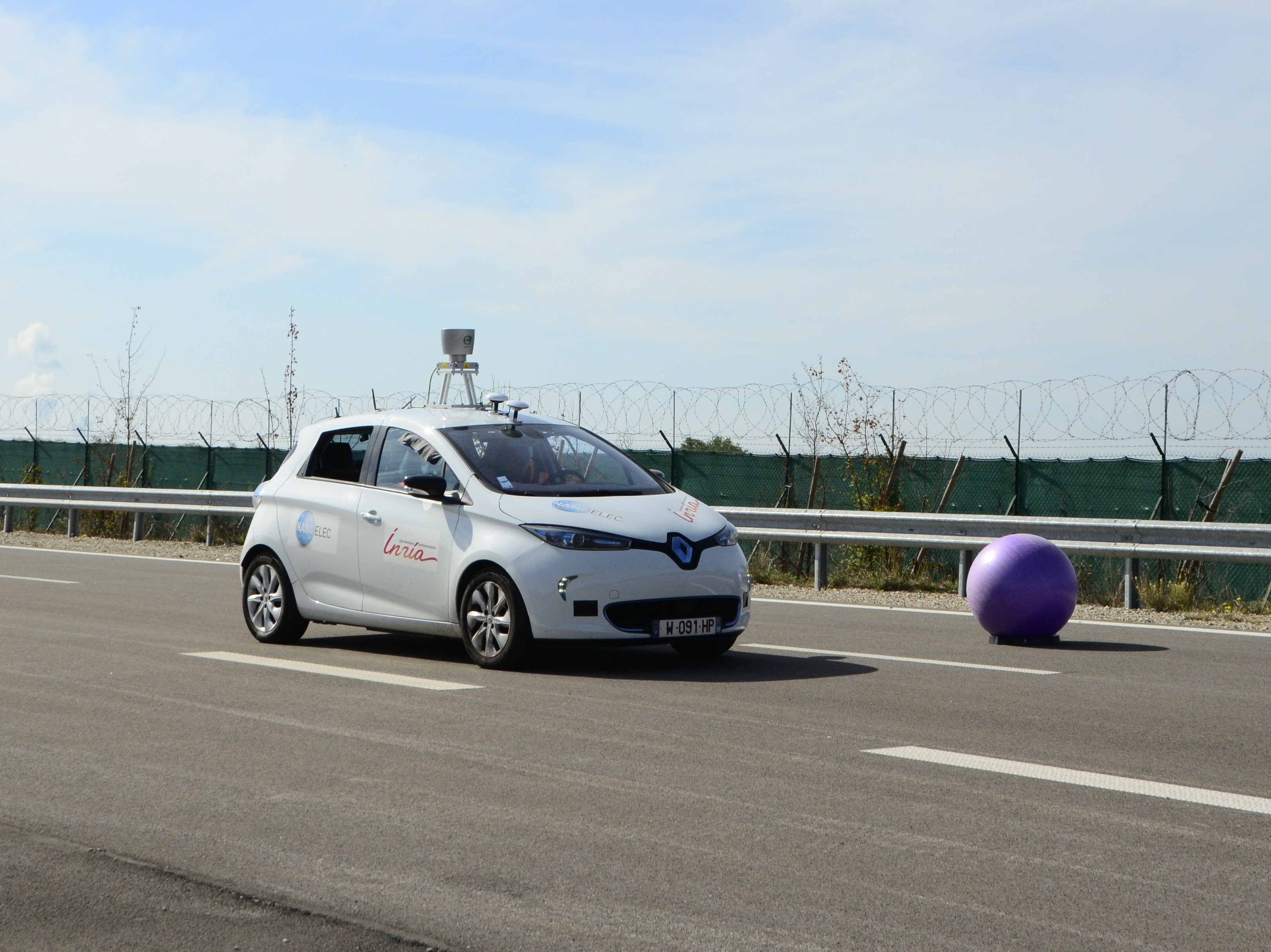
7.2.3 System architecture for autonomous driving
Participants: Rabbia Asghar, Jerome Lussereau, Christian Laugier.
In scope Project Tornado, the developed work focuses on problem of autonomous vehicle navigation on Route du Bray, Rambouillet. For this task, a complete system architecture has been built that deals with various segments of autonomous driving including hierarchical decision-making, vehicle localization 19, perception of the environment, path planning and control of the vehicle 47.
Perceiving the surrounding environment and extracting adequate information is critical for scene understanding and decision-making. For a reliable curve estimation, results of Nvidia DriveWorks lane detection module have been fused with Velodyne (lidar), odometry and offline map. Subsequently, this information from curve estimation is overlayed on the CMCDOT state grid to generate a costmap and assist in obstacle detection and characterization. For motion planning and control, lane keeping module has been one of the primary focus of development. The local motion planner, utilizes this information provided by perception module and optimizes the path to ensure collision-free and safe path in the center of the lane.
The developed modules have been tested and validated on Zoe experimental platform. Autonomous vehicle testing has been conducted at the Transpolis facility and lane keeping capabilities in controlled environment has been validated at low speed of 20-30km/h.
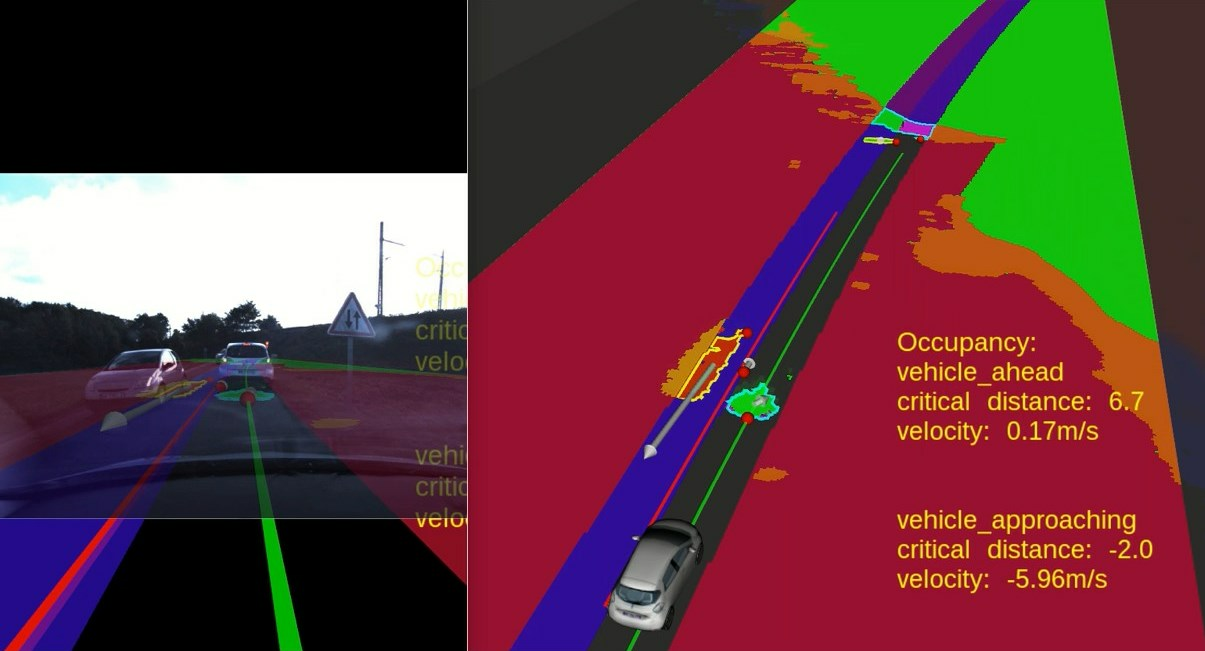
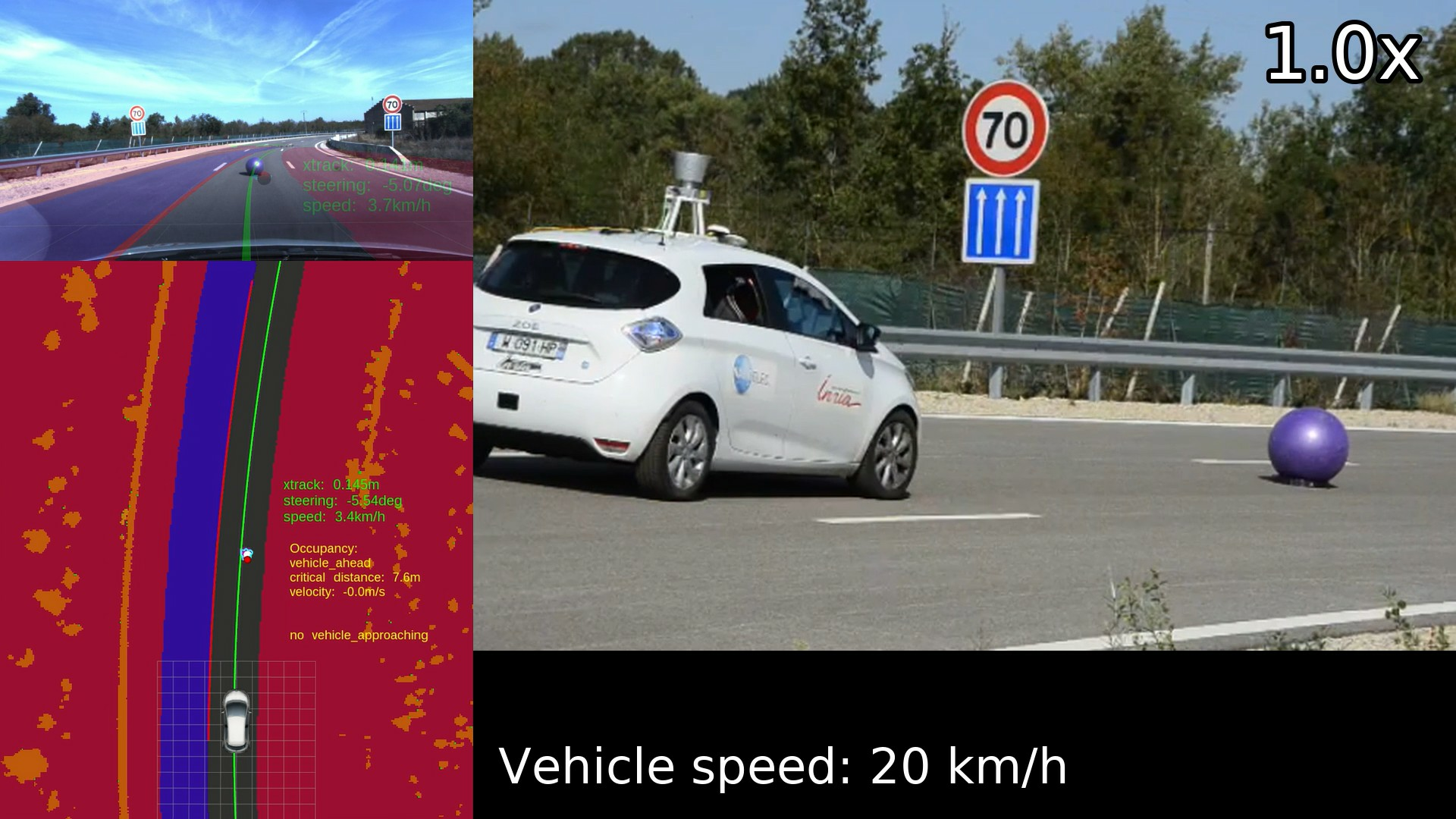
7.2.4 Validation of AI-based algorithms in autonomous vehicles
Participants: Alessandro Renzaglia, Anshul Paigwar, Radu Mateescu, Christian Laugier.
In the last years, there has been an increasing demand for regulating and validating intelligent vehicle systems to ensure their correct functioning and build public trust in their use. Yet, the analysis of safety and reliability poses a significant challenge. More and more solutions for autonomous driving are based on complex AI-based algorithms whose validation is particularly challenging to achieve. Perception systems dealing with dynamic and unexpected scenarios is one example where the use of probabilistic approaches becomes essential to accurately confront the uncertainties in the environment. Due to the stochasticity and complexity of these systems, validation through standard approaches is usually not viable. For instance, validation through exhaustive techniques is usually infeasible since it requires checking desired property in all reachable sets. An important part of our work has been recently devoted to tackle this problem, finding new suitable approaches to validate probabilistic algorithms for perception and decision making in autonomous driving, and investigating how simulations and experiments in controlled environment can help to solve this challenge.
This work, started with our participation in the European project Enable-S3 (2016-2019), has been conducted in collaboration with other teams expert in validation end verification methods, namely , and the group of A. Legay at the Université de Louvain (Belgium). With these two collaborations we explored two viable solutions to the validation problem: Formal Methods (FM) and Statistical Model Checking.
In particular, we proposed an SMC-based validation scheme to analyze the collision risk prediction provided by a probabilistic perception system 32. The key contributions of this study can be summarized as follows: we presented an SMC-based approach for statistical validation of probabilistic collision risk estimation; we used CMCDOT to obtain collision risk estimations and showed how our SMC-based approach can be adapted to validate probabilistic algorithms; by relying on CARLA simulator, we presented a simulation framework that can be parameterized to generate large numbers of execution traces reproducing realistic collisions and almost-collisions scenarios; we performed real experiments and quantitative comparisons to prove the integrity of our simulation-based approach.
During this year, the main work with the Convecs team has been the preparation of the joint participation in the PRISSMA project, which will start in early 2021 and will increase the level of collaboration and research on this subject. PRISSMA is a project prepared as part of the "Grand Défi: Sécuriser, certifier et fiabiliser les systèmes fondés sur l’intelligence artificielle". This project will aim at ensuring the transparency and validation of autonomous systems based on artificial intelligence.
7.3 Situation Awareness & Decision-making for Autonomous Vehicles
Participants: Ozgur Erkent, David Sierra-González, Anshul Paigwar, Christian Laugier, Manuel Alejandro Diaz-Zapata, Andrés Gómez H., Jilles Dibangoye, Luiz Serafim-Guardini, Anne Spalanzani.
In this section, we present all the novel results in the domains of perception, motion prediction and decision-making for autonomous vehicles.
7.3.1 Semantic Segmentation with Unsupervised Domain Adaptation Under Varying Weather Conditions for Autonomous Vehicles
Participants: Özgür Erkent, Christian Laugier.
Detection of the objects around a vehicle is important for a safe and successful navigation of an autonomous vehicle. Instance segmentation provides a fine and accurate classification of the objects such as cars, trucks, pedestrians, etc. In this study, we propose a fast and accurate approach which can detect and segment the object instances which can be adapted to new conditions without requiring the labels from the new condition. Furthermore, the performance of the instance segmentation does not degrade in detection of the objects in the original condition after it adapts to the new condition. To our knowledge, currently there are not other methods which perform unsupervised domain adaptation for the task of instance segmentation using non-synthetic datasets. We evaluate the adaptation capability of our method on two datasets. Firstly, we test its capacity of adapting to a new domain; secondly, we test its ability to adapt to new weather conditions. The results show that it can adapt to new conditions with an improved accuracy while preserving the accuracy of the original condition.
This work has been published at IEEE Robotics and Automation Letters (RA-L) and presented at IEEE International Conference on Robotics and Automation (ICRA) 2020 14.
7.3.2 Human-like motion prediction & planning for autonomous driving in real traffic situations
Participants: David Sierra González, Anshul Paigwar, Özgür Erkent, Vladislav Shlenskii, Jilles Dibangoye, Christian Laugier.
The main goals of this joint project with Toyota Motor Europe are the development and the experimental deployment of machine learning models for motion prediction and motion planning suitable for realistic traffic scenarios.
As a necessary first step before prediction and planning, we dedicated 2020 to develop a reliable 3D obstacle detection model based on Lidar input data 39. The main novelty of the proposed model is that it leverages the output of a dynamic occupancy grid filter (CMCDOT 82) to: 1) improve the accuracy of the detections; and 2) reduce the detection performance loss when deploying the model on lower-density point clouds. The second advantage is particularly important for the project, as our Inria-TME Lexus experimental platform is equipped with 4-layer Lidar sensors in the bumpers and a 8-layer Lidar sensor on the roof. This contrasts with the 64 layer data of the training dataset (KITTI).
The architecture of the model is shown in Fig. 8. The results obtained show that having access to the environment dynamics improves by 27% the ability of the detection algorithm to predict the orientation of smaller obstacles such as pedestrians. Furthermore, the 3D and bird’s eye view bounding box predictions for pedestrians in challenging cases also see a 7% improvement. Qualitatively speaking, the dynamics help with the detection of partially occluded and far-away obstacles.
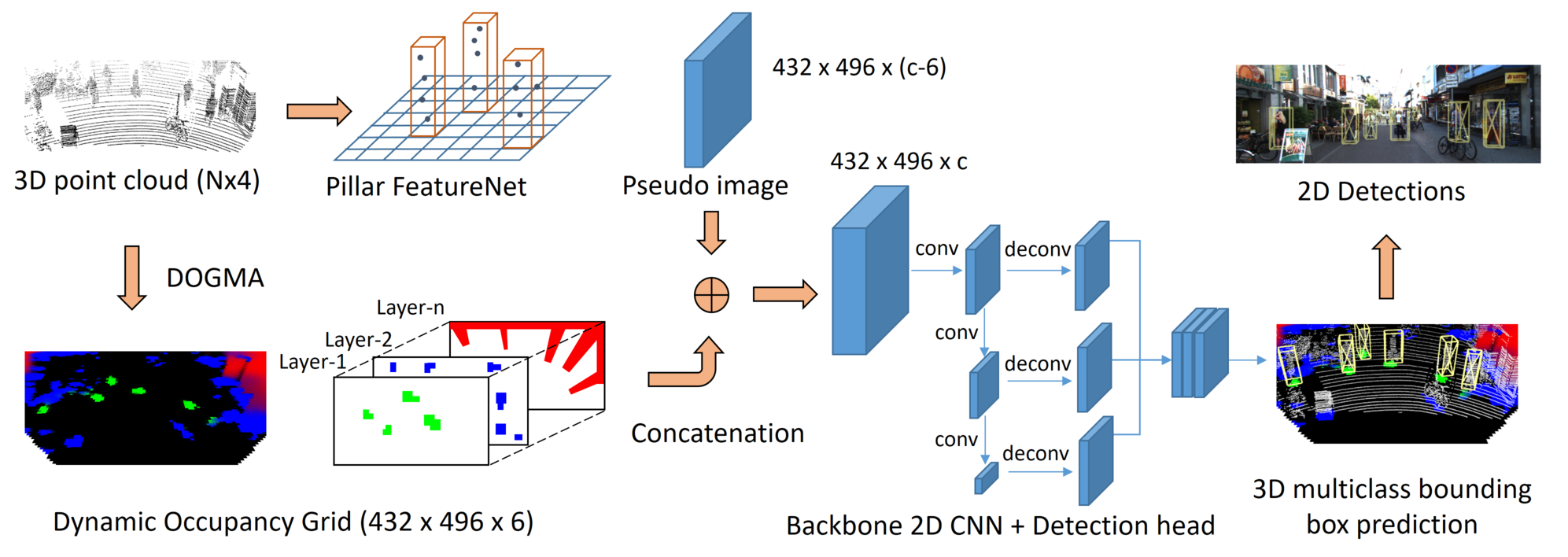
In the scope of this project, we hosted Vladislav Shlenskii, a MSc student from the MSc program in industrial and applied mathematics (MSIAM) of the Université Grenoble-Alpes. He prepared with us his MSc dissertation, titled "Domain adaptation for cross-sensor 3D object detection on point-clouds". In his dissertation, he studied the effect that input point cloud density has on the accuracy of state-of-the-art 3D detection models and how domain adaptation techniques can be used to limit performance degradation when deploying models on test data from a different distribution.
Our current efforts are dedicated to the development of a deep learning model for motion prediction that considers the interactions of the target with the road environment (map or perception data) and with surrounding traffic users.
7.3.3 Panoptic Segmentation
Participants: Manuel Alejandro Diaz-Zapata, Özgür Erkent, Christian Laugier.
Detection of the objects around a vehicle is important for a safe and successful navigation of an autonomous vehicle. Instance segmentation provides a fine and accurate classification of the objects such as cars, trucks, pedestrians, etc. In this study, we propose a fast and accurate approach which can detect and segment the object instances which can be adapted to new conditions without requiring the labels from the new condition. Furthermore, the performance of the instance segmentation does not degrade in detection of the objects in the original condition after it adapts to the new condition. To our knowledge, currently there are not other methods which perform unsupervised domain adaptation for the task of instance segmentation using non-synthetic datasets. We evaluate the adaptation capability of our method on two datasets. Firstly, we test its capacity of adapting to a new domain; secondly, we test its ability to adapt to new weather conditions. The results show that it can adapt to new conditions with an improved accuracy while preserving the accuracy of the original condition. This work has been published in ICARCV 2020 25.
Given the recent challenge of Panoptic Segmentation, where every pixel in an image must be given a label, as in semantic segmentation, and an instance id, a new YOLO-based architecture has been also proposed for this computer vision task. This network uses the YOLOv3 architecture, plus parallel semantic and instance segmentation heads to perform full scene parsing. A set of solutions for each of these two segmentation tasks are proposed and evaluated, where a Pyramid Pooling Module is found to be the best semantic feature extractor given a set of feature maps from the Darknet-53 backbone network. The network gives good segmentation results for both stuff and thing classes by training with a frozen backbone, where boundaries between background classes are consistent with the ground truth and the instance masks match closely the true shapes of the objects present in a scene.
This second work has been accomplished during the master internship of Manuel Alejandro Diaz Zapata in Chroma under supervision of Özgür Erkent, and Christian Laugier. Manuel Alejandro Diaz Zapata was a student of the Master of Science in Informatics from Grenoble with Specialization in Graphics, Vision and Robotics during his internship 50.
7.3.4 Recognize Moving Objects Around an Autonomous Vehicle Considering a Deep-learning Detector Model and Dynamic Bayesian Occupancy
Participants: Andrés E. Gómez H., Özgür Erkent, Christian Laugier.
Perception systems on autonomous vehicles have the challenge of understanding the traffic scene in different situations. The fusion of redundant information obtained from different sources has been shown considerable progress under different methodologies to achieve this objective. However, new opportunities are available to obtain better fusion results with the advance of deep-learning models and computing hardware. In this paper, we aim to recognize moving objects in traffic scenes through the fusion of semantic information with occupancy-grid estimations. Our approach considers a deep-learning model with inference times between 22 to 55 milliseconds. Moreover, we use a Bayesian occupancy framework with a Highly-parallelized design to obtain the occupancy-grid estimations. We validate our approach using experimental results with real-world data on urban scenery.
This work has been published and presented at the 16th International Conference on Control, Automation, Robotics and Vision, ICARCV 2020 26.
7.3.5 GndNet: Fast Ground Plane Estimation and Point Cloud Segmentation for Autonomous Vehicles
Participants: Anshul Paigwar, Özgür Erkent, David Sierra-Gonzalez, Christian Laugier.
Ground plane estimation and ground point segmentation is a crucial precursor for many applications in robotics and intelligent vehicles like navigable space detection and occupancy grid generation, 3D object detection, point cloud matching for localization and registration for mapping. In this paper, we present GndNet, a novel end-to-end approach that estimates the ground plane elevation information in a grid-based representation and segments the ground points simultaneously in real-time. GndNet uses PointNet and Pillar Feature Encoding network to extract features and regresses ground height for each cell of the grid. We augment the SemanticKITTI dataset to train our network. We demonstrate qualitative and quantitative evaluation of our results for ground elevation estimation and semantic segmentation of point cloud. GndNet establishes a new state-of-the-art, achieves a run-time of 55Hz for ground plane estimation and ground point segmentation.
This work has been published and presented at the IEEE/RSJ International Conference on Intelligent Robots and Systems (IROS) 2020 33.
7.3.6 Contextualized Emergency Trajectory Planning using severity curves
Participants: Luiz Serafim Guardini, Anne Spalanzani, Christian Laugier, Philippe Martinet.
Over the years, Advanced Driver-Assistance Systems (ADAS) have been developed to enhance safety and better driving by minimizing human error. Nevertheless, ADAS systems still lack interchangeability among the different and separate systems. The objective of this work is to converge the available information in the scene to output an optimal evasive/mitigation maneuver according to given constraints.
During the first year of his PhD, Luiz Serafim Guardini focused on the development of a probabilistic cost map that expresses the Probability of Collision with Injury Risk (PCIR). On top of the information gathered by sensors, it includes the severity of injury in the event of a collision between ego and the objects in the scene.
During the second year, Luiz Serafim Guardini worked on the development of a motion planning method using the Model Predictive Path Integral (MPPI) method to generate an optimal trajectory considering the PCIR as one of the constraints of the problem. This work has been published in 38.
The proposed MPPI framework generates emergency maneuvers in complex scenarios. It conceives emergency trajectories considering the global injury risk for all binary collisions between ego vehicle and a given object in the scene (see an example on Figure 9).
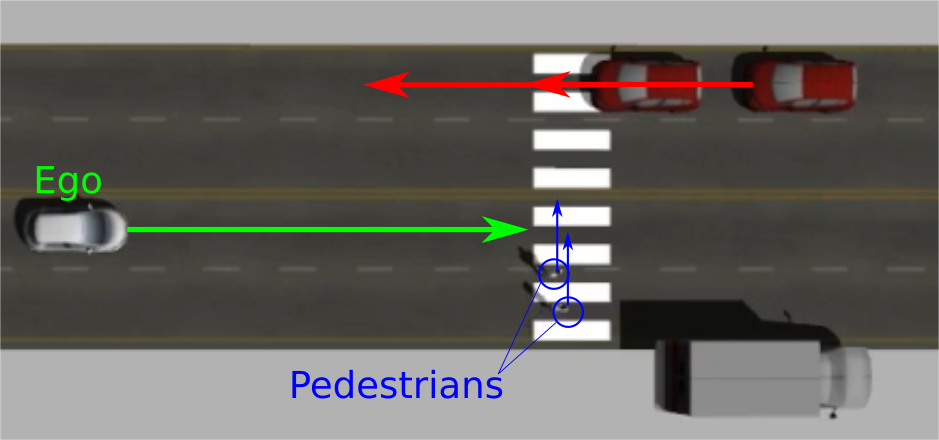
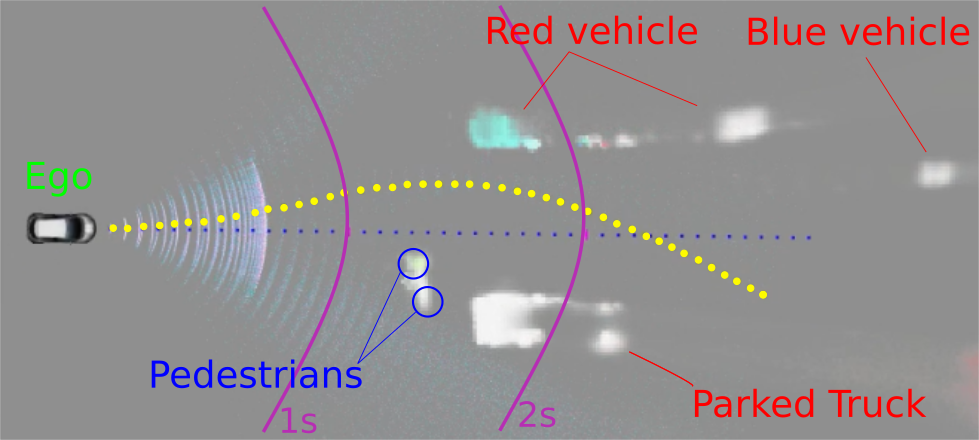
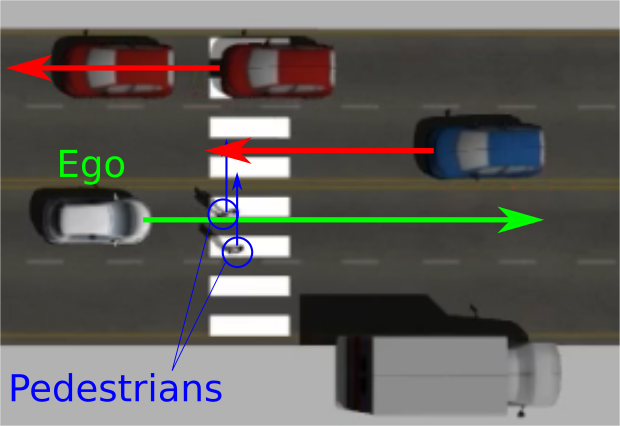
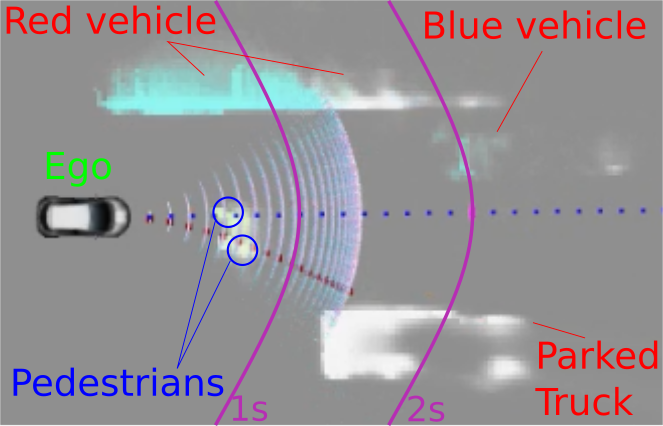
7.4 Motion-planning in dense pedestrian environments
Under the coordination of Anne Splanazani we study new motion planning algorithms to allow robots/ vehicles to navigate in human populated environment while predicting pedestrians' motions. We model pedestrians and crowds behaviors using notions of social forces and cooperative behavior estimations. We investigate new methods to build safe and socially compliant trajectories using theses models of behaviors. We propose proactive navigation solutions as well as deep learning ones. The works of year 2020 are presented below.
7.4.1 Modelling crowds and autonomous vehicles using Extended Social Force Models
Participants: Manon Predhumeau, Anne Spalanzani, Julie Dugdale.
The focus of this work has been on the realistic simulation of crowds in shared spaces with an autonomous vehicle (AV), see Fig. 10. We proposed an agent-based model for pedestrian reactions to an AV in a shared space, based on empirical studies and the state of the art. The model includes an AV with Renault Zoé car’s characteristics, and pedestrians’ reactions to the vehicle. The model extends the Social Force Model with a new decision model, which integrates various observed reactions of pedestrians and pedestrians groups. We performed a qualitative and quantitative evaluation of the proposed model, through comparisons of the simulated trajectories with ground truth trajectories. The proposed model reproduces well the various pedestrians behaviors: running to cross, stopping to wait without deviating, staying in social group to avoid the AV. The model can be used for predicting pedestrians’ displacement, linear velocity, orientation, and approach distance around the AV in fundamental interactions (AAMAS'21 35).
We implemented this model using Pedsim_ros and proposed an open source simulation tool to help the robotic community with AV developments. The simulator integrates various observed pedestrians behaviours, with individual pedestrians as well as pedestrian groups with four different types of relationships (SCS'20 34). Complex shared spaces situations have been modeled, which require the interaction of a large number of pedestrians responding to the AV movement. The simulated AV can be controlled by an external ROS module, so that AV navigation algorithms can be tested in various realistic, crowded shared space environments, before real-world experiments. Moreover, simulations can reproduce real world scenes and can run faster than real-time to help live prediction of pedestrian trajectories around the AV.
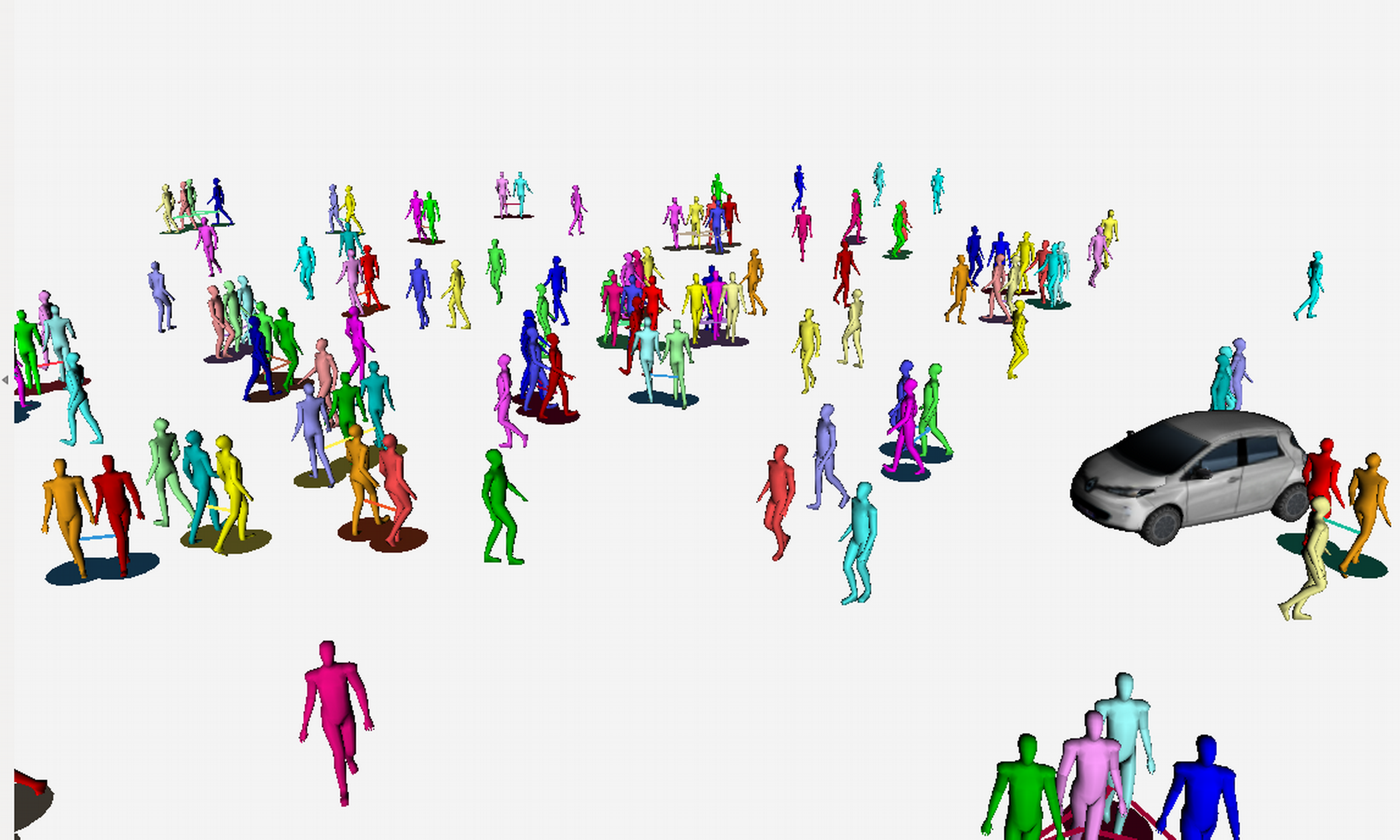
7.4.2 Proactive Navigation for navigating dense human populated environments
Participants: Maria Kabtoul, Anne Spalanzani, Philippe Martinet.
Developing autonomous vehicles capable of navigating safely and socially around pedestrians is a major challenge in intelligent transportation. This challenge cannot be met without understanding pedestrians’ behavioral response to an autonomous vehicle, and the task of building a clear and quantitative description of the pedestrian to vehicle interaction remains a key milestone in autonomous navigation research. As a step towards safe proactive navigation in a spaceshared with pedestrians, we start to introduce in 2018 a pedestrian-vehicle interaction behavioral model. The model estimates the pedestrian’s cooperation with the vehicle in an interaction scenario by a quantitative time-varying function. Using this cooperation estimation the pedestrian’s trajectory is predicted by a cooperation-based trajectory planning model (see Fig. 11.a). Both parts of the model are tested and validated using real-life recorded scenarios of pedestrian-vehicle interaction. The model is capable of describing and predicting agents’ behaviors when interacting with a vehicle in both lateral and frontal crossing scenarios. In early 2019, we used this cooperation based behavioral model to design a proactive longitudinal velocity controller. The suggested velocity control strategy enabled the vehicle to cooperate and proactively interact with its surrounding rather than merely reacting to the behavior of the pedestrians. This resulted in more natural driving patterns, avoiding the sub-optimal navigation solutions which result in over-reactive methods. The method is tested and validated in lateral interactions with a flow of pedestrians. The method showed a high gain in the time to reach the goal in comparison with reactive navigation, while ensuring the pedestrians safety. In 2020, we integrated the previous navigation system with the PedSim simulator under ROS. Next, we worked on a proactive and human-like maneuvering system to deploy the steering control part of the navigation system. We adapted a two-step approach. In the first step, the navigation space is explored proactively and an optimal navigation channel is found using a fuzzy logic model. This proactive dynamic channel is guaranteed to exist even in dense scenarios where obstacle free paths do not exist. In the second step, a human-like steering is adapted using a Quintic transition path and a sliding mode control approach. The resulting system is tested under ROS in combination with the longitudinal velocity controller and the cooperation-based behavioral model. The resulting system is validated in both frontal and lateral interactions with dense crowds (see Fig. 11.b). The system results in major advantage in the travel time avoiding the freezing of the vehicle, while ensuring the pedestrian’s safety. This work has been published in ITSC'20 30 and ICRA'20 31.
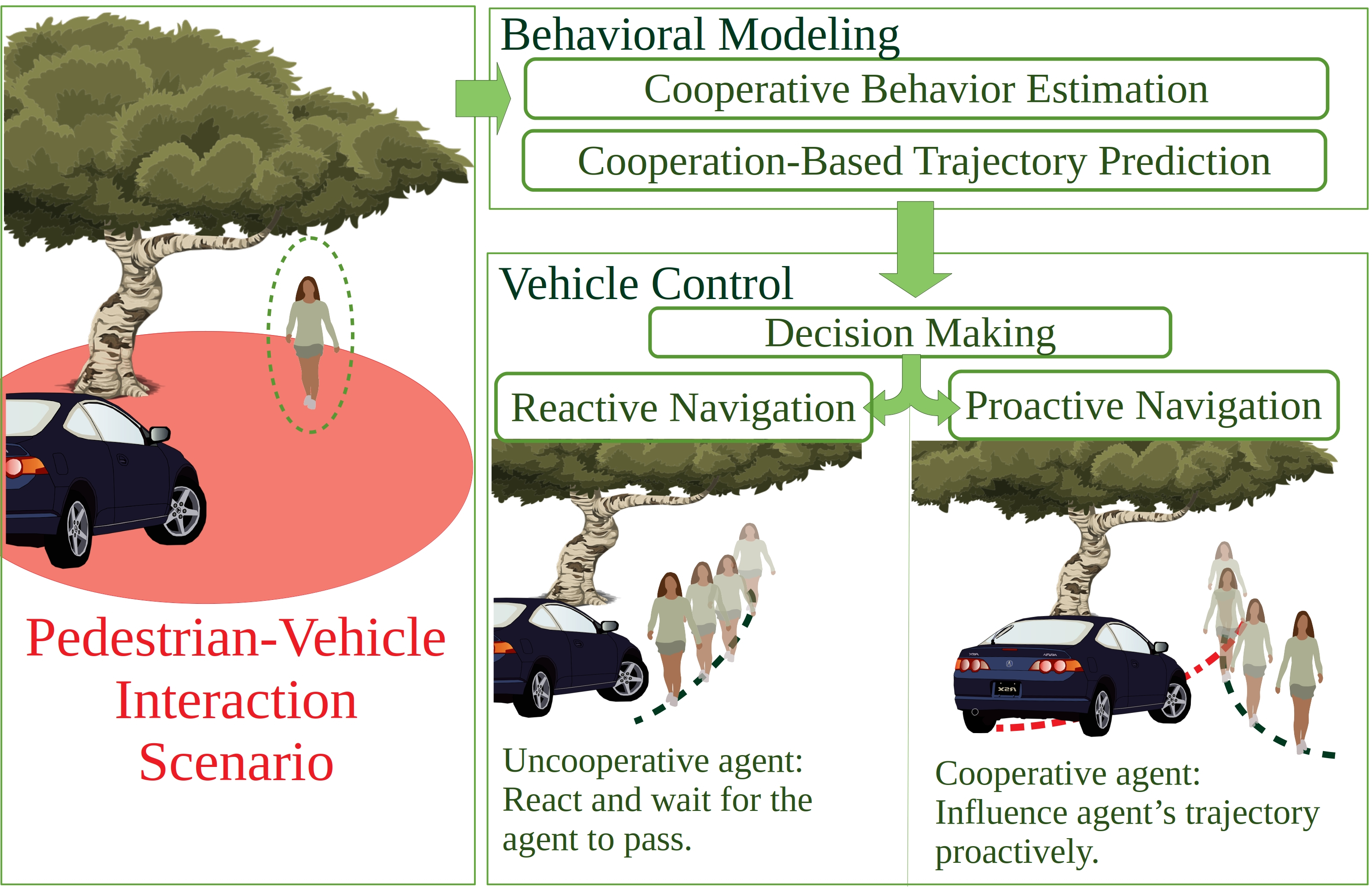
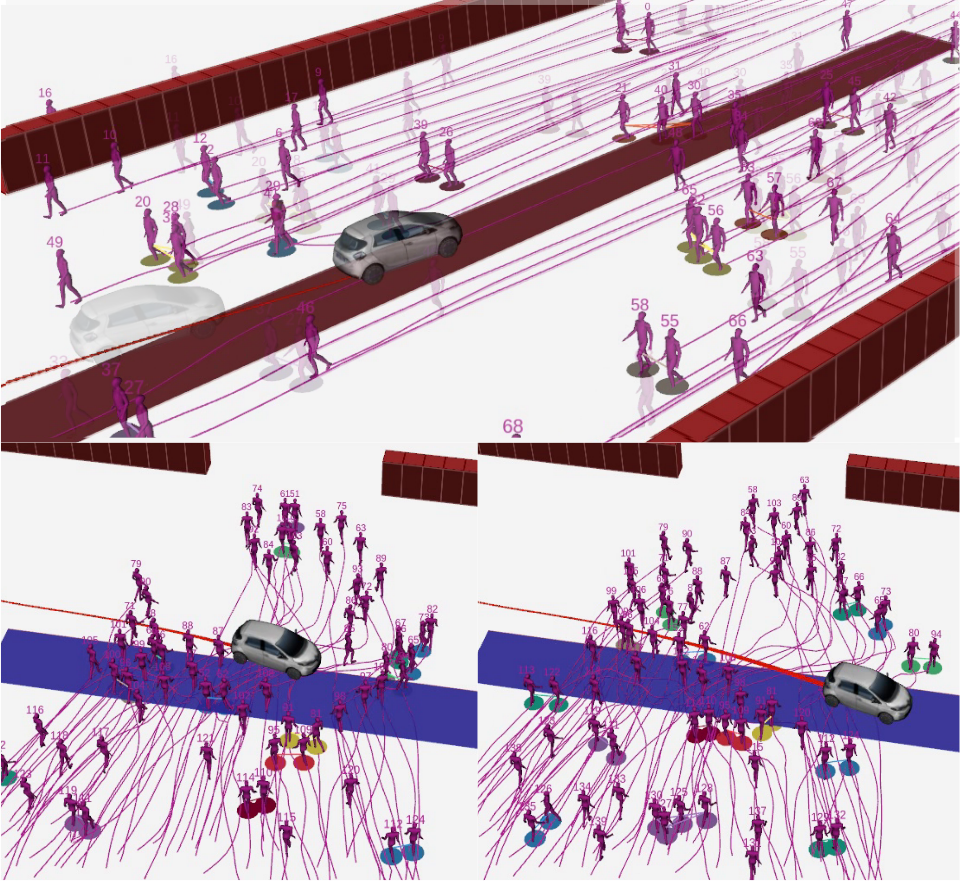
7.4.3 Deep Reinforcement Learning based Vehicle Navigation amongst pedestrians
Participants: Niranjan Deshpande, Anne Spalanzani, Dominique Vaufreydaz.
The objective of this work is to develop a navigation system for an autonomous vehicle in urban environments. The urban environment consists of other road users as well, including other vehicles and pedestrians (see Figure 12). Specifically, the focus is on the decision making (behaviour planning) aspect of navigation. In this work, we propose to use Deep Reinforcement Learning as a method to learn decision making. We have developed two Deep Q-Networks (DQN) based agents for decision making amongst pedestrians in a realistic simulator called CARLA. The first agent uses convolutional neural networks with DQN while the second agent incorporates prediction in decision making by using a LSTM layer after the CNN. We have published this work with CNN-LSTM DQN in ICARCV 2020 (24). Currently, we are thoroughly evaluating these two approaches and developing enhancements to incorporate a mixed traffic (pedestrians and vehicles together) scenario.
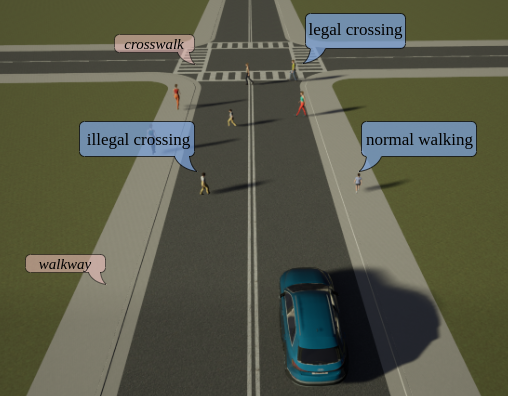
7.5 (Multi-)Robot Task and Motion Planning
7.5.1 Learning task-based motion planning
Participants: Edward Beeching, Pierre Marza, Christian Wolf, Jilles Dibangoye, Olivier Simonin, Laetitia Matignon.
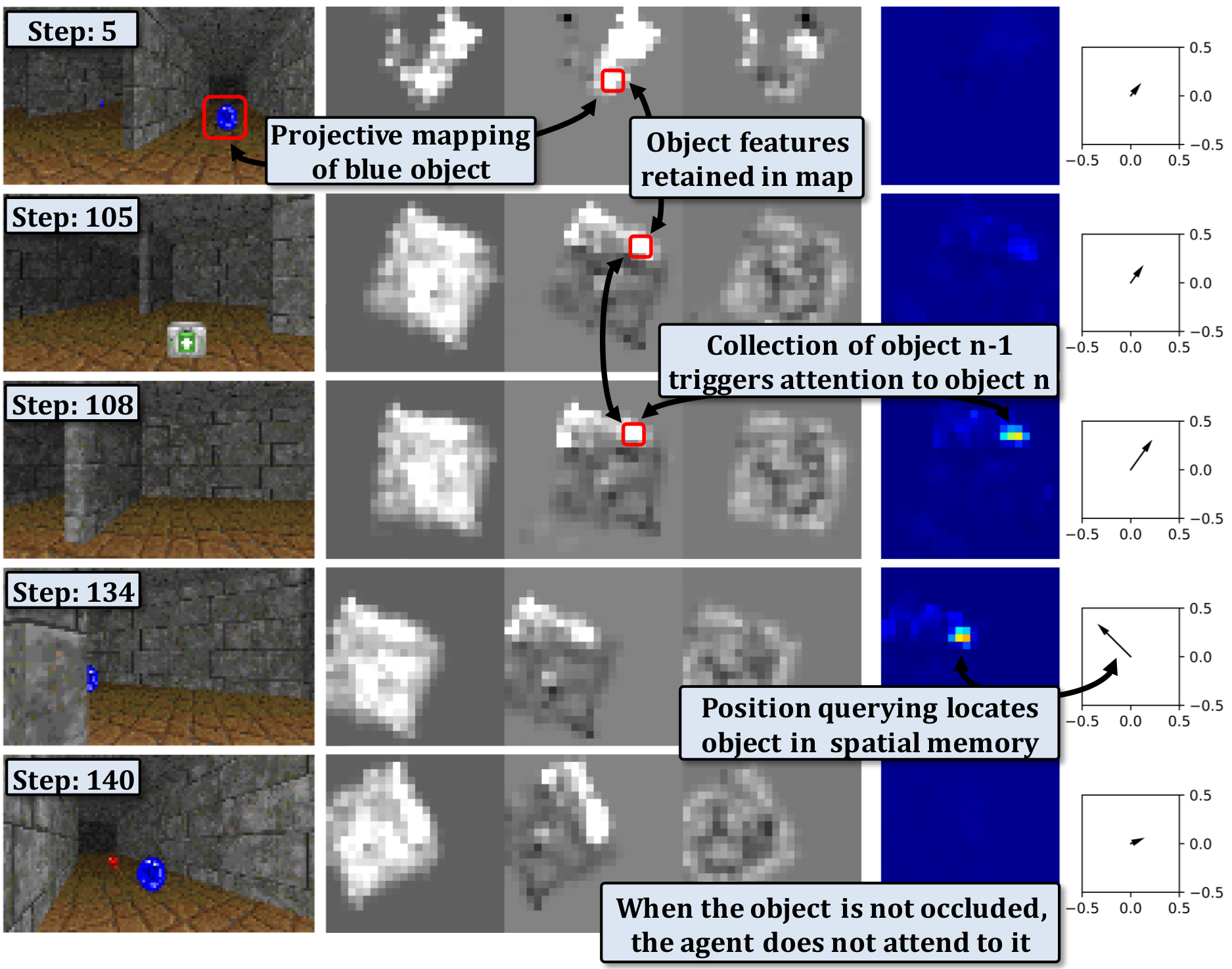
Analysis of the EgoMap for key steps (different rows) during an episode. Left column - RGB observations, central column - the three largest PCA components of features mapped in the spatially structured memory, right - attention heat map (result of the query) and x,y query position vector.
Our goal is the automatic learning of robot navigation in complex environments based on specific tasks and from visual input. The robot automatically navigates in the environment in order to solve a specific problem, which can be posed explicitly and be encoded in the algorithm (e.g. find all occurences of a given object in the environment, or recognize the current activities of all the actors in this environment) or which can be given in an encoded form as additional input, like text. Addressing these problems requires competences in computer vision, machine learning and AI, and robotics (navigation and paths planning).
A critical part for solving these kind of problems involving autonomous agents is handling memory and planning. An example can be derived from biology, where an animal that is able to store and recall pertinent information about their environment is likely to exceed the performance of an animal whose behavior is purely reactive. Many control problems in partially observed 3D environments involve long term dependencies and planning. Solving these problems requires agents to learn several key capacities: spatial reasoning (exploring the environment and learning spatio-temporal regularities) and semantic mapping (discovering semantics from interactions). While solutions exist for semantic mapping and semantic SLAM 60, 85, a more interesting problem arises when the semantics of objects and their affordances are not supervised, but defined through the task and thus learned from reward.
We started this work in the end of 2017, following the arrival of Christian Wolf and his 2 year delegation in the team between Sept 2017 and Sept. 2019. The underlying scientific challenge here is to automatically learn representations which allow the agent to solve multiple sub problems required for the task. In particular, the robot needs to learn a metric representation (a map) of its environment based from a sequence of ego-centric observations. Secondly, to solve the problem, it needs to create a representation which encodes the history of ego-centric observations which are relevant to the recognition problem. Both representations need to be connected, in order for the robot to learn to navigate to solve the problem.
Creating agents capable of high-level reasoning based on structured memory is main topic of the AI Chair "REMEMBER" obtained by C.Wolf in late 2019 and which involves O. Simonin, J. Dibangoye and L. Matignon (LIRIS/Univ Lyon 1). The chair is co-financed by ANR, Naver Labs Europe and INSA-Lyon.
PhD thesis of Edward Beeching (since 2018, Inria grant): In a first work we proposed a new 3D benchmark for Reinforcement learning, which requires high-level reasoning through the automatic discovery of object affordances. Then we proposed EgoMap, a spatially structured metric neural memory architecture integrating projective geometry in deep reinforcement learning, which we show to outperform classical recurrent baselines. In particular, we show that through visualizations that the agent learns to map relevant objects in its spatial memory without any supervision purely from reward (see Fig. 13). In 2020, we published these results in 22 (ICPR), 21 (ECCV) and 20 (ECML).
PhD thesis of Pierre Marza (since Dec. 2020). In the context of the REMEMBER AI Chair, P. Marza started a PhD on the subject "Large-scale learning of high-level navigation skills for autonomous agents in 3D environments" (supervized by C. Wolf, L. Matignon and O. Simonin). We propose to extend the advances to the sequential decision-making of agents in a context of planning and control in complex 3D environments. A key question is the ability to learn representations with minimal human intervention and annotation. It requires the efficient use of raw data and the discovery of patterns through different learning formalisms (supervised, unsupervised or self-supervised, by reward or intrinsic motivation).
7.5.2 Navigation Among Movable Obstacles : extension to multi-robot and social constraints
Participants: Benoit Renault, Olivier Simonin, Jacques Saraydaryan, Fabrice Jumel.
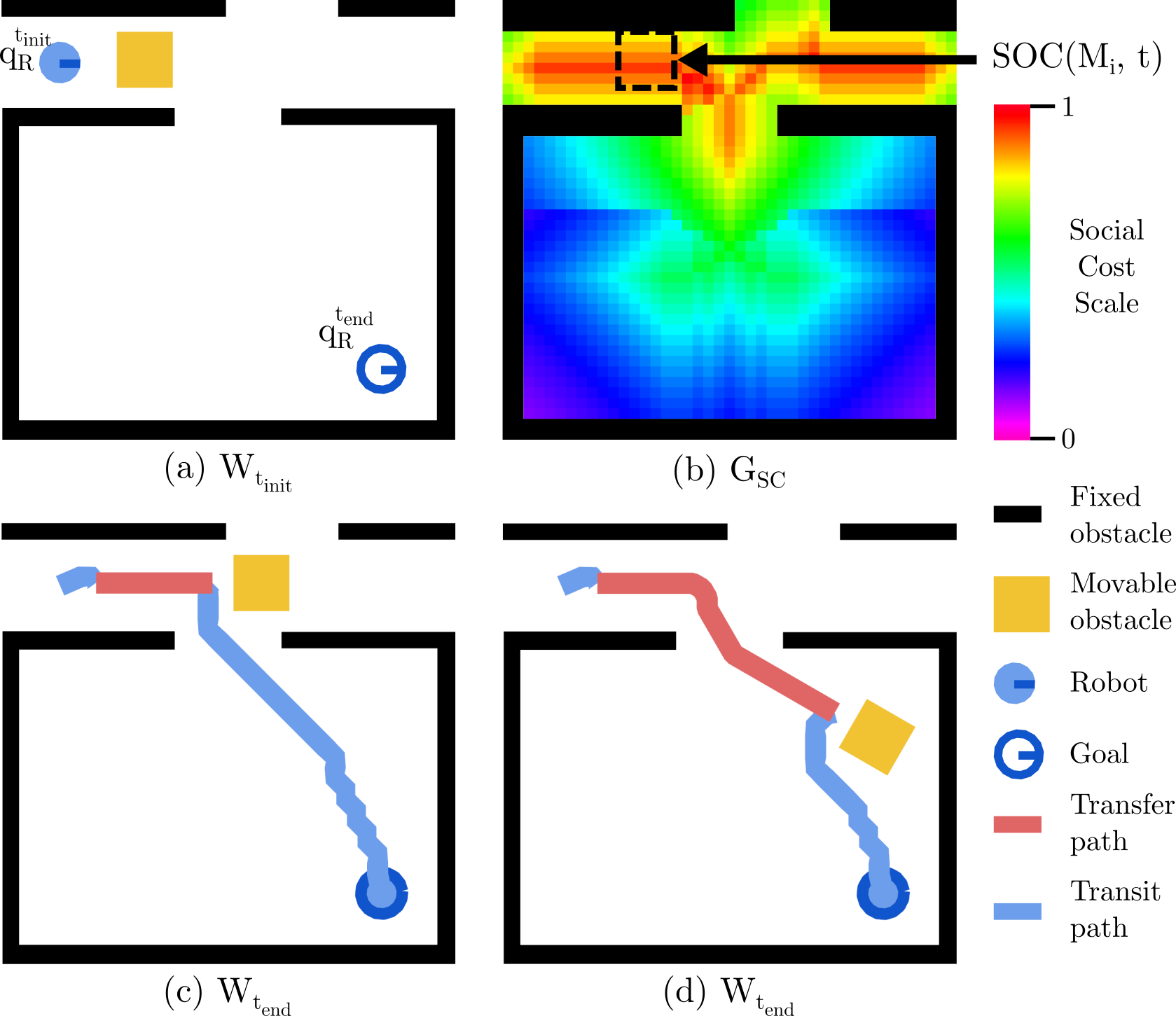
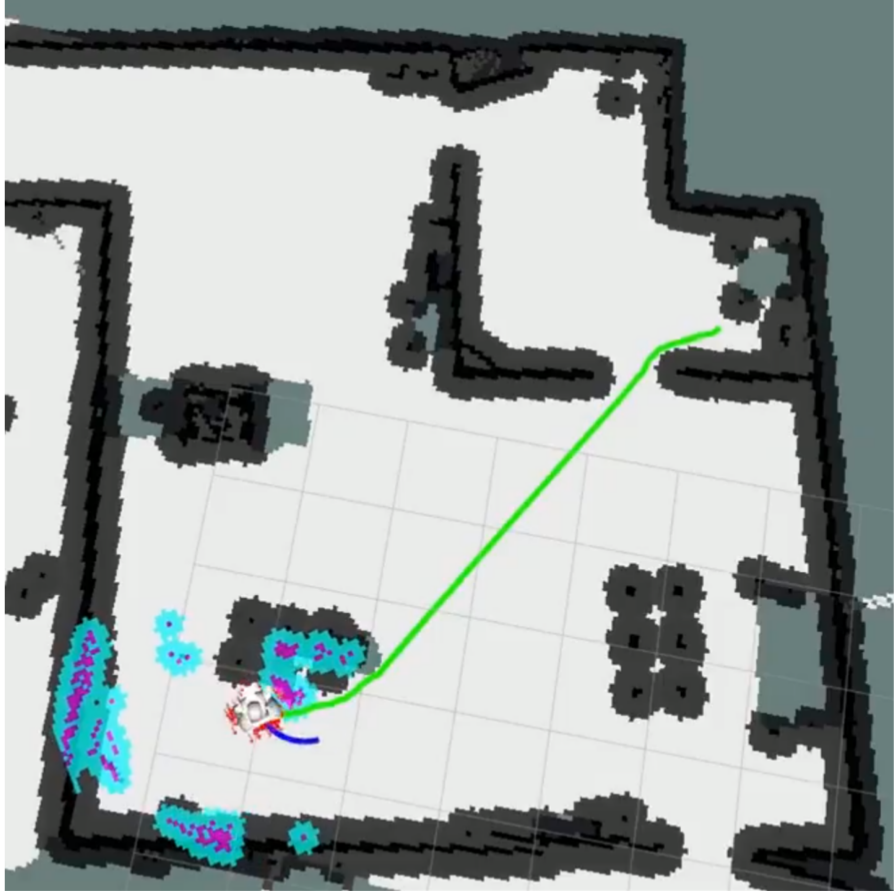
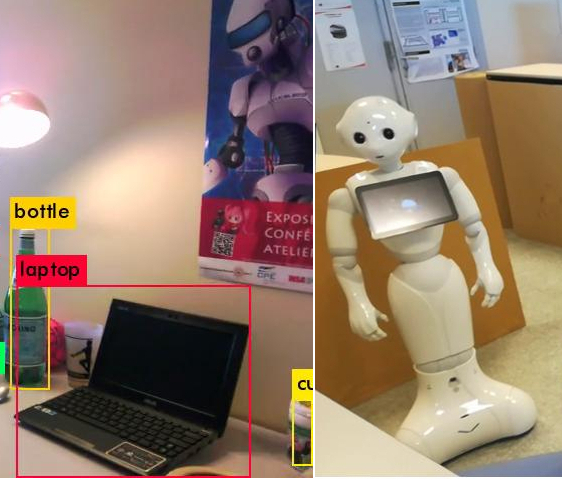
In 2018 we started to study the NAMO planning problem (Navigation Among Movable Obstacles). Since 2019, the PhD. thesis of Benoit Renault aims to extend NAMO in two directions :
- Defining Social-NAMO, by considering where obstacles should be optimally moved with regards to space access. We have defined new spatial cost functions and extended existing NAMO algorithms with the ability to maintain area accesses (connectivity) for humans and robots, see IROS publication 36. We also developed a simulator for NAMO, and its extensions, called S-NAMO-SIM (see Fig. 14.a).
- Defining Multi-agent NAMO (MA-NAMO). It consists in generalizing the problem to robots that can move the objects of the environment in order to reach their individual goal positions. We have proposed a first coordination strategy based on local rules. We have shown that considering the social cost we introduced with S-NAMO offers a significant gain for solving MA-NAMO because of the rearranging it provides.
In parallel of this work we continue to develop our activities in the context of the RoboCup international competition. The competition was canceled in 2020 and will be virtual in 2021. Our team, 'LyonTech' (INSA/Inria/CPE/UCBL), will participate to the @Home simulated category. We continue to investigate several issues to make humanoid robots (Pepper in our case) able to evolve in an indoor environment : decision making and navigation (Fig. 14.b), human and object recognition based on deep learning techniques (Fig. 14.c) and human-robot interaction.
7.5.3 Multi-Robot Path Planning with cable constraints
Participants: Xiao Peng, Christine Solnon, Olivier Simonin.
The research is done within the context of the european project BugWright2 that aims at designing an adaptable autonomous multi-robot solution for servicing ship outer hulls.
PhD thesis of Xiao Peng (since Nov. 2020). The goal of this PhD thesis is to design algorithms for planning a set of mobile robots, each attached to a flexible cable, to cover an area with obstacles. We started to work on multi-robot plath planning (MAPF) under the constraints brought by the cables (which extends the MAPF problem). That is planning robot paths from a set of initial positions to a set of target positions while avoiding cable entanglements.
7.6 Multi-Agent Sequential Decision-Making under Uncertainty
This research is the follow up of a subgroup led by Jilles S. Dibangoye carried out during the last five years, which include foundations of sequential decision making by a group of cooperative or competitive robots or more generally artificial agents. To this end, we explore combinatorial, convex optimization and reinforcement learning methods.
7.6.1 Optimally solving zero-sum games using centralized planning for decentralized control theory
Participants: Jilles S. Dibangoye, Olivier Buffet, Vincent Thomas, Abdallah Saffidine, Christopher Amato, François Charpillet.
During the last two years, we investigated deep and standard reinforcement learning for solving systems with multiple agents and different information structures. Our preliminary results include:
- (Theoretical) – As an extension of 63 in the competitive cases, we characterize the optimal solution of two-player fully and partially observable stochastic games.
- (Theoretical) – We further exhibit new underlying structures of the optimal solution for both non-cooperative two-player settings with information asymmetry, one agent sees what the other does and sees.
- (Algorithmic) – We extend a non-trivial procedure for computing such optimal solutions.
This work aims at reinforcing a recent theory and algorithms to optimally solving a two-person zero-sum POSGs (zs-POSGs). That is, a general framework for modeling and solving two-person zero-sum games (zs-Games) with imperfect information. Our theory builds upon a proof that the original problem is reducible to a zs-Game—but now with perfect information. In this form, we show that the dynamic programming theory applies. In particular, we extended Bellman equations 56 for zs-POSGs, and coined them maximin (resp. minimax) equations. Even more importantly, we demonstrated Von Neumann & Morgenstern’s minimax theorem 9089 holds in zs-POSGs. We further proved that value functions—solutions of maximin (resp. minimax) equations—yield special structures. More specifically, the optimal value functions are Lipchitz-continuous. Together these findings allow us to extend planning techniques from simpler settings to zs-POSGs. To cope with high-dimensional settings, we also investigated low-dimensional (possibly non-convex) representations of the approximations of the optimal value function. In that direction, we extended algorithms that apply for convex value functions to lipschitz value functions. This research direction lead to many publications in different avenues 41 along with technical reports 12.
7.6.2 Heuristic Search Value Iteration for zero-sum Stochastic Games
Participants: Jilles S. Dibangoye, Olivier Buffet, Vincent Thomas, Abdallah Saffidine.
This work investigates a simpler version of POSGs, see paper 12. In sequential decision-making, heuristic search algorithms allow exploiting both the initial situation and an admissible heuristic to efficiently search for an optimal solution, often for planning purposes. Such algorithms exist for problems with uncertain dynamics, partial observability, multiple criteria, or multiple collaborating agents. Here we look at two-player zero-sum stochastic games with discounted criterion, in a view to propose a solution tailored to the fully observable case, while solutions have been proposed for particular, though still more general, partially observable cases. This setting induces reasoning on both a lower and an upper bound of the value function, which leads us to proposing zsSG-HSVI, an algorithm based on Heuristic Search Value Iteration (HSVI), and which thus relies on generating trajectories. We demonstrate that, each player acting optimistically, and employing simple heuristic initializations, HSVI's convergence in finite time to an -optimal solution is preserved. An empirical study of the resulting approach is conducted on benchmark problems of various sizes.
7.6.3 Multiagent Planning and Learning As MILP
Participants: Jilles S. Dibangoye, Olivier Buffet, Akshat Kumar.
During the last year, we investigated a more practical way to optimally solving decentralized decision-making applications 42. The decentralized partially observable Markov decision process offers a unified framework for sequential decision- making by multiple collaborating agents but remains intractable. Mixed-integer linear formulations proved useful for partially observable domains, unfortunately existing applications restrict to domains with one or two agents. In this paper, we exploit a linearization property that allows us to reformulate nonlinear constraints from n-agent settings into linear ones. We further present planning and learning approaches relying on MILP formulations for general and special cases, including network-distributed and transition-independent problems. Experiments on standard 2-agent benchmarks as well as domains with a large number of agents provide strong empirical support to the methodology.
7.6.4 Optimally Solving Two-Agent Decentralized POMDPs Under One-Sided Information Sharing
Participants: Jilles S. Dibangoye, Xie Yuxuan, Olivier Buffet.
This work is the result of an effort to provide a strong theory for optimally solving decentralized decision-making problems, represented as decentralized partially observable Markov decision processes (Dec-POMDPs), with tractable structures 40. Optimally solving Dec-POMDPs under either full or no information sharing received significant attention in recent years. However, little is known about how partial information sharing affects existing theory and algorithms. This work addresses this question for a team of two agents, with one-sided information sharing, i.e. both agents have imperfect information about the state of the world, but only one has access to what the other sees and does. From the perspective of a central planner, we show that the original problem can be reformulated into an equivalent information-state Markov decision process and solved as such. Besides, we prove that the optimal value function exhibits a specific form of uniform continuity. We also present heuristic search algorithms utilizing this property and providing the first results for this family of problems.
7.6.5 Stable Reinforcement Learning
Participants: Jilles S. Dibangoye, Nicolas Marchand, Ahmad Hably, Estéban Carvalho, Vincent Andrieu, Daniele Astolfi, Giacomo Casadeic, Madiha Nadri, Samuele Zoboli.
This recent research project is at the intersection of three closely related research fields: optimal control, machine learning, and mobile robotics. In this project, we try to find the best compromise between control methodologies to provide stability guarantees and machine learning methodologies that can scale up and converge towards a locally optimal solution. Applications of this hybrid methodology concern mobile robotics, including the driving of drones at very high speed. Two PhD. theses have been started on this problem the last year.
PhD thesis of Estéban Carvalho (since Oct. 2019). The first thesis focuses on high-speed navigation for UAVs in collaboration with the laboratory GIPSA. The goal of this PhD is to develop safe and aggressive piloting algorithms for UAVs. Piloting algorithms will be designed on event-switched control coupled with AI routines to cope with unmodeled and uncertain part of the system to reach as much as possible the limits of aggressive flight.
PhD thesis of Samuele Zoboli (since Jul. 2020, ANR DELICIO). In control theory, a natural approach for the design of stabilizing control is to consider a linear approximation around the operating point of the dynamic system. In this way, it is possible to design control laws that are optimal and which guaranteed stability for the linearized system. However, this behavior is only guaranteed for the original system around this operating point (local approach). The aim of this PhD thesis is to develop new real time algorithms (contol law and estimation algorithms for dynamical systems) that combines tools coming from control theory and reinforcement learning. The problem under study will be to find means to achieve globalization of local optimal control laws and observers. The idea is to use reinforcement learning approaches in order to design a global law of control that continually “joins” the local and optimal control. A first step to achieve this goal, could be an approach to ensure that the first-order approximation of the obtained control coincides with the linear optimal control law. Another line of research that will be addressed is the observer design for nonlinear systems in two steps: 1) A local optimal filter design, 2) Its globalization following the same approach as in the control design. Kalman filters can be used as an example. The application example is the synthesis of synchronizing control laws for a UAV fleet. First, we consider the mono-agent problem. The multi-agent framework will be considered in a second step.
7.7 Multi-Robot Routing
7.7.1 Deep Multi-Agent Reinforcement for Vehicle Routing Problems
Participants: Guillaume Bono, Jilles Dibangoye, Laetitia Matignon, Olivier Simonin, Florian Peyreron.
This study was carried out as part of the PhD thesis of Guillaume Bono, which he defended on October 28th, 2020 45. The title of the thesis is "Deep Multi-Agent Reinforcement Learning for Dynamic and Stochastic Vehicle Routing Problems". This PhD was funded by the INSA-VOLVO Chair.
In his PhD, G. Bono studied how to apply Deep RL methods to rich Dynamic and Stochastic VRPs (DS-VRP) as multi-agent systems. Finding an efficient, compact and sufficiently expressive state representation is the key challenge in applying RL to complex combinatorial problems such as VRPs.
The main contributions of the thesis are :
- In the class of policy-based approaches in Multi-Agent RL, we extended the mathematical foundation of Actor-Critic methods for Decentralized Partially Observable MDPs (Dec-POMDPs) to a somewhat new paradigm inspired by planning called Centralized Training for Decentralized Control (CTDC).
- To address multi-agent planning where only one agent decide to act at a time, like in VRP problems, we introduced a new sequential multi-agent model called sMMDP (seq. Multi-Agent MDP). Once we established the classical Bellman equations, we experimented on its performance compared to the existing MMDP.
- We used sMMDP to model a rich DS-VRP. To represent the state of the customers and the vehicles in this new model, we designed a new modular policy network we called MARDAM (Multi-Agent Routing Deep Attention Mechanisms).
- We developed a set of artificial benchmarks showing the flexibility, the robustness and the generalization capabilities of MARDAM compared to OR standard VRP solvers.
All these results were published in IEEE Transactions on Intelligent Transportation Systems 11.
7.7.2 Integration of traffic conditions
Participants: Nicolas Chiabaut, Jilles S. Dibangoye, Romain Fontaine, Omar Rifki, Christine Solnon.
In classical vehicle routing problems, travel times between locations to visit are assumed to be constant. This is not realistic because traffic conditions are not constant throughout the day, especially in a urban context. As a consequence, quickest paths (i.e., successions of road links), and travel times between locations may change along the day. To fill this lack of realism, cost functions that define travel times must become time-dependent. These time-dependent cost functions are defined by exploiting data coming from sensors, and the frequency of the measures as well as the number and the position of the sensors may have an impact on tour quality. In 17, we study this impact for various vehicle routing problems, using a micro-simulation software of the Lyon conurbation to obtain realistic data. In particular, we show that exploiting time-dependent data has a strong impact on the satisfaction of time window constraints, especially when they are tight.
PhD thesis of Romain Fontaine (since Oct. 2020). State-of-the-art approaches for solving vehicle routing problems hardly scale when considering time-dependent cost functions. The goal of the PhD thesis of Romain is to design new algorithms based on dynamic programming. Constraints (such as, for example, time-windows or pickup-and-delivery constraints) are exploited to prune states, and new bounds and heuristics are designed to guide the search.
7.8 Swarm of UAVs : control, tasks and communication
7.8.1 Multi-UAV Exploration and Visual Coverage of 3D Environments
Participants: Alessandro Renzaglia, Olivier Simonin, Jilles Dibangoye, Vincent Le Doze.
Multi-robot teams, especially when involving aerial vehicles (UAVs 31), are extremely efficient systems to help humans in acquiring information on large and complex environments. In these scenarios, two fundamental tasks are static coverage (for observation) and exploration (for mapping). In both cases, the robots' goal is to navigate through the environment and cooperate to maximize the observed area, either by finding the optimal static configuration which provides the best global view in the case of the coverage or by maximizing the new observed areas at every step until the environment becomes completely known in the case of the exploration.
Although these tasks are usually considered separately in the literature, we proposed a common framework where both problems are formulated as the maximization of online acquired information via the definition of single-robot optimization functions, which differs only slightly in the two cases to take into account the static and dynamic nature of coverage and exploration respectively 7 (JINT journal). A common derivative-free approach based on a stochastic approximation of these functions and their successive optimization is proposed, resulting in a fast and decentralized solution. The locality of this methodology limits however this solution to have local optimality guarantees and specific additional layers are proposed for the two problems to improve the final performance.
For the exploration problem, this resulted in a novel decentralized approach which alternates gradient-free stochastic optimization and a frontier-based approach 81 (IROS'19). Our method allows each robot to generate its own trajectory based on the collected data and the local map built integrating the information shared by its teammates. Whenever a local optimum is reached, which corresponds to a location surrounded by already explored areas, the algorithm identifies the closest frontier to get over it and restarts the local optimization. Its low computational cost, the capability to deal with constraints and the decentralized decision-making make it particularly suitable for multi-robot applications in complex 3D environments.
In the case of visual coverage, we studied how suitable initializations for the UAVs' positions can be computed offline based on a partial knowledge on the environment and how they can affect the final performance of the online measurements-based optimization. The main contribution of this work was thus to add another layer, based on the concept of Centroidal Voronoi Tessellation, to the optimization scheme in order to exploit an a priori sparse information on the environment to cover. The resulting method, taking advantages of the complementary properties of geometric and stochastic optimization, significantly improves the result of the uninitialized solution and notably reduces the probability of a far-to-optimal final configuration. Moreover, the number of iterations necessary for the convergence of the on-line algorithm is also reduced 7.
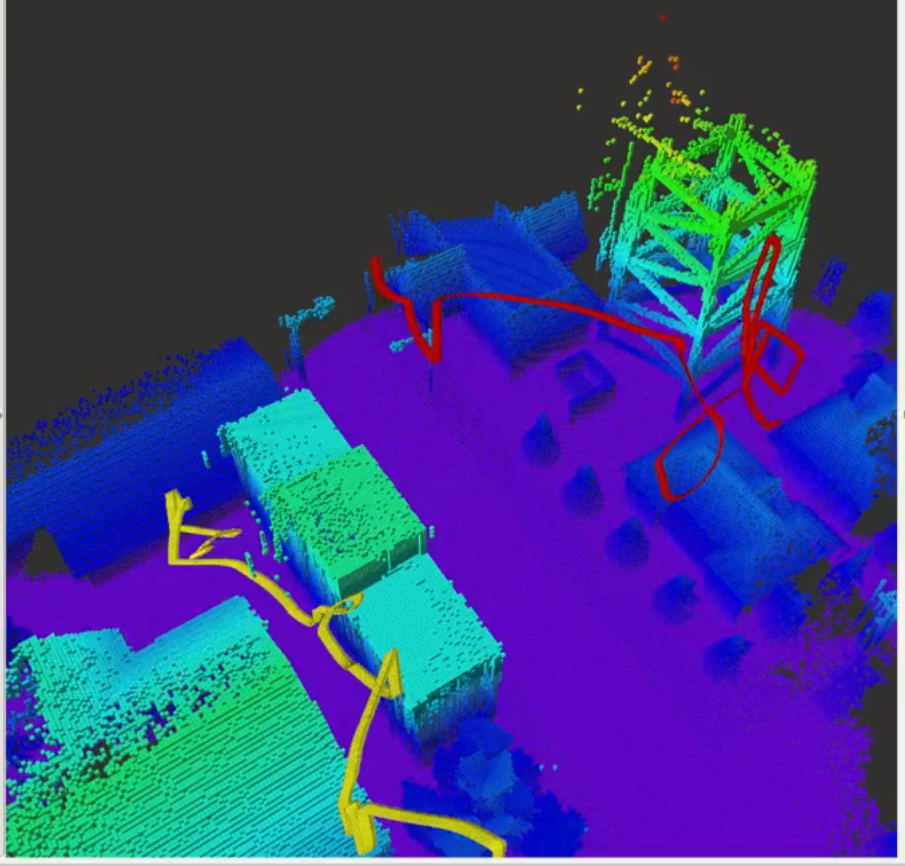
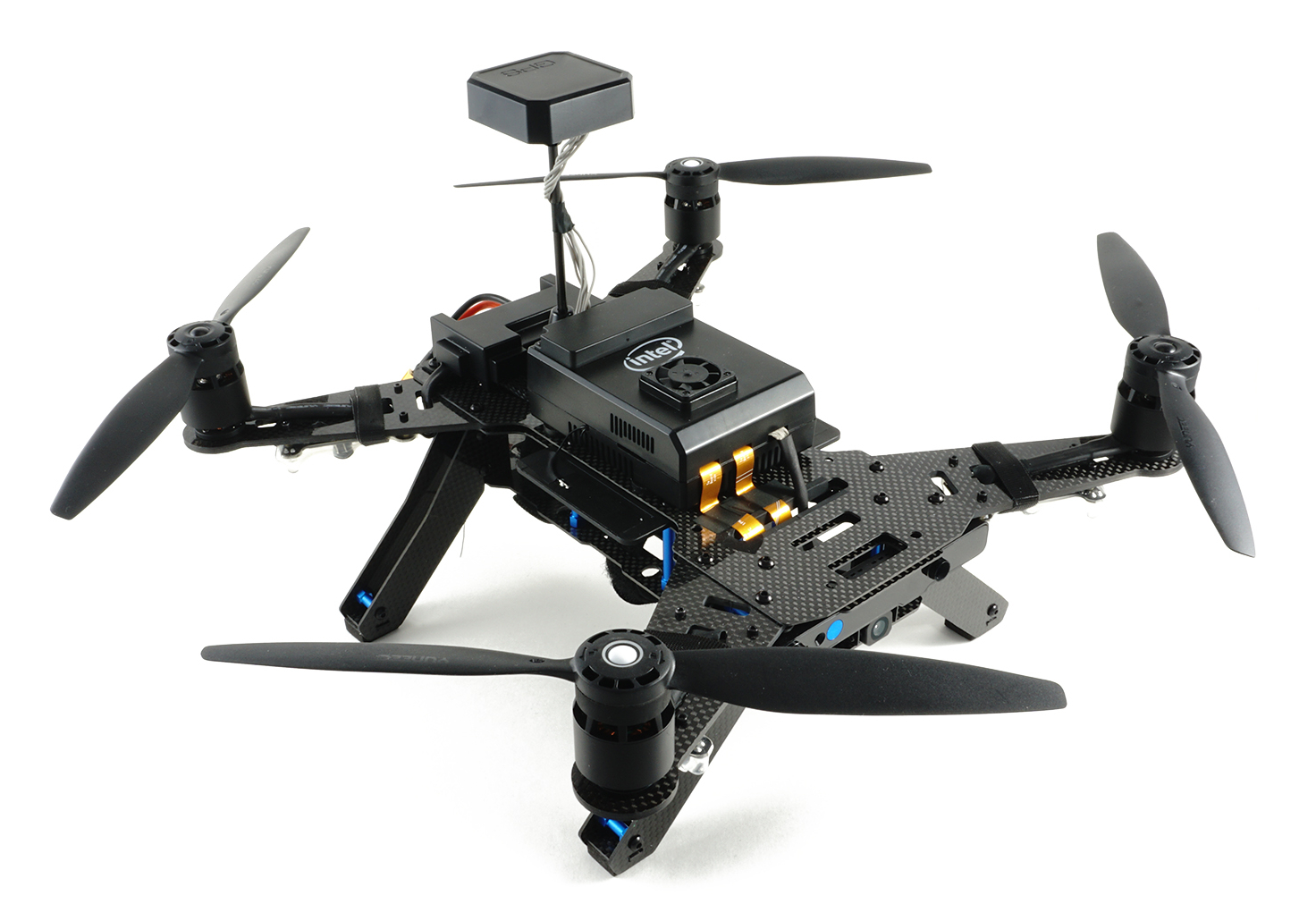
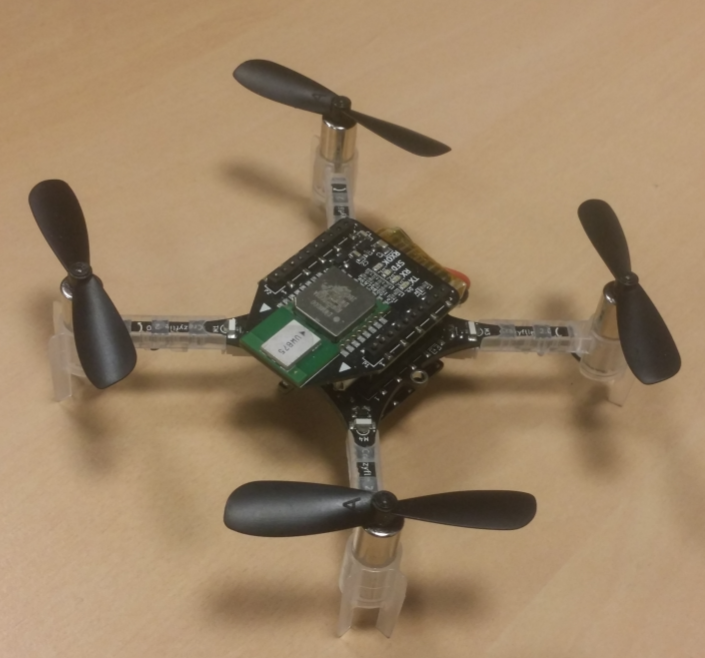
Both previous approaches have been tested in realistic simulations based on our extension of Gazebo, called SimuDronesGR (see Fig. 15.a). The development of this UAVs simulator, which includes realistic models of both the environment and the aerial vehicle's dynamics and sensors, is an important current activity in Chroma. Such a simulator has the fundamental role of allowing for realistic tests to validate the developed algorithms and to better prepare the implementation of these solutions on the robotic platform of the team (Intel Aero quadrotors, Fig. 15.b) for real experiments.
7.8.2 Controlled mobility & communication optimization in swarm of UAVs
Participants: Remy Grunblatt, Isabelle Guerin-Lassous, Olivier Simonin.
Intel WiFi controllers are used in many common devices, such as laptops, but also in the Intel Aero Ready-to-Fly UAVs (Unmanned Aerial Vehicle). The mobility capabilities of these devices lead to greater dynamics in radio conditions, and therefore introduce a need for a suitable and efficient rate adaptation algorithm.
This work has been conduced during the PhD of Remy Grunblatt, which he defended on January 8th 2021 46. We evaulated wifi performances in order to optimize its use in a fleet of mobile robots, such as UAVs. This PhD, intituled "From WiFi Performance Evaluation to Controlled Mobility in Drone Networks" was funded by Inria & DGA and co-supervised by I. Guerin-Lassous and O. Simonin. The main contributions of this work are :
- We have reverse-engineered the Intel rate adaptation mechanism from the source code of the IwlWifi Linux driver, and we have given, in a comprehensive form, the underlying rate adaptation algorithm named Iwl-Mvm-Rs. We have also implemented the Iwl-Mvm-Rs algorithm in the NS-3 simulator. Thanks to this implementation, we have evaluated the performance of Iwl-Mvm-Rs in different scenarios (static and with mobility, with and without fast fading). We also compared the performances of Iwl-Mvm-Rs with the ones of Minstrel-HT and IdealWifi, also implemented in the NS-3 simulator. This work has been published in 2019 ACM MSWiM conference (A) 67.
- We proposed a controlled mobility solution which leverages the antenna radiation pattern of the drones. The approach optimizes, online, antenna orientation following a local search approach. This efficiency of the algorithm was evaluated thanks to a customized simulation framework for antenna and drone simulation, based on NS-3. This solution, which works with any rate adaptation algorithm, is distributed, and do not require a global coordination that would be costly. It also does not require a full and complete control of the drone mobility as existing controlled mobility solutions require, which makes this solution compatible with various applications. This work has been published in 2020 ACM MSWiM 29.
7.8.3 Decentralized control of swarm of UAVs : Flocking models
Participants: Alexandre Bonnefond, Isabelle Guerrin-Lassous, Olivier Simonin, Vincent Dufour.
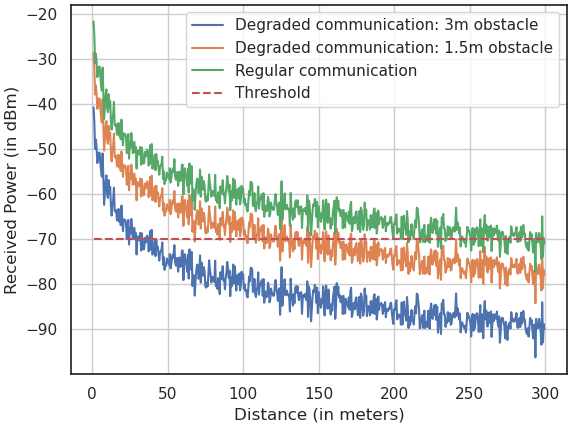
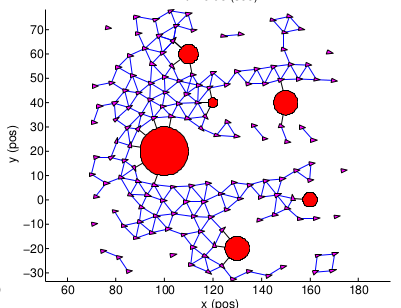
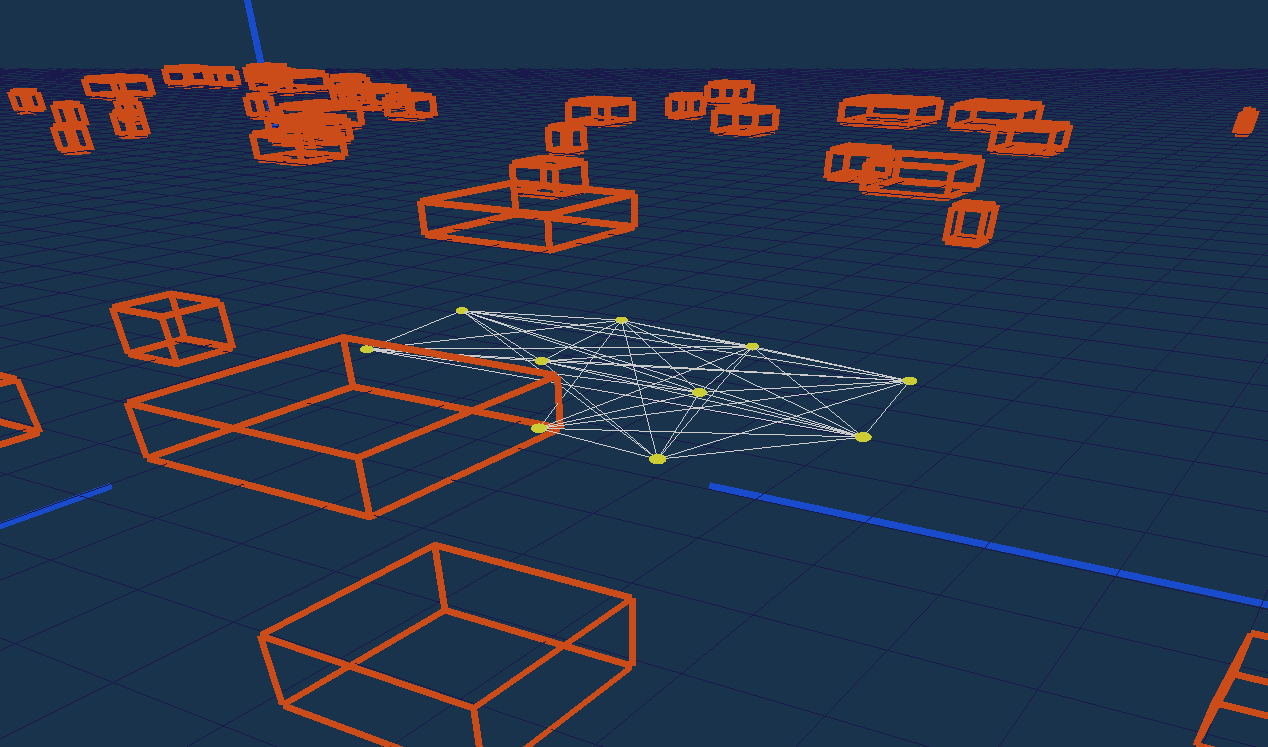
This work is conduced through the Inria/DGA "AI" project "DYNAFLOCK" (2019-22). It funds the PhD thesis of Alexandre Bonnefond.
In this work, we study existing flocking models (decentralized swarm navigation) and propose extensions to improve their abilities to deal with environments having obstacles impacting the communication quality. Often depicted as robust systems, there is yet a lack of understanding how flocking models compare and how they are impacted by the communication quality when they exchange control data.
- First, by taking into account the radio propagation, we modeled the obstacles’ impact on communications in a simulator that we use to optimize flocking parameters (see. Fig. 16 a,b).
- Second, we extended and combined two standard models, Olfati-Saber 32 and Vásárhelyi et al. 33 (see Fig. 16 b,c), to improve their ability to stay connected while evolving in environments with different obstacles distributions. The simulation results show the efficiency of the proposed models and how they adapt to different environmental constraints.
These works have been submitted to IROS int. conference and JFSMA nat. conference.
In the context of the DYNAFLOCK project we will acquire a new UAVs platform (spring 2021) in order to implement and experiment some flocking models, outdoor. Vincent Dufour, who joined Chroma in the end of 2020 as research engineer, is in charge of the 3D simulations (with Gazebo) and the experiments with real drones.
7.9 Constraint Programming
Participants: Maxime Chabert, Lucas Groleaz, Samba Ndojh Ndiaye, David Pisinger, Loïc Rouquette, David Sacramento, Christine Solnon.
In many of our applications, we have to solve constrained optimization problems (COPs) such as vehicle routing problems, or multi-agent path finding problems, for example. These COPs are usually NP-hard, and we study and design algorithms for solving these problems. We more particularly study approaches based on Constraint Programming (CP), that provides declarative languages for describing these problems by means of constraints, and generic algorithms for solving them by means of constraint propagation.
In 18, 28, and 27, we consider scheduling problems, and we propose new hybrid approaches that combine CP solvers with metaheuristics (Local Search and Ant Colony Optimization).
In 13, we introduce a new global constraint dedicated to exact cover problems, and we design new algorithms for efficiently propagating this constraint. We experimentally show the interest of this global constraint for solving conceptual clustering problems.
PhD thesis of Loïc Rouquette (since Oct. 2019). This thesis is funded by the ANR project DeCrypt, that aims at designing declarative languages (based on CP) for solving cryptanalysis problems. During the first year of his thesis, Loïc has designed new algorithms for efficiently propagating xor equations, which are widely used in symmetric bloc ciphers (such as the AES, for example). These algorithms are described in 37, and they are integrated in the Choco CP library.
8 Bilateral contracts and grants with industry
8.1 Bilateral contracts with industry
8.1.1 VOLVO-Renault Trucks Group (2016-2020)
Participants: Olivier Simonin, Jilles Dibangoye, Guillaume Bono, Mohamad Hobballah, Laetitia Matignon.
This collaboration has been built inside the INSA-VOLVO Chair, led by Prof. Didier Remond (INSA). In this context, the Chair funds the PhD Thesis of Guillaume Bono (2016-2020) in Chroma. The objective is to study how machine learning techniques can deal with optimization of goods distribution using a fleet of autonomous vehicles. In the following of the first results, VOLVO proposed to extend our collaboration by funding a Post-doc position concerning good distribution with platoons of autonomous vehicles. This is the Post-Doc of Mohamad Hobballah (02/2018-02/20).
8.1.2 Toyota Motor Europe (2006 - 2022)
Participants: Christian Laugier, David Sierra González, Özgür Erkent, Jilles Dibangoye, Christian Wolf.
The contract with Toyota Motors Europe is a joint collaboration involving Toyota Motors Europe, Inria and ProbaYes. It follows a first successful short term collaboration with Toyota in 2005. This contract aims at developing innovative technologies in the context of automotive safety. The idea is to improve road safety in driving situations by equipping vehicles with the technology to model on the fly the dynamic environment, to sense and identify potentially dangerous traffic participants or road obstacles, and to evaluate the collision risk. The sensing is performed using sensors commonly used in automotive applications such as cameras and lidar.This collaboration has been extended in 2018 for 4 years (period 2018-2021) and Toyota provides us with an experimental vehicle Lexus equipped with various sensing and control capabilities. Several additional connected technical contracts have also been signed, and an exploitation license for the CMCDOT software has been bought by Toyota in 2018.
8.2 Bilateral grants with industry
8.2.1 Renault (2019 - 2022)
Participants: Luiz Alberto Guardini Serafim, Christian Laugier, Anne Spalanzani, Philippe Martinet.
This contract was linked to the PhD Thesis of Luiz Alberto Guardini Serafim (Cifre Thesis). The objective is to address the open problem of emergency obstacle avoidance in complex traffic situations (for ADAS or AD applications) using planning in grid occupancy.8.2.2 IRT Nanoelec – Security of Autonomous Vehicles project (2018 - 2020)
Participants: Christian Laugier, Lukas Rummelhard, Jerome Lussereau, Jean-Alix David, Thomas Genevois, Nicolas Turro.
Security of Autonomous Vehicles is a project supported by ANR in the scope of the program PULSE of IRT Nanoelec. The objective of this project is to integrate, develop and promote technological bricks of context capture, for the safety of the autonomous vehicle. Building on Embedded Bayesian Perception for Dynamic Environment, Bayesian data fusion and filtering technologies from sets of heterogeneous sensors, these bricks make it possible to secure the movements of vehicles, but also provide them with an enriched and useful representation for autonomy functions themselves. In this context, various demonstrators embedding those technology bricks are developed in cooperation with industrial partners.9 Partnerships and cooperations
9.1 International initiatives
Informal international partners
- Robotics and Perception Group, University of Zurich, Switzerland, A. Martinelli have collaborations with Prof. Davide Scaramuzza.
- Univ. New South Wales, Australia, J. Dibangoye have collaborations with Abdallah Saffidine (PhD. Research Associate).
- Northeastern University, US., J. Dibangoye have collaborations with Christopher Amato (Assist. Professor).
9.2 European initiatives
9.2.1 FP7 & H2020 Projects
The H2020 "BugWright2" project (2020-24)
Title : "Autonomous Robotic Inspection and Maintenance on Ship Hulls and Storage Tanks".
BugWright2 is an ICT 'Robotics in Application Areas' project, funding 10M€, and led by Cedric Pradalier (CNRS, GeorgiaTech Metz). Chroma is partner and coordinates the WP6 on multi-robot systems.
- O. Simonin leads the "Multi-Robot Inspection" work-package (WP6) (main partners are LakeSide lab. Austria, CNRS-GeorgiaTech Metz and INSA Lyon/Inria Chroma & Agora)
- Chroma works on multi-robot planning with cable constraints (Xiao Peng PhD thesis) and on structure inspection with UAV fleets. The Agora team (CITI/Inria/INSA Lyon) is also involved (H. Rivano, O. Iova) and works on communication architecture and localization based on Ultra-WideBand technology.
- Funding for Chroma & Agora teams : 600K€
-
https://
cordis. europa. eu/ project/ id/ 871260
9.2.2 Collaborations with major European organizations
- ETHZ, Zurich, Autonomous System laboratory, (Switzerland)
- University of Zurich, Robotics and Perception Group (Switzerland) Vision and IMU data Fusion for 3D navigation in GPS denied environment.
- Karlsruhe Institut fur Technologie (KIT, Germany) Autonomous Driving.
- Lakeside Labs LSL (Self-Orgazing Networked Systems), Klagenfurt and University of Klagenfurt (Austria).
- Vislab Parma (Italy) Embedded Perception & Autonomous Driving (visits, projects submissions, and book chapter in the new edition of the Handbook of Robotics).
9.3 National initiatives
9.3.1 ANR
EquipEx+ "TIRREX" (2021-30)
Title : "Technological Infrastructure for Robotics Research of Excellence" ( 12M€).
TIRREX is led by CNRS and involves 32 laboratories. Chroma is involved in two research axes :
- "Aerial Robotics", Chroma/CITI lab. is a major partner of this axis (INSA Lyon & Inria)
- "Autonomous land robotics", Chroma/CITI lab. is a secondary partner of this axis (INSA Lyon & Inria).
The ANR "CONCERTO" (2020-23)
Title : mobilité Contrôlée et cOmmunicatioNs effiCaces dans une flottE de dRones auTo-Organisée
The project is led by Isabelle Guerin-Lassous (LIP/Inria Dante).
Partners : LIP (UCBL/Inria Dante), CITI (INSA/Inria Chroma), LS2N (CNRS, Nantes), LORIA (UL, Nancy), Alerion (company in Nancy).
Funding : 300K€ - (ASTRID ANR)
Chaire IA "REMEMBER" (2020-23) (Chair of research and teaching in artificial intelligence)
Title : Learning Reasoning, Memory and Behavior (funding 574K€)
This Chair is led by Christian Wolf (INSA Lyon/LIRIS lab.).
Partners : O. Simonin and J. Dibangoye from CHROMA, and L. Matignon from LIRIS/Lyon 1.
ANR JCJC "Plasma" (2019-2022)
The ANR JCJC Plasma, led by Jilles S. Dibangoye, aims at developing a general theory and algorithms with provable guarantees to treat planning and (deep) RL problems arising from the study of multi-agent sequential decision-making, which may be described as Partially Observable Stochastic Games (POSG), see Figure 1. We shall contribute to the development of theoretical foundations of the fields of intelligent agents and MASs by characterizing the underlying structure of the multi-agent decision-making problems and designing scalable and error-bounded algorithms. The research group is made of four senior researchers, O. Simonin, C. Wolf (INSA Lyon), F. Charpillet (INRIA Nancy) and O. Buffet (INRIA Nancy), and two junior researchers Jilles S. Dibangoye and A. Saffidine (Univeristy of New South Whales). We plan to hire one PhD and one post-doc for two years as well as internships. We received a support for 42-months starting in March 2020 with a financial support of about 254K euros.
ANR "Delicio" (2019-2022)
The ANR Delicio (Data and Prior, Machine Learning and Control), led by C. Wolf (INSA Lyon, LIRIS), proposes fundamental and applied research in the areas of Machine Learning and Control with applications to drone (UAV) fleet control. The consortium is made of 3 academic partners: INSA-Lyon/LIRIS, INSA-Lyon/CITI (Chroma team), University Lyon 1/LAGEPP, and ONERA. We received a support for 48-months starting in October 2019 with a financial support of about 540K euros.
ANR "HIANIC" (2018-21)
The HIANIC project, led by A. Spalanzani, proposes to endow autonomous vehicles with smart behaviors (cooperation, negotiation, socially acceptable movements) that better suit complex SharedSpace situations. It will integrate models of human behaviors (pedestrian, crowds and passengers), social rules, as well as smart navigation strategies that will manage interdependent behaviors of road users and of cybercars. The consortium is made of 3 academic partners: Inria (RITS, Chroma, Pervasive Interaction teams), LIG Laboratory (Hawai team) and LS2N laboratory (ARMEN and PACCE teams).
PIA Ademe "CAMPUS" (2017-20)
The CAMPUS project aims to identify, develop and deploy new functions for the autonomous cars in urban environments. In this project, Chroma will focus on finding solutions to navigate in complex situations such as crowded environments or dense traffic. The consortium is made of 1 academic partner: Inria (Rits and Chroma teams) and 3 companies: Safran electronics, Gemalto and Valeo.
9.3.2 FUI Projects
FUI Tornado (2017 – 2020)
Participants: Rabbia Asghar, Anne Spalanzani, Christian Laugier, Olivier Simonin.
The project Tornado is coordinated by Renault. The academic partners of the project are Inria Grenoble-Rhône Alpes, UTC, Institut Pascal, University of Pau, IFSTTAR. The industrial and application partners are Renault, Easymile, Neavia, Exoskills, 4D-Virtualiz, MBPC and Rambouillet Territoires. The objective of the project is to demonstrate the feasibility of a mobility service systems operating in the commercial zone of Rambouillet and on some public roads located in its vicinity, with several autonomous cars (Autonomous Renault Zoe). The IRT Nanoelec is also involved in the project as a subcontractor, for testing the perception, decision-making, navigation and controls components developed in the project.FUI STAR (2018 – 2021)
Participants: Andres Gomez Hernandez, Olivier Simonin, Christian Laugier.
The Project STAR is coordinated by IVECO. The academic partners of the project are Inria Grenoble-Rhône-Alpes, IFSTTAR, ISAE-Supaéro. The industrial and application partners are IVECO, Easymile, Transpolis, Transdev and Sector Groupe. The goal of the project is to build an autonomous bus that will operate on a safe lane Inria is involved in helping design situation awareness perception, especialy in special case like docking at the bus stop and handling dynamicity of any obstacle. The IRT Nanoelec is also involved in the project as a subcontractor, for testing the perception, decision-making, navigation and controls components developed in the project.9.3.3 DGA/INRIA AI projects
"DYNAFLOCK" (2019-2023)
Participants: Alexandre Bonnefond, Olivier Simonin, Isabelle Guerin-Lassous, Vincent Dufour.
The DYNAFLOCK project, led by O. Simonin, aims to extend flocking-based decentralized control of swarm of UAVs by considering the link quality between communicating entities. The consortium is made of 2 Inria teams from Lyon : Chroma and Dante (involving Prof. I. Guerin-Lassous). The PhD student (Alexandre Bonnefond) recruited in this project aims at defining dynamic flocking models based on the link quality. In 2020, an engineer will be recruited to conduct experiments with a quadrotors platform.
Funding of Dynaflock : 250 K€.
9.3.4 Regional Initiatives
Inria ADT 'COLOC' (2019-20)
Participants: Vincent Le Doze, Olivier Simonin, Jilles Dibangoye, Alessandro Renzaglia.
The COLOC ADT, which follows the CORDES ADT (2017-19), aims to coordinate a team of UAVs using both SLAM techniques and communication-based localization, considering outdoor urban environments. These ADT are coordinated by Olivier Simonin. They fund an Inria expert engineer position in Chroma (Vincent Le Doze, 10/17-11/20) focusing on UAVs control and localization. The project provides both a 3D simulator of UAV fleets (SimuDronesGR) and a new experimental platform exploiting IntelAero UAVs. In the end of the COLOC ADT, Vincent Le Doze proposed the creation of the Startup CrowFox.
WIFI-Drones project, FIL (Federation d'Informatique de Lyon), 2019-21
Participants: Remy Grunblatt, Isabelle Guerin-Lassous, Olivier Simonin.
Project between two teams of two laboratories from Lyon : DANTE (LIP) and CHROMA (CITI), entitled "Performances des communications Wi-Fi dans les réseaux de drones : une approche expérimentale", 2019-2021. Leader : I. Guerin-Lassous & O. Simonin.
The project aims to experimentally evaluate the Wireless communication in UAVs fleet scenarios. We consider the recent version of Wi-Fi based on 802.11n and 802.11 ac. Experimental measures will be used to build propagation models in order to be integrated in UAVs fleet simulations (in particular with Gazebo and NS3 simulators).
10 Dissemination
10.1 Promoting scientific activities
10.1.1 Scientific events: organisation
General chair, scientific chair
- C. Laugier co-organized the PPNIV 2020 Workshop at IEEE IROS 2020 Las Vegas. Virtual Scientific Event. 90 participants.
- C. Laugier co-organized with Prof Danwei Wang (NUS Singapore) 2 special sessions on Intelligent Mobile Robots at IEEE ICARCV 2020 Singapore. Virtual Scientific Event.
- O. Simonin co-chaired and co-organized the "IA & Robotics" french AFIA day, on 15/12/20, with Christophe Leroux (CEA-LIST). This virtualized conference welcomed 70 participants and 10 invited talks. url : https://
afia. asso. fr/ rob-ia20/
10.1.2 Scientific events: selection
Chair of conference program committees
- C. Solnon was chair of the track "Ant Colony Optimisation and Swarm Intelligence" of the Genetic and Evolutionary Computation Conference (GECCO).
Member of the conference program committees
- C. Solnon was senior PC member for the International Conference on Principles and Practice of Constraint Programming (CP).
- J. Dibangoye was PC member of International Joint Conference on Artificial Intelligence (IJCAI), AAAI Conference on Artificial Intelligence, European Conference on Artificial Intelligence (ECAI), International Conference on Machine Learning (ICML), Conference on Neural Information Processing Systems (NeurIPS).
- C. Solnon was PC member of International Joint Conference on Artificial Intelligence (IJCAI), AAAI Conference on Artificial Intelligence, European Conference on Artificial Intelligence (ECAI), International Conference on Parallel Problem Solving from Nature (PPSN) and International Conference on Machine Learning, Optimization, and Data Science (LOD).
- O. Simonin was Senior PC member of European Conference on Artificial Intelligence (ECAI).
- O. Simonin was PC member of International Joint Conference on Artificial Intelligence (IJCAI) and AAAI Conference on Artificial Intelligence.
- O. Simonin was PC member of the Genetic and Evolutionary Computation Conference (GECCO), ACO-SI Track.
- C. Laugier was Associate Editor for the following International Conferences: IEEE ICRA 2020, IEEE IROS 2020, IEEE IV 2020, IEEE ITSC 2020.
- C. Laugier was member of the Senior Programm Committee of IEEE IROS 2020. The committee meeting was done by videoconference.
- A. Martinelli is Associate Editor for conference IEEE ICRA 2021.
Reviewer
- Chroma researchers are regular reviewers of most of the major robotics conferences (ICRA, IROS, ..) and AI conferences (IJCAI, AAAI, AAMAS, ..).
10.1.3 Journal
Member of the editorial boards
- C. Solnon is member of the editorial boards of Annals of Mathematics and Artificial Intelligence (Springer), Constraints (Springer), and Swarm Intelligence (Springer)
- O. Simonin is member of the editorial board of ROIA (Revue Ouverte d’Intelligence Artificielle).
- C. Laugier is member of the Editorial Board of the journal IEEE Transactions on Intelligent Vehicles (Senior Editor). He is also member of the Steering Committee of the Journal.
- C. Laugier is member of the Editorial Board of the IEEE Journal Robomech.
Reviewer - reviewing activities
- O. Simonin was reviewer for Robotics and Autonomous Systems journal.
10.1.4 Invited talks
- C. Laugier has given 2 invited talks by video conference at respectively the French Coboteam workshop on "Robot Navigation" (May 28,2020) and at the Tornado Workshop on "Safety of Autonomous Vehicles" (November 5, 2020).
- C. Laugier has given a broadcasted invited talk at the IRT Nanoelec Annual Scientific event, Minatec Grenoble (September 8, 2020).
- F. Jumel was invited speaker at French Science Academic event, Lyon, February 14, 2020 "Intelligent robots".
10.1.5 Leadership within the scientific community
- C. Solnon is member of the executive committee and the scientific committee of the GDR IA (https://
www. gdria. fr/) - O. Simonin is member of the TIRREX EquipEx+ (2021-29) (shared national robotic platforms). He represents Chroma/CITI lab-Inria/INSA Lyon as major lab partner in the "Aerial Robot" axis.
- O. Simonin is member of the GDR Robotics (he chaired its bi-annual conference on automn 2019).
- O. Simonin was member of the excutive committee of AFIA, 2018-2020 (Association Française pour l'Intelligence Artificielle).
- C. Laugier is member of the Steering Committee of the IEEE/RSJ IROS conference.
- C. Laugier was member of the Advisory Committee of IEEE ICRA 2020 conference.
- C. Laugier is member of the Scientific Committee of the French GDR Robotics.
- C. Laugier was member of the Best Paper Award committee and of the Best Student Paper Award committee of IEEE ICARCV 2020.
10.1.6 Scientific expertise
- C. Solnon was vice-president of the Hcéres evaluation committee of the LIRMM (Montpellier)
10.1.7 Teaching
- INSA Lyon 5th year : O. Simonin, Resp. of the Robotics option (25 students): AI for Robotics, Multi-Robot Systems, Robotics Projects, 90h, M2, Telecom Dept., France.
- INSA Lyon 4th year : O. Simonin, Introduction to AI, 12h (90 students), L4, Telecom Dept., France.
- INSA Lyon 3rd year : O. Simonin, Resp. of Introduction to Algorithmics, 32h (100 students), L3, Telecom Dept., France.
- INSA Lyon 4th year : Jilles S. Dibangoye, Resp. of Introduction to AI, 20h (90 students), L4, Telecom Dept., France.
- INSA Lyon 3rd year : Jilles S. Dibangoye, Algorithmics, 24h, L3, Dept. Telecom INSA Lyon, France.
- INSA Lyon 3rd year : Jilles S. Dibangoye, WEB, 42h, L3, Dept. Telecom INSA Lyon, France.
- INSA Lyon 3rd year : Jilles S. Dibangoye, Operating Systems, 56h, L3, Dept. Telecom INSA Lyon, France.
- INSA Lyon 4rd year : Jilles S. Dibangoye, Operating Systems, 16h, Master, Dept. Telecom INSA Lyon, France.
- INSA Lyon 5th year : Jilles S. Dibangoye, the Robotics option : AI for Robotics, Robotics projects, 8h, M2, Dept. Telecom INSA Lyon, France.
- INSA Lyon 3rd year: C. Solnon, Resp. of Advanced algorithms for artificial intelligence and graphs, 40h (130 students), L3, Computer Science Dept.
- INSA Lyon 4th year: C. Solnon, Resp. of Object Oriented and Agile software development, 62h (130 students), M1, Computer Science Dept.
- INSA Lyon 5th year: C. Solnon, Resp. of Fundamental Computer Science, 20h (60 students), M2, Computer Science Dept.
- INSA Lyon 5th year: C. Solnon, Prescriptive Data Analytics, 6h (30 students), M2, Computer Science Dept.
- M2R MoSIG: A. Martinelli, Autonomous Robotics, 12h, ENSIMAG Grenoble.
- CPE Lyon 4-5th year : F. Jumel, resp. of the Robotics option, 400h M1/ M2, Dept. SN CPE Lyon France.
- CPE Lyon 4-5th year : F. Jumel, 250h (robotic vision, cognitive science, Interface robot machine, deeplearning, Robotic frameworks, robotic plateforms, Kalman Filter)
- CPE Lyon 4-5th year : J. Saraydaryan, resp. of the Software Design and Big Data option, 400h M1/ M2, Dept. SN CPE Lyon France.
- CPE Lyon 4-5th year : J. Saraydaryan, Software Architecture, 140h, M1/ M2, Dept. SN CPE Lyon France.
- CPE Lyon 4-5th year : J. Saraydaryan, Introduction to Cyber Security, 48h, M1/ M2, Dept. SN CPE Lyon France.
- CPE Lyon 5th year : J. Saraydaryan, Robotics Middleware, 16h, M2, Dept. SN CPE Lyon France.
- CPE Lyon 5th year : J. Saraydaryan, Particle Filter, 12h, M2, Dept. SN CPE Lyon France.
- CPE Lyon 5th year : J. Saraydaryan, Autonomous Robot Navigation, 18h, M2, Dept. SN CPE Lyon France.
10.1.8 Supervision
PhD defended in 2020 :
- Guillaume Bono, Apprentissage par Renforcement Profond Multi-agent pour les Problèmes de Planification de Tournées Dynamiques et Stochastiques, O. Simonin, J. Dibangoye, L. Matignon. Defended on oct. 28, 2020.
- Remy Grunblatt, Mobilité contrôlée dans les réseaux de drones autonomes, defended on 8/01/2021, I. Guerrin-Lassous (Inria Dante) and O. Simonin (Inria Chroma), Inria/DGA funding.
Phd in progress :
- Maria Kabtoul, Proactive Navigation in dense crowds, A. Spalanzani and P. Martinet (Inria Chorale).
- Benoit Renault, Navigation coopérative et sociale de robots mobiles en environnement modifiable, O. Simonin and J. Saraydaryan.
- Edward Beeching, Large-scale automatic learning of autonomous agent behavior with structured deep reinforcement learning, C. Wolf, O. Simonin and J. Dibangoye.
- Manon Prédhumeau, Crowd simulation and autonomous vehicle, A. Spalanzani and J. Dugdale (LIG).
- Luiz Serafim-Guardini, Conduite Automobile Autonome : Utilisation de grilles d'occupation probabilistes dynamiques pour la planification contextualisée de trajectoire d'urgence à criticité minimale, A. Spalanzani, C. Laugier, P. Martinet (Inria Chorale).
- Alexandre Bonnefond, Large-scale automatic learning of autonomous agent behavior with structured deep reinforcement learning, O. Simonin and I. Guerrin-Lassous (Inria Dante).
- Mihai Popescu, Planification multi-agents pour la patrouille d'un réseau de cibles découvertes dynamiquement, O. Simonin, A. Spalanzani, F. Valois (CITI/Inria Agora) (on break in 2020).
- Estéban Carvalho, Safe and aggressive piloting of UAVs, 2019, Ahmad Hably (Gipsa-Lab), Nicolas Marchand (Gipsa-Lab), Jilles S. Dibangoye.
- Xiao Peng, Planification sous contraintes d’une flotte de robots mobiles pour des tâches de couverture spatiale, O. Simonin, C. Solnon, European H2020 BugWright2 project funding.
- Pierre Marza, Apprentissage large-échelle d'agents autonomes capables de naviguer dans des environnements réels, C. Wolf, L. Matignon, O. Simonin, funded by Chaire IA "REMEMBER".
- Loic Rouquette, Programmation par contraintes pour la cryptanalyse symétrique, M. Minier (LORIA) and C. Solnon
- Romain Fontaine, Optimisation de la mobilité en ville en tenant compte des conditions de traffic, J. Dibangoye and C. Solnon
10.1.9 Juries
HDR :
- O. Simonin was reviewer and chair of the defense committee of the HDR of Benoit Piranda, Université de Franche-Comté, "Designing and Programming Lattice-Based Modular Robots for Creating Programmable Matter", 12/11/2020.
PhD thesis :
- A. Spalanzani was reviewer and member of the defense committee of the PhD thesis of José Mendes Filho, école nationale supérieure de techniques avancées, December 19th, 2019.
- O. Simonin was reviewer and member of the defense committee of the PhD thesis of Florien Pescher, Université Franche-Comté, December 18th, 2020.
- O. Simonin was member of the defense committee of the PhD thesis of Titouan Verdu, Univ. Toulouse - INSA Toulouse, December 9th, 2020.
- O. Simonin was member of the defense committee of the PhD thesis of Soufian Toufga, Université Toulouse 3, October 29th, 2020.
- O. Simonin was member of the defense committee of the PhD thesis of Alexandre Schmitt, Université Le Mans, October 19th, 2020.
- O. Simonin was reviewer and member of the defense committee of the PhD thesis of François Suro, Université Montpellier 2 - LIRMM, September 17th, 2020.
- C. Solnon was member of the defense committee of the PhD thesis of Adriana Pacheco, Université de Toulouse, December 17th 2020
- C. Solnon was reviewer and member of the defense committee of the PhD thesis of Dimitri Justeau-Allaire, Université de Montpellier, December 16th, 2020
- C. Solnon was president and member of the defense committee of the PhD thesis of Léo Gautheron, Université Jean Monet Saint-Etienne, December 8th, 2020
- C. Solnon was member of the defense committee of the PhD thesis of Flavien Lucas, Université Bretagne Sud, November 19th, 2020
- C. Solnon was reviewer and member of the defense committee of Ruixin Wang, Université de Toulouse, September 17th, 2020
- C. Solnon was member of the defense committee of the PhD thesis of Luc Libralesso, UGA Grenoble, July 24th, 2020
- C. Solnon was president and member of the defense committee of the PhD thesis of Humberto Gonzales, ENTPE Lyon, May 13th, 2020
- C. Solnon was president and member of the defense committee of the PhD thesis of Mohammed-Bachir Belaid, Université de Montpellier, January 8th, 2020
- C. Laugier was member of the defense committee of the PhD thesis of Wei chen, University Paris Saclay, September 2020.
- C. Laugier was member of the second year PhD Thesis Committee of Johan Laconte, Institut Pascal Clermond-Ferrand, September 2020.
10.2 Popularization
10.2.1 Internal or external Inria responsibilities
- O. Simonin is member of the Auvergne-Rhone-Alpes Robotics cluster (Coboteam), for Inria and INSA Lyon entities.
- O. Simonin is member of the Scientific Council of the Digital League (Auvergne-Rhone-Alpes).
- F. Jumel is member of the organisation committee of the International Robocup competition, 2020-2021, Bordeaux.
- F. Jumel is member of the organisation committee Worldskills France, 2020-2021, Mobile robotics.
10.2.2 Articles and contents
- C. Solnon has written an article on "Ethics of algorithms" for the bulletin de la société française de recherche opérationnelle et aide à la décision 54 (https://
www. roadef. org/ bulletins/ bulletinNo42. pdf)
10.2.3 Interventions
- C. Solnon has given a 2 hour tutorial on Artificial Intelligence to prepare students of the Faculté de droit de l’Ucly for a fictive trial on consequences of Artificial Intelligence.
11 Scientific production
11.1 Major publications
- 1 articleSolving Multi-Agent Routing Problems Using Deep Attention MechanismsIEEE Transactions on Intelligent Transportation SystemsJuly 2020, 1-10
- 2 incollection Handbook of Robotics 2nd edition, Chapter 62 on ''Intelligent Vehicles'' Handbook of Robotics 2nd Edition Springer Verlag July 2016
- 3 articleOptimally Solving Dec-POMDPs as Continuous-State MDPsJournal of Artificial Intelligence Research55February 2016, 443-497
- 4 inproceedingsSemantic Grid Estimation with a Hybrid Bayesian and Deep Neural Network ApproachIROS 2018 - IEEE/RSJ International Conference on Intelligent Robots and SystemsMadrid, SpainIEEEOctober 2018, 1-8
- 5 inproceedingsTowards Proactive Navigation: A Pedestrian-Vehicle Cooperation Based Behavioral Model2020 IEEE International Conference on Robotics and Automation (ICRA)IEEE International Conference on Robotics and Automation ProceedingsParis, FranceMay 2020, 6958-6964
- 6 articleCooperative Visual-Inertial Odometry: Analysis of Singularities, Degeneracies and Minimal CasesIEEE Robotics and Automation Letters522020, 668 - 675
- 7 article A Common Optimization Framework for Multi-Robot Exploration and Coverage in 3D Environments Journal of Intelligent and Robotic Systems 2020
- 8 articleOn the impact of spatio-temporal granularity of traffic conditions on the quality of pickup and delivery optimal toursTransportation Research Part E: Logistics and Transportation Review1422020, 102085
- 9 article From Proxemics Theory to Socially-Aware Navigation: A Survey International Journal of Social Robotics April 2015
- 10 inproceedings Interaction-Aware Driver Maneuver Inference in Highways Using Realistic Driver Models Proceedings of the 2017 IEEE 20th International Conference on Intelligent Transportation Systems (ITSC 2017) Yokohama, Japan October 2017
11.2 Publications of the year
International journals
International peer-reviewed conferences
National peer-reviewed Conferences
Scientific books
Scientific book chapters
Doctoral dissertations and habilitation theses
Reports & preprints
Other scientific publications
11.3 Other
Scientific popularization
11.4 Cited publications
- 55 inproceedingsThreat assessment design for driver assistance system at intersectionsIntelligent Transportation Systems (ITSC), 2010 13th International IEEE Conference onsept. 2010, 1855 -1862
- 56 book Dynamic Programming Dover Publications, Incorporated 1957
- 57 inproceedings 3 Known Landmarks are Enough for Solving Planar Bearing SLAM and Fully Reconstruct Unknown Inputs International Conference on Intelligent Robots and Systems (IROS) IEEE December 2010
- 58 articleLearning motion patterns of people for compliant robot motionInt. J. of Robot. Res.242005, 31--48
- 59 inproceedings SDfR -Service discovery for multi-robot systems ICAART 2016 The 8th International Conference on Agents and Artificial Intelligence Rome, Italy February 2016
- 60 inproceedings Towards semantic SLAM using a monocular camera IROS 2011
- 61 articleBayesian Occupancy Filtering for Multi-Target Tracking: an Automotive ApplicationInt. Journal of Robotics Research251January 2006, 19--30
- 62 inproceedingsExploiting Separability in Multiagent Planning with Continuous-State MDPs (Extended Abstract)24th International Joint Conference on Artificial Intelligence (IJCAI)2015, 4254--4260
- 63 articleOptimally Solving Dec-POMDPs as Continuous-State MDPsJournal of Artificial Intelligence Research55February 2016, 443-497
- 64 articleBayesian Human Motion Intentionality Prediction in urban environmentsPattern Recognition Letters440Pattern Recognition and Crowd Analysis2014, 134 - 140URL: http://www.sciencedirect.com/science/article/pii/S0167865513003176
- 65 inproceedings SVO: Fast Semi-Direct Monocular Visual Odometry ICRA 2014
- 66 inproceedingsProbabilistic navigation in dynamic environment using Rapidly-exploring Random Trees and Gaussian ProcessesIEEE/RSJ 2008 International Conference on Intelligent RObots and SystemsFrance Nice2008, URL: http://hal.inria.fr/inria-00332595/en/
- 67 inproceedingsSimulation and Performance Evaluation of the Intel Rate Adaptation AlgorithmMSWiM 2019 - 22nd ACM International Conference on Modeling, Analysis and Simulation of Wireless and Mobile SystemsMiami Beach, United StatesACMNovember 2019, 27-34
- 68 articleConsistency analysis and improvement of vision-aided inertial navigationTrans. on Rob.3012014, 158--176
- 69 inproceedings Probabilistic Decision Making for Collision Avoidance Systems: Postponing Decisions IEEE/RSJ International Conference on Intelligent Robots and Systems Tokyo, Japan 2013
- 70 article Keyframe-based visual-inertial odometry using nonlinear optimization Int. J. of Rob. Res. 2014
- 71 articleClosed-form solution of visual-inertial structure from motionInternational Journal of Computer Vision1062January 2014, 138--152
- 72 articleObservabilty Properties and Deterministic Algorithms in Visual-Inertial Structure from MotionFoundations and Trends in Robotics (FnTROB)December 2013, 1--75
- 73 article Cooperative Visual-Inertial Sensor Fusion: the Analytic Solution IEEE Robotics and Automation Letters, in Press 4 2 2019
- 74 article Cooperative Visual-Inertial Sensor Fusion: Fundamental Equations and State Determination in Closed-Form Autonomous Robots 2019
- 75 articleState Estimation based on the Concept of Continuous Symmetry and Observability Analysis: The Case of CalibrationIEEE Transactions on RoboticsMay 2011, URL: http://hal.inria.fr/hal-00578795/en
- 76 article Vision and IMU Data Fusion: Closed-Form Solutions for Attitude, Speed, Absolute Scale and Bias Determination Transaction on Robotics 28 1 2012
- 77 inproceedingsMulti-Robot Simultaneous Coverage and Mapping of Complex Scene - Comparison of Different StrategiesAAMAS 2018 - 17th International Conference on Autonomous Agents and Multiagent Systems - Robotics TrackStockholm, SwedenACMJuly 2018, 559-567
- 78 inproceedings Hybrid Sampling Bayesian Occupancy Filter IEEE Intelligent Vehicles Symposium (IV) Dearborn, United States June 2014
- 79 inproceedingsA framework towards a socially aware Mobile Robot motion in Human-Centered dynamic environmentIEEE/RSJ International Conference on Intelligent Robots and Systemsoct. 2010, 5855 -5860
- 80 inproceedings Multi-robot Patrolling in Wireless Sensor Networks using Bounded Cycle Coverage ICTAI 2016 28th International Conference on Tools with Artificial Intelligence IEEE San Jose, United States November 2016
- 81 inproceedings Combining Stochastic Optimization and Frontiers for Aerial Multi-Robot Exploration of 3D Terrains IROS 2019 - IEEE/RSJ International Conference on Intelligent Robots and Systems Macau, China November 2019
- 82 inproceedings Conditional Monte Carlo Dense Occupancy Tracker 18th IEEE International Conference on Intelligent Transportation Systems Las Palmas, Spain September 2015
- 83 articleRevisiting wavefront construction with collective agents: an approach to foragingSwarm Intelligence82June 2014, 113-138
- 84 incollectionRisk Based Navigation DecisionsHandbook of Intelligent Vehicles1Springer VerlagFebruary 2012, URL: http://hal.inria.fr/hal-00743336
- 85 inproceedings CNN-SLAM: Real-time dense monocular SLAM with learned depth prediction CVPR 2017
- 86 bookProbabilistic RoboticsThe MIT PressSeptember 2005, 1
- 87 inproceedingsStop-Free Strategies for Traffic Networks: Decentralized On-line OptimizationECAI 2014 - 21st European Conference on Artificial Intelligence, 18-22 August 2014, Prague, Czech Republic - Including Prestigious Applications of Intelligent Systems (PAIS 2014)2014, 1191--1196URL: http://dx.doi.org/10.3233/978-1-61499-419-0-1191
- 88 inproceedings An open framework for human-like autonomous driving using Inverse Reinforcement Learning IEEE Vehicle Power and Propulsion Conference 2014
- 89 book Theory of Games and Economic Behavior Princeton University Press 1944
- 90 book Theory of games and economic behavior Princeton University Press 2007